Introduction #
The authors create CholecSeg8k: A Semantic Segmentation Dataset for Laparoscopic Cholecystectomy Based on Cholec80 by extracting 8,080 laparoscopic cholecystectomy image frames from 17 video clips in Cholec80 and annotated the images. Each of these images are annotated at pixel level for thirteen classes, which are commonly founded in laparoscopic cholecystectomy surgery.
Motivation
Endoscopy serves as a vital procedure for detecting, diagnosing, and treating conditions in organs that are typically challenging to examine without resorting to surgery, such as the esophagus, stomach, and colon. In clinical settings, an endoscopist guides the endoscope using a handle while simultaneously observing the recorded output video on an external monitor. However, the success of endoscopic procedures heavily relies on the operator’s level of training and expertise. Factors like unsteady hand control and organ movement can significantly impact the accuracy of image analysis and surgical interventions. To aid surgeons in conducting endoscopic surgeries, various computer-assisted systems have been developed. These systems offer guidance and relevant contextual information to surgeons during operations. Some employ magnetic or radio-based external sensors to estimate and track the endoscope’s position within the patient’s body. However, these methods are susceptible to errors, making it challenging to achieve sub-centimeter localization accuracy.
An alternative approach, Simultaneous Localization and Mapping (SLAM), offers promising solutions. SLAM utilizes an image-based method to localize the camera with pixel-level accuracy, requiring no external infrastructure. This technique creates a real-time 3D map by comparing sensed data with reference data, providing an estimate of the camera’s position in 3D space. Fortunately, reference data can be pre-collected using advanced technologies like 256-beam LiDAR and GPS-RTK to create high-definition point cloud maps. During runtime, less complex LiDAR systems can collect point cloud data, enabling localization with an accuracy of up to 5 centimeters. Implementing SLAM for endoscope navigation necessitates accurate semantic segmentation of images. This step is crucial for ensuring precise localization and navigation during procedures.
Accurate SLAM relies heavily on utilizing appropriate reference data for comparison. While GPS is commonly used in outdoor environments to obtain large-scale location data and retrieve corresponding reference data indexed by GPS coordinates, this method is impractical for endoscopic procedures. To address this challenge, one potential approach involves identifying the organs depicted in the images and using this information to query for the relevant reference data. The initial step can be accomplished through either semantic segmentation, which assigns object classes to each pixel, or object detection, which identifies object classes within bounding boxes. However, both semantic segmentation and object detection require well-labeled image datasets to train prediction networks, a resource that is not readily available for endoscope images.
Dataset description
The authors construct an open semantic segmentation endoscopic dataset, which is available to medical and computer vision communities. This dataset consists of in total 8,080 frames extracted from 17 video clips in Cholec80 dataset. The annotation has thirteen classes.
Example of Semantic Segmentation Label of Endoscope Image.
The CholecSeg8K dataset builds upon the endoscopic images sourced from Cholec80, a dataset originally published by the CAMMA (Computational Analysis and Modeling of Medical Activities) research group. This collaborative effort involved the University Hospital of Strasbourg, IHU Strasbourg, and IRCAD. The Cholec80 dataset comprises 80 videos capturing cholecystectomy surgeries conducted by 13 surgeons. Each video in Cholec80 was recorded at a frame rate of 25 frames per second and includes annotations detailing instruments and operation phases. In their work, the authors of CholecSeg8K selected a subset of closely related videos from the Cholec80 dataset. They then meticulously annotated semantic segmentation masks on frames extracted from these chosen videos.
Data in CholecSeg8K Dataset are grouped into a two level directory for better organization and accessibility. Each directory on the first level collected the data of the video id clips extract from Cholec80 and is named by the filename of the video clips. Each directory on the secondary level tree stores the data for 80 images from the video clip and is named by the video filename and the frame index of the first image in the selected video clip. Each secondary level directory stores the raw image data, annotation, and color masks for 80 frames. There are a total of 101 directories and the total number of frames is 8,080. The resolution of each image is 854 pixels × 480 pixels.
During the pixel annotation process, the annotation classes were meticulously crafted to focus specifically on cholecystectomy surgeries, the primary operations targeted within the Cholec80 dataset. In particular, these annotation classes were designed to identify key anatomical structures such as the liver and gallbladder. To ensure comprehensive coverage, additional annotation classes were defined to encompass elements beyond the immediate scope of the surgeries. Notably, two classes were established with broader coverage. The first encompasses the gastrointestinal tract, encompassing structures like the stomach, small intestine, and adjacent tissues. The second class pertains to liver ligaments, including the coronary ligament, triangular ligament, falciform ligament, ligamentum teres (hepatis), ligamentum venosum, and lesser omentum. In this dataset, not all 13 classes appear in every frame at the same time.
Class ID | Class Name |
---|---|
Class 0 | Black Background |
Class 1 | Abdominal Wall |
Class 2 | Liver |
Class 3 | Gastrointestinal Tract |
Class 4 | Fat |
Class 5 | Grasper |
Class 6 | Connective Tissue |
Class 7 | Blood |
Class 8 | Cystic Duct |
Class 9 | L-hook Electrocautery |
Class 10 | Gallbladder |
Class 11 | Hepatic Vein |
Class 12 | Liver Ligament |
Class numbers and their corresponding class names.
The classes are not well balanced, which may lead to poor training results if using without cautions.
Group with larger proportion | Group with smaller proportion |
---|---|
Example of Semantic Segmentation Annotation of Gallbladder Endoscope Image.
Summary #
CholecSeg8k: A Semantic Segmentation Dataset for Laparoscopic Cholecystectomy Based on Cholec80 is a dataset for a semantic segmentation task. It is used in the medical industry.
The dataset consists of 8080 images with 53690 labeled objects belonging to 13 different classes including liver, black background, fat, and other: abdominal wall, gallbladder, grasper, gastrointestinal tract, l-hook electrocautery, connective tissue, blood, hepatic vein, cystic duct, and liver ligament.
Images in the CholecSeg8k dataset have pixel-level semantic segmentation annotations. All images are labeled (i.e. with annotations). There are no pre-defined train/val/test splits in the dataset. Additionally, every image marked with its video id and sequence tags. The dataset was released in 2020 by the National University, Taiwan.
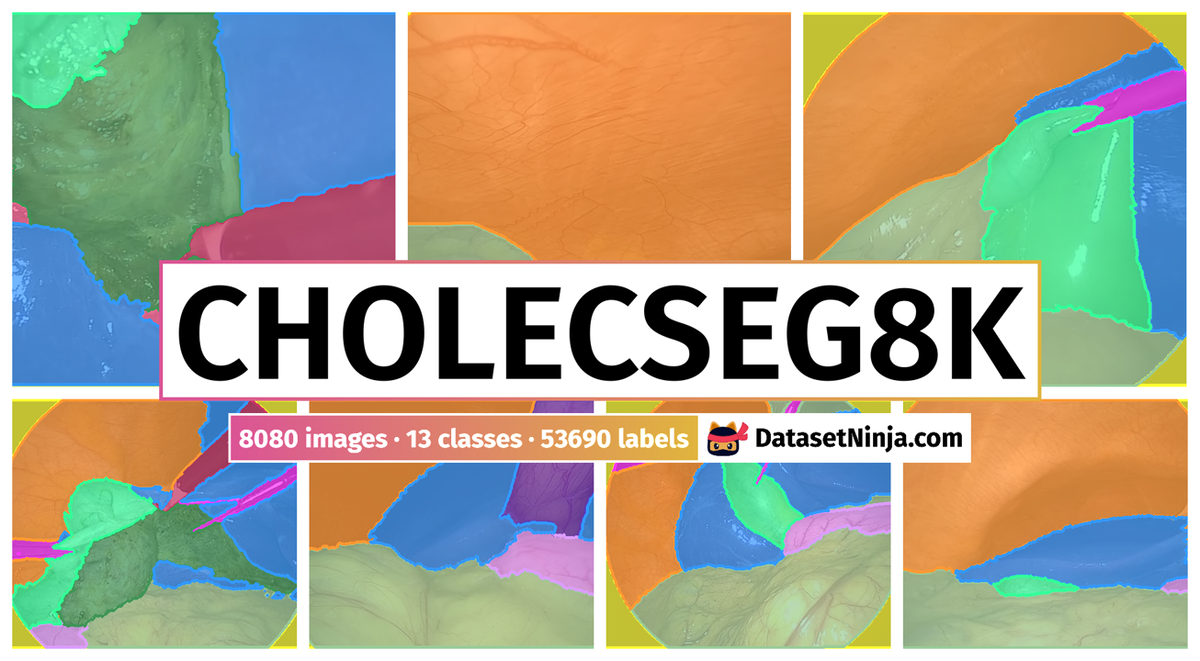
Explore #
CholecSeg8k dataset has 8080 images. Click on one of the examples below or open "Explore" tool anytime you need to view dataset images with annotations. This tool has extended visualization capabilities like zoom, translation, objects table, custom filters and more. Hover the mouse over the images to hide or show annotations.
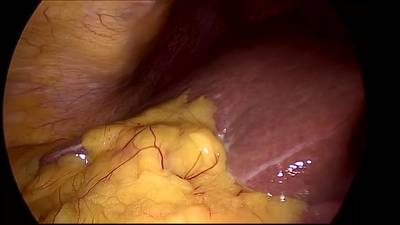
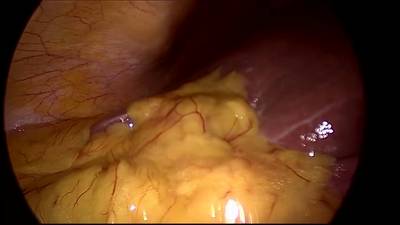
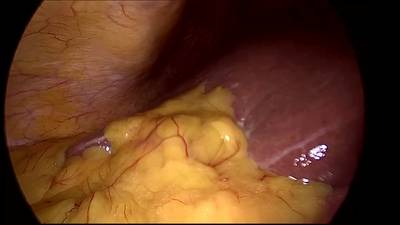
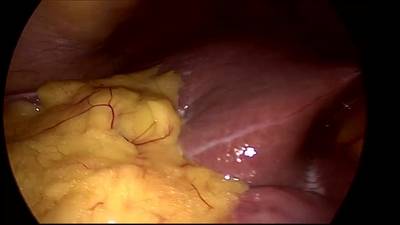
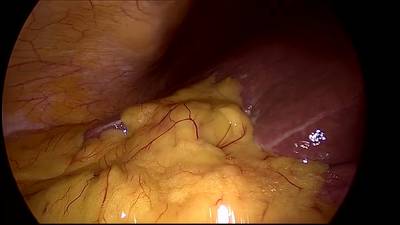
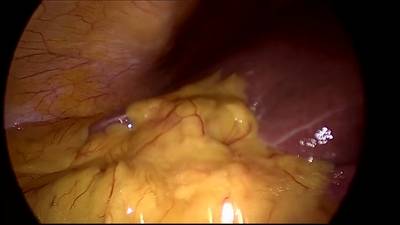
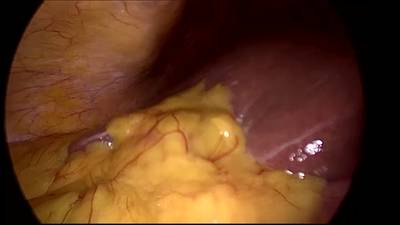
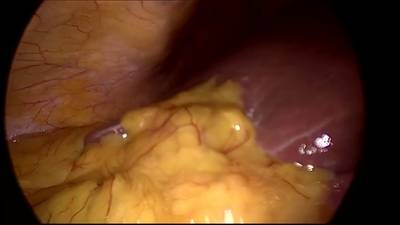

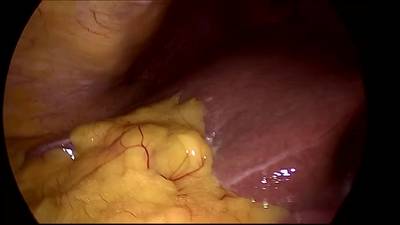

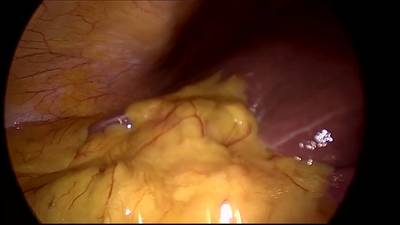

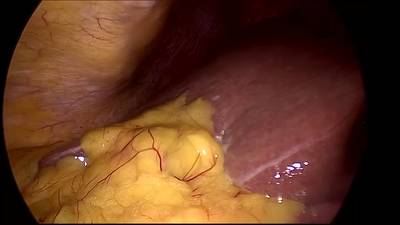


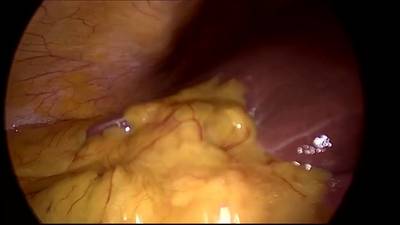
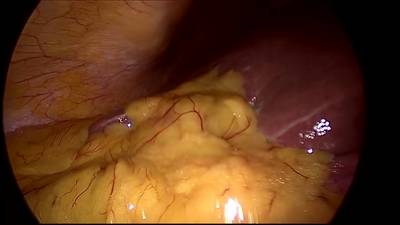
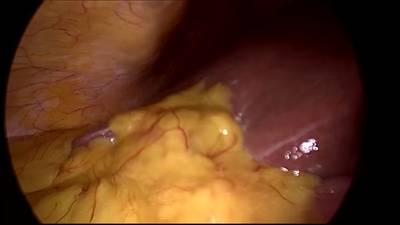
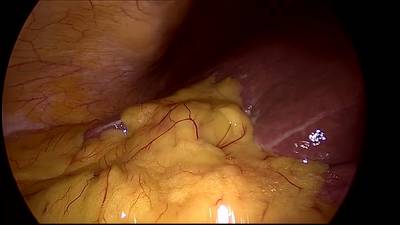
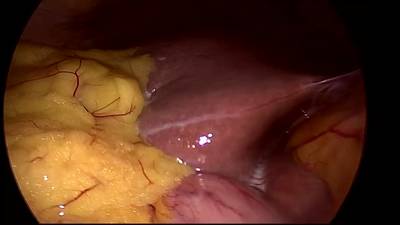

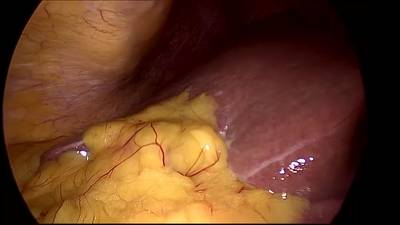
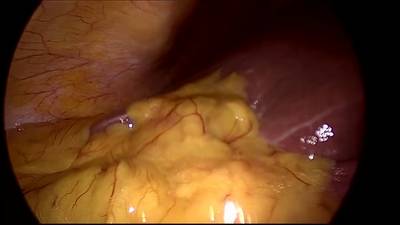
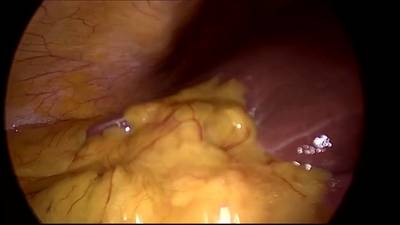
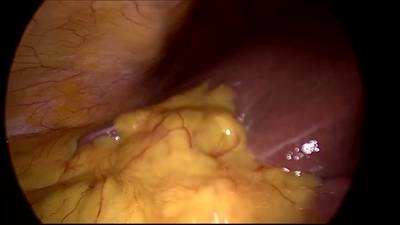

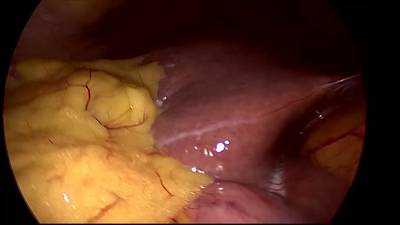
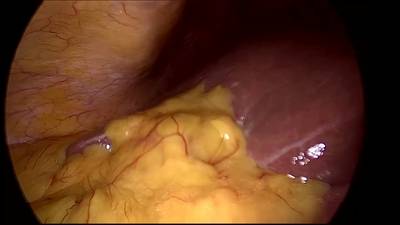
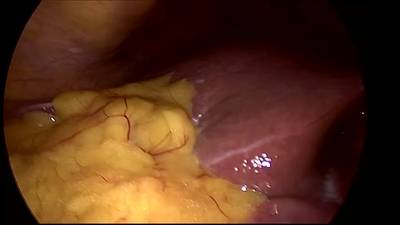
Class balance #
There are 13 annotation classes in the dataset. Find the general statistics and balances for every class in the table below. Click any row to preview images that have labels of the selected class. Sort by column to find the most rare or prevalent classes.
Class ã…¤ | Images ã…¤ | Objects ã…¤ | Count on image average | Area on image average |
---|---|---|---|---|
liverâž” mask | 8080 | 8080 | 1 | 21.27% |
black backgroundâž” mask | 8055 | 8055 | 1 | 26.58% |
fatâž” mask | 7510 | 7510 | 1 | 15.84% |
abdominal wallâž” mask | 7255 | 7255 | 1 | 24.02% |
gallbladderâž” mask | 6861 | 6861 | 1 | 7.57% |
grasperâž” mask | 6020 | 6020 | 1 | 3.25% |
gastrointestinal tractâž” mask | 4558 | 4558 | 1 | 3.33% |
l-hook electrocauteryâž” mask | 2254 | 2254 | 1 | 4.7% |
connective tissueâž” mask | 1600 | 1600 | 1 | 11.45% |
bloodâž” mask | 692 | 692 | 1 | 4.93% |
Co-occurrence matrix #
Co-occurrence matrix is an extremely valuable tool that shows you the images for every pair of classes: how many images have objects of both classes at the same time. If you click any cell, you will see those images. We added the tooltip with an explanation for every cell for your convenience, just hover the mouse over a cell to preview the description.
Images #
Explore every single image in the dataset with respect to the number of annotations of each class it has. Click a row to preview selected image. Sort by any column to find anomalies and edge cases. Use horizontal scroll if the table has many columns for a large number of classes in the dataset.
Object distribution #
Interactive heatmap chart for every class with object distribution shows how many images are in the dataset with a certain number of objects of a specific class. Users can click cell and see the list of all corresponding images.
Class sizes #
The table below gives various size properties of objects for every class. Click a row to see the image with annotations of the selected class. Sort columns to find classes with the smallest or largest objects or understand the size differences between classes.
Class | Object count | Avg area | Max area | Min area | Min height | Min height | Max height | Max height | Avg height | Avg height | Min width | Min width | Max width | Max width |
---|---|---|---|---|---|---|---|---|---|---|---|---|---|---|
liver mask | 8080 | 21.27% | 64.9% | 2.97% | 89px | 18.54% | 478px | 99.58% | 326px | 67.84% | 160px | 18.74% | 852px | 99.77% |
black background mask | 8055 | 26.58% | 41.88% | 5.68% | 478px | 99.58% | 480px | 100% | 478px | 99.58% | 247px | 28.92% | 854px | 100% |
fat mask | 7510 | 15.84% | 41.69% | 0.34% | 23px | 4.79% | 478px | 99.58% | 305px | 63.59% | 97px | 11.36% | 833px | 97.54% |
abdominal wall mask | 7255 | 24.02% | 70.34% | 0.04% | 14px | 2.92% | 478px | 99.58% | 333px | 69.39% | 23px | 2.69% | 852px | 99.77% |
gallbladder mask | 6861 | 7.57% | 26.99% | 0.01% | 9px | 1.88% | 478px | 99.58% | 220px | 45.84% | 14px | 1.64% | 829px | 97.07% |
grasper mask | 6020 | 3.25% | 19.57% | 0.05% | 8px | 1.67% | 478px | 99.58% | 198px | 41.34% | 15px | 1.76% | 773px | 90.52% |
gastrointestinal tract mask | 4558 | 3.33% | 20.76% | 0% | 1px | 0.21% | 478px | 99.58% | 102px | 21.26% | 1px | 0.12% | 684px | 80.09% |
l-hook electrocautery mask | 2254 | 4.7% | 16.1% | 0% | 5px | 1.04% | 478px | 99.58% | 159px | 33.17% | 7px | 0.82% | 746px | 87.35% |
connective tissue mask | 1600 | 11.45% | 30.39% | 2.89% | 121px | 25.21% | 478px | 99.58% | 264px | 54.93% | 152px | 17.8% | 759px | 88.88% |
blood mask | 692 | 4.93% | 11.32% | 0.02% | 4px | 0.83% | 478px | 99.58% | 323px | 67.26% | 14px | 1.64% | 759px | 88.88% |
Spatial Heatmap #
The heatmaps below give the spatial distributions of all objects for every class. These visualizations provide insights into the most probable and rare object locations on the image. It helps analyze objects' placements in a dataset.
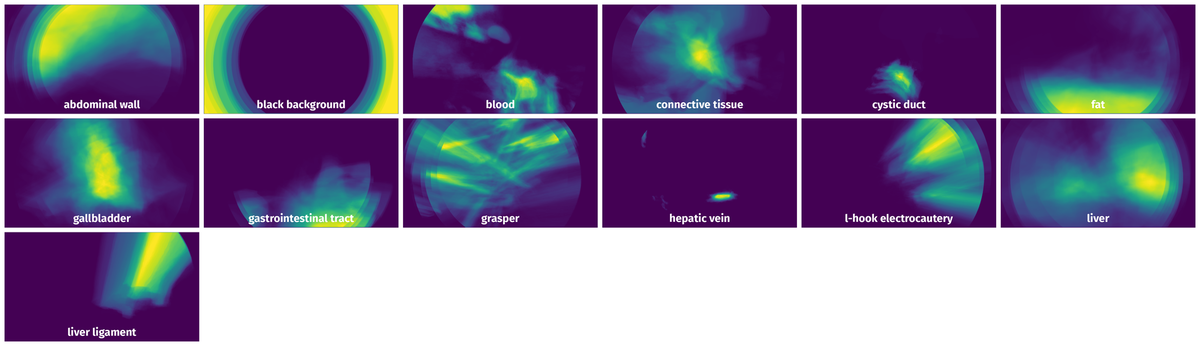
Objects #
Table contains all 53690 objects. Click a row to preview an image with annotations, and use search or pagination to navigate. Sort columns to find outliers in the dataset.
Object ID ã…¤ | Class ã…¤ | Image name click row to open | Image size height x width | Height ã…¤ | Height ã…¤ | Width ã…¤ | Width ã…¤ | Area ã…¤ |
---|---|---|---|---|---|---|---|---|
1âž” | hepatic vein mask | video12_frame_19521_endo.png | 480 x 854 | 44px | 9.17% | 17px | 1.99% | 0.1% |
2âž” | black background mask | video12_frame_19521_endo.png | 480 x 854 | 478px | 99.58% | 852px | 99.77% | 35.07% |
3âž” | l-hook electrocautery mask | video12_frame_19521_endo.png | 480 x 854 | 208px | 43.33% | 323px | 37.82% | 4.93% |
4âž” | grasper mask | video12_frame_19521_endo.png | 480 x 854 | 30px | 6.25% | 127px | 14.87% | 0.54% |
5âž” | fat mask | video12_frame_19521_endo.png | 480 x 854 | 449px | 93.54% | 608px | 71.19% | 16.78% |
6âž” | abdominal wall mask | video12_frame_19521_endo.png | 480 x 854 | 355px | 73.96% | 358px | 41.92% | 6.26% |
7âž” | connective tissue mask | video12_frame_19521_endo.png | 480 x 854 | 321px | 66.88% | 461px | 53.98% | 11.26% |
8âž” | liver mask | video12_frame_19521_endo.png | 480 x 854 | 444px | 92.5% | 511px | 59.84% | 22.55% |
9âž” | gallbladder mask | video12_frame_19521_endo.png | 480 x 854 | 83px | 17.29% | 277px | 32.44% | 1.87% |
10âž” | black background mask | video12_frame_19746_endo.png | 480 x 854 | 478px | 99.58% | 852px | 99.77% | 35% |
License #
CholecSeg8k: A Semantic Segmentation Dataset for Laparoscopic Cholecystectomy is under CC BY-NC-SA 4.0 license.
Citation #
If you make use of the CholecSeg8k data, please cite the following reference:
@dataset{CholecSeg8k,
author={W.-Y. Hong and C.-L. Kao and Y.-H. Kuo and J.-R. Wang and W.-L. Chang and C.-S. Shih},
title={CholecSeg8k: A Semantic Segmentation Dataset for Laparoscopic Cholecystectomy},
year={2020},
url={https://www.kaggle.com/datasets/newslab/cholecseg8k}
}
If you are happy with Dataset Ninja and use provided visualizations and tools in your work, please cite us:
@misc{ visualization-tools-for-cholec-seg8k-dataset,
title = { Visualization Tools for CholecSeg8k Dataset },
type = { Computer Vision Tools },
author = { Dataset Ninja },
howpublished = { \url{ https://datasetninja.com/cholec-seg8k } },
url = { https://datasetninja.com/cholec-seg8k },
journal = { Dataset Ninja },
publisher = { Dataset Ninja },
year = { 2025 },
month = { feb },
note = { visited on 2025-02-05 },
}
Download #
Dataset CholecSeg8k can be downloaded in Supervisely format:
As an alternative, it can be downloaded with dataset-tools package:
pip install --upgrade dataset-tools
… using following python code:
import dataset_tools as dtools
dtools.download(dataset='CholecSeg8k', dst_dir='~/dataset-ninja/')
Make sure not to overlook the python code example available on the Supervisely Developer Portal. It will give you a clear idea of how to effortlessly work with the downloaded dataset.
The data in original format can be downloaded here.
Disclaimer #
Our gal from the legal dep told us we need to post this:
Dataset Ninja provides visualizations and statistics for some datasets that can be found online and can be downloaded by general audience. Dataset Ninja is not a dataset hosting platform and can only be used for informational purposes. The platform does not claim any rights for the original content, including images, videos, annotations and descriptions. Joint publishing is prohibited.
You take full responsibility when you use datasets presented at Dataset Ninja, as well as other information, including visualizations and statistics we provide. You are in charge of compliance with any dataset license and all other permissions. You are required to navigate datasets homepage and make sure that you can use it. In case of any questions, get in touch with us at hello@datasetninja.com.