Introduction #
The Cheque Detection dataset is sourced from the IDRBT Cheque Image Dataset and features a diverse range of textures and ink colors. It comprises a total of 112 cheque leaves from four different Indian banks. The dataset is organized into seven classes, including sign, dateiss, chqno, amt, acno, receivername and issuebank which represent various elements commonly found on cheques. This dataset is particularly valuable for tasks related to automated cheque processing, such as signature verification, date extraction, and the extraction of relevant information such as check numbers, amounts, account numbers, payee names, and issuing banks.
In order to simulate the pen ink difference in cheque leaves, seven blue and seven black pens are used. To avoid bias due to writing, nine different volunteers have taken active participation in preparing the data set. A total of 14×9 = 126 pen−volunteer combinations (fourteen pens and nine volunteers) are used for pen ink data generation. In a practical scenario, similar color pens are used for the addition of new words in the source document. Each cheque is written by two volunteers using two different pens (either blue or black). Hence, the data set is created with 42 possible combinations of blue and black pens. All the cheque leaves are scanned in the normal scanner at 300 dpi resolution. Handwritten words from each scanned cheque are cropped manually and grouped based on the pen used to write the words.
The authors have introduced a novel method for identifying pen ink differences in handwritten bank checks. They frame the problem as a binary classification task and extract thirty features for each word pair instance. These features serve as the basis for training a Multi-Layer Perceptron (MLP) classifier using known pen ink data. The performance of the MLP classifier is assessed on both known and unknown pen ink pixels. A thorough analysis and comparison of the results demonstrate the superior performance of the proposed method when compared to existing approaches, particularly for distinguishing between black and blue pen samples in handwritten checks.
Forensics in handwritten Bank cheques from the perspective of differentiating pen ink has great importance to the judicial system. In handwritten Bank cheque forensics, it is often important to establish a relation between the pen inks. It helps to identify whether a single pen has been used to write the Bank cheque or multiple pens. Numerous possibilities of fraud exist in handwritten Bank cheques. In this work, authors focus only on pen ink differentiation in Bank cheques. Possibilities of fraud in any Bank cheque and its consequences help to understand the importance of the work.
An example of new word addition in a Bank cheque using a different pen is depicted in the Figure below which is elaborated as follows. The cheque was initially issued to Mr. Ravi Kumar Singh, amounting to Seventy thousand only. Later, the forger appended new words in the pay name and amount section as marked by red circles. This difference in pen ink can not be always perceived by the naked eye. This type of case helps to understand the possibility of the addition of new words in handwritten Bank cheques. A number of handwritten document frauds are possible in bills, business agreements, educational documents, etc. This motivates authors to differentiate pen ink in Bank cheques.
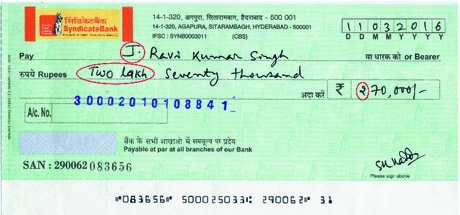
Addition of handwritten words (marked by red circles) in a Bank cheque image.
Summary #
Cheque Detection is a dataset for an object detection task. It is used in the security industry, and in the optical character recognition (OCR) domain.
The dataset consists of 112 images with 784 labeled objects belonging to 7 different classes including issuebank, receivername, acno, and other: amt, chqno, dateiss, and sign.
Images in the Cheque Detection dataset have bounding box annotations. All images are labeled (i.e. with annotations). There are 2 splits in the dataset: train (90 images) and test (22 images). The dataset was released in 2020 by the Indian Institute of Technology and Institute for Development and Research in Banking Technology, India.
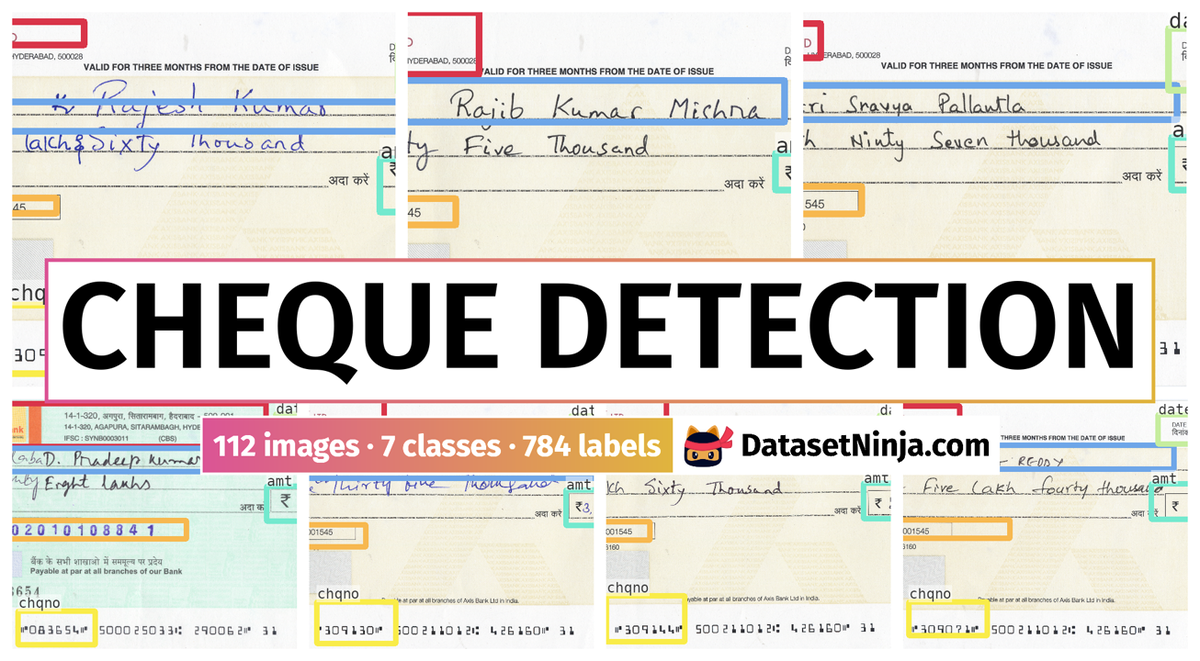
Explore #
Cheque Detection dataset has 112 images. Click on one of the examples below or open "Explore" tool anytime you need to view dataset images with annotations. This tool has extended visualization capabilities like zoom, translation, objects table, custom filters and more. Hover the mouse over the images to hide or show annotations.
Class balance #
There are 7 annotation classes in the dataset. Find the general statistics and balances for every class in the table below. Click any row to preview images that have labels of the selected class. Sort by column to find the most rare or prevalent classes.
Class ã…¤ | Images ã…¤ | Objects ã…¤ | Count on image average | Area on image average |
---|---|---|---|---|
signâž” rectangle | 112 | 112 | 1 | 2% |
dateissâž” rectangle | 112 | 112 | 1 | 3.63% |
chqnoâž” rectangle | 112 | 112 | 1 | 2.24% |
amtâž” rectangle | 112 | 112 | 1 | 3.63% |
acnoâž” rectangle | 112 | 112 | 1 | 2.87% |
receivernameâž” rectangle | 112 | 112 | 1 | 6.55% |
issuebankâž” rectangle | 112 | 112 | 1 | 5.43% |
Co-occurrence matrix #
Co-occurrence matrix is an extremely valuable tool that shows you the images for every pair of classes: how many images have objects of both classes at the same time. If you click any cell, you will see those images. We added the tooltip with an explanation for every cell for your convenience, just hover the mouse over a cell to preview the description.
Images #
Explore every single image in the dataset with respect to the number of annotations of each class it has. Click a row to preview selected image. Sort by any column to find anomalies and edge cases. Use horizontal scroll if the table has many columns for a large number of classes in the dataset.
Object distribution #
Interactive heatmap chart for every class with object distribution shows how many images are in the dataset with a certain number of objects of a specific class. Users can click cell and see the list of all corresponding images.
Class sizes #
The table below gives various size properties of objects for every class. Click a row to see the image with annotations of the selected class. Sort columns to find classes with the smallest or largest objects or understand the size differences between classes.
Class | Object count | Avg area | Max area | Min area | Min height | Min height | Max height | Max height | Avg height | Avg height | Min width | Min width | Max width | Max width |
---|---|---|---|---|---|---|---|---|---|---|---|---|---|---|
sign rectangle | 112 | 2% | 3.36% | 1.08% | 95px | 8.64% | 294px | 27.61% | 178px | 16.4% | 234px | 9.89% | 393px | 16.62% |
dateiss rectangle | 112 | 3.63% | 4.65% | 1.19% | 64px | 5.93% | 183px | 16.87% | 145px | 13.35% | 472px | 19.96% | 704px | 29.77% |
chqno rectangle | 112 | 2.24% | 2.9% | 0.44% | 49px | 4.54% | 244px | 22.61% | 170px | 15.65% | 230px | 9.73% | 341px | 14.42% |
amt rectangle | 112 | 3.63% | 3.83% | 1.73% | 79px | 7.32% | 155px | 14.55% | 151px | 13.89% | 499px | 21.1% | 623px | 26.34% |
acno rectangle | 112 | 2.87% | 5.87% | 1.16% | 44px | 4.08% | 127px | 11.62% | 89px | 8.15% | 626px | 26.47% | 1198px | 50.66% |
receivername rectangle | 112 | 6.55% | 9.32% | 2.61% | 41px | 3.73% | 130px | 12.21% | 102px | 9.37% | 1160px | 49.05% | 1806px | 76.36% |
issuebank rectangle | 112 | 5.43% | 11.37% | 2.14% | 69px | 6.39% | 205px | 19% | 151px | 13.91% | 562px | 23.76% | 1577px | 66.68% |
Spatial Heatmap #
The heatmaps below give the spatial distributions of all objects for every class. These visualizations provide insights into the most probable and rare object locations on the image. It helps analyze objects' placements in a dataset.
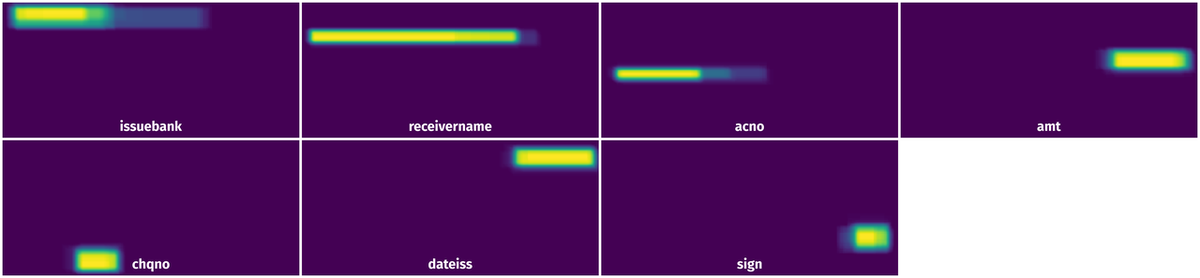
Objects #
Table contains all 784 objects. Click a row to preview an image with annotations, and use search or pagination to navigate. Sort columns to find outliers in the dataset.
Object ID ã…¤ | Class ã…¤ | Image name click row to open | Image size height x width | Height ã…¤ | Height ã…¤ | Width ã…¤ | Width ã…¤ | Area ã…¤ |
---|---|---|---|---|---|---|---|---|
1âž” | sign rectangle | Cheque309070.jpg.tiff | 1079 x 2365 | 132px | 12.23% | 295px | 12.47% | 1.53% |
2âž” | issuebank rectangle | Cheque309070.jpg.tiff | 1079 x 2365 | 119px | 11.03% | 736px | 31.12% | 3.43% |
3âž” | receivername rectangle | Cheque309070.jpg.tiff | 1079 x 2365 | 103px | 9.55% | 1656px | 70.02% | 6.68% |
4âž” | acno rectangle | Cheque309070.jpg.tiff | 1079 x 2365 | 81px | 7.51% | 924px | 39.07% | 2.93% |
5âž” | amt rectangle | Cheque309070.jpg.tiff | 1079 x 2365 | 155px | 14.37% | 622px | 26.3% | 3.78% |
6âž” | chqno rectangle | Cheque309070.jpg.tiff | 1079 x 2365 | 217px | 20.11% | 341px | 14.42% | 2.9% |
7âž” | dateiss rectangle | Cheque309070.jpg.tiff | 1079 x 2365 | 131px | 12.14% | 653px | 27.61% | 3.35% |
8âž” | sign rectangle | Cheque309076.jpg.tiff | 1100 x 2365 | 165px | 15% | 295px | 12.47% | 1.87% |
9âž” | issuebank rectangle | Cheque309076.jpg.tiff | 1100 x 2365 | 186px | 16.91% | 707px | 29.89% | 5.05% |
10âž” | receivername rectangle | Cheque309076.jpg.tiff | 1100 x 2365 | 103px | 9.36% | 1657px | 70.06% | 6.56% |
License #
License is unknown for the Cheque Detection dataset.
Citation #
If you make use of the Cheque Detection data, please cite the following reference:
@InProceedings{10.1007/978-3-319-69900-4_83,
author="Dansena, Prabhat and Bag, Soumen and Pal, Rajarshi",
editor="Shankar, B. Uma and Ghosh, Kuntal and Mandal, Deba Prasad and Ray, Shubhra Sankar and Zhang, David and Pal, Sankar K.",
title="Differentiating Pen Inks in Handwritten Bank Cheques Using Multi-layer Perceptron",
booktitle="Pattern Recognition and Machine Intelligence",
year="2017",
publisher="Springer International Publishing",
address="Cham",
pages="655--663",
isbn="978-3-319-69900-4"
}
If you are happy with Dataset Ninja and use provided visualizations and tools in your work, please cite us:
@misc{ visualization-tools-for-cheque-detection-dataset,
title = { Visualization Tools for Cheque Detection Dataset },
type = { Computer Vision Tools },
author = { Dataset Ninja },
howpublished = { \url{ https://datasetninja.com/cheque-detection } },
url = { https://datasetninja.com/cheque-detection },
journal = { Dataset Ninja },
publisher = { Dataset Ninja },
year = { 2025 },
month = { jul },
note = { visited on 2025-07-05 },
}
Download #
Please visit dataset homepage to download the data.
Disclaimer #
Our gal from the legal dep told us we need to post this:
Dataset Ninja provides visualizations and statistics for some datasets that can be found online and can be downloaded by general audience. Dataset Ninja is not a dataset hosting platform and can only be used for informational purposes. The platform does not claim any rights for the original content, including images, videos, annotations and descriptions. Joint publishing is prohibited.
You take full responsibility when you use datasets presented at Dataset Ninja, as well as other information, including visualizations and statistics we provide. You are in charge of compliance with any dataset license and all other permissions. You are required to navigate datasets homepage and make sure that you can use it. In case of any questions, get in touch with us at hello@datasetninja.com.