Introduction #
Based upon the autonomous driving dataset Argoverse 1.1, the authors build their dataset with high-frame-rate annotations for streaming evaluation that they name Argoverse-HD (High-frame-rate Detection). Despite being created for streaming evaluation, Argoverse-HD can also be used for study on image/video object detection, multi-object tracking, and forecasting.
Motivation
Embodied perception entails an autonomous agent’s capacity to perceive and respond to its environment effectively. An essential factor influencing the agent’s responsiveness is its reaction time. In practical scenarios like self-driving vehicles or augmented/virtual reality (AR/VR), achieving reaction times comparable to humans, typically around 200 milliseconds (ms) for visual stimuli, is crucial. In these contexts, the deployment of low-latency algorithms is imperative to ensure safe operation or provide a truly immersive experience. Historically, the computer vision community has not prioritized algorithmic latency, leading to the development of a diverse range of techniques for robotic vision, often explored in offline settings. However, vision-for-online-perception introduces distinct latency demands; by the time an algorithm completes processing a frame, say, after 200ms, the surrounding environment has already changed. Consequently, perception must inherently incorporate predictive elements to anticipate future events—an aspect mirrored in human vision, such as when a baseball player predicts the trajectory of a fast ball. Thus, the realm of streaming perception holds significance for researchers across the broader computer vision domain.
Latency is inevitable in a real-world perception system. The system takes a snapshot of the world at t1 (the car is at location A), and when the algorithm finishes processing this observation, the surrounding world has already changed at t2 (the car is now at location B, and thus there is a mismatch between prediction A and ground truth B).
Dataset description
The authors extend the publicly available video dataset Argoverse 1.1 with their annotations for streaming evaluation, which was named Argoverse-HD (High-framerate Detection). It contains diverse urban outdoor scenes from two US cities. The authors select Argoverse for its embodied setting (autonomous driving) and its high frame rate sensor data (30 FPS). We focus on the task of 2D object detection for our streaming evaluation. While Argoverse has multiple sensors, they only use the center RGB camera for simplicity. The authors collect annotations since the dataset does not provide dense 2D annotations. For the annotations, the authors follow MS COCO class definitions and format. They use only a subset of 8 classes (from 80 MS COCO classes) that are directly relevant to autonomous driving: person, bicycle, car, motorcycle, bus, truck, traffic light, and stop sign. This definition allows them to evaluate off-the-shelf models trained on MS COCO. No training is involved in the following experiments unless otherwise specified. All numbers are computed on the validation set, which contains 24 videos ranging from 15–30 seconds each (the total number of frames is 15k).
Comparison between Argoverse-HD and MS COCO. Top shows an example image from Argoverse-HD, overlaid with our dense 2D annotation (at 30 FPS). Bottom presents results of Mask R-CNN (ResNet 50) evaluated on the two datasets.
Summary #
Argoverse-HD is a dataset for object detection and identification tasks. It is used in the automotive industry.
The dataset consists of 66953 images with 1025715 labeled objects belonging to 8 different classes including car, truck, person, and other: traffic light, bus, stop sign, bicycle, and motorcycle.
Images in the Argoverse HD dataset have bounding box annotations. There are 12526 (19% of the total) unlabeled images (i.e. without annotations). There are 3 splits in the dataset: train (39384 images), val (15062 images), and test (12507 images). Additionally, every image contains information about its sequence. Labels contain information about track. Explore it in supervisely labeling tool. The dataset was released in 2021 by the Carnegie Mellon University, USA.
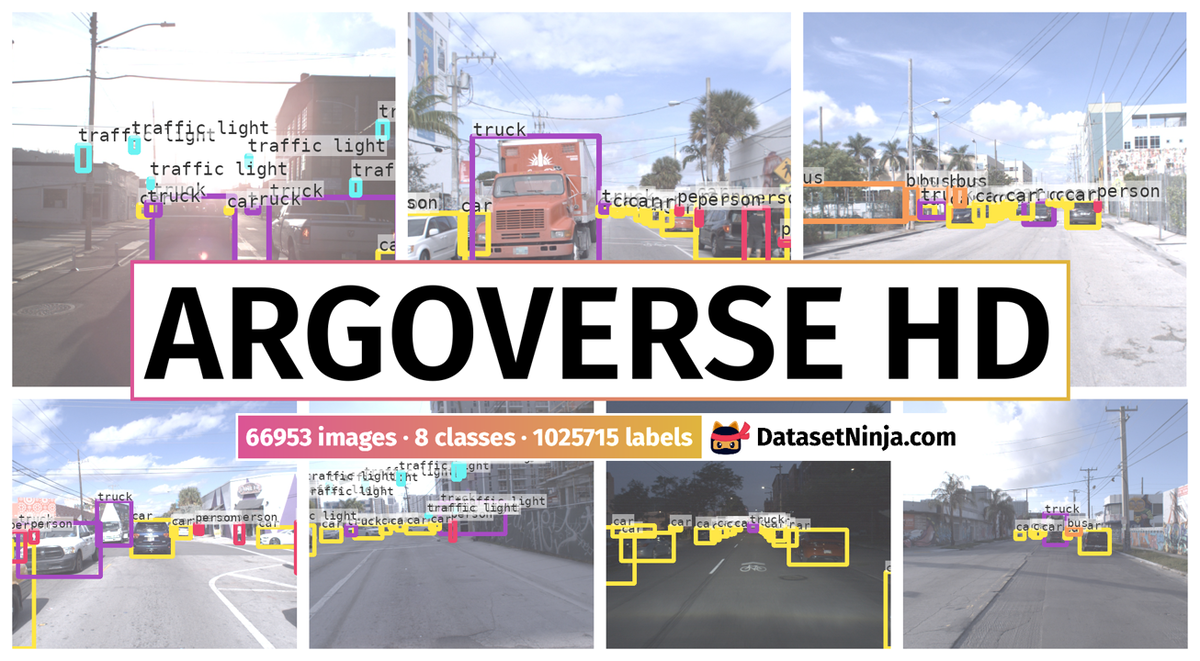
Explore #
Argoverse HD dataset has 66953 images. Click on one of the examples below or open "Explore" tool anytime you need to view dataset images with annotations. This tool has extended visualization capabilities like zoom, translation, objects table, custom filters and more. Hover the mouse over the images to hide or show annotations.
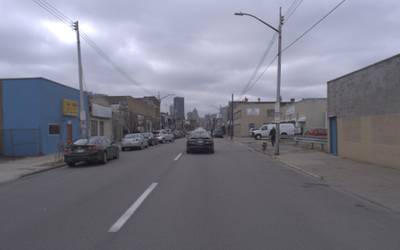
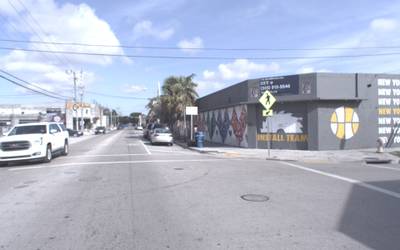
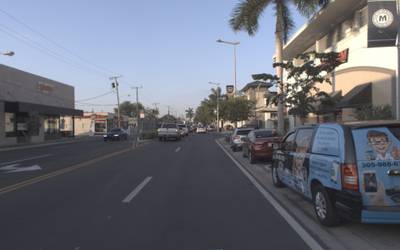
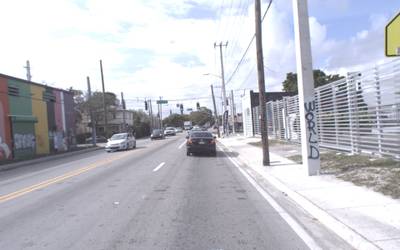
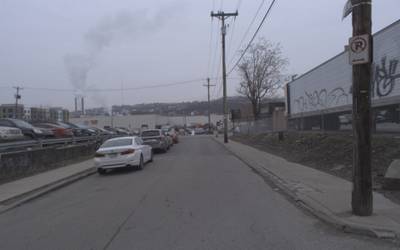
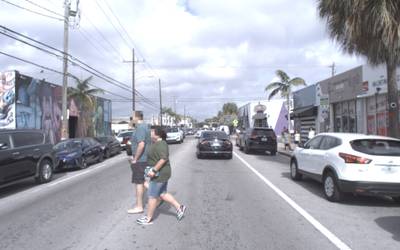

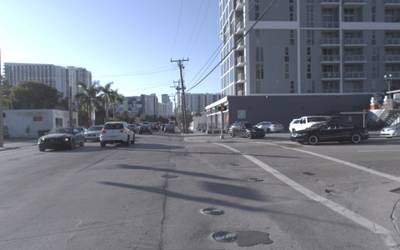
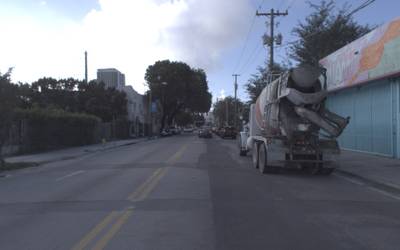
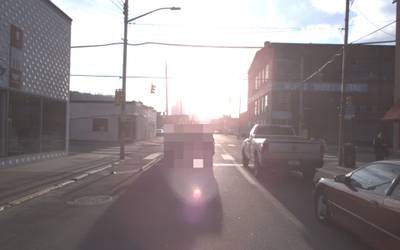
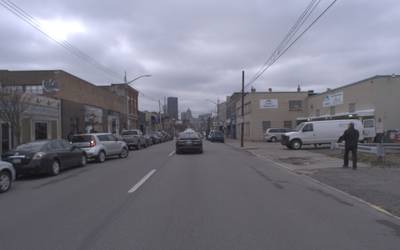
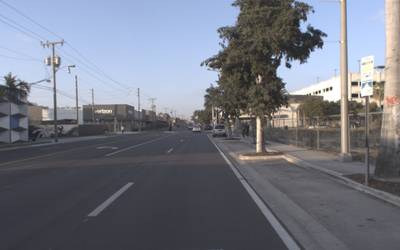
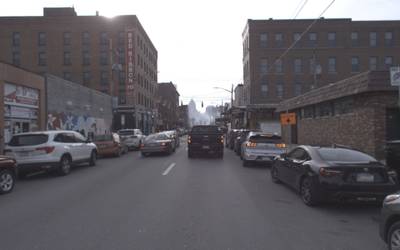
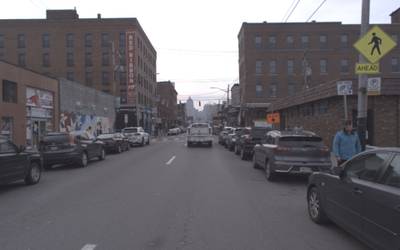
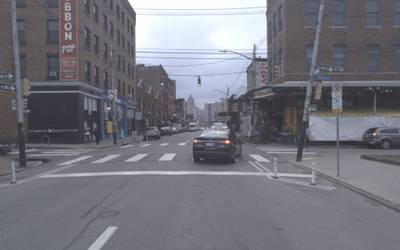
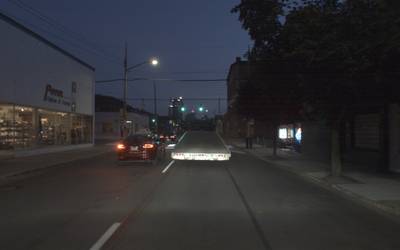
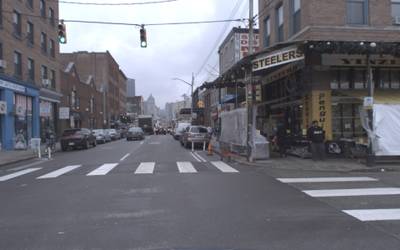
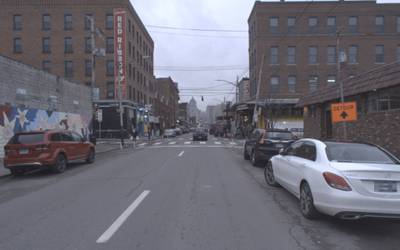

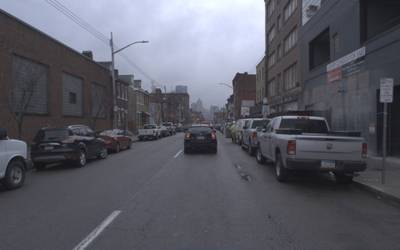
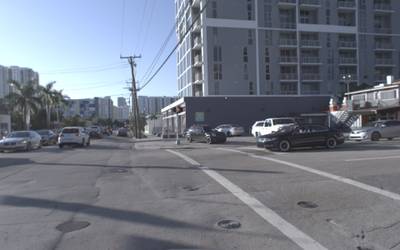
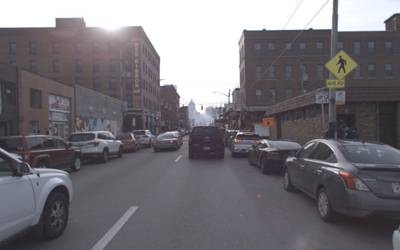
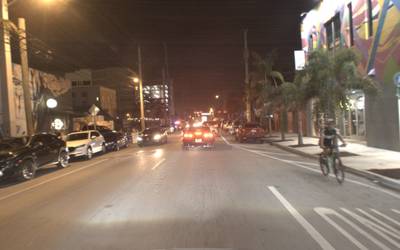
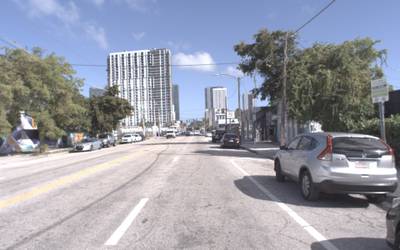
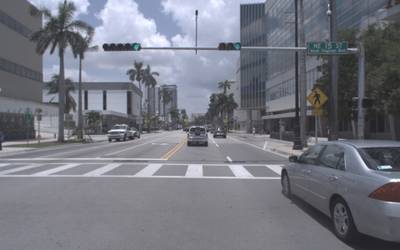
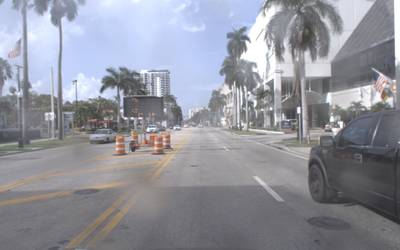
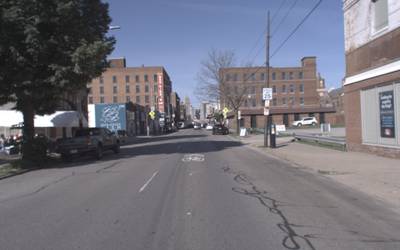
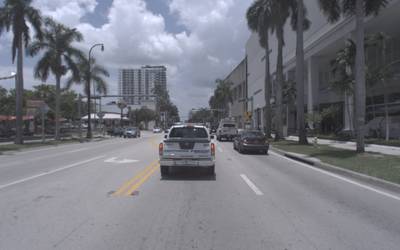
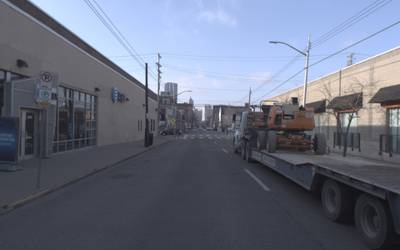
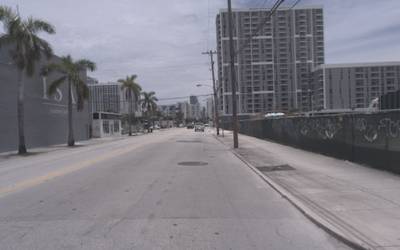
Class balance #
There are 8 annotation classes in the dataset. Find the general statistics and balances for every class in the table below. Click any row to preview images that have labels of the selected class. Sort by column to find the most rare or prevalent classes.
Class ã…¤ | Images ã…¤ | Objects ã…¤ | Count on image average | Area on image average |
---|---|---|---|---|
carâž” rectangle | 54117 | 633012 | 11.7 | 7.27% |
truckâž” rectangle | 42881 | 95795 | 2.23 | 3.15% |
personâž” rectangle | 32767 | 122634 | 3.74 | 0.89% |
traffic lightâž” rectangle | 21869 | 134255 | 6.14 | 0.37% |
busâž” rectangle | 9664 | 17980 | 1.86 | 10.37% |
stop signâž” rectangle | 6571 | 7378 | 1.12 | 0.09% |
bicycleâž” rectangle | 4902 | 9522 | 1.94 | 0.67% |
motorcycleâž” rectangle | 4663 | 5139 | 1.1 | 0.43% |
Co-occurrence matrix #
Co-occurrence matrix is an extremely valuable tool that shows you the images for every pair of classes: how many images have objects of both classes at the same time. If you click any cell, you will see those images. We added the tooltip with an explanation for every cell for your convenience, just hover the mouse over a cell to preview the description.
Images #
Explore every single image in the dataset with respect to the number of annotations of each class it has. Click a row to preview selected image. Sort by any column to find anomalies and edge cases. Use horizontal scroll if the table has many columns for a large number of classes in the dataset.
Object distribution #
Interactive heatmap chart for every class with object distribution shows how many images are in the dataset with a certain number of objects of a specific class. Users can click cell and see the list of all corresponding images.
Class sizes #
The table below gives various size properties of objects for every class. Click a row to see the image with annotations of the selected class. Sort columns to find classes with the smallest or largest objects or understand the size differences between classes.
Class | Object count | Avg area | Max area | Min area | Min height | Min height | Max height | Max height | Avg height | Avg height | Min width | Min width | Max width | Max width |
---|---|---|---|---|---|---|---|---|---|---|---|---|---|---|
car rectangle | 633012 | 0.68% | 25.37% | 0% | 2px | 0.17% | 757px | 63.08% | 72px | 6.02% | 2px | 0.1% | 983px | 51.2% |
traffic light rectangle | 134255 | 0.06% | 1.35% | 0% | 2px | 0.17% | 221px | 18.42% | 41px | 3.44% | 2px | 0.1% | 286px | 14.9% |
person rectangle | 122634 | 0.26% | 11.99% | 0% | 3px | 0.25% | 752px | 62.67% | 85px | 7.12% | 2px | 0.1% | 516px | 26.88% |
truck rectangle | 95795 | 1.44% | 40.05% | 0% | 5px | 0.42% | 1200px | 100% | 118px | 9.81% | 1px | 0.05% | 960px | 50% |
bus rectangle | 17980 | 5.63% | 40.78% | 0% | 9px | 0.75% | 1200px | 100% | 254px | 21.15% | 6px | 0.31% | 1208px | 62.92% |
bicycle rectangle | 9522 | 0.35% | 6.62% | 0% | 3px | 0.25% | 437px | 36.42% | 74px | 6.16% | 3px | 0.16% | 410px | 21.35% |
stop sign rectangle | 7378 | 0.08% | 2.28% | 0% | 9px | 0.75% | 268px | 22.33% | 35px | 2.95% | 5px | 0.26% | 239px | 12.45% |
motorcycle rectangle | 5139 | 0.39% | 11.08% | 0% | 3px | 0.25% | 512px | 42.67% | 72px | 5.98% | 3px | 0.16% | 520px | 27.08% |
Spatial Heatmap #
The heatmaps below give the spatial distributions of all objects for every class. These visualizations provide insights into the most probable and rare object locations on the image. It helps analyze objects' placements in a dataset.
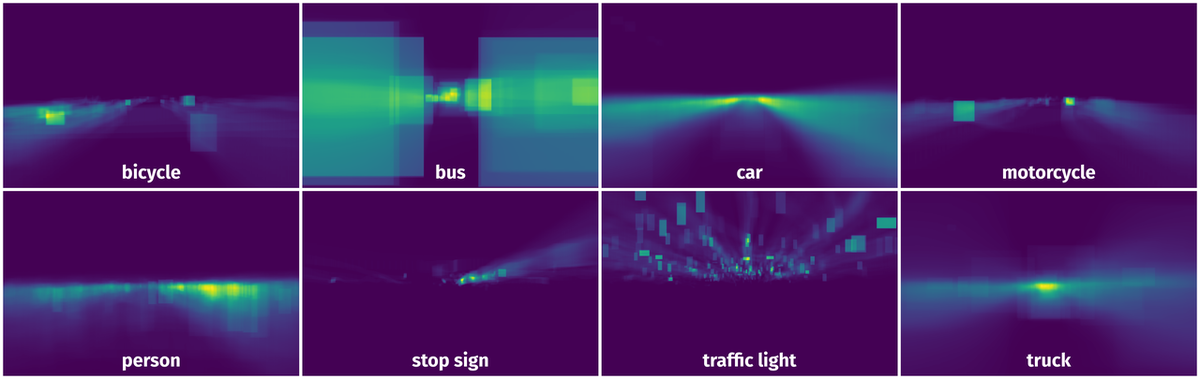
Objects #
Table contains all 100641 objects. Click a row to preview an image with annotations, and use search or pagination to navigate. Sort columns to find outliers in the dataset.
Object ID ã…¤ | Class ã…¤ | Image name click row to open | Image size height x width | Height ã…¤ | Height ã…¤ | Width ã…¤ | Width ã…¤ | Area ã…¤ |
---|---|---|---|---|---|---|---|---|
1âž” | truck rectangle | ring_front_center_315972333719202264.jpg | 1200 x 1920 | 701px | 58.42% | 456px | 23.75% | 13.87% |
2âž” | car rectangle | ring_front_center_315972333719202264.jpg | 1200 x 1920 | 63px | 5.25% | 63px | 3.28% | 0.17% |
3âž” | truck rectangle | ring_front_center_315972333719202264.jpg | 1200 x 1920 | 575px | 47.92% | 629px | 32.76% | 15.7% |
4âž” | car rectangle | ring_front_center_315972333719202264.jpg | 1200 x 1920 | 98px | 8.17% | 139px | 7.24% | 0.59% |
5âž” | car rectangle | ring_front_center_315972333719202264.jpg | 1200 x 1920 | 18px | 1.5% | 24px | 1.25% | 0.02% |
6âž” | car rectangle | ring_front_center_315972333719202264.jpg | 1200 x 1920 | 227px | 18.92% | 298px | 15.52% | 2.94% |
7âž” | car rectangle | ring_front_center_315972333719202264.jpg | 1200 x 1920 | 172px | 14.33% | 202px | 10.52% | 1.51% |
8âž” | truck rectangle | ring_front_center_315972333719202264.jpg | 1200 x 1920 | 51px | 4.25% | 71px | 3.7% | 0.16% |
9âž” | car rectangle | ring_front_center_315972333719202264.jpg | 1200 x 1920 | 49px | 4.08% | 22px | 1.15% | 0.05% |
10âž” | car rectangle | ring_front_center_315972333719202264.jpg | 1200 x 1920 | 41px | 3.42% | 21px | 1.09% | 0.04% |
License #
ADD CUSTOM LICENSE MANUALLY
Citation #
If you make use of the Argoverse HD data, please cite the following reference:
@article{Li2020StreamingP,
title={Towards Streaming Perception},
author={Li, Mengtian and Wang, Yuxiong and Ramanan, Deva},
journal={ECCV},
year={2020}
}
If you are happy with Dataset Ninja and use provided visualizations and tools in your work, please cite us:
@misc{ visualization-tools-for-argoverse-hd-dataset,
title = { Visualization Tools for Argoverse HD Dataset },
type = { Computer Vision Tools },
author = { Dataset Ninja },
howpublished = { \url{ https://datasetninja.com/argoverse-hd } },
url = { https://datasetninja.com/argoverse-hd },
journal = { Dataset Ninja },
publisher = { Dataset Ninja },
year = { 2025 },
month = { feb },
note = { visited on 2025-02-05 },
}
Download #
Dataset Argoverse HD can be downloaded in Supervisely format:
As an alternative, it can be downloaded with dataset-tools package:
pip install --upgrade dataset-tools
… using following python code:
import dataset_tools as dtools
dtools.download(dataset='Argoverse HD', dst_dir='~/dataset-ninja/')
Make sure not to overlook the python code example available on the Supervisely Developer Portal. It will give you a clear idea of how to effortlessly work with the downloaded dataset.
The data in original format can be downloaded here.
Disclaimer #
Our gal from the legal dep told us we need to post this:
Dataset Ninja provides visualizations and statistics for some datasets that can be found online and can be downloaded by general audience. Dataset Ninja is not a dataset hosting platform and can only be used for informational purposes. The platform does not claim any rights for the original content, including images, videos, annotations and descriptions. Joint publishing is prohibited.
You take full responsibility when you use datasets presented at Dataset Ninja, as well as other information, including visualizations and statistics we provide. You are in charge of compliance with any dataset license and all other permissions. You are required to navigate datasets homepage and make sure that you can use it. In case of any questions, get in touch with us at hello@datasetninja.com.