Introduction #
The authors of the Aerial Power Infrastructure Detection Dataset explore the application of Unmanned Aerial Vehicles (UAVs) in autonomously inspecting power networks. They acknowledge the rapid progress in UAV technology and emphasize the need for efficient data acquisition in order to navigate power networks and acquire high-resolution data safely and swiftly. The dataset aims to aid the research community in training online detection models, thereby assisting UAV localization within the network.
The dataset contains top-view images of Medium Voltage (MV) poles captured across various locations in Cyprus. The images encompass different seasons, background conditions, and heights to ensure versatility. Annotations were converted into formats suitable for various frameworks. The dataset aids the detection of objects known as “t-bars,” which represent poles.
Their specific focus is on the “Pole Detection” process within the ICARUS toolkit. The ICARUS is a UAV-based platform that combines UAV technology and deep learning algorithms to automate power infrastructure inspection, including data acquisition and analysis. The system employs multiple sensors and advanced navigation techniques to minimize errors and accurately identify poles. The UAV employs known pole coordinates and detects poles using a one-class detection model, correcting its position to align directly above the pole for accurate inspection.
Authors utilize commercially available UAV equipment such as DJI Matrice 300 RTK, equipped with cameras and multispectral sensors. The NVIDIA Jetson Xavier NX embedded platform enables real-time deep learning and navigation algorithms.
In the inspection procedure, the UAV autonomously takes off, navigates a predefined path, collects data, and returns to the starting point. Pole locations can be supplied with a certain tolerance. The “Pole Detection” task involves using tiny-You-Only-Look-Once (tiny-YOLO) v4 to identify poles in top-view images from various conditions and seasons.
The authors’ work seeks to enhance the efficiency and accuracy of power network inspection through UAV technology, deep learning, and advanced data acquisition techniques.
Summary #
Aerial Power Infrastructure Detection Dataset v2.2 is a dataset for an object detection task. It is used in the energy industry.
The dataset consists of 13831 images with 14919 labeled objects belonging to 1 single class (t-bar).
Images in the Aerial Power Infrastructure dataset have bounding box annotations. There are 41 (0% of the total) unlabeled images (i.e. without annotations). There are 3 splits in the dataset: train (10012 images), valid (2370 images), and test (1449 images). The dataset was released in 2023 by the KIOS Research and Innovation Center of Excellence, University of Cyprus.
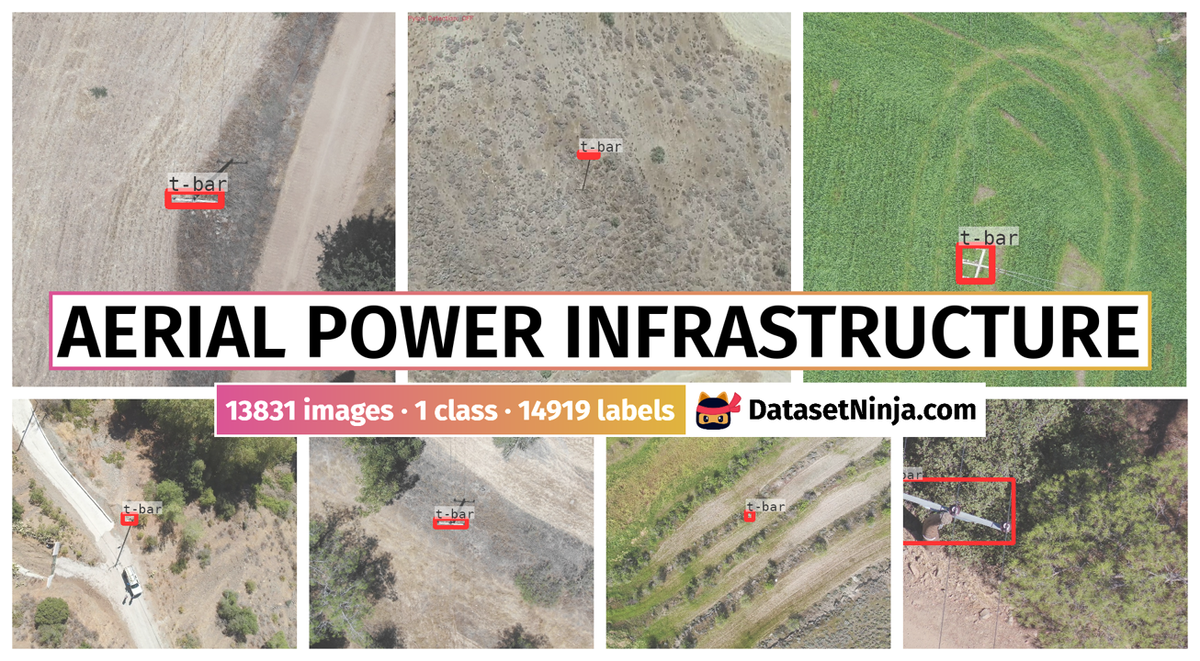
Explore #
Aerial Power Infrastructure dataset has 13831 images. Click on one of the examples below or open "Explore" tool anytime you need to view dataset images with annotations. This tool has extended visualization capabilities like zoom, translation, objects table, custom filters and more. Hover the mouse over the images to hide or show annotations.
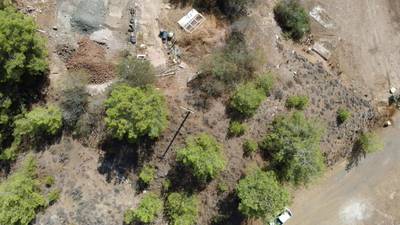



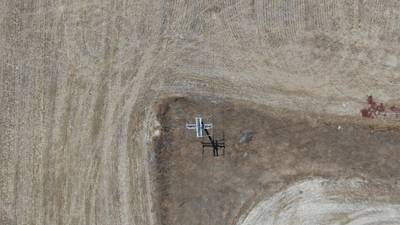
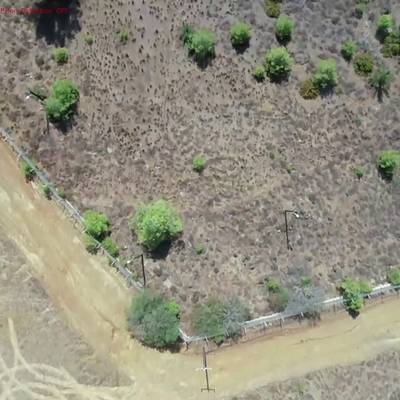
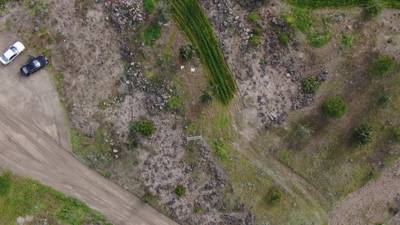

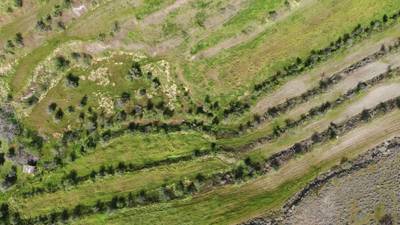
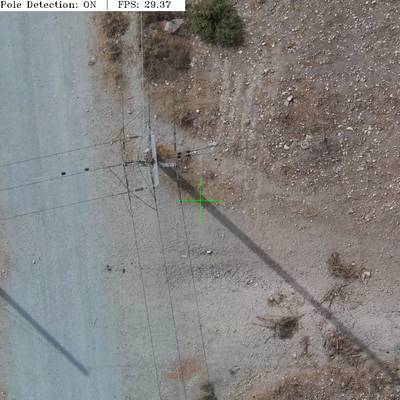
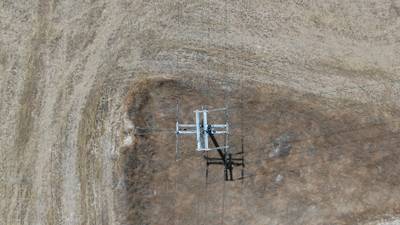

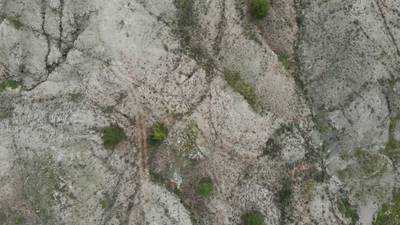
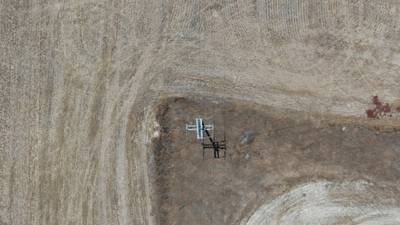

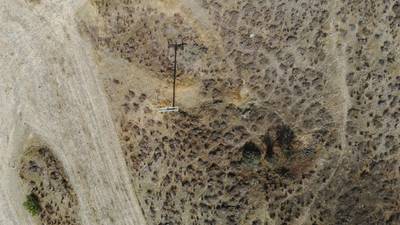
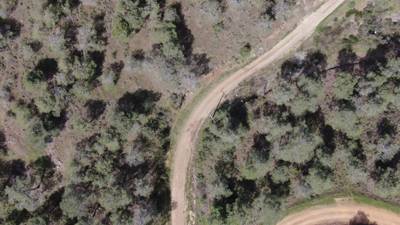
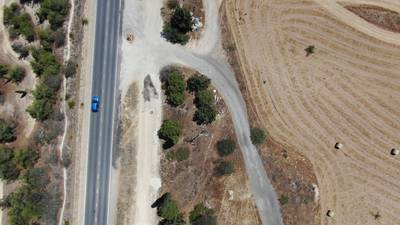
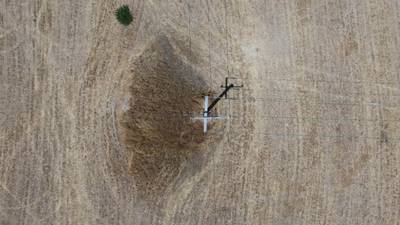
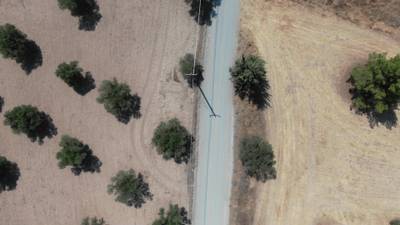
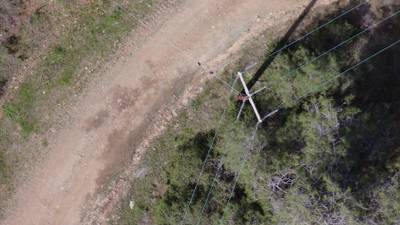
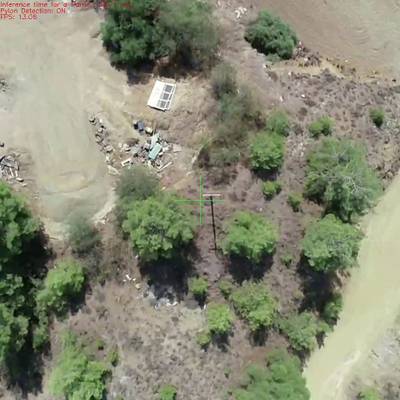

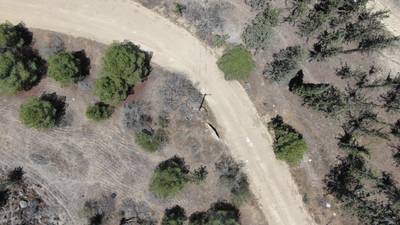

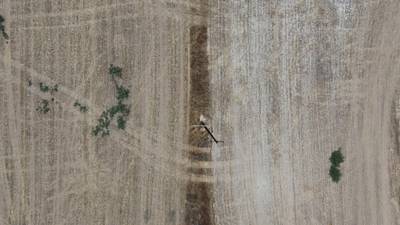
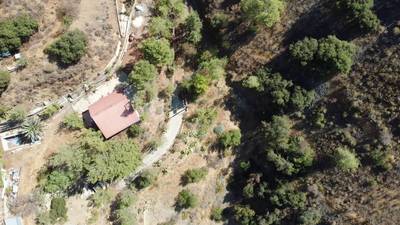

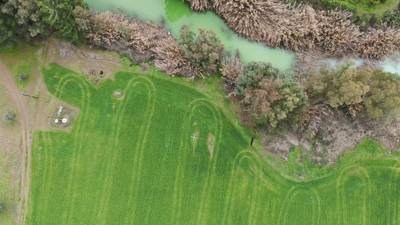
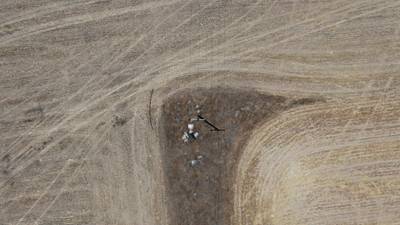
Class balance #
There are 1 annotation classes in the dataset. Find the general statistics and balances for every class in the table below. Click any row to preview images that have labels of the selected class. Sort by column to find the most rare or prevalent classes.
Class ㅤ | Images ㅤ | Objects ㅤ | Count on image average | Area on image average |
---|---|---|---|---|
t-bar➔ rectangle | 13790 | 14919 | 1.08 | 0.63% |
Images #
Explore every single image in the dataset with respect to the number of annotations of each class it has. Click a row to preview selected image. Sort by any column to find anomalies and edge cases. Use horizontal scroll if the table has many columns for a large number of classes in the dataset.
Object distribution #
Interactive heatmap chart for every class with object distribution shows how many images are in the dataset with a certain number of objects of a specific class. Users can click cell and see the list of all corresponding images.
Class sizes #
The table below gives various size properties of objects for every class. Click a row to see the image with annotations of the selected class. Sort columns to find classes with the smallest or largest objects or understand the size differences between classes.
Class | Object count | Avg area | Max area | Min area | Min height | Min height | Max height | Max height | Avg height | Avg height | Min width | Min width | Max width | Max width |
---|---|---|---|---|---|---|---|---|---|---|---|---|---|---|
t-bar rectangle | 14919 | 0.59% | 39.57% | 0.01% | 4px | 0.37% | 1023px | 94.72% | 49px | 5.73% | 11px | 0.57% | 802px | 61.58% |
Spatial Heatmap #
The heatmaps below give the spatial distributions of all objects for every class. These visualizations provide insights into the most probable and rare object locations on the image. It helps analyze objects' placements in a dataset.
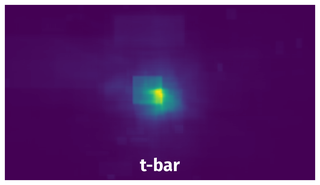
Objects #
Table contains all 14919 objects. Click a row to preview an image with annotations, and use search or pagination to navigate. Sort columns to find outliers in the dataset.
Object ID ㅤ | Class ㅤ | Image name click row to open | Image size height x width | Height ㅤ | Height ㅤ | Width ㅤ | Width ㅤ | Area ㅤ |
---|---|---|---|---|---|---|---|---|
1➔ | t-bar rectangle | 5712_2211_det_img_1490.jpg | 720 x 1280 | 17px | 2.36% | 44px | 3.44% | 0.08% |
2➔ | t-bar rectangle | 1195_1373_pylon_720.jpg | 1080 x 1920 | 36px | 3.33% | 31px | 1.61% | 0.05% |
3➔ | t-bar rectangle | 239_3460_X4S_t2_310820_n248.jpg | 960 x 960 | 23px | 2.4% | 49px | 5.1% | 0.12% |
4➔ | t-bar rectangle | 7788_6181_det_img_1620.jpg | 720 x 1280 | 114px | 15.83% | 98px | 7.66% | 1.21% |
5➔ | t-bar rectangle | 7431_2510_det_img_80.jpg | 720 x 1280 | 68px | 9.44% | 53px | 4.14% | 0.39% |
6➔ | t-bar rectangle | 3073_4274_det_img_370.jpg | 720 x 1280 | 40px | 5.56% | 31px | 2.42% | 0.13% |
7➔ | t-bar rectangle | 6355_527_det_img_180.jpg | 720 x 1280 | 37px | 5.14% | 50px | 3.91% | 0.2% |
8➔ | t-bar rectangle | 3490_5478_X4S_t2_310820_n49.jpg | 960 x 960 | 16px | 1.67% | 46px | 4.79% | 0.08% |
9➔ | t-bar rectangle | 8590_MainCamera_Flight_1665559775_frame_00074.png | 1088 x 1088 | 25px | 2.3% | 37px | 3.4% | 0.08% |
10➔ | t-bar rectangle | 8469_6314_det_img_700.jpg | 720 x 1280 | 30px | 4.17% | 31px | 2.42% | 0.1% |
License #
Citation #
If you make use of the Aerial Power Infrastructure data, please cite the following reference:
Antonis Savva, Rafael Makrigiorgis, Panayiotis Kolios, & Christos Kyrkou. (2022). Aerial Power Infrastructure Detection Dataset (2.1) [Data set]. Zenodo. https://doi.org/10.5281/zenodo.718131
@dataset{antonis_savva_2023_7781388,
author = {Antonis Savva and
Rafael Makrigiorgis and
Panayiotis Kolios and
Christos Kyrkou},
title = {Aerial Power Infrastructure Detection Dataset},
month = mar,
year = 2023,
publisher = {Zenodo},
version = {2.2},
doi = {10.5281/zenodo.7781388},
url = {https://doi.org/10.5281/zenodo.7781388}
}
If you are happy with Dataset Ninja and use provided visualizations and tools in your work, please cite us:
@misc{ visualization-tools-for-aerial-power-dataset,
title = { Visualization Tools for Aerial Power Infrastructure Dataset },
type = { Computer Vision Tools },
author = { Dataset Ninja },
howpublished = { \url{ https://datasetninja.com/aerial-power } },
url = { https://datasetninja.com/aerial-power },
journal = { Dataset Ninja },
publisher = { Dataset Ninja },
year = { 2025 },
month = { apr },
note = { visited on 2025-04-02 },
}
Download #
Dataset Aerial Power Infrastructure can be downloaded in Supervisely format:
As an alternative, it can be downloaded with dataset-tools package:
pip install --upgrade dataset-tools
… using following python code:
import dataset_tools as dtools
dtools.download(dataset='Aerial Power Infrastructure', dst_dir='~/dataset-ninja/')
Make sure not to overlook the python code example available on the Supervisely Developer Portal. It will give you a clear idea of how to effortlessly work with the downloaded dataset.
The data in original format can be downloaded here:
Disclaimer #
Our gal from the legal dep told us we need to post this:
Dataset Ninja provides visualizations and statistics for some datasets that can be found online and can be downloaded by general audience. Dataset Ninja is not a dataset hosting platform and can only be used for informational purposes. The platform does not claim any rights for the original content, including images, videos, annotations and descriptions. Joint publishing is prohibited.
You take full responsibility when you use datasets presented at Dataset Ninja, as well as other information, including visualizations and statistics we provide. You are in charge of compliance with any dataset license and all other permissions. You are required to navigate datasets homepage and make sure that you can use it. In case of any questions, get in touch with us at hello@datasetninja.com.