Introduction #
Authors introduce the Bangladeshi License Plate Recognition: Character Recognition dataset, which serves as a valuable resource for object detection within the surveillance industry. This dataset comprises 4,061 images, encompassing 35,705 labeled objects distributed across 27 distinct classes such as metro, dhaka, and numerical digits, including 1, 2, 3, 4, 5, 6, 7, 8, 9, as well as various characters and city names like khulna, ba, ja, chattogram and more. The dataset is partitioned into three subsets: train (3,480 images), validation (363 images), and test (218 images), making it a comprehensive tool for character recognition and license plate analysis in the Bangladeshi context.
About Automatic License Plate Recognition System For Bangladeshi Vehicles Using Deep Neural Network
The Bangladeshi License Plate Recognition: License Plate Localization dataset is a part of Automatic License Plate Recognition System (ALPRS) For Bangladeshi Vehicles Using Deep Neural Network work, which includes two datasets:
- Bangladeshi License Plate Recognition: License Plate Localization (available on DatasetNinja)
- Bangladeshi License Plate Recognition: Character Recognition (current)
The authors highlight that an ALPRS faces three main challenges as a whole. These are properly detecting a vehicle’s license plate, segmenting the texts in the license plate, andrecognizing the texts or characters or digits. Apart from these three challenges, another important challenge is to generate properly formatted output which is ready to use. Authors focuses on various ways of overcoming these challenges with the help of Deep Neural Networks and presents the most feasible solution for a complete ALPR system. Experimenting with different DNN models, authors have come up with the most effcient & robust solution in every stage. Authors have merged the segmentation phase with the recognition phase to make this process easy. By doing such, authors can save a lot of time and computational effort. Finally, authors have presented their custom algorithm, which is computationally effcient and generates a properly formatted output for their system.

Workflow diagram of the proposed system.
About Datasets:
One of the main contributions of authors’ work is the rich datasets for both localization and recognition of the Bangladeshi license plate. Authors’ first dataset (Bangladeshi License Plate Recognition: License Plate Localization) contains almost 2800 images for localization. The second dataset (Bangladeshi License Plate Recognition: Character Recognition) contains around 4000 license plate images cropped from the first dataset which are the most so far in this sector. Authors have split their datasets into 70:15:15 and 85:10:5 for training, validation, and testing purpose in license plate localization and text recognition stage respectively. Authors’ datasets contain images from different cities of Bangladesh: Dhaka, Khulna, Chattogram, Jashore including different vehicle categories license plates from both private and trading vehicles. Authors’ datasets are diverse enough and cover almost every possible condition, angle, and environment. To create this datasets more diverse authors have gathered images from different sources. From Nooruddin et al. authors are given their dataset of only trading vehicles. Most of the private vehicle images are used from paper Rahman et al., and the rest of them are collected by authors.
Summary #
Bangladeshi License Plate Recognition: Character Recognition is a dataset for an object detection task. It is used in the surveillance industry.
The dataset consists of 4061 images with 35705 labeled objects belonging to 27 different classes including metro, dhaka, 1, and other: 2, 0, ga, 3, 4, 5, 9, 7, 8, 6, ta, khulna, ba, ja, gha, kha, jashore, chattogram, ha, ca, da, ka, va, and na.
Images in the Bangladeshi License Plate Recognition: Character Recognition dataset have bounding box annotations. All images are labeled (i.e. with annotations). There are 3 splits in the dataset: train (3480 images), validation (363 images), and test (218 images). The dataset was released in 2022 by the Khulna University of Engineering & Technology, Bangladesh.
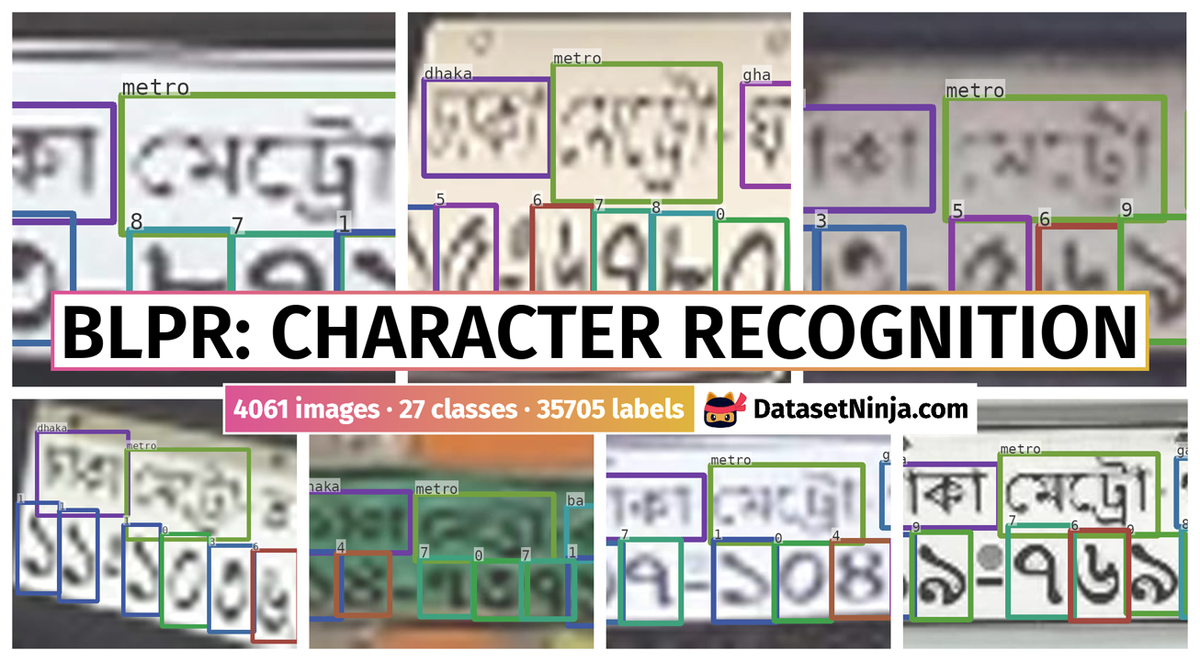
Explore #
Bangladeshi License Plate Recognition: Character Recognition dataset has 4061 images. Click on one of the examples below or open "Explore" tool anytime you need to view dataset images with annotations. This tool has extended visualization capabilities like zoom, translation, objects table, custom filters and more. Hover the mouse over the images to hide or show annotations.
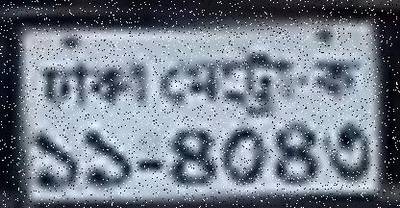
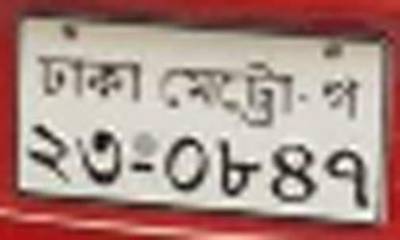
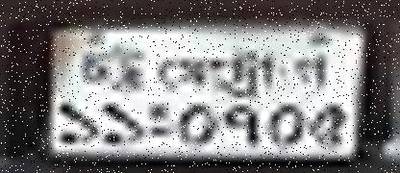
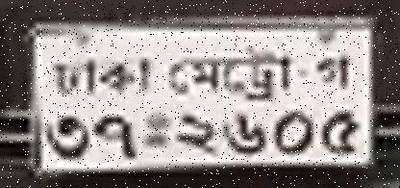
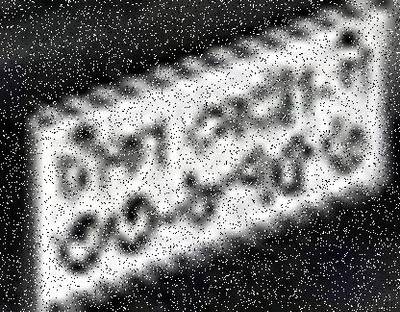

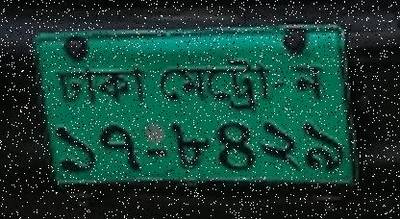
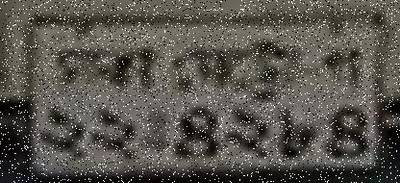
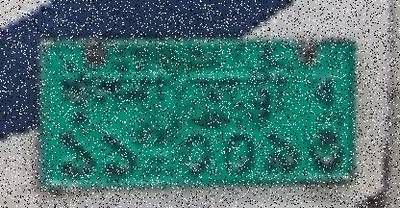
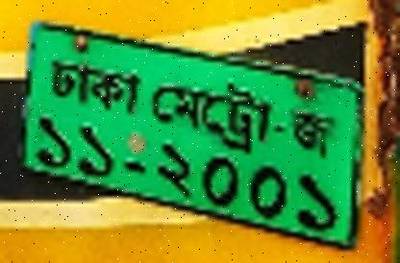
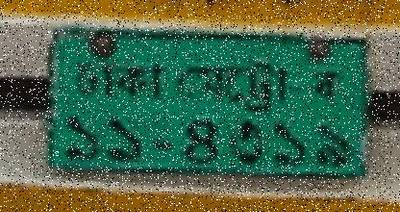
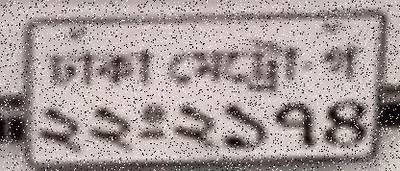
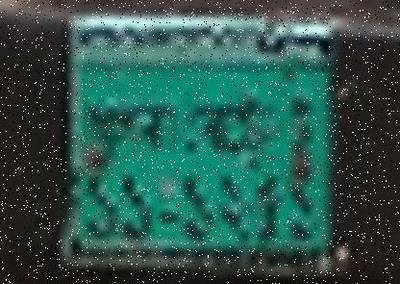
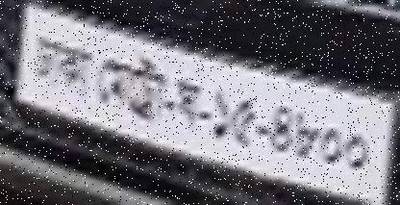
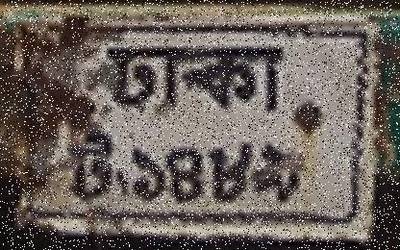
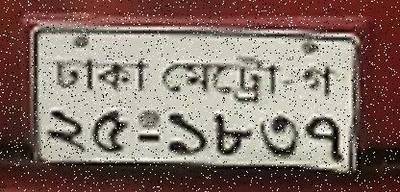
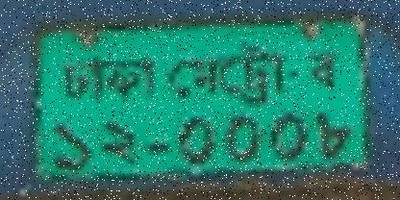
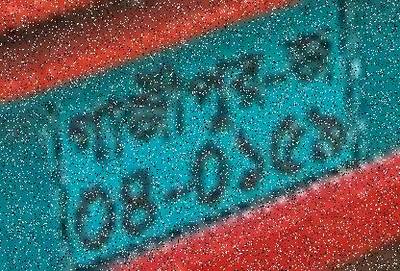
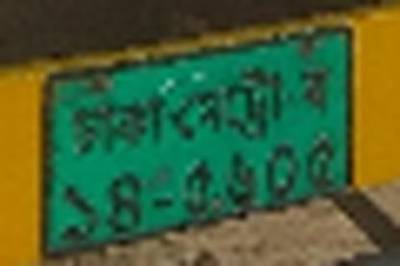
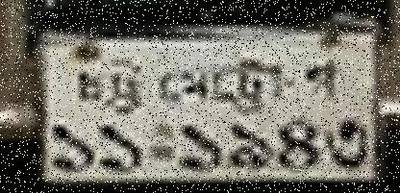
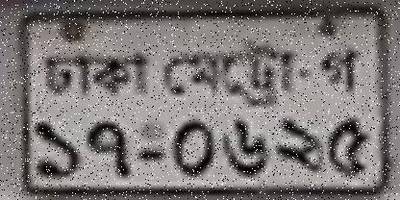
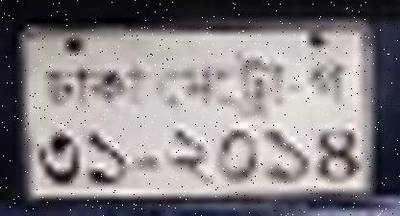
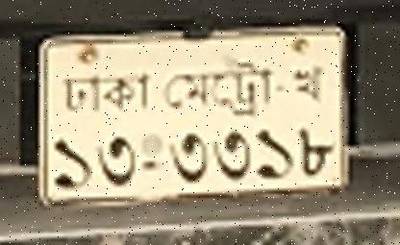
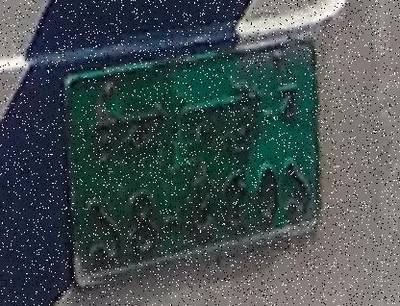
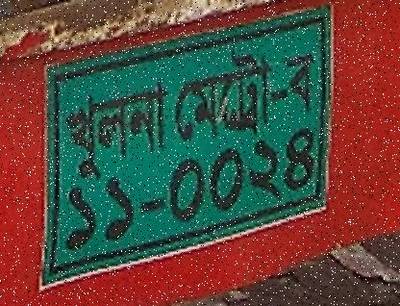
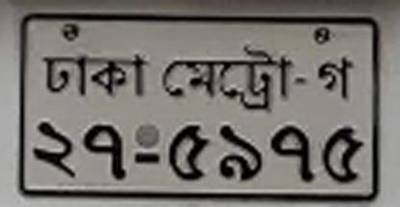
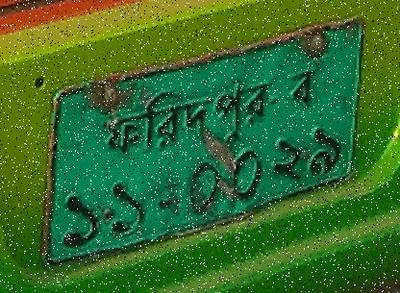
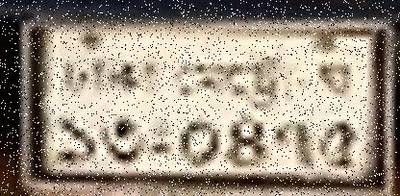

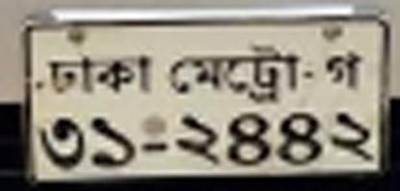
Class balance #
There are 27 annotation classes in the dataset. Find the general statistics and balances for every class in the table below. Click any row to preview images that have labels of the selected class. Sort by column to find the most rare or prevalent classes.
Class ㅤ | Images ㅤ | Objects ㅤ | Count on image average | Area on image average |
---|---|---|---|---|
metro➔ rectangle | 3720 | 3722 | 1 | 11.46% |
dhaka➔ rectangle | 3235 | 3239 | 1 | 8.55% |
1➔ rectangle | 3108 | 5304 | 1.71 | 6.76% |
2➔ rectangle | 2077 | 2598 | 1.25 | 5.4% |
0➔ rectangle | 2017 | 2861 | 1.42 | 5.4% |
ga➔ rectangle | 1859 | 1860 | 1 | 3.57% |
3➔ rectangle | 1851 | 2462 | 1.33 | 5.83% |
4➔ rectangle | 1704 | 2080 | 1.22 | 5.1% |
5➔ rectangle | 1695 | 2188 | 1.29 | 5.03% |
9➔ rectangle | 1502 | 1781 | 1.19 | 5% |
Co-occurrence matrix #
Co-occurrence matrix is an extremely valuable tool that shows you the images for every pair of classes: how many images have objects of both classes at the same time. If you click any cell, you will see those images. We added the tooltip with an explanation for every cell for your convenience, just hover the mouse over a cell to preview the description.
Images #
Explore every single image in the dataset with respect to the number of annotations of each class it has. Click a row to preview selected image. Sort by any column to find anomalies and edge cases. Use horizontal scroll if the table has many columns for a large number of classes in the dataset.
Object distribution #
Interactive heatmap chart for every class with object distribution shows how many images are in the dataset with a certain number of objects of a specific class. Users can click cell and see the list of all corresponding images.
Class sizes #
The table below gives various size properties of objects for every class. Click a row to see the image with annotations of the selected class. Sort columns to find classes with the smallest or largest objects or understand the size differences between classes.
Class | Object count | Avg area | Max area | Min area | Min height | Min height | Max height | Max height | Avg height | Avg height | Min width | Min width | Max width | Max width |
---|---|---|---|---|---|---|---|---|---|---|---|---|---|---|
1 rectangle | 5304 | 4.01% | 7.48% | 1.6% | 34px | 14.01% | 159px | 52.25% | 78px | 32.37% | 17px | 4.09% | 121px | 29.09% |
metro rectangle | 3722 | 11.46% | 17.14% | 3.34% | 34px | 19.02% | 172px | 65.48% | 81px | 34.4% | 54px | 12.98% | 173px | 41.59% |
dhaka rectangle | 3239 | 8.54% | 17.18% | 3.49% | 35px | 15.85% | 157px | 56.76% | 69px | 29.73% | 64px | 15.38% | 202px | 48.56% |
0 rectangle | 2861 | 3.83% | 6.88% | 1.63% | 37px | 13.55% | 147px | 60.71% | 80px | 32.16% | 23px | 5.53% | 103px | 24.76% |
2 rectangle | 2598 | 4.34% | 7.4% | 1.99% | 43px | 17.59% | 152px | 54.64% | 80px | 34.09% | 25px | 6.01% | 85px | 20.43% |
3 rectangle | 2462 | 4.4% | 7.17% | 2.06% | 34px | 16.15% | 140px | 55.64% | 78px | 33.6% | 23px | 5.53% | 80px | 19.23% |
5 rectangle | 2188 | 3.91% | 6.2% | 1.81% | 37px | 14.79% | 146px | 67.86% | 78px | 33.41% | 22px | 5.29% | 66px | 15.87% |
4 rectangle | 2080 | 4.19% | 7.01% | 1.83% | 37px | 14.93% | 153px | 50% | 80px | 33.75% | 25px | 6.01% | 126px | 30.29% |
ga rectangle | 1860 | 3.57% | 8.67% | 2.07% | 33px | 16.13% | 123px | 37.87% | 65px | 28.76% | 34px | 8.17% | 125px | 30.05% |
9 rectangle | 1781 | 4.23% | 6.98% | 1.85% | 44px | 15.62% | 166px | 47.57% | 81px | 33.28% | 25px | 6.01% | 78px | 18.75% |
Spatial Heatmap #
The heatmaps below give the spatial distributions of all objects for every class. These visualizations provide insights into the most probable and rare object locations on the image. It helps analyze objects' placements in a dataset.
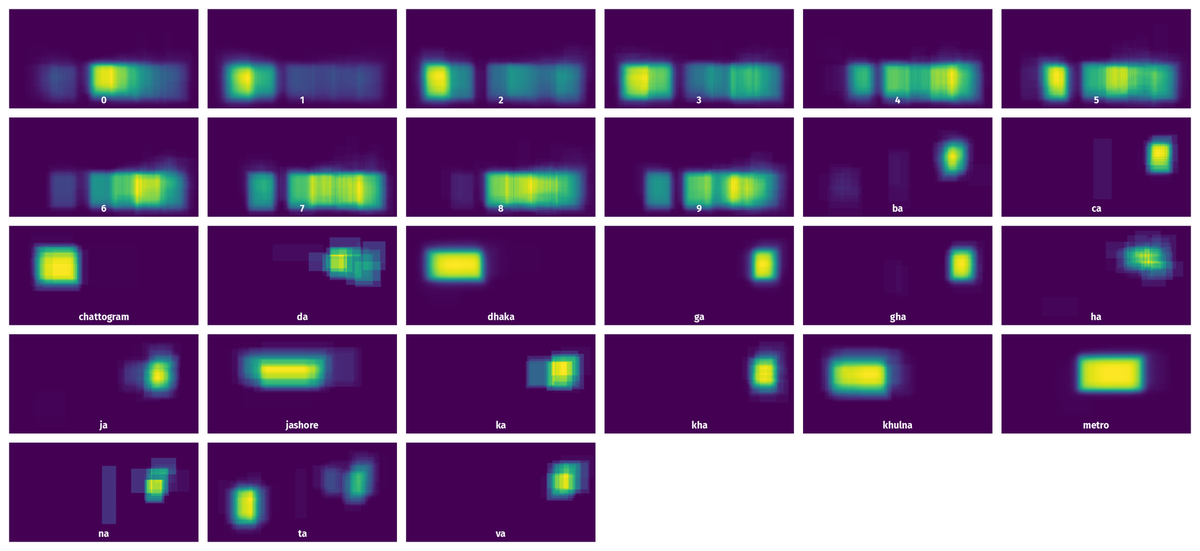
Objects #
Table contains all 35705 objects. Click a row to preview an image with annotations, and use search or pagination to navigate. Sort columns to find outliers in the dataset.
Object ID ㅤ | Class ㅤ | Image name click row to open | Image size height x width | Height ㅤ | Height ㅤ | Width ㅤ | Width ㅤ | Area ㅤ |
---|---|---|---|---|---|---|---|---|
1➔ | dhaka rectangle | car-6285_68_1_jpg.rf.a94caafa68440fed951d8fa27adf826f.jpg | 214 x 416 | 60px | 28.04% | 117px | 28.12% | 7.89% |
2➔ | metro rectangle | car-6285_68_1_jpg.rf.a94caafa68440fed951d8fa27adf826f.jpg | 214 x 416 | 68px | 31.78% | 134px | 32.21% | 10.24% |
3➔ | ga rectangle | car-6285_68_1_jpg.rf.a94caafa68440fed951d8fa27adf826f.jpg | 214 x 416 | 55px | 25.7% | 48px | 11.54% | 2.97% |
4➔ | 3 rectangle | car-6285_68_1_jpg.rf.a94caafa68440fed951d8fa27adf826f.jpg | 214 x 416 | 58px | 27.1% | 54px | 12.98% | 3.52% |
5➔ | 1 rectangle | car-6285_68_1_jpg.rf.a94caafa68440fed951d8fa27adf826f.jpg | 214 x 416 | 61px | 28.5% | 51px | 12.26% | 3.49% |
6➔ | 1 rectangle | car-6285_68_1_jpg.rf.a94caafa68440fed951d8fa27adf826f.jpg | 214 x 416 | 61px | 28.5% | 51px | 12.26% | 3.49% |
7➔ | 2 rectangle | car-6285_68_1_jpg.rf.a94caafa68440fed951d8fa27adf826f.jpg | 214 x 416 | 61px | 28.5% | 53px | 12.74% | 3.63% |
8➔ | 0 rectangle | car-6285_68_1_jpg.rf.a94caafa68440fed951d8fa27adf826f.jpg | 214 x 416 | 62px | 28.97% | 51px | 12.26% | 3.55% |
9➔ | 4 rectangle | car-6285_68_1_jpg.rf.a94caafa68440fed951d8fa27adf826f.jpg | 214 x 416 | 61px | 28.5% | 55px | 13.22% | 3.77% |
10➔ | khulna rectangle | IMG_20190413_123737_677_1_jpg.rf.ae35db2d6dfa38520981b5db8070e0db.jpg | 216 x 416 | 65px | 30.09% | 127px | 30.53% | 9.19% |
License #
Bangladeshi License Plate Recognition: Character Recognition is under Apache 2.0 license.
Citation #
If you make use of the BLPR: Character Recognition data, please cite the following reference:
@InProceedings{10.1007/978-981-16-6636-0_8,
author="Hossain, Syed Nahin and Hassan, Md. Zahim and Masba, Md. Masum Al",
editor="Arefin, Mohammad Shamsul and Kaiser, M. Shamim and Bandyopadhyay, Anirban and Ahad, Md. Atiqur Rahman and Ray, Kanad",
title="Automatic License Plate Recognition System for Bangladeshi Vehicles Using Deep Neural Network",
booktitle="Proceedings of the International Conference on Big Data, IoT, and Machine Learning",
year="2022",
publisher="Springer Singapore",
address="Singapore",
pages="91--102",
isbn="978-981-16-6636-0"
}
If you are happy with Dataset Ninja and use provided visualizations and tools in your work, please cite us:
@misc{ visualization-tools-for-bangladeshi-license-plate-recognition-character-dataset,
title = { Visualization Tools for Bangladeshi License Plate Recognition: Character Recognition Dataset },
type = { Computer Vision Tools },
author = { Dataset Ninja },
howpublished = { \url{ https://datasetninja.com/bangladeshi-license-plate-recognition-character } },
url = { https://datasetninja.com/bangladeshi-license-plate-recognition-character },
journal = { Dataset Ninja },
publisher = { Dataset Ninja },
year = { 2024 },
month = { nov },
note = { visited on 2024-11-21 },
}
Download #
Dataset Bangladeshi License Plate Recognition: Character Recognition can be downloaded in Supervisely format:
As an alternative, it can be downloaded with dataset-tools package:
pip install --upgrade dataset-tools
… using following python code:
import dataset_tools as dtools
dtools.download(dataset='Bangladeshi License Plate Recognition: Character Recognition', dst_dir='~/dataset-ninja/')
Make sure not to overlook the python code example available on the Supervisely Developer Portal. It will give you a clear idea of how to effortlessly work with the downloaded dataset.
The data in original format can be downloaded here.
Disclaimer #
Our gal from the legal dep told us we need to post this:
Dataset Ninja provides visualizations and statistics for some datasets that can be found online and can be downloaded by general audience. Dataset Ninja is not a dataset hosting platform and can only be used for informational purposes. The platform does not claim any rights for the original content, including images, videos, annotations and descriptions. Joint publishing is prohibited.
You take full responsibility when you use datasets presented at Dataset Ninja, as well as other information, including visualizations and statistics we provide. You are in charge of compliance with any dataset license and all other permissions. You are required to navigate datasets homepage and make sure that you can use it. In case of any questions, get in touch with us at hello@datasetninja.com.