Introduction #
The provided Chest Xray Masks and Labels dataset includes X-rays along with their corresponding masks. Notably, some masks may be absent, so cross-referencing images and masks is recommended. This dataset is derived from a modification of an original dataset, which combines Shenzhen and Montgomery County publicly available chest X-ray datasets.
The authors of the dataset address the significant global health concern posed by tuberculosis (TB) and the growing interest in developing computer-aided diagnostic systems for TB detection. They recognize that progress in this field has been hindered by the scarcity of publicly available radiographs suitable for training machine learning algorithms for diagnostic systems. In response, the U.S. National Library of Medicine has released two datasets of postero-anterior (PA) chest radiographs, namely the Montgomery County (MC) set and the Shenzhen set, for research purposes. Both sets include clinical readings, and the MC set also offers manually segmented lung masks, enhancing the scope for evaluating automatic lung segmentation methods. These datasets were de-identified and exempted from institutional review board (IRB) review. Initial classification and lung segmentation results have been presented, serving as benchmarks for the research community.
The Montgomery County chest X-ray set (MC) was collected in collaboration with the Department of Health and Human Services in Maryland, USA. Comprising 138 frontal chest X-rays, this set includes both normal cases (80) and cases with TB manifestations (58). Captured using a stationary X-ray machine, the images are provided in 12-bit gray level PNG format, with the option of obtaining them in DICOM format. The image dimensions are either 4,020×4,892 or 4,892×4,020 pixels. Each reading contains patient information including age, gender, and observed lung abnormalities. Additionally, manual lung segmentations are available, created under radiologist supervision.
Here is the pattern of a typical medical reading:
Patient’s Sex: F
Patient’s Age: 031Y
cavitary nodular infiltrate in RUL; active TB
The Shenzhen chest X-ray set was gathered in collaboration with Shenzhen No.3 People’s Hospital in China. This set comprises 662 frontal chest X-rays, encompassing normal cases (326) and cases with TB manifestations (336), including pediatric X-rays (AP). The X-rays were captured as part of routine outpatient clinic activities over a 1-month period using a Philips DR Digital Diagnost system. Provided in PNG format, these X-rays have an approximate size of 3K × 3K pixels. Each reading includes patient details and observed lung abnormalities.
Here is the pattern of a typical medical reading:
male 46yrs
bilateral PTB
Both sets contribute valuable resources to the research community, enabling the development and assessment of computer-aided diagnostic systems for TB detection.
Summary #
Chest Xray Masks and Labels is a dataset for instance segmentation, semantic segmentation, and object detection tasks. It is used in the medical industry.
The dataset consists of 896 images with 1627 labeled objects belonging to 2 different classes including healthy lung and tuberculosis lung.
Images in the Chest Xray Masks and Labels dataset have pixel-level instance segmentation annotations. Due to the nature of the instance segmentation task, it can be automatically transformed into a semantic segmentation (only one mask for every class) or object detection (bounding boxes for every object) tasks. There are 192 (21% of the total) unlabeled images (i.e. without annotations). There are 2 splits in the dataset: CXRpng-train (800 images) and test (96 images). Alternatively, the dataset could be split into 2 origins of data: Shenzhen (662 images) and Montgomery County (138 images). Additionaly, every image has info with text medical recordings. The dataset was released in 2019 by the National Institutes of Health, USA, The Chinese University of Hong Kong, China, and The Shenzhen No. 3 People’s Hospital, China.
Here is the visualized example grid with animated annotations:
Explore #
Chest Xray Masks and Labels dataset has 896 images. Click on one of the examples below or open "Explore" tool anytime you need to view dataset images with annotations. This tool has extended visualization capabilities like zoom, translation, objects table, custom filters and more. Hover the mouse over the images to hide or show annotations.
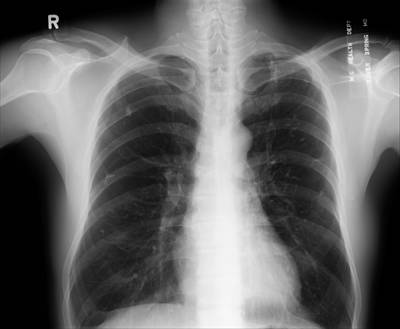
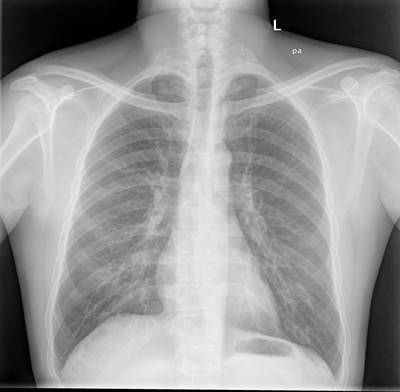
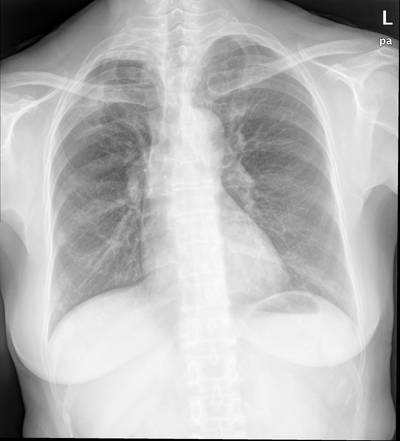
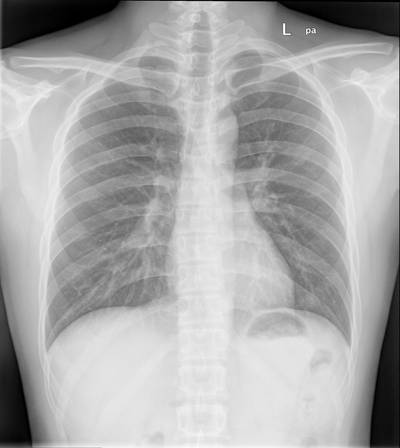
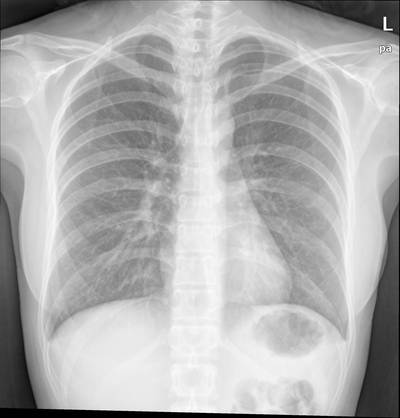
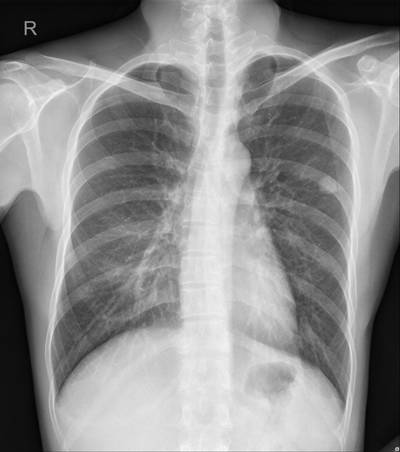
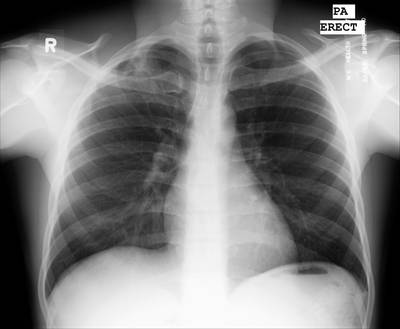

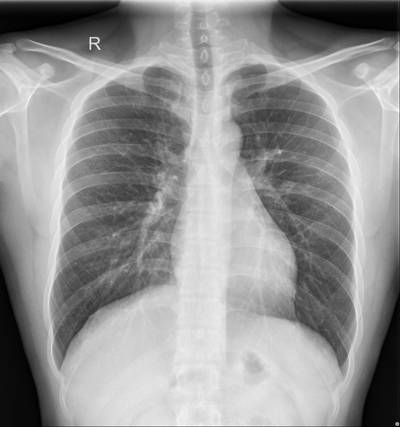
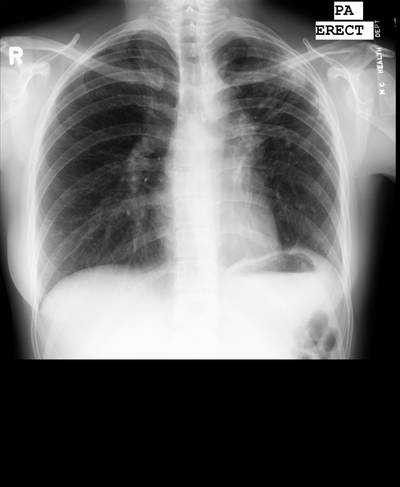
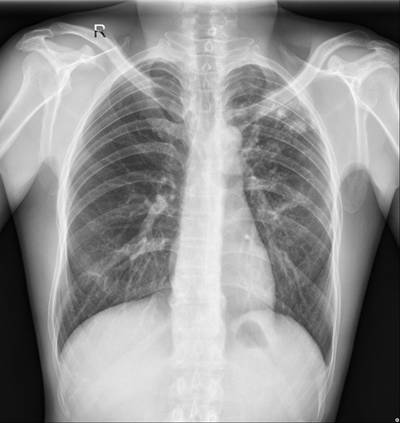
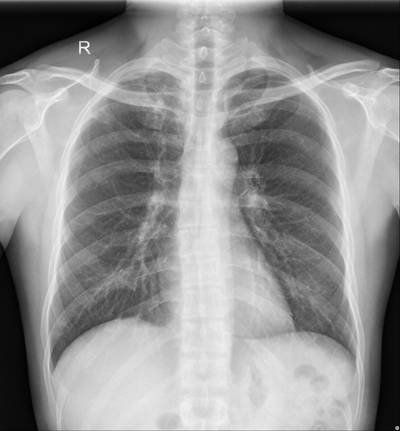
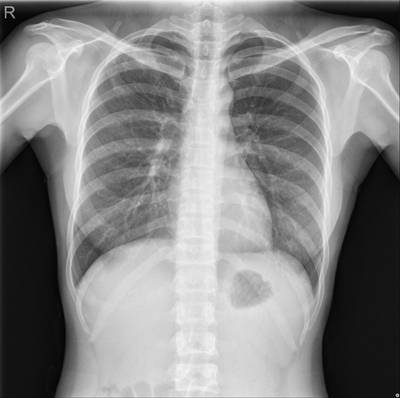
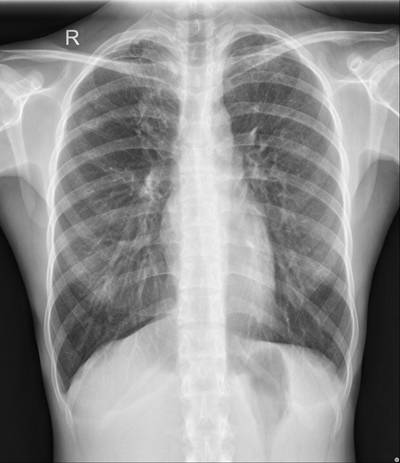
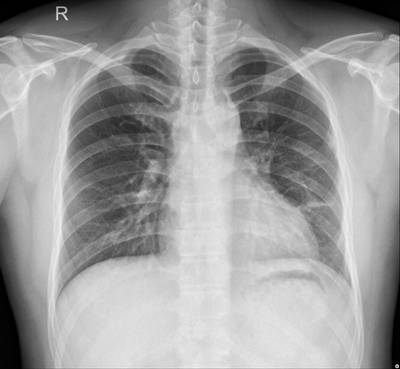
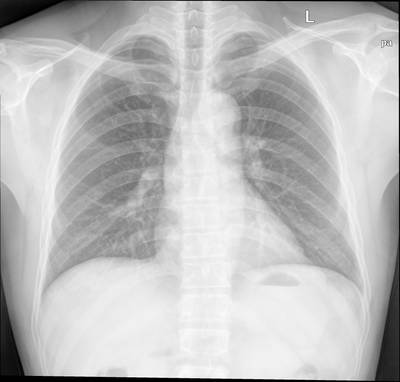
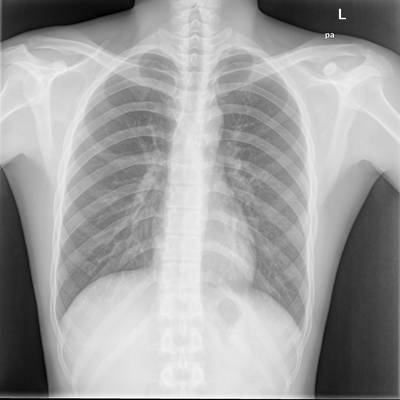
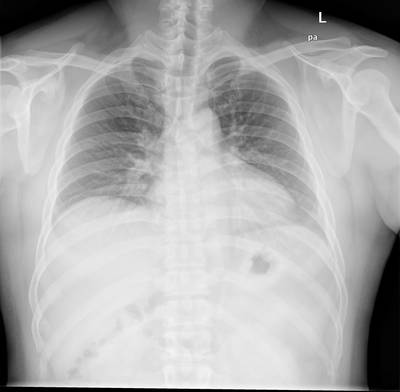
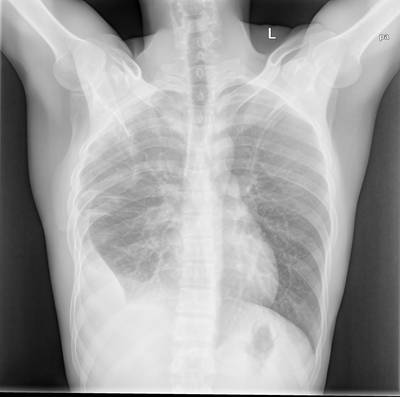
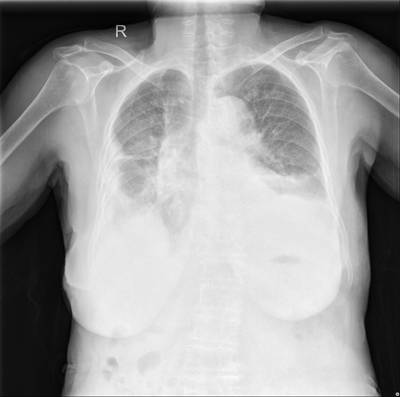
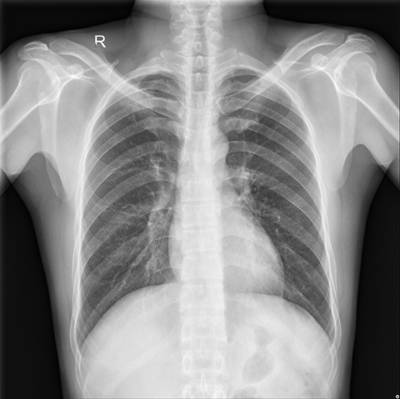
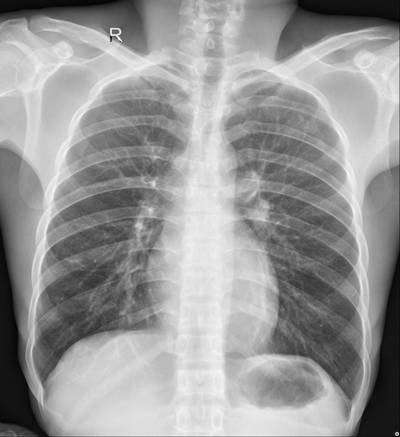
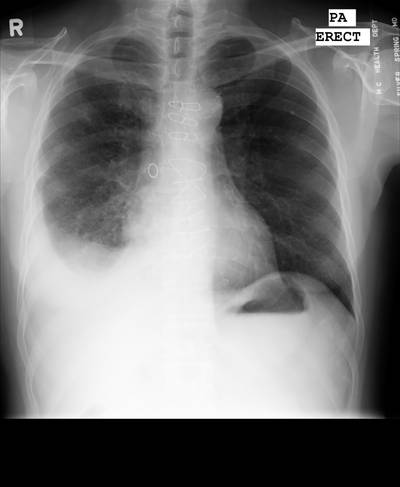
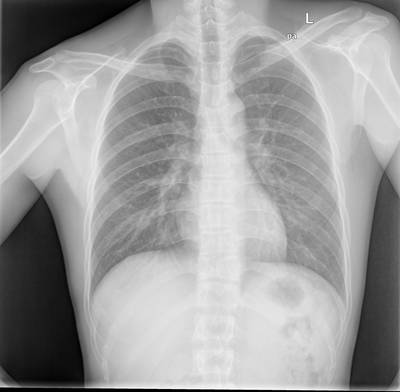

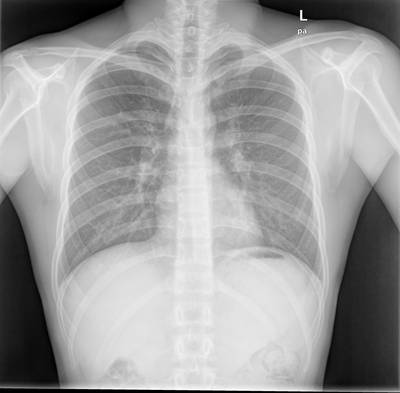
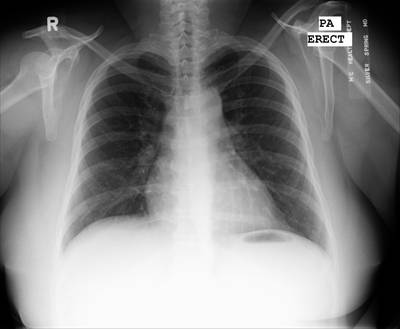

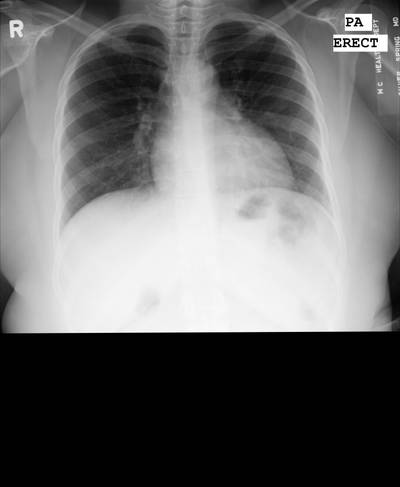
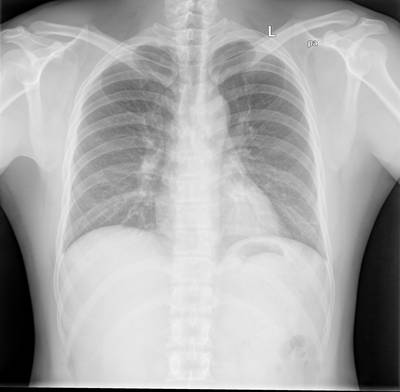
Class balance #
There are 2 annotation classes in the dataset. Find the general statistics and balances for every class in the table below. Click any row to preview images that have labels of the selected class. Sort by column to find the most rare or prevalent classes.
Class ã…¤ | Images ã…¤ | Objects ã…¤ | Count on image average | Area on image average |
---|---|---|---|---|
healthy lungâž” mask | 359 | 819 | 2.28 | 23.56% |
tuberculosis lungâž” mask | 345 | 808 | 2.34 | 27.15% |
Co-occurrence matrix #
Co-occurrence matrix is an extremely valuable tool that shows you the images for every pair of classes: how many images have objects of both classes at the same time. If you click any cell, you will see those images. We added the tooltip with an explanation for every cell for your convenience, just hover the mouse over a cell to preview the description.
Images #
Explore every single image in the dataset with respect to the number of annotations of each class it has. Click a row to preview selected image. Sort by any column to find anomalies and edge cases. Use horizontal scroll if the table has many columns for a large number of classes in the dataset.
Object distribution #
Interactive heatmap chart for every class with object distribution shows how many images are in the dataset with a certain number of objects of a specific class. Users can click cell and see the list of all corresponding images.
Class sizes #
The table below gives various size properties of objects for every class. Click a row to see the image with annotations of the selected class. Sort columns to find classes with the smallest or largest objects or understand the size differences between classes.
Class | Object count | Avg area | Max area | Min area | Min height | Min height | Max height | Max height | Avg height | Avg height | Min width | Min width | Max width | Max width |
---|---|---|---|---|---|---|---|---|---|---|---|---|---|---|
healthy lung mask | 819 | 10.33% | 21.98% | 0% | 1px | 0.02% | 3671px | 91.32% | 1749px | 55.05% | 1px | 0.02% | 1980px | 43.76% |
tuberculosis lung mask | 808 | 11.59% | 25.8% | 0% | 1px | 0.02% | 4043px | 89.63% | 1798px | 58.17% | 1px | 0.02% | 1906px | 46.72% |
Spatial Heatmap #
The heatmaps below give the spatial distributions of all objects for every class. These visualizations provide insights into the most probable and rare object locations on the image. It helps analyze objects' placements in a dataset.
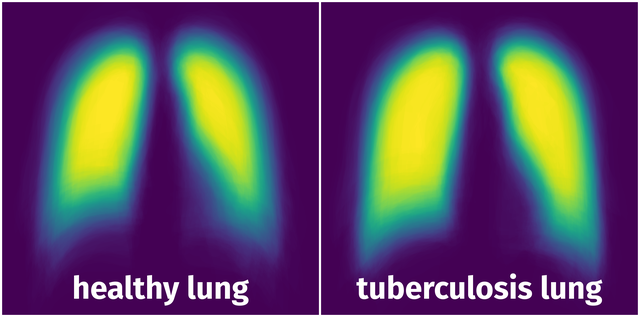
Objects #
Table contains all 1627 objects. Click a row to preview an image with annotations, and use search or pagination to navigate. Sort columns to find outliers in the dataset.
Object ID ã…¤ | Class ã…¤ | Image name click row to open | Image size height x width | Height ã…¤ | Height ã…¤ | Width ã…¤ | Width ã…¤ | Area ã…¤ |
---|---|---|---|---|---|---|---|---|
1âž” | tuberculosis lung mask | CHNCXR_0612_1.png | 2929 x 2735 | 2099px | 71.66% | 977px | 35.72% | 12.65% |
2âž” | tuberculosis lung mask | CHNCXR_0612_1.png | 2929 x 2735 | 1px | 0.03% | 2px | 0.07% | 0% |
3âž” | tuberculosis lung mask | CHNCXR_0612_1.png | 2929 x 2735 | 1px | 0.03% | 1px | 0.04% | 0% |
4âž” | tuberculosis lung mask | CHNCXR_0612_1.png | 2929 x 2735 | 2px | 0.07% | 6px | 0.22% | 0% |
5âž” | tuberculosis lung mask | CHNCXR_0612_1.png | 2929 x 2735 | 1px | 0.03% | 2px | 0.07% | 0% |
6âž” | tuberculosis lung mask | CHNCXR_0612_1.png | 2929 x 2735 | 2062px | 70.4% | 857px | 31.33% | 13.68% |
7âž” | tuberculosis lung mask | MCUCXR_0383_1.png | 4892 x 4020 | 2904px | 59.36% | 1273px | 31.67% | 10.89% |
8âž” | tuberculosis lung mask | MCUCXR_0383_1.png | 4892 x 4020 | 2664px | 54.46% | 1309px | 32.56% | 12.16% |
9âž” | healthy lung mask | CHNCXR_0047_0.png | 2927 x 2699 | 2054px | 70.17% | 1062px | 39.35% | 17.1% |
10âž” | healthy lung mask | CHNCXR_0047_0.png | 2927 x 2699 | 2000px | 68.33% | 900px | 33.35% | 16.89% |
License #
Citation #
If you make use of the Chest Xray Masks and Labels data, please cite the following reference:
Jaeger S, Karargyris A, Candemir S, Folio L, Siegelman J, Callaghan F, Xue Z, Palaniappan K, Singh RK, Antani S, Thoma G, Wang YX, Lu PX, McDonald CJ. Automatic tuberculosis screening using chest radiographs. IEEE Trans Med Imaging. 2014 Feb;33(2):233-45. doi: 10.1109/TMI.2013.2284099. PMID: 24108713
Candemir S, Jaeger S, Palaniappan K, Musco JP, Singh RK, Xue Z, Karargyris A, Antani S, Thoma G, McDonald CJ. Lung segmentation in chest radiographs using anatomical atlases with nonrigid registration. IEEE Trans Med Imaging. 2014 Feb;33(2):577-90. doi: 10.1109/TMI.2013.2290491. PMID: 24239990
If you are happy with Dataset Ninja and use provided visualizations and tools in your work, please cite us:
@misc{ visualization-tools-for-chest-xray-dataset,
title = { Visualization Tools for Chest Xray Masks and Labels Dataset },
type = { Computer Vision Tools },
author = { Dataset Ninja },
howpublished = { \url{ https://datasetninja.com/chest-xray } },
url = { https://datasetninja.com/chest-xray },
journal = { Dataset Ninja },
publisher = { Dataset Ninja },
year = { 2025 },
month = { mar },
note = { visited on 2025-03-26 },
}
Download #
Dataset Chest Xray Masks and Labels can be downloaded in Supervisely format:
As an alternative, it can be downloaded with dataset-tools package:
pip install --upgrade dataset-tools
… using following python code:
import dataset_tools as dtools
dtools.download(dataset='Chest Xray Masks and Labels', dst_dir='~/dataset-ninja/')
Make sure not to overlook the python code example available on the Supervisely Developer Portal. It will give you a clear idea of how to effortlessly work with the downloaded dataset.
The data in original format can be downloaded here.
Disclaimer #
Our gal from the legal dep told us we need to post this:
Dataset Ninja provides visualizations and statistics for some datasets that can be found online and can be downloaded by general audience. Dataset Ninja is not a dataset hosting platform and can only be used for informational purposes. The platform does not claim any rights for the original content, including images, videos, annotations and descriptions. Joint publishing is prohibited.
You take full responsibility when you use datasets presented at Dataset Ninja, as well as other information, including visualizations and statistics we provide. You are in charge of compliance with any dataset license and all other permissions. You are required to navigate datasets homepage and make sure that you can use it. In case of any questions, get in touch with us at hello@datasetninja.com.