Introduction #
The authors of the Cows2021 Dataset feature identity annotations of the Holstein-Friesian cattle on a large scale. Additionally, they present a pioneering self-supervision framework tailored for the video-based identification of individual animals. Cows2021 encompasses an extensive collection of 10,402 RGB images, each meticulously labeled with both localization and identity information. Furthermore, the dataset incorporates 301 videos obtained from the same cattle herd. These videos provide top-down in-barn imagery, adept at capturing the distinctive black and white coat patterns characteristic of this breed. Note, that the present version of the dataset at datasetninja has only images.
Given the substantial labeling effort typically required for the development of visual cattle identification systems, the authors propose a novel approach. They leverage the temporal consistency in coat pattern appearance across videos as a self-supervision signal for learning animal identities. To facilitate this, they employ an individual-agnostic cattle detector that produces oriented bounding boxes. These boxes are then used to form rotation-normalized tracklets for individual cattle through a tracking-by-detection process, further enhanced by augmentations. This process generates a “positive” sample set for each tracklet, which is subsequently paired with a “negative” set randomly sampled from other videos featuring different cattle. The authors then employ frame-triplet contrastive learning to construct a metric latent space for identity learning.
The Cows2021 features 186 Holstein-Friesian cattle and was captured using an Intel D435 camera at the University of Bristol’s Wyndhurst Farm in Langford Village, UK. The camera was positioned 4 meters above the ground, overlooking a walkway connecting the milking parlour and holding pens. Recordings, triggered by motion, spanned a month after milking sessions.
The dataset provides images with a resolution of 1280 x 720 pixels per frame, utilizing 8 bits per RGB channel. It encompasses a total of 10,402 still images and 301 videos, each with a duration of 5.5 seconds and a frame rate of 30 frames per second. The distribution of still images across individuals and time aligns with the natural operation of the farm. Additionally, the dataset includes various expert-generated ground truth annotations.
For cattle torso annotations, adhering to VOC 2012 guidelines for object annotation, all visible cattle torso instances were manually labeled, excluding the head, neck, legs, and tail. Torso instances with significant clipping were tagged as “clipped” and excluded from further analysis.
Regarding animal identity annotations, a total of 13,784 detected cattle instances were manually assigned to one of 182 individuals, with four all-black cows excluded from the identity study for future research. The number of occurrences for each individual cow_id varies, with a mean (µ) of 77.5 and a standard deviation (σ) of 39.9.
The dataset also includes video data with tracklet annotations. These tracklets, vital for self-supervision in identity learning, were generated by utilizing a highly reliable ID-agnostic cattle detector and employing tracking-by-detection techniques. The average number of tracklets per video is 1.45.
Summary #
Cows2021 Dataset is a dataset for object detection, identification, and self supervised learning tasks. It is used in the livestock industry.
The dataset consists of 42422 images with 13178 labeled objects belonging to 1 single class (cattle torso).
Images in the Cows2021 dataset have bounding box annotations. There are 32020 (75% of the total) unlabeled images (i.e. without annotations). There are 5 splits in the dataset: identification-train (23350 images), identification-test (8670 images), detection_and_localisation-train (7248 images), detection_and_localisation-test (2131 images), and detection_and_localisation-val (1023 images). Additionally, images in identification-test and identification-train splits contain information about cow_id. The dataset was released in 2021 by the University of Bristol.
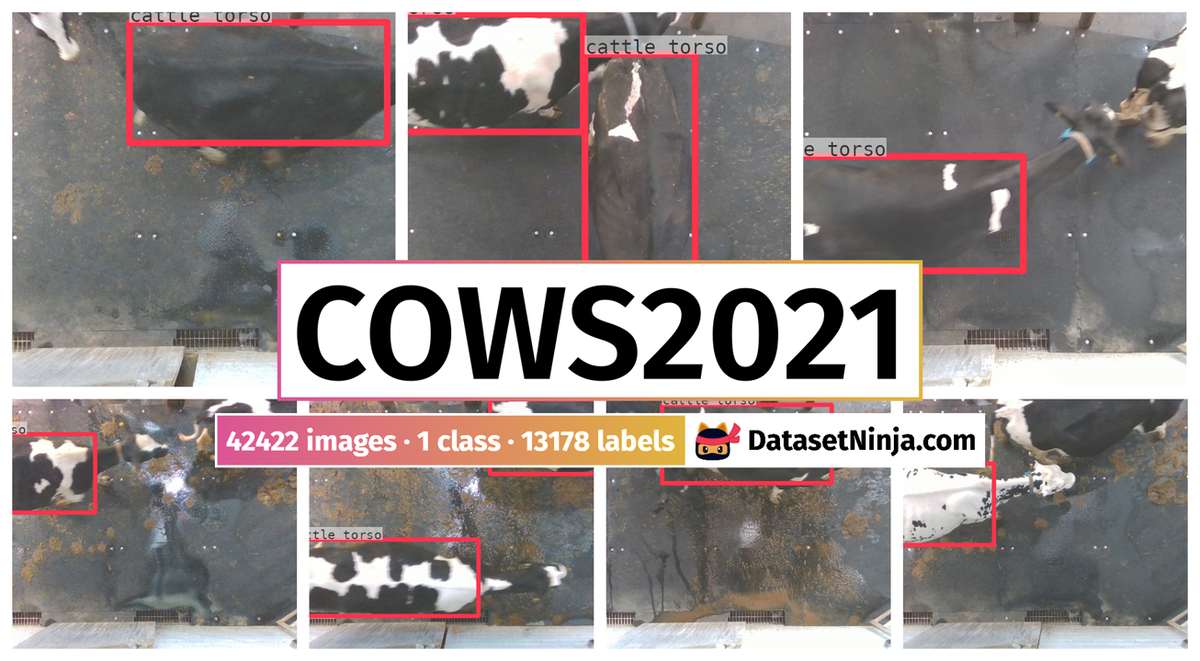
Explore #
Cows2021 dataset has 42422 images. Click on one of the examples below or open "Explore" tool anytime you need to view dataset images with annotations. This tool has extended visualization capabilities like zoom, translation, objects table, custom filters and more. Hover the mouse over the images to hide or show annotations.
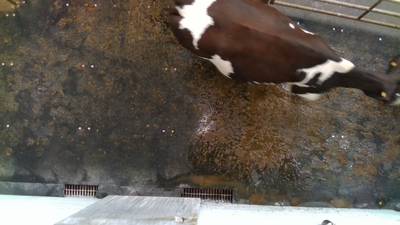
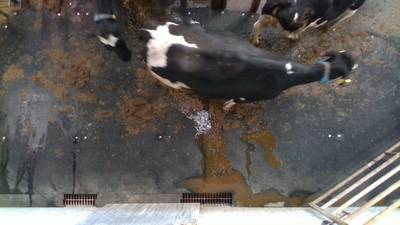
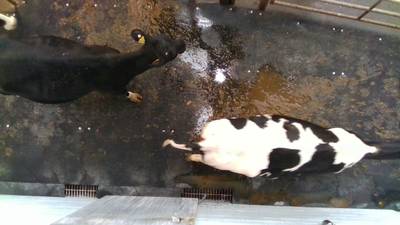

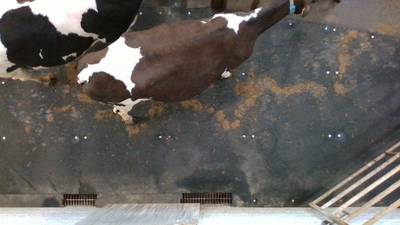


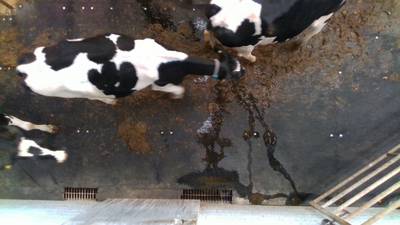

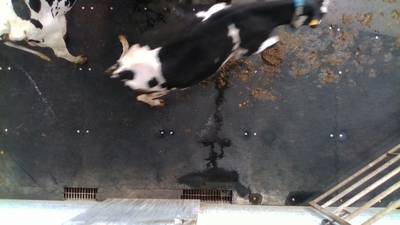


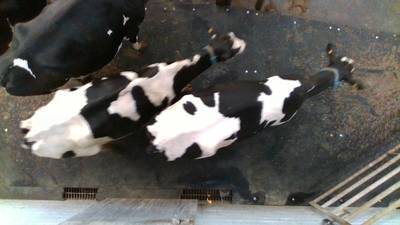
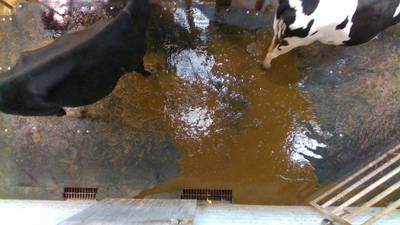
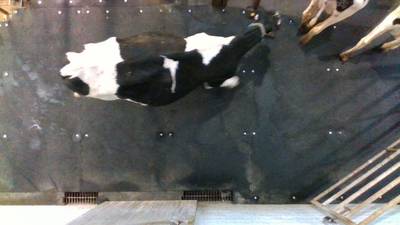
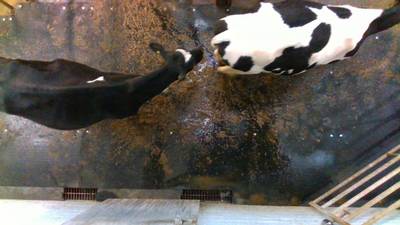

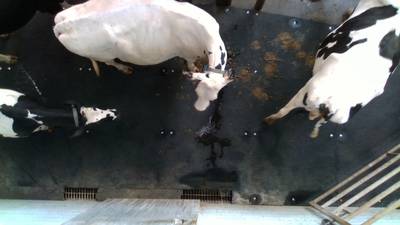


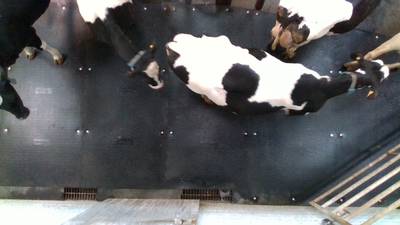
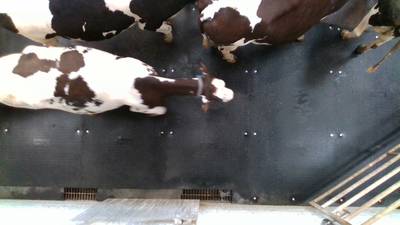
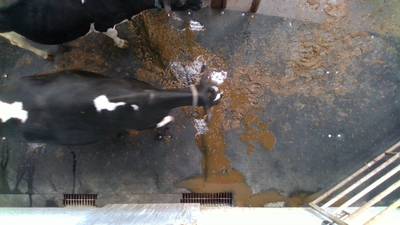
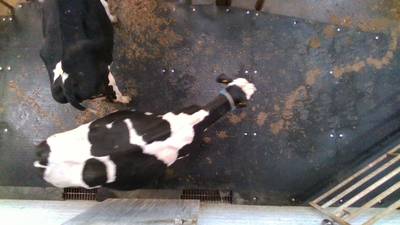
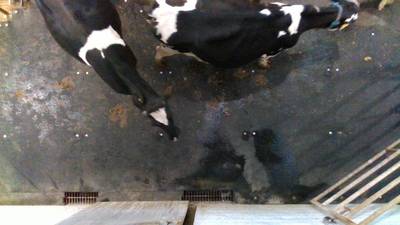
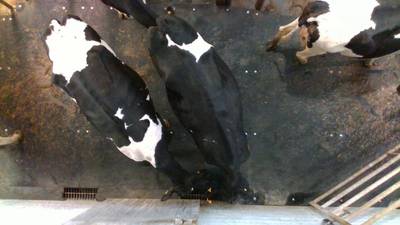

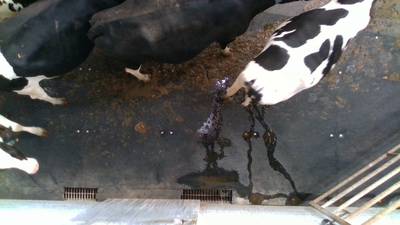
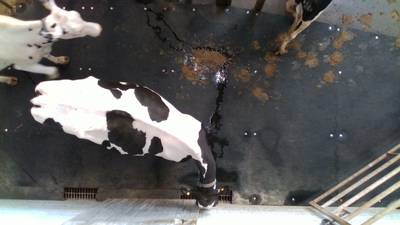

Class balance #
There are 1 annotation classes in the dataset. Find the general statistics and balances for every class in the table below. Click any row to preview images that have labels of the selected class. Sort by column to find the most rare or prevalent classes.
Class ㅤ | Images ㅤ | Objects ㅤ | Count on image average | Area on image average |
---|---|---|---|---|
cattle torso➔ rectangle | 10402 | 13178 | 1.27 | 15.66% |
Images #
Explore every single image in the dataset with respect to the number of annotations of each class it has. Click a row to preview selected image. Sort by any column to find anomalies and edge cases. Use horizontal scroll if the table has many columns for a large number of classes in the dataset.
Object distribution #
Interactive heatmap chart for every class with object distribution shows how many images are in the dataset with a certain number of objects of a specific class. Users can click cell and see the list of all corresponding images.
Class sizes #
The table below gives various size properties of objects for every class. Click a row to see the image with annotations of the selected class. Sort columns to find classes with the smallest or largest objects or understand the size differences between classes.
Class | Object count | Avg area | Max area | Min area | Min height | Min height | Max height | Max height | Avg height | Avg height | Min width | Min width | Max width | Max width |
---|---|---|---|---|---|---|---|---|---|---|---|---|---|---|
cattle torso rectangle | 13178 | 12.47% | 18.85% | 0.28% | 27px | 3.75% | 599px | 83.19% | 245px | 33.98% | 53px | 4.14% | 641px | 50.08% |
Spatial Heatmap #
The heatmaps below give the spatial distributions of all objects for every class. These visualizations provide insights into the most probable and rare object locations on the image. It helps analyze objects' placements in a dataset.
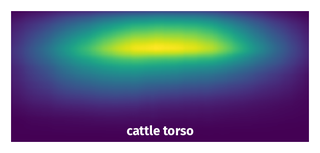
Objects #
Table contains all 13178 objects. Click a row to preview an image with annotations, and use search or pagination to navigate. Sort columns to find outliers in the dataset.
Object ID ㅤ | Class ㅤ | Image name click row to open | Image size height x width | Height ㅤ | Height ㅤ | Width ㅤ | Width ㅤ | Area ㅤ |
---|---|---|---|---|---|---|---|---|
1➔ | cattle torso rectangle | 08507.jpg | 720 x 1280 | 227px | 31.53% | 465px | 36.33% | 11.45% |
2➔ | cattle torso rectangle | 08507.jpg | 720 x 1280 | 201px | 27.92% | 491px | 38.36% | 10.71% |
3➔ | cattle torso rectangle | 08333.jpg | 720 x 1280 | 237px | 32.92% | 511px | 39.92% | 13.14% |
4➔ | cattle torso rectangle | 10205.jpg | 720 x 1280 | 239px | 33.19% | 527px | 41.17% | 13.67% |
5➔ | cattle torso rectangle | 09201.jpg | 720 x 1280 | 253px | 35.14% | 599px | 46.8% | 16.44% |
6➔ | cattle torso rectangle | 10311.jpg | 720 x 1280 | 255px | 35.42% | 521px | 40.7% | 14.42% |
7➔ | cattle torso rectangle | 08979.jpg | 720 x 1280 | 219px | 30.42% | 537px | 41.95% | 12.76% |
8➔ | cattle torso rectangle | 09435.jpg | 720 x 1280 | 231px | 32.08% | 519px | 40.55% | 13.01% |
9➔ | cattle torso rectangle | 09376.jpg | 720 x 1280 | 219px | 30.42% | 555px | 43.36% | 13.19% |
10➔ | cattle torso rectangle | 09091.jpg | 720 x 1280 | 215px | 29.86% | 491px | 38.36% | 11.45% |
License #
Citation #
If you make use of the Cows2021 data, please cite the following reference:
Neill Campbell, Tilo Burghardt, Jing Gao, William Andrew, Andrew Dowsey (2021):
Cows2021 Dataset.
https://doi.org/10.5523/bris.4vnrca7qw1642qlwxjadp87h7
If you are happy with Dataset Ninja and use provided visualizations and tools in your work, please cite us:
@misc{ visualization-tools-for-cows2021-dataset,
title = { Visualization Tools for Cows2021 Dataset },
type = { Computer Vision Tools },
author = { Dataset Ninja },
howpublished = { \url{ https://datasetninja.com/cows2021 } },
url = { https://datasetninja.com/cows2021 },
journal = { Dataset Ninja },
publisher = { Dataset Ninja },
year = { 2025 },
month = { jan },
note = { visited on 2025-01-22 },
}
Download #
Dataset Cows2021 can be downloaded in Supervisely format:
As an alternative, it can be downloaded with dataset-tools package:
pip install --upgrade dataset-tools
… using following python code:
import dataset_tools as dtools
dtools.download(dataset='Cows2021', dst_dir='~/dataset-ninja/')
Make sure not to overlook the python code example available on the Supervisely Developer Portal. It will give you a clear idea of how to effortlessly work with the downloaded dataset.
The data in original format can be downloaded here.
Disclaimer #
Our gal from the legal dep told us we need to post this:
Dataset Ninja provides visualizations and statistics for some datasets that can be found online and can be downloaded by general audience. Dataset Ninja is not a dataset hosting platform and can only be used for informational purposes. The platform does not claim any rights for the original content, including images, videos, annotations and descriptions. Joint publishing is prohibited.
You take full responsibility when you use datasets presented at Dataset Ninja, as well as other information, including visualizations and statistics we provide. You are in charge of compliance with any dataset license and all other permissions. You are required to navigate datasets homepage and make sure that you can use it. In case of any questions, get in touch with us at hello@datasetninja.com.