Introduction #
To benchmark the more challenging multi-person human parsing task, authors build a large-scale dataset called the CIHP: Crowd Instance-level Human Parsing dataset, which has several appealing properties. First, with 38,280 diverse human images, it is the largest multi-person human parsing dataset to date. Second, CIHP is annotated with rich information on items with persons. The images in this dataset are labeled with pixel-wise annotations on 20 categories and instance-level identification. Third, the images collected from the real-world scenarios contain people appearing with challenging poses and viewpoints, heavy occlusions, various appearances, and in a wide range of resolutions. Some examples are shown in the Figure below. With the CIHP dataset, authors propose a new benchmark for instance-level human parsing together with a standard evaluation server where the test set will be kept secret to avoid overfitting.
Left: Statistics on the number of persons in one image. Right: The data distribution on 19 semantic part labels in the CIHP dataset.
The images in the CIHP are collected from unconstrained resources like Google and Bing. authors manually specify several keywords (e.g., family, couple, party, meeting, etc.) to gain a great diversity of multi-person images. The crawled images are elaborately annotated by a professional labeling organization with good quality control. The authors supervise the whole annotation process and conduct a second-round check for each annotated image. Authors remove the unusable images that are of low resolution, or image quality, or contain one or no person instance. In total, 38,280 images are kept to construct the CIHP dataset. Following random selection, authors arrive at a unique split that consists of 28,280 training and 5,000 validation images with publicly available annotations, as well as 5,000 test images with annotations withheld for benchmarking purposes.
Authors now introduce the images and categories in the CIHP dataset with more statistical details. Superior to the previous attempts with an average of one or two-person instances in an image, all images of the CIHP dataset contain two or more instances with an average of 3.4. Generally, authors follow LIP to define and annotate the semantic part labels. However, they find that the Jumpsuit label defined in LIP is infrequent compared to other labels. To parse the human more completely and precisely, authors use a more common body part label (Tosor-skin) instead. The 19 semantic part labels in the CIHP are hat, hair, sunglasses, upper-clothes, dress, coat, socks, pants, gloves, scarf, skirt, torsoskin, face, right_arm, left_arm, right_leg, left_leg, right_shoe and left_shoe.
Summary #
CIHP: Crowd Instance-level Human Parsing is a dataset for instance segmentation, semantic segmentation, and object detection tasks. It is used in the surveillance industry.
The dataset consists of 38280 images with 768446 labeled objects belonging to 19 different classes including face, hair, torso_skin, and other: upperclothes, right_arm, left_arm, pants, coat, left_shoe, right_shoe, right_leg, left_leg, hat, dress, socks, sunglasses, skirt, scarf, and glove.
Images in the CIHP dataset have pixel-level instance segmentation annotations. Due to the nature of the instance segmentation task, it can be automatically transformed into a semantic segmentation (only one mask for every class) or object detection (bounding boxes for every object) tasks. There are 5000 (13% of the total) unlabeled images (i.e. without annotations). There are 3 splits in the dataset: training (28280 images), testing (5000 images), and validation (5000 images). The dataset was released in 2018 by the Sun Yat-sen University, SenseTime Group (Limited), and CVTE Research.
Here are the visualized examples for the classes:
Explore #
CIHP dataset has 38280 images. Click on one of the examples below or open "Explore" tool anytime you need to view dataset images with annotations. This tool has extended visualization capabilities like zoom, translation, objects table, custom filters and more. Hover the mouse over the images to hide or show annotations.
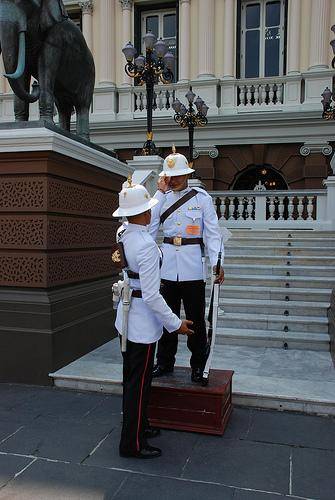
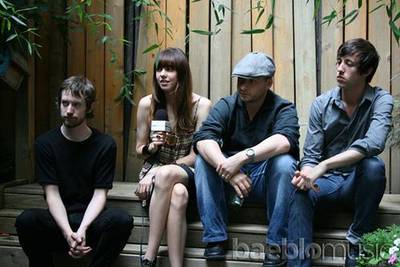
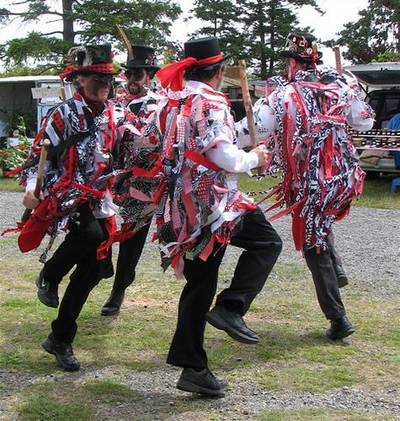
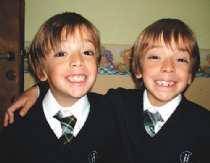
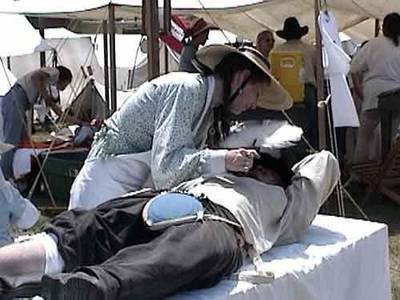
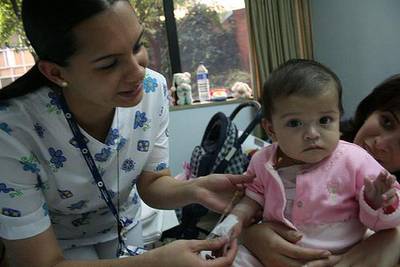
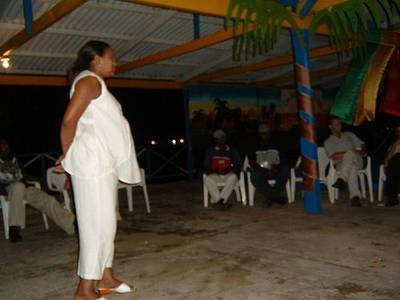
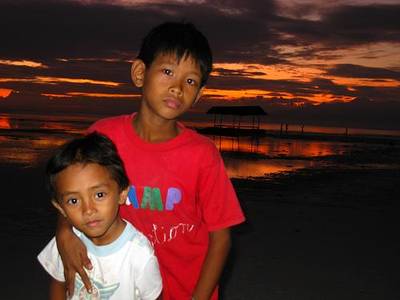
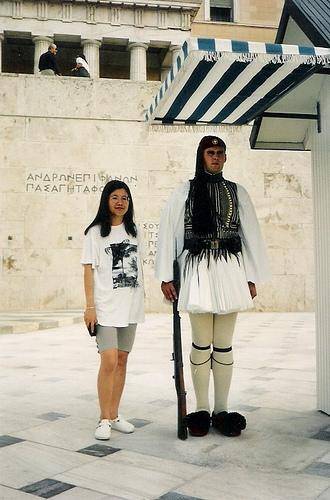

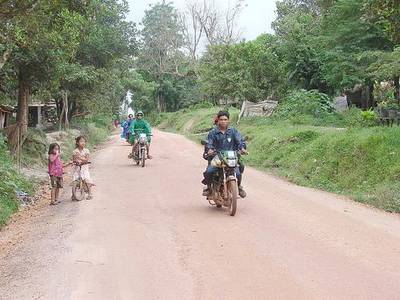
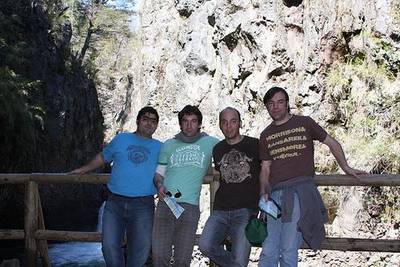
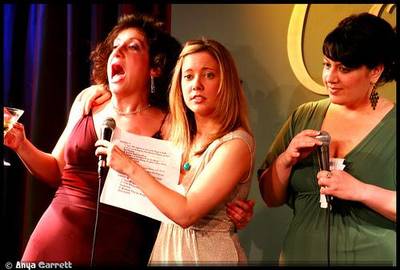
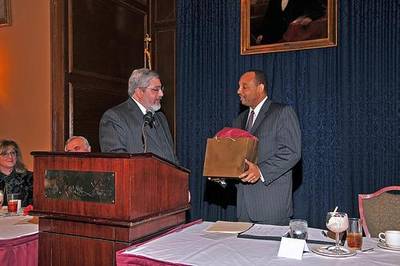
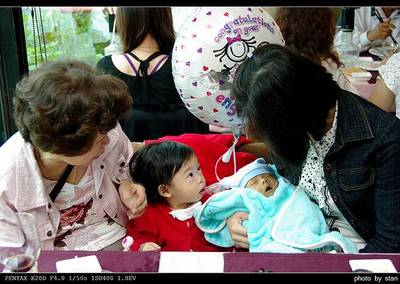
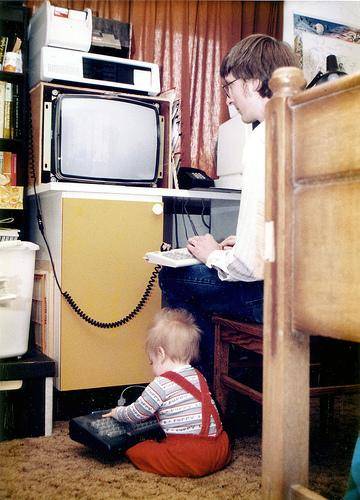
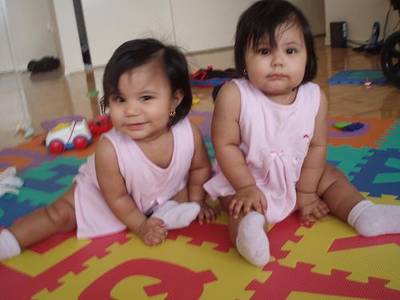
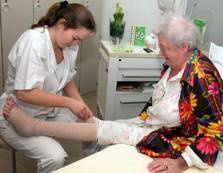
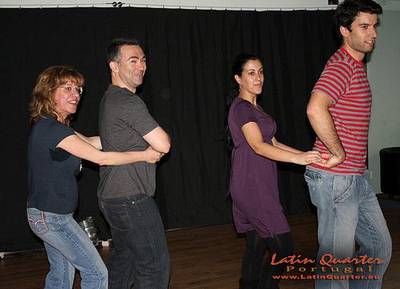
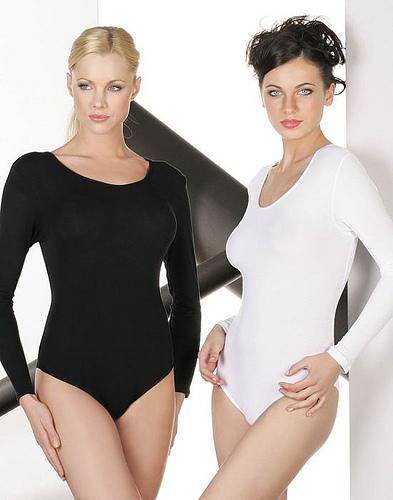
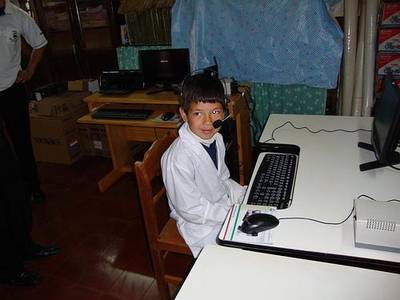
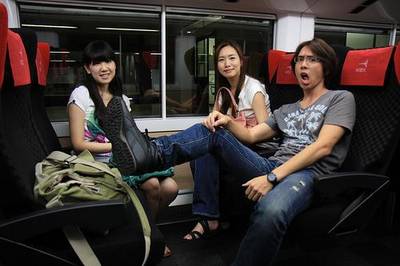
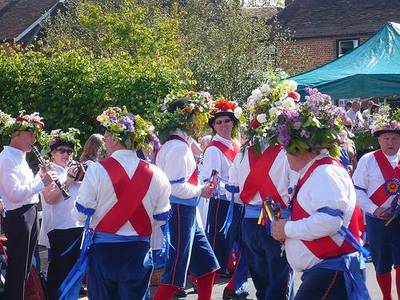


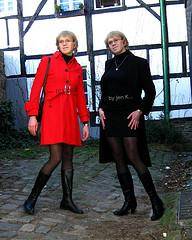
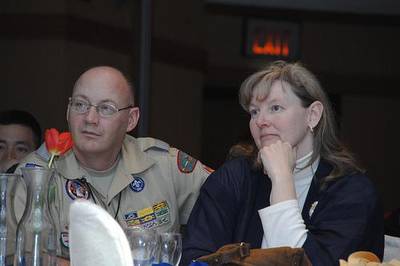
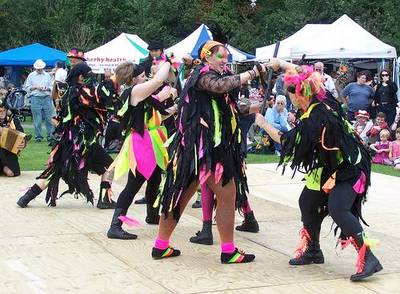


Class balance #
There are 19 annotation classes in the dataset. Find the general statistics and balances for every class in the table below. Click any row to preview images that have labels of the selected class. Sort by column to find the most rare or prevalent classes.
Class ã…¤ | Images ã…¤ | Objects ã…¤ | Count on image average | Area on image average |
---|---|---|---|---|
faceâž” mask | 33064 | 104477 | 3.16 | 4.6% |
hairâž” mask | 32489 | 99636 | 3.07 | 4.87% |
torso_skinâž” mask | 32235 | 92365 | 2.87 | 1.54% |
upperclothesâž” mask | 30400 | 85544 | 2.81 | 10.78% |
right_armâž” mask | 29740 | 73282 | 2.46 | 1.91% |
left_armâž” mask | 29308 | 70363 | 2.4 | 1.79% |
pantsâž” mask | 23124 | 60430 | 2.61 | 5.76% |
coatâž” mask | 16760 | 38731 | 2.31 | 15.15% |
left_shoeâž” mask | 11058 | 29430 | 2.66 | 0.63% |
right_shoeâž” mask | 11044 | 29437 | 2.67 | 0.64% |
Co-occurrence matrix #
Co-occurrence matrix is an extremely valuable tool that shows you the images for every pair of classes: how many images have objects of both classes at the same time. If you click any cell, you will see those images. We added the tooltip with an explanation for every cell for your convenience, just hover the mouse over a cell to preview the description.
Images #
Explore every single image in the dataset with respect to the number of annotations of each class it has. Click a row to preview selected image. Sort by any column to find anomalies and edge cases. Use horizontal scroll if the table has many columns for a large number of classes in the dataset.
Object distribution #
Interactive heatmap chart for every class with object distribution shows how many images are in the dataset with a certain number of objects of a specific class. Users can click cell and see the list of all corresponding images.
Class sizes #
The table below gives various size properties of objects for every class. Click a row to see the image with annotations of the selected class. Sort columns to find classes with the smallest or largest objects or understand the size differences between classes.
Class | Object count | Avg area | Max area | Min area | Min height | Min height | Max height | Max height | Avg height | Avg height | Min width | Min width | Max width | Max width |
---|---|---|---|---|---|---|---|---|---|---|---|---|---|---|
face mask | 104477 | 1.46% | 53.62% | 0% | 1px | 0.27% | 500px | 100% | 53px | 14.78% | 1px | 0.2% | 429px | 81.6% |
hair mask | 99636 | 1.59% | 45.81% | 0% | 1px | 0.2% | 843px | 100% | 65px | 17.97% | 1px | 0.16% | 569px | 100% |
torso_skin mask | 92365 | 0.54% | 50.81% | 0% | 1px | 0.17% | 482px | 100% | 39px | 10.62% | 1px | 0.16% | 716px | 100% |
upperclothes mask | 85544 | 3.83% | 47.92% | 0% | 1px | 0.12% | 639px | 100% | 110px | 30.11% | 1px | 0.17% | 929px | 100% |
right_arm mask | 73282 | 0.78% | 22.47% | 0% | 1px | 0.2% | 610px | 100% | 51px | 13.76% | 1px | 0.19% | 550px | 100% |
left_arm mask | 70363 | 0.74% | 30.37% | 0% | 1px | 0.2% | 601px | 100% | 50px | 13.52% | 1px | 0.2% | 536px | 100% |
pants mask | 60430 | 2.2% | 27.66% | 0% | 1px | 0.3% | 610px | 100% | 83px | 22.02% | 1px | 0.2% | 500px | 100% |
coat mask | 38731 | 6.56% | 53.4% | 0% | 4px | 1% | 668px | 100% | 143px | 39.75% | 2px | 0.4% | 626px | 100% |
right_shoe mask | 29437 | 0.24% | 13.75% | 0% | 1px | 0.3% | 466px | 88.11% | 24px | 6.12% | 1px | 0.2% | 290px | 59.16% |
left_shoe mask | 29430 | 0.24% | 7.53% | 0% | 1px | 0.2% | 453px | 90.6% | 24px | 6.11% | 1px | 0.2% | 234px | 68.17% |
Spatial Heatmap #
The heatmaps below give the spatial distributions of all objects for every class. These visualizations provide insights into the most probable and rare object locations on the image. It helps analyze objects' placements in a dataset.
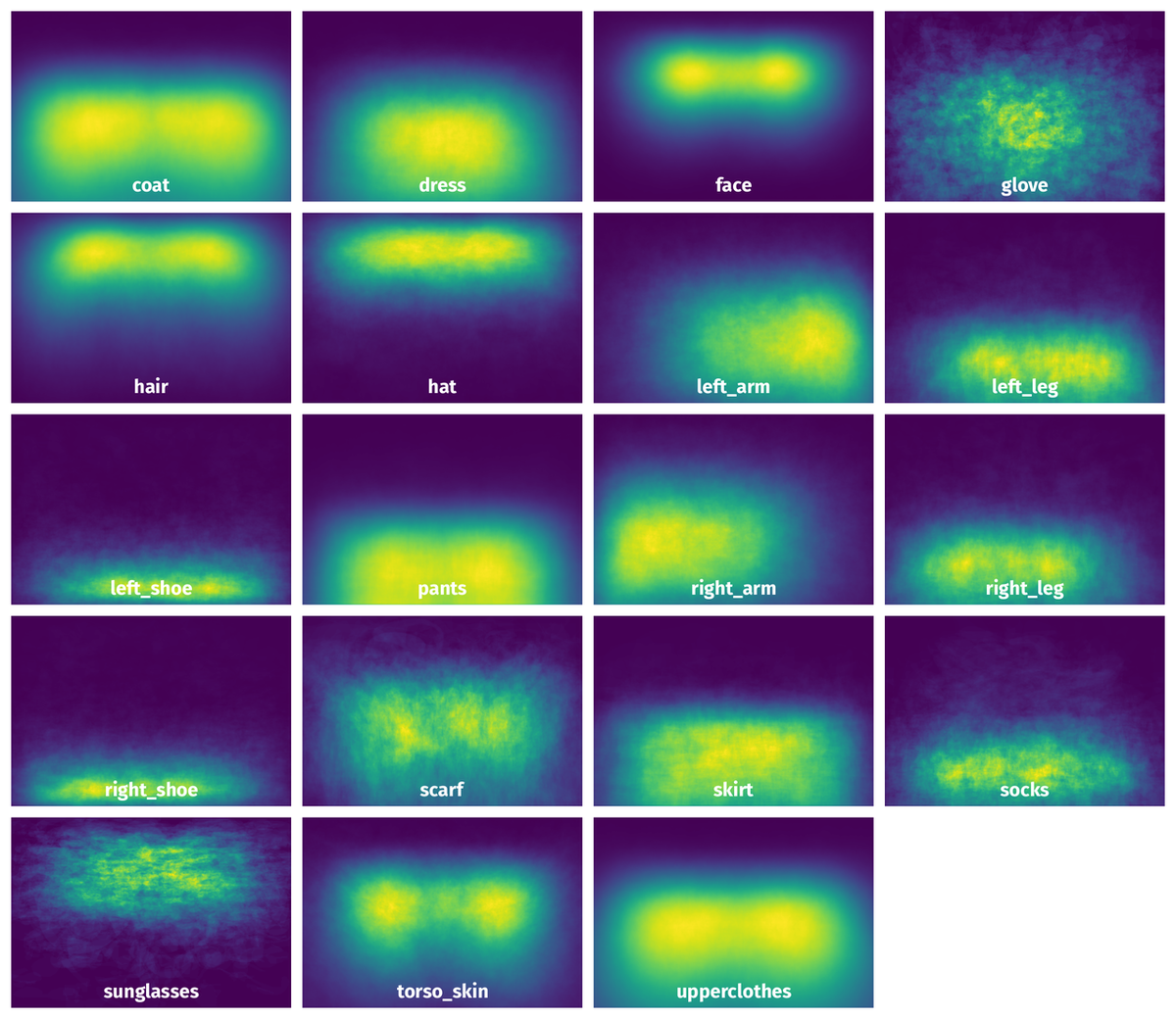
Objects #
Table contains all 100057 objects. Click a row to preview an image with annotations, and use search or pagination to navigate. Sort columns to find outliers in the dataset.
Object ID ã…¤ | Class ã…¤ | Image name click row to open | Image size height x width | Height ã…¤ | Height ã…¤ | Width ã…¤ | Width ã…¤ | Area ã…¤ |
---|---|---|---|---|---|---|---|---|
1âž” | hair mask | 0011630.jpg | 500 x 375 | 86px | 17.2% | 115px | 30.67% | 1.31% |
2âž” | hair mask | 0011630.jpg | 500 x 375 | 76px | 15.2% | 64px | 17.07% | 1.06% |
3âž” | torso_skin mask | 0011630.jpg | 500 x 375 | 125px | 25% | 100px | 26.67% | 1.58% |
4âž” | left_arm mask | 0011630.jpg | 500 x 375 | 87px | 17.4% | 47px | 12.53% | 1.14% |
5âž” | torso_skin mask | 0011630.jpg | 500 x 375 | 36px | 7.2% | 45px | 12% | 0.44% |
6âž” | face mask | 0011630.jpg | 500 x 375 | 70px | 14% | 67px | 17.87% | 1.17% |
7âž” | upperclothes mask | 0011630.jpg | 500 x 375 | 296px | 59.2% | 349px | 93.07% | 28.41% |
8âž” | face mask | 0011630.jpg | 500 x 375 | 143px | 28.6% | 125px | 33.33% | 6.71% |
9âž” | upperclothes mask | 0011630.jpg | 500 x 375 | 273px | 54.6% | 62px | 16.53% | 2.77% |
10âž” | right_arm mask | 0011630.jpg | 500 x 375 | 72px | 14.4% | 93px | 24.8% | 1.08% |
License #
Citation #
If you make use of the CIHP data, please cite the following reference:
@misc{gong2018instancelevel,
title={Instance-level Human Parsing via Part Grouping Network},
author={Ke Gong and Xiaodan Liang and Yicheng Li and Yimin Chen and Ming Yang and Liang Lin},
year={2018},
eprint={1808.00157},
archivePrefix={arXiv},
primaryClass={cs.CV}
}
If you are happy with Dataset Ninja and use provided visualizations and tools in your work, please cite us:
@misc{ visualization-tools-for-cihp-dataset,
title = { Visualization Tools for CIHP Dataset },
type = { Computer Vision Tools },
author = { Dataset Ninja },
howpublished = { \url{ https://datasetninja.com/cihp } },
url = { https://datasetninja.com/cihp },
journal = { Dataset Ninja },
publisher = { Dataset Ninja },
year = { 2025 },
month = { feb },
note = { visited on 2025-02-22 },
}
Download #
Dataset CIHP can be downloaded in Supervisely format:
As an alternative, it can be downloaded with dataset-tools package:
pip install --upgrade dataset-tools
… using following python code:
import dataset_tools as dtools
dtools.download(dataset='CIHP', dst_dir='~/dataset-ninja/')
Make sure not to overlook the python code example available on the Supervisely Developer Portal. It will give you a clear idea of how to effortlessly work with the downloaded dataset.
The data in original format can be downloaded here.
Disclaimer #
Our gal from the legal dep told us we need to post this:
Dataset Ninja provides visualizations and statistics for some datasets that can be found online and can be downloaded by general audience. Dataset Ninja is not a dataset hosting platform and can only be used for informational purposes. The platform does not claim any rights for the original content, including images, videos, annotations and descriptions. Joint publishing is prohibited.
You take full responsibility when you use datasets presented at Dataset Ninja, as well as other information, including visualizations and statistics we provide. You are in charge of compliance with any dataset license and all other permissions. You are required to navigate datasets homepage and make sure that you can use it. In case of any questions, get in touch with us at hello@datasetninja.com.