Introduction #
CelebAMask-HQ is an extensive dataset featuring 30,000 high-resolution face images. These images were carefully chosen from the CelebA dataset by adhering to the CelebA-HQ guidelines. Each image in this dataset comes with a segmentation mask outlining facial attributes that correspond to the CelebA dataset. This initiative resulted in the creation of a substantial dataset for face attribute labeling, all based on the CelebAHQ collection consisting of 30,000 high-resolution face images from CelebA.
It has several appealing properties:
- Comprehensive Annotations. CelebAMask-HQ was precisely hand-annotated with the size of 512×512 and 19 classes including all facial components and accessories such as skin, nose, eyes, eyebrows, ears, mouth, lip, hair, hat, eyeglass, earring, necklace, neck, and cloth.
-
Label Size Selection. The size of images in CelebAHQ were 1024×1024. However, we chose the size of 512×512 because the cost of the labeling would be quite high for labeling the face at 1024×1024. Besides, we could easily extend the labels from 512×512 to 1024x1024 by nearest-neighbor interpolation without introducing noticeable artifacts.
-
Quality Control. After manual labeling, we had a quality control check on every single segmentation mask. Furthermore, we asked annotators to refine all masks with several rounds of iterations.
-
Amodal Handling. For occlusion handling, if the facial component was partly occluded, we asked annotators to label the occluded parts of the components by human inferring. On the other hand, we skipped the annotations for those components that are totally occluded.
CelebAMask-HQ can be used to train and evaluate algorithms of face parsing, face recognition, and GANs for face generation and editing.
Summary #
CelebAMask-HQ is a dataset for a semantic segmentation task. It is used in the entertainment industry.
The dataset consists of 30000 images with 372766 labeled objects belonging to 18 different classes including skin, nose, l_lip, and other: u_lip, hair, r_eye, l_eye, neck, l_brow, r_brow, mouth, cloth, l_ear, r_ear, ear_r, neck_l, eye_g, and hat.
Images in the CelebAMask-HQ dataset have pixel-level semantic segmentation annotations. All images are labeled (i.e. with annotations). There are no pre-defined train/val/test splits in the dataset. Additionally, every image contains information about visual characteristics of a person. The dataset was released in 2019 by the SenseTime Research, The Chinese University of Hong Kong, and The University of Hong Kong.
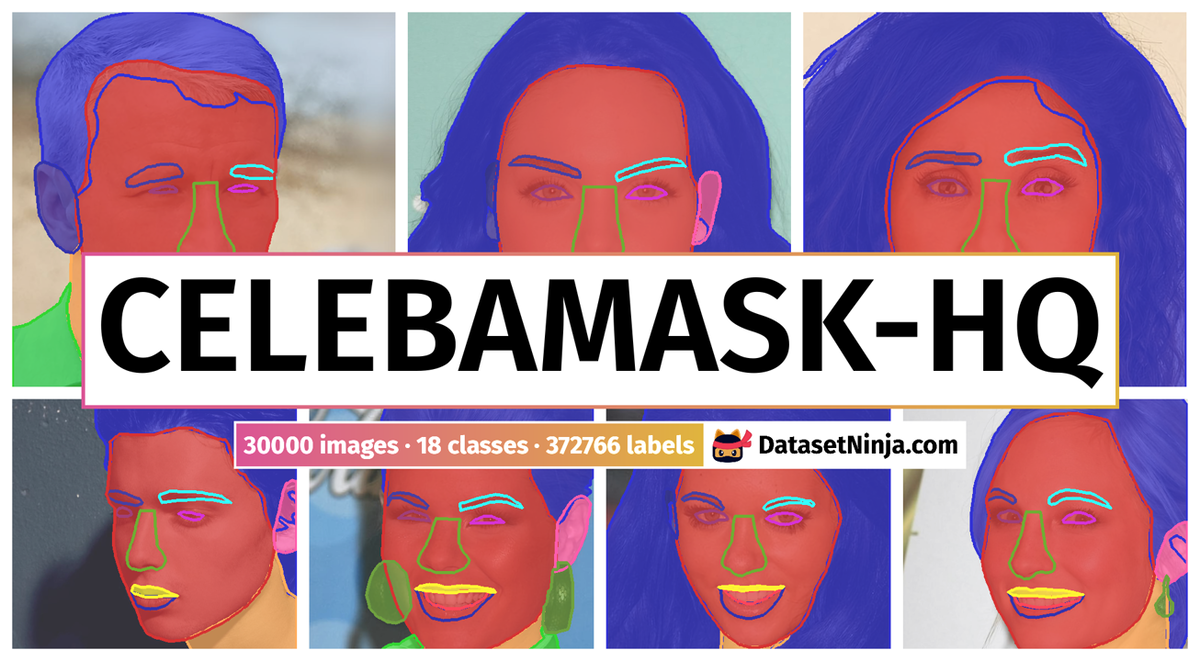
Explore #
CelebAMask-HQ dataset has 30000 images. Click on one of the examples below or open "Explore" tool anytime you need to view dataset images with annotations. This tool has extended visualization capabilities like zoom, translation, objects table, custom filters and more. Hover the mouse over the images to hide or show annotations.
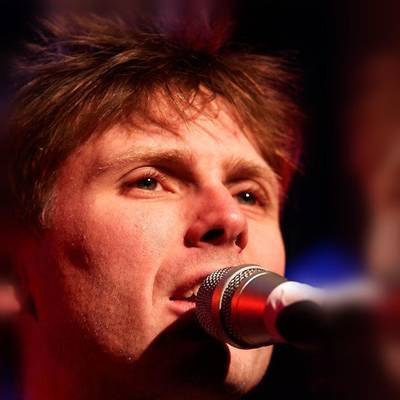
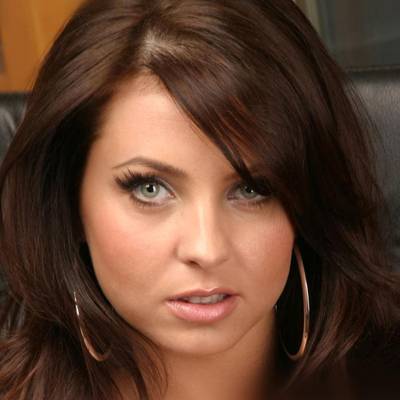
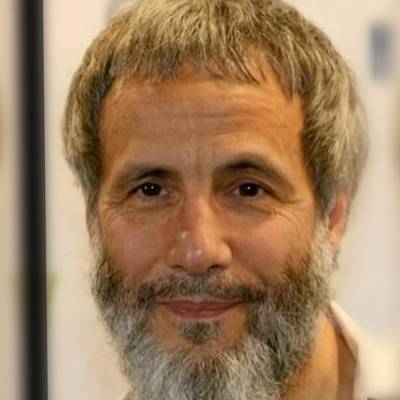
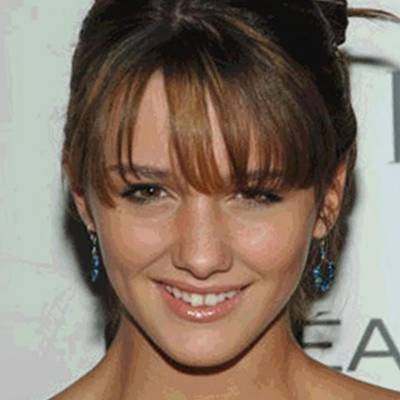
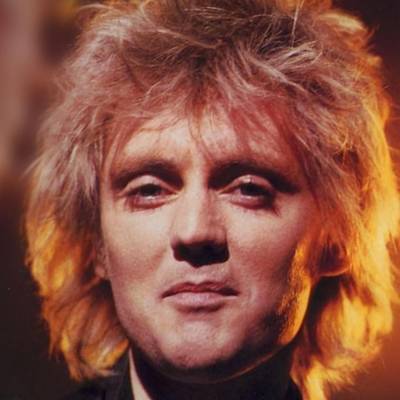


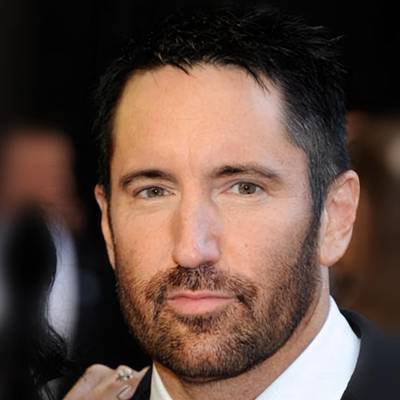
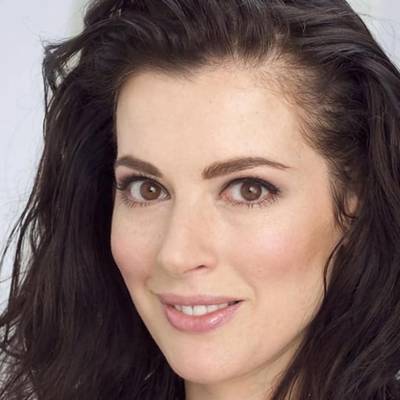
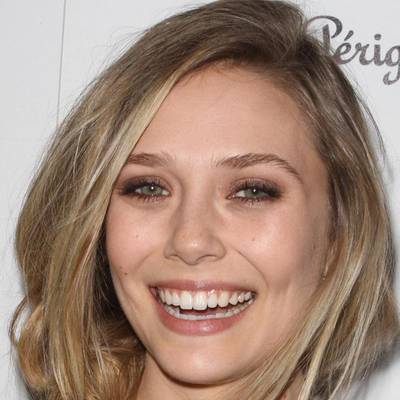
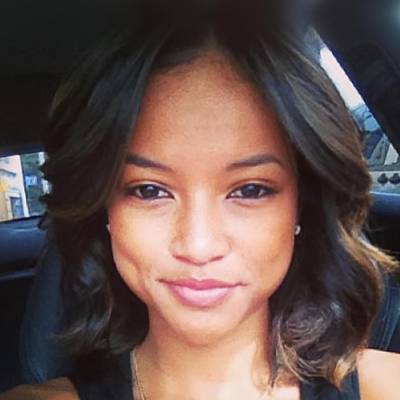
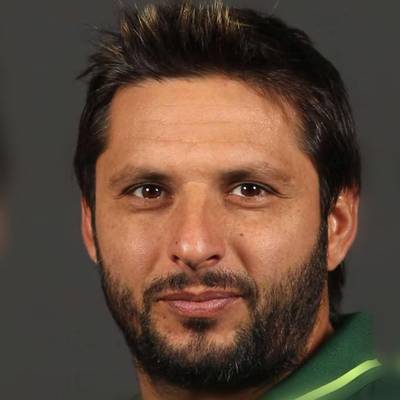
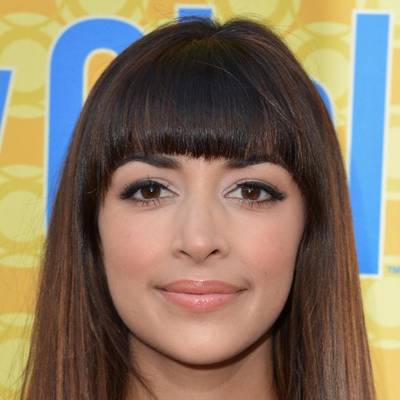
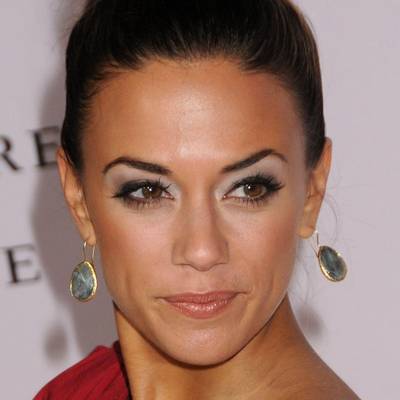
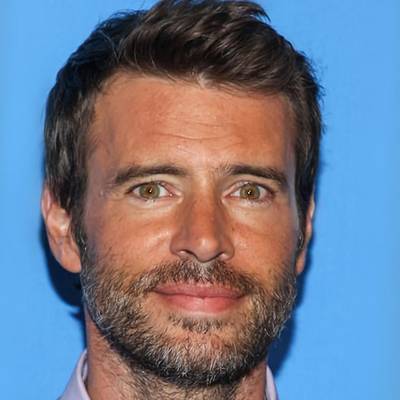

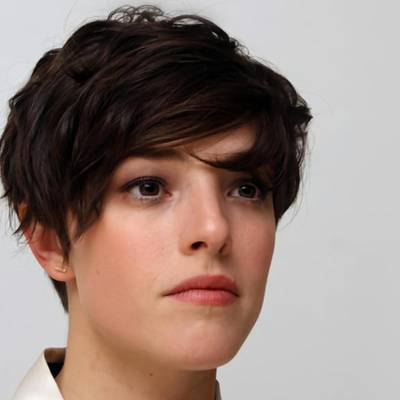
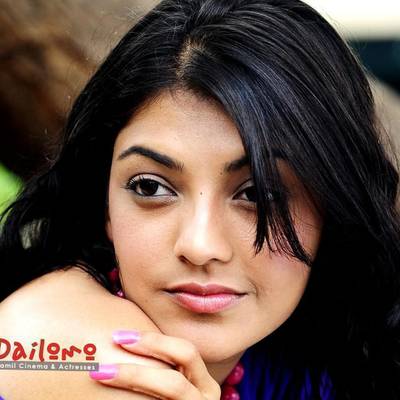
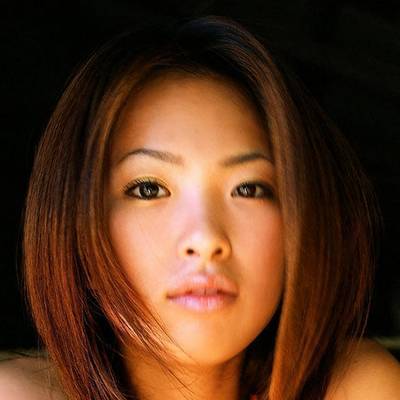
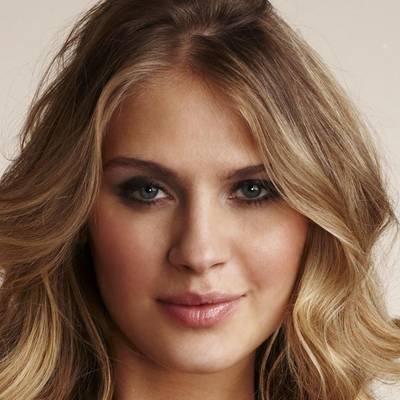
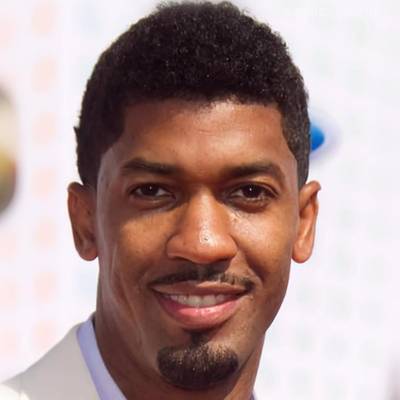
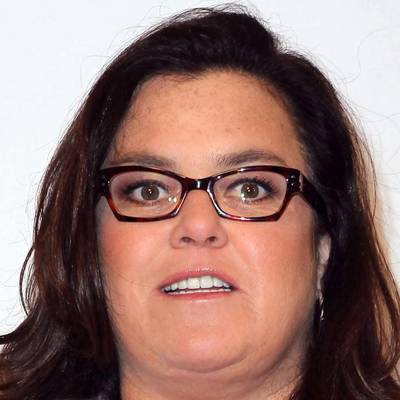
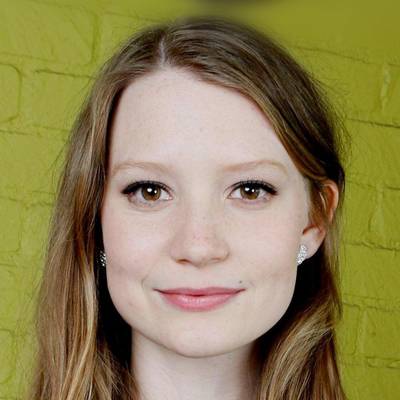
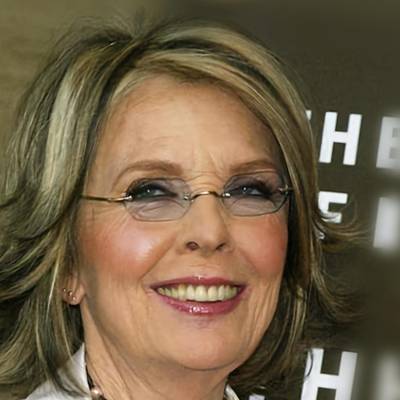
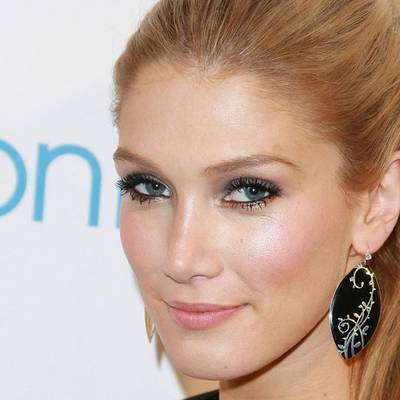

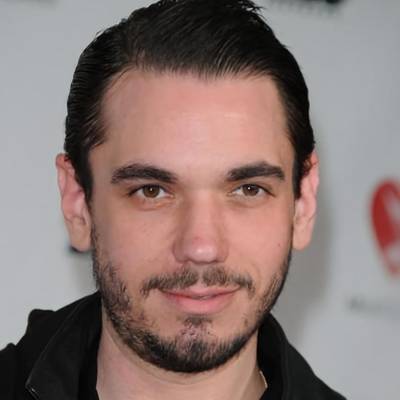
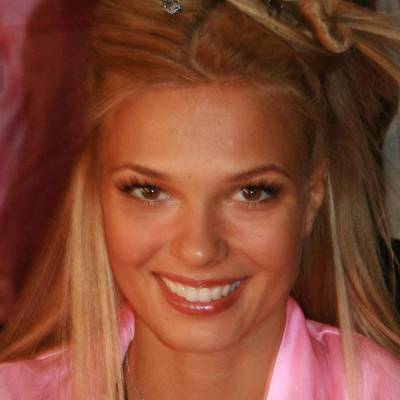
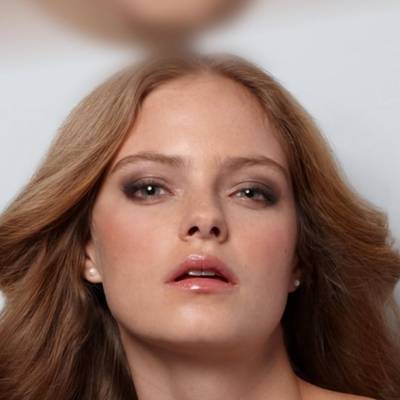
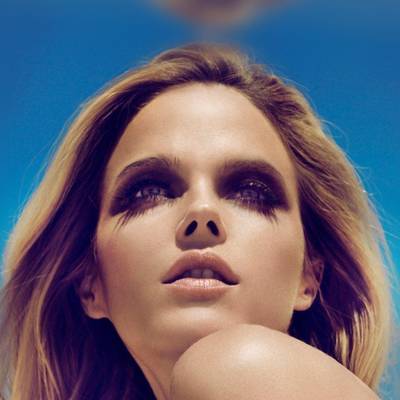
Class balance #
There are 18 annotation classes in the dataset. Find the general statistics and balances for every class in the table below. Click any row to preview images that have labels of the selected class. Sort by column to find the most rare or prevalent classes.
Class ã…¤ | Images ã…¤ | Objects ã…¤ | Count on image average | Area on image average |
---|---|---|---|---|
skinâž” mask | 30000 | 30000 | 1 | 32% |
noseâž” mask | 29998 | 29998 | 1 | 2.07% |
l_lipâž” mask | 29896 | 29896 | 1 | 0.68% |
u_lipâž” mask | 29870 | 29870 | 1 | 0.43% |
hairâž” mask | 29300 | 29300 | 1 | 32.67% |
r_eyeâž” mask | 29260 | 29260 | 1 | 0.23% |
l_eyeâž” mask | 29258 | 29258 | 1 | 0.23% |
neckâž” mask | 29148 | 29148 | 1 | 4.28% |
l_browâž” mask | 29029 | 29029 | 1 | 0.46% |
r_browâž” mask | 28968 | 28968 | 1 | 0.45% |
Co-occurrence matrix #
Co-occurrence matrix is an extremely valuable tool that shows you the images for every pair of classes: how many images have objects of both classes at the same time. If you click any cell, you will see those images. We added the tooltip with an explanation for every cell for your convenience, just hover the mouse over a cell to preview the description.
Images #
Explore every single image in the dataset with respect to the number of annotations of each class it has. Click a row to preview selected image. Sort by any column to find anomalies and edge cases. Use horizontal scroll if the table has many columns for a large number of classes in the dataset.
Object distribution #
Interactive heatmap chart for every class with object distribution shows how many images are in the dataset with a certain number of objects of a specific class. Users can click cell and see the list of all corresponding images.
Class sizes #
The table below gives various size properties of objects for every class. Click a row to see the image with annotations of the selected class. Sort columns to find classes with the smallest or largest objects or understand the size differences between classes.
Class | Object count | Avg area | Max area | Min area | Min height | Min height | Max height | Max height | Avg height | Avg height | Min width | Min width | Max width | Max width |
---|---|---|---|---|---|---|---|---|---|---|---|---|---|---|
skin mask | 30000 | 32% | 61.21% | 1.17% | 144px | 14.06% | 1024px | 100% | 774px | 75.63% | 110px | 10.74% | 838px | 81.84% |
nose mask | 29998 | 2.07% | 6.6% | 0.07% | 44px | 4.3% | 562px | 54.88% | 213px | 20.84% | 28px | 2.73% | 562px | 54.88% |
l_lip mask | 29896 | 0.68% | 4.99% | 0.02% | 10px | 0.98% | 788px | 76.95% | 74px | 7.23% | 36px | 3.52% | 672px | 65.62% |
u_lip mask | 29870 | 0.43% | 7.05% | 0.02% | 8px | 0.78% | 354px | 34.57% | 40px | 3.86% | 38px | 3.71% | 530px | 51.76% |
hair mask | 29300 | 32.67% | 81.95% | 0.02% | 38px | 3.71% | 1024px | 100% | 846px | 82.66% | 14px | 1.37% | 1024px | 100% |
r_eye mask | 29260 | 0.23% | 2.91% | 0.01% | 6px | 0.59% | 346px | 33.79% | 37px | 3.59% | 10px | 0.98% | 674px | 65.82% |
l_eye mask | 29258 | 0.23% | 2.96% | 0.01% | 8px | 0.78% | 298px | 29.1% | 37px | 3.59% | 14px | 1.37% | 488px | 47.66% |
neck mask | 29148 | 4.28% | 15% | 0.02% | 18px | 1.76% | 630px | 61.52% | 261px | 25.47% | 18px | 1.76% | 1024px | 100% |
l_brow mask | 29029 | 0.46% | 2.24% | 0.01% | 12px | 1.17% | 378px | 36.91% | 58px | 5.64% | 22px | 2.15% | 500px | 48.83% |
r_brow mask | 28968 | 0.45% | 3.02% | 0.02% | 12px | 1.17% | 378px | 36.91% | 58px | 5.66% | 22px | 2.15% | 520px | 50.78% |
Spatial Heatmap #
The heatmaps below give the spatial distributions of all objects for every class. These visualizations provide insights into the most probable and rare object locations on the image. It helps analyze objects' placements in a dataset.
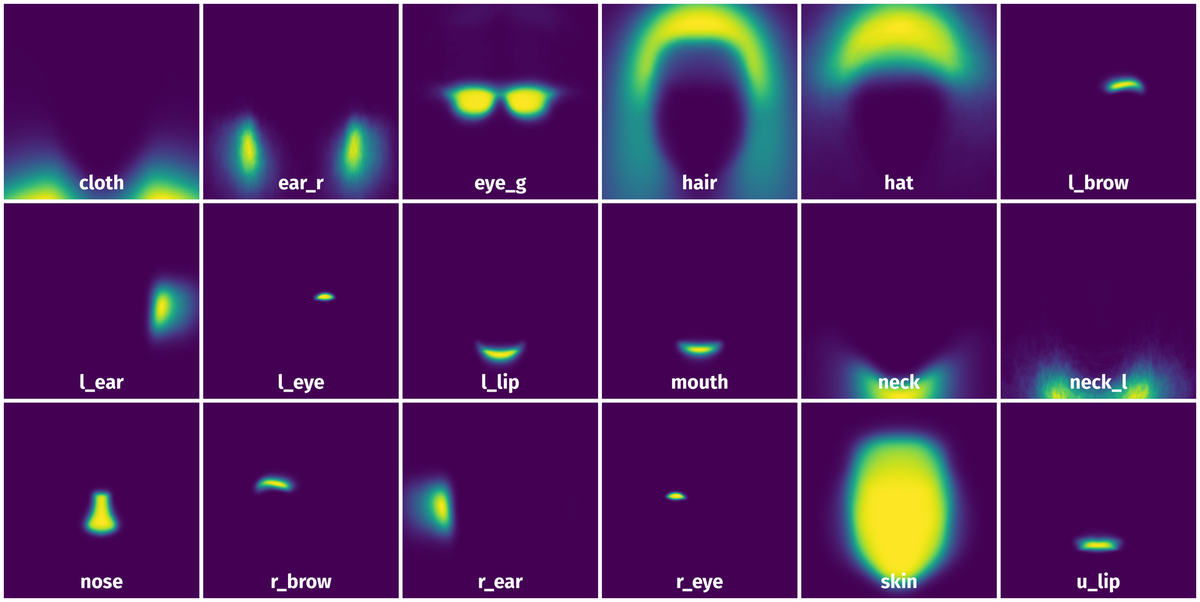
Objects #
Table contains all 372766 objects. Click a row to preview an image with annotations, and use search or pagination to navigate. Sort columns to find outliers in the dataset.
Object ID ã…¤ | Class ã…¤ | Image name click row to open | Image size height x width | Height ã…¤ | Height ã…¤ | Width ã…¤ | Width ã…¤ | Area ã…¤ |
---|---|---|---|---|---|---|---|---|
1âž” | hair mask | 25638.jpg | 1024 x 1024 | 554px | 54.1% | 760px | 74.22% | 16.41% |
2âž” | l_brow mask | 25638.jpg | 1024 x 1024 | 62px | 6.05% | 150px | 14.65% | 0.42% |
3âž” | l_eye mask | 25638.jpg | 1024 x 1024 | 28px | 2.73% | 90px | 8.79% | 0.15% |
4âž” | l_lip mask | 25638.jpg | 1024 x 1024 | 32px | 3.12% | 216px | 21.09% | 0.31% |
5âž” | mouth mask | 25638.jpg | 1024 x 1024 | 20px | 1.95% | 144px | 14.06% | 0.2% |
6âž” | nose mask | 25638.jpg | 1024 x 1024 | 200px | 19.53% | 188px | 18.36% | 2.37% |
7âž” | r_brow mask | 25638.jpg | 1024 x 1024 | 62px | 6.05% | 166px | 16.21% | 0.54% |
8âž” | r_eye mask | 25638.jpg | 1024 x 1024 | 28px | 2.73% | 84px | 8.2% | 0.15% |
9âž” | skin mask | 25638.jpg | 1024 x 1024 | 780px | 76.17% | 580px | 56.64% | 35.71% |
10âž” | u_lip mask | 25638.jpg | 1024 x 1024 | 30px | 2.93% | 232px | 22.66% | 0.36% |
License #
- The CelebAMask-HQ dataset is available for non-commercial research purposes only.
- You agree not to reproduce, duplicate, copy, sell, trade, resell or exploit for any commercial purposes, any portion of the images and any portion of derived data.
- You agree not to further copy, publish or distribute any portion of the CelebAMask-HQ dataset. Except, for internal use at a single site within the same organization it is allowed to make copies of the dataset.
Citation #
If you make use of the CelebAMask-HQ data, please cite the following reference:
@inproceedings{CelebAMask-HQ,
title={MaskGAN: Towards Diverse and Interactive Facial Image Manipulation},
author={Lee, Cheng-Han and Liu, Ziwei and Wu, Lingyun and Luo, Ping},
booktitle={IEEE Conference on Computer Vision and Pattern Recognition (CVPR)},
year={2020}
}
If you are happy with Dataset Ninja and use provided visualizations and tools in your work, please cite us:
@misc{ visualization-tools-for-celebamask-hq-dataset,
title = { Visualization Tools for CelebAMask-HQ Dataset },
type = { Computer Vision Tools },
author = { Dataset Ninja },
howpublished = { \url{ https://datasetninja.com/celebamask-hq } },
url = { https://datasetninja.com/celebamask-hq },
journal = { Dataset Ninja },
publisher = { Dataset Ninja },
year = { 2025 },
month = { jun },
note = { visited on 2025-06-25 },
}
Download #
Dataset CelebAMask-HQ can be downloaded in Supervisely format:
As an alternative, it can be downloaded with dataset-tools package:
pip install --upgrade dataset-tools
… using following python code:
import dataset_tools as dtools
dtools.download(dataset='CelebAMask-HQ', dst_dir='~/dataset-ninja/')
Make sure not to overlook the python code example available on the Supervisely Developer Portal. It will give you a clear idea of how to effortlessly work with the downloaded dataset.
Disclaimer #
Our gal from the legal dep told us we need to post this:
Dataset Ninja provides visualizations and statistics for some datasets that can be found online and can be downloaded by general audience. Dataset Ninja is not a dataset hosting platform and can only be used for informational purposes. The platform does not claim any rights for the original content, including images, videos, annotations and descriptions. Joint publishing is prohibited.
You take full responsibility when you use datasets presented at Dataset Ninja, as well as other information, including visualizations and statistics we provide. You are in charge of compliance with any dataset license and all other permissions. You are required to navigate datasets homepage and make sure that you can use it. In case of any questions, get in touch with us at hello@datasetninja.com.