Introduction #
The CCP: Clothing Co-Parsing dataset contains 2,098 high-resolution fashion photos with diverse human/clothing variations, including superpixel-level annotations with 57 tags for over 1,000 images, along with image-level tags for the rest of the dataset, all meticulously produced by a professional team.
Authors introduce a system aimed at parsing clothing images to provide accurate pixel-wise annotations of clothing items, addressing challenges such as diverse clothing styles, human pose variations, and a large number of fine-grained clothing categories. The system comprises two sequential phases: image co-segmentation for extracting distinct clothing regions and region co-labeling for recognizing various garment items.
Clothing recognition and retrieval have huge potential in internet-based e-commerce, as the revenue of online clothing sales keeps increasing every year. The authors focused on building an engineered and applicable system to jointly parse a batch of clothing images and produce accurate pixel-wise annotation of clothing items. They consider the following challenges to build such a system:
- The appearances of clothes and garment items are often diverse with different styles and textures, compared with other common objects. It is usually hard to segment and recognize clothes via only bottom-up image features.
- The variations of human poses and self-occlusions are non-trivial issues for clothing recognition, although the clothing images can be in clear resolution and nearly frontal view.
- The number of fine-grained clothes categories is very large, e.g., more than 50 categories in the Fashionista dataset; the categories are relatively fewer in existing co-segmentation systems.
To address the above issues, authors develop a system consisting of two sequential phases of inference over a set of clothing images, i.e. image co-segmentation for extracting distinguishable clothes regions, and region co-labeling for recognizing various garment items.
Illustration of the proposed clothing co-parsing framework, which consists of two sequential phases of optimization: (a) clothing co-segmentation for extracting coherent clothes regions, and (b) region co-labeling for recognizing various clothes garments. Specifically, clothing co-segmentation iterates with three steps: (a1) grouping superpixels into regions, (a2) selecting confident foreground regions to train E-SVM classifiers, and (a3) propagating segmentations by applying E-SVM templates over all images. Given the segmented regions, clothing co-labeling is achieved based on a multi-image graphical model, as illustrated in (b).
Summary #
CCP: Clothing Co-Parsing is a dataset for semantic segmentation and unsupervised learning tasks. It is used in the retail industry.
The dataset consists of 2098 images with 8273 labeled objects belonging to 59 different classes including null, skin, hair, and other: shoes, bag, pants, sunglasses, dress, purse, coat, accessories, blouse, belt, shirt, skirt, jeans, sweater, hat, t-shirt, blazer, stockings, suit, bracelet, scarf, jacket, sandals, socks, shorts, and 31 more.
Images in the CCP dataset have pixel-level semantic segmentation annotations. There are 1094 (52% of the total) unlabeled images (i.e. without annotations). There are no pre-defined train/val/test splits in the dataset. Additionally, the non-labeled images contain clothing tags. The dataset was released in 2014 by the Sun Yat-sen University, The Chinese University of Hong Kong, and SYSU-CMU Shunde International Joint Research Institute.
Here is a visualized example for randomly selected sample classes:
Explore #
CCP dataset has 2098 images. Click on one of the examples below or open "Explore" tool anytime you need to view dataset images with annotations. This tool has extended visualization capabilities like zoom, translation, objects table, custom filters and more. Hover the mouse over the images to hide or show annotations.


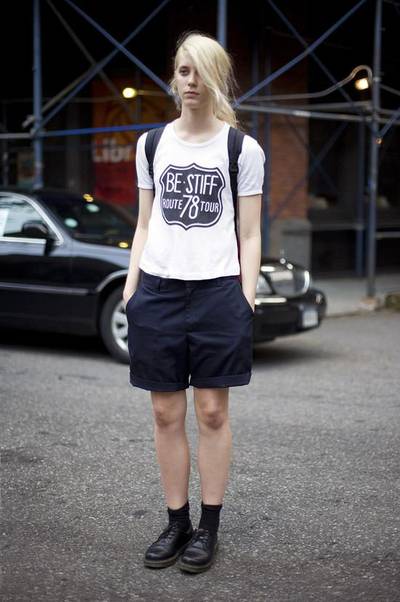
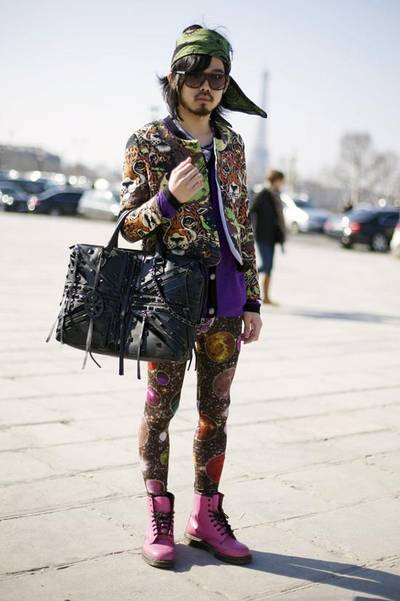
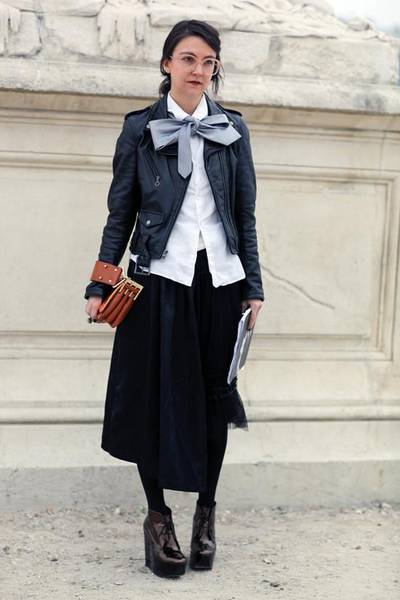


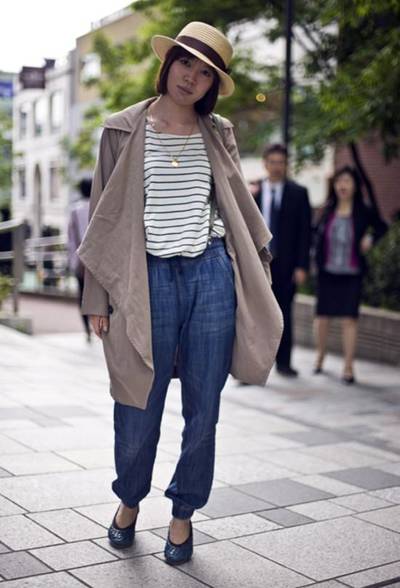


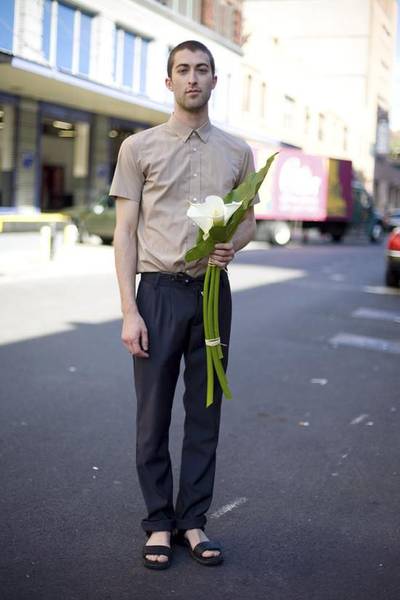

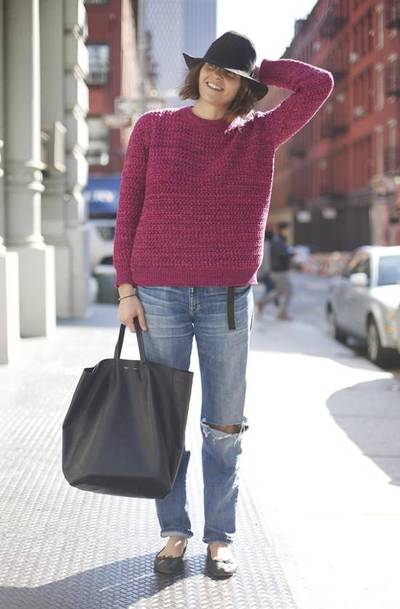

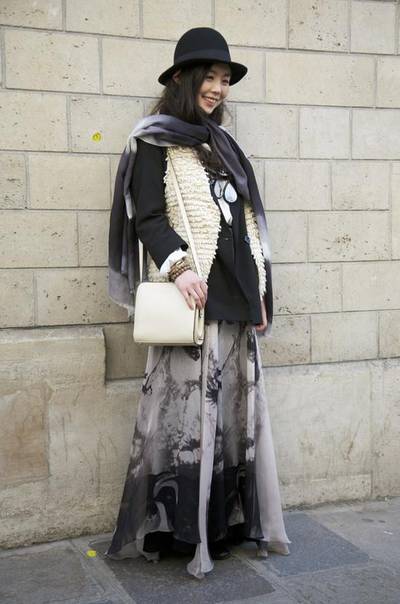
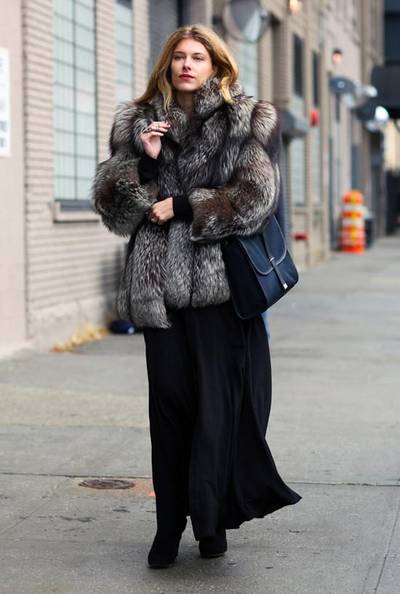


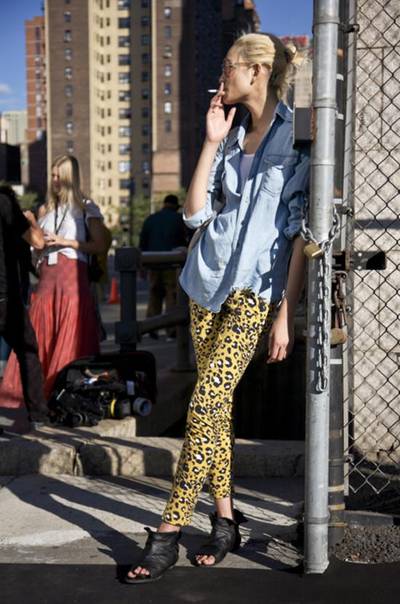
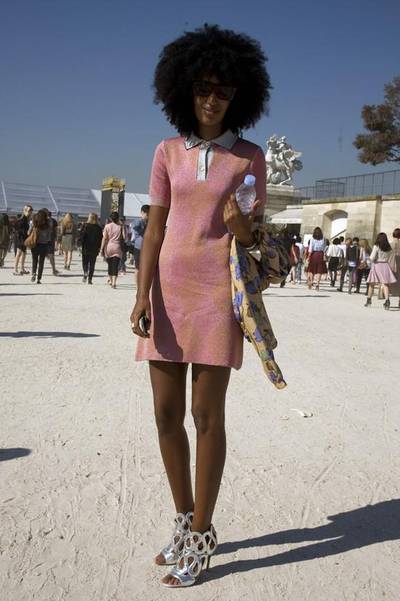

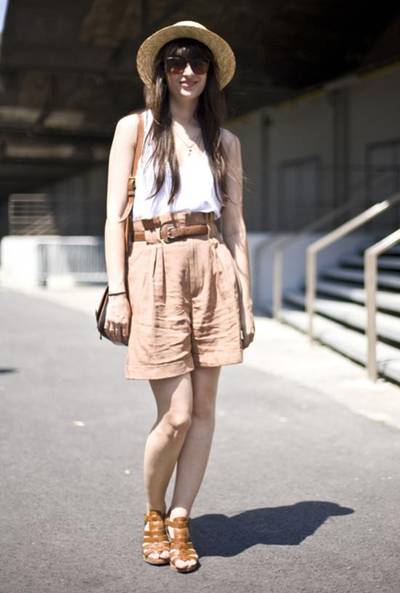
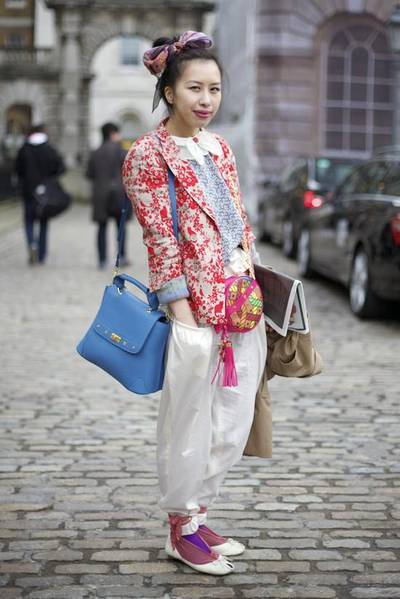
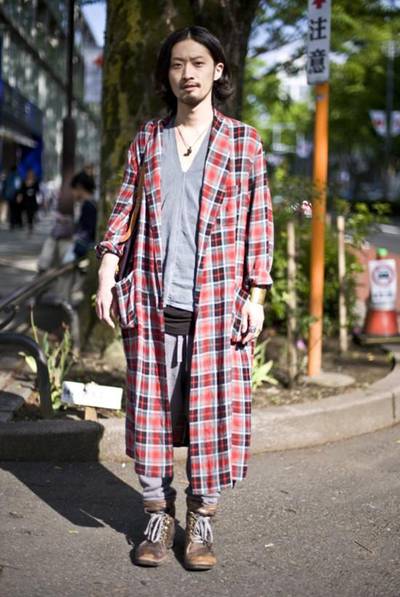
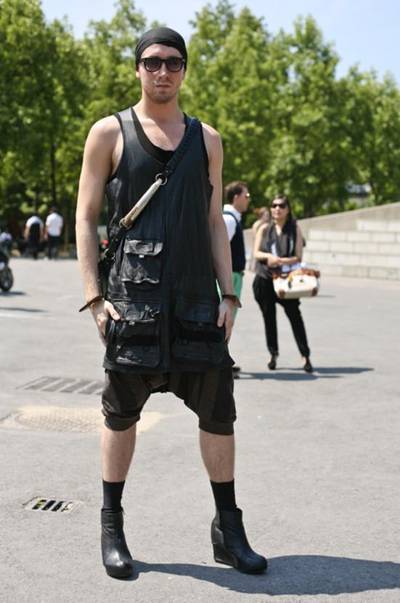

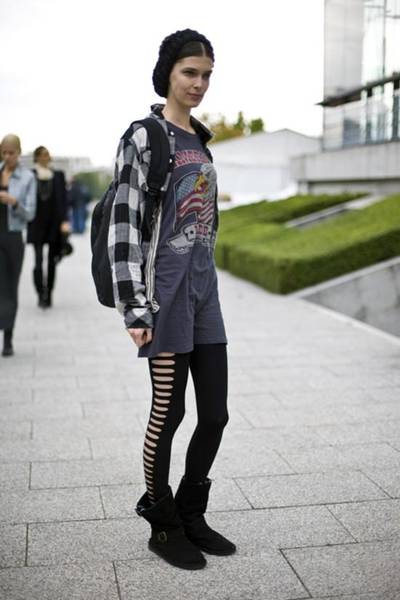

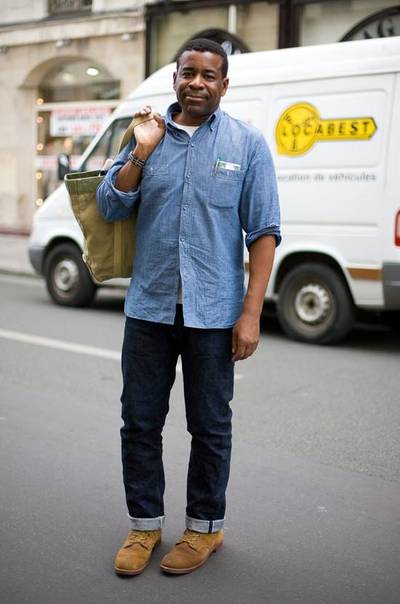

Class balance #
There are 59 annotation classes in the dataset. Find the general statistics and balances for every class in the table below. Click any row to preview images that have labels of the selected class. Sort by column to find the most rare or prevalent classes.
Class ã…¤ | Images ã…¤ | Objects ã…¤ | Count on image average | Area on image average |
---|---|---|---|---|
nullâž” mask | 1004 | 1004 | 1 | 76.9% |
skinâž” mask | 1003 | 1003 | 1 | 2.92% |
hairâž” mask | 960 | 960 | 1 | 1.31% |
shoesâž” mask | 775 | 775 | 1 | 1.08% |
bagâž” mask | 443 | 443 | 1 | 2.03% |
pantsâž” mask | 302 | 302 | 1 | 8.02% |
sunglassesâž” mask | 293 | 293 | 1 | 0.21% |
dressâž” mask | 271 | 271 | 1 | 10.06% |
purseâž” mask | 234 | 234 | 1 | 1.79% |
coatâž” mask | 232 | 232 | 1 | 10.76% |
Images #
Explore every single image in the dataset with respect to the number of annotations of each class it has. Click a row to preview selected image. Sort by any column to find anomalies and edge cases. Use horizontal scroll if the table has many columns for a large number of classes in the dataset.
Object distribution #
Interactive heatmap chart for every class with object distribution shows how many images are in the dataset with a certain number of objects of a specific class. Users can click cell and see the list of all corresponding images.
Class sizes #
The table below gives various size properties of objects for every class. Click a row to see the image with annotations of the selected class. Sort columns to find classes with the smallest or largest objects or understand the size differences between classes.
Class | Object count | Avg area | Max area | Min area | Min height | Min height | Max height | Max height | Avg height | Avg height | Min width | Min width | Max width | Max width |
---|---|---|---|---|---|---|---|---|---|---|---|---|---|---|
null mask | 1004 | 76.9% | 100% | 64.74% | 801px | 100% | 873px | 100% | 828px | 100% | 550px | 100% | 550px | 100% |
skin mask | 1003 | 2.92% | 10.01% | 0.03% | 11px | 1.36% | 798px | 92.38% | 519px | 62.66% | 19px | 3.45% | 458px | 83.27% |
hair mask | 960 | 1.31% | 4.82% | 0.03% | 13px | 1.59% | 652px | 79.13% | 123px | 14.81% | 13px | 2.36% | 220px | 40% |
shoes mask | 775 | 1.08% | 6.51% | 0.03% | 14px | 1.7% | 573px | 68.3% | 87px | 10.56% | 11px | 2% | 347px | 63.09% |
bag mask | 443 | 2.03% | 9.02% | 0.08% | 38px | 4.63% | 563px | 68.55% | 185px | 22.36% | 21px | 3.82% | 358px | 65.09% |
pants mask | 302 | 8.02% | 17.89% | 0.73% | 72px | 8.65% | 668px | 81.27% | 349px | 42.11% | 68px | 12.36% | 371px | 67.45% |
sunglasses mask | 293 | 0.21% | 0.42% | 0.03% | 10px | 1.19% | 375px | 44.7% | 30px | 3.57% | 11px | 2% | 145px | 26.36% |
dress mask | 271 | 10.06% | 25.25% | 0.01% | 5px | 0.6% | 675px | 80.55% | 379px | 45.73% | 7px | 1.27% | 372px | 67.64% |
purse mask | 234 | 1.79% | 6.45% | 0.14% | 38px | 4.57% | 517px | 63.13% | 178px | 21.51% | 17px | 3.09% | 287px | 52.18% |
coat mask | 232 | 10.76% | 23.58% | 2.43% | 150px | 18.03% | 609px | 74.45% | 373px | 45% | 159px | 28.91% | 402px | 73.09% |
Spatial Heatmap #
The heatmaps below give the spatial distributions of all objects for every class. These visualizations provide insights into the most probable and rare object locations on the image. It helps analyze objects' placements in a dataset.
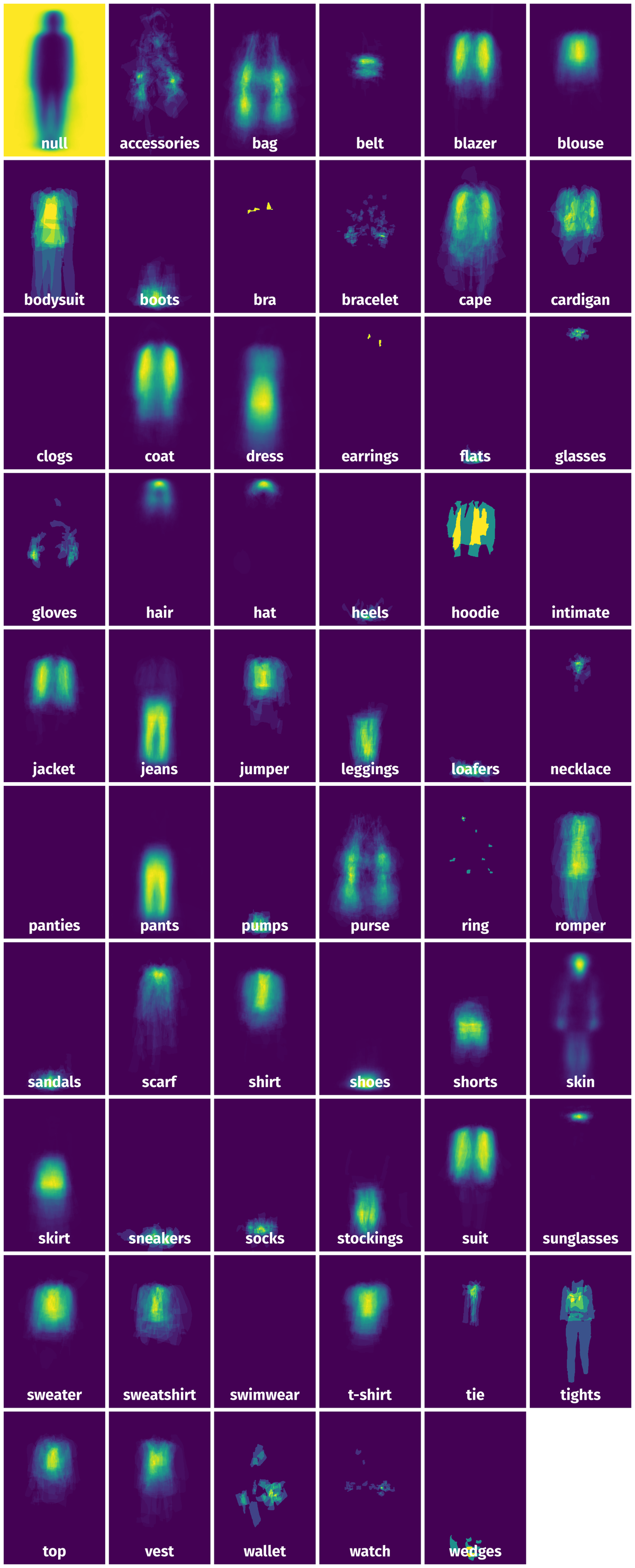
Objects #
Table contains all 8273 objects. Click a row to preview an image with annotations, and use search or pagination to navigate. Sort columns to find outliers in the dataset.
Object ID ã…¤ | Class ã…¤ | Image name click row to open | Image size height x width | Height ã…¤ | Height ã…¤ | Width ã…¤ | Width ã…¤ | Area ã…¤ |
---|---|---|---|---|---|---|---|---|
1âž” | hair mask | 0214.jpg | 812 x 550 | 140px | 17.24% | 123px | 22.36% | 1.51% |
2âž” | shoes mask | 0214.jpg | 812 x 550 | 69px | 8.5% | 79px | 14.36% | 0.81% |
3âž” | dress mask | 0214.jpg | 812 x 550 | 312px | 38.42% | 176px | 32% | 7.91% |
4âž” | skin mask | 0214.jpg | 812 x 550 | 703px | 86.58% | 177px | 32.18% | 7.96% |
5âž” | null mask | 0214.jpg | 812 x 550 | 812px | 100% | 550px | 100% | 80.59% |
6âž” | accessories mask | 0214.jpg | 812 x 550 | 30px | 3.69% | 171px | 31.09% | 0.08% |
7âž” | bag mask | 0214.jpg | 812 x 550 | 222px | 27.34% | 98px | 17.82% | 1.01% |
8âž” | sunglasses mask | 0214.jpg | 812 x 550 | 17px | 2.09% | 56px | 10.18% | 0.14% |
9âž” | hair mask | 0680.jpg | 820 x 550 | 56px | 6.83% | 83px | 15.09% | 0.43% |
10âž” | shoes mask | 0680.jpg | 820 x 550 | 89px | 10.85% | 69px | 12.55% | 0.88% |
License #
CCP: Clothing Co-Parsing Dataset is under Apache 2.0 license.
Citation #
If you make use of the Clothing Co-Parsing data, please cite the following reference:
@inproceedings{yang2014clothing,
title={Clothing Co-Parsing by Joint Image Segmentation and Labeling},
author={Yang, Wei and Luo, Ping and Lin, Liang}
booktitle={Computer Vision and Pattern Recognition (CVPR), 2014 IEEE Conference on},
year={2013},
organization={IEEE}
}
If you are happy with Dataset Ninja and use provided visualizations and tools in your work, please cite us:
@misc{ visualization-tools-for-clothing-co-parsing-dataset,
title = { Visualization Tools for CCP Dataset },
type = { Computer Vision Tools },
author = { Dataset Ninja },
howpublished = { \url{ https://datasetninja.com/clothing-co-parsing } },
url = { https://datasetninja.com/clothing-co-parsing },
journal = { Dataset Ninja },
publisher = { Dataset Ninja },
year = { 2025 },
month = { feb },
note = { visited on 2025-02-22 },
}
Download #
Dataset CCP can be downloaded in Supervisely format:
As an alternative, it can be downloaded with dataset-tools package:
pip install --upgrade dataset-tools
… using following python code:
import dataset_tools as dtools
dtools.download(dataset='CCP', dst_dir='~/dataset-ninja/')
Make sure not to overlook the python code example available on the Supervisely Developer Portal. It will give you a clear idea of how to effortlessly work with the downloaded dataset.
Disclaimer #
Our gal from the legal dep told us we need to post this:
Dataset Ninja provides visualizations and statistics for some datasets that can be found online and can be downloaded by general audience. Dataset Ninja is not a dataset hosting platform and can only be used for informational purposes. The platform does not claim any rights for the original content, including images, videos, annotations and descriptions. Joint publishing is prohibited.
You take full responsibility when you use datasets presented at Dataset Ninja, as well as other information, including visualizations and statistics we provide. You are in charge of compliance with any dataset license and all other permissions. You are required to navigate datasets homepage and make sure that you can use it. In case of any questions, get in touch with us at hello@datasetninja.com.