Introduction #
The authors created the DAC-SDC: Design Automation Conference System Design Contest 2022 Dataset to design and implement new algorithms based on object detection in images acquired from unmanned aerial vehicles (UAVs). The dataset includes 95 categories and 150 thousand images provided by a UAV company DJI.
Motivation
The 55th Design Automation Conference (DAC) introduced its inaugural System Design Contest (SDC) in 2018, featuring the Lower Power Object Detection Challenge (LPODC). This challenge tasked participants with devising and implementing innovative algorithms for object detection in images captured from unmanned aerial vehicles (UAVs). By providing a unified platform, the LPODC facilitated the development and comparison of state-of-the-art object detection algorithms while also fostering discussions on the insights gained from the entries.
The focus of the LPODC at DAC-SDC’18 was on applications involving unmanned aerial vehicles (UAVs), which typically entail stringent requirements for accuracy, real-time processing, and energy efficiency. Specifically, the LPODC entailed detecting a single object of interest, a critical task in UAV applications. Unlike conventional computer vision challenges like ImageNet and PASCAL VOC, which prioritize accuracy alone, the LPODC assessed overall performance based on a combination of throughput, power consumption, and detection accuracy. This comprehensive evaluation framework took into account the unique features of UAV applications, including real-time processing, energy constraints, and detection accuracy. Moreover, the dataset used in the LPODC comprised images captured from actual UAVs, thereby reflecting the real-world conditions and challenges encountered in UAV applications. Additionally, the LPODC offered participating teams the choice of two hardware platforms for their implementations: an embedded GPU (Jetson TX2 from Nvidia) and an FPGA SoC (PYNQ Z-1 board from Xilinx). These platforms, widely adopted for energy-efficient processing in UAVs, provided teams with flexibility in designing their solutions.
The publicly released dataset comprises a substantial number of manually annotated training images, while the testing dataset is reserved exclusively for evaluation purposes. In total, there are 150,000 images available provided by a UAV company DJI. Participating teams utilized the training dataset to train their models and algorithms. Subsequently, they submitted these trained models and algorithms to the organizers for evaluation. The evaluation process involved assessing various metrics such as throughput, energy efficiency, and detection accuracy. Evaluations were conducted at the conclusion of each month, with detailed rankings released thereafter. The final rankings were announced at the conclusion of the competition. The top three entries from both GPU and FPGA categories were honored, with their creators invited to present their work at a dedicated technical session during DAC.
The objective of the LPODC task is to conduct single-object detection in each image, with an axis-aligned bounding box delineating the object’s position and scale. Given the focus on UAV applications, certain key considerations come into play. Firstly, the object detection task entails pinpointing a specific object from the training dataset, rather than merely identifying objects belonging to a broader category. For instance, if images featuring person A are included in the training dataset, the goal is to detect person A specifically, rather than other individuals. Secondly, the object detection task necessitates achieving both high throughput and high accuracy, aligning with the stringent requirements of UAV applications. This combination of precision is crucial for effectively serving the needs of UAV operations.
Dataset description
The dataset sourced from DJI encompasses 12 categories of images and 95 sub-categories. Notably, in contrast to prevalent general-purpose datasets such as ImageNet and PASCAL VOC, the objects in this dataset are captured from a UAV perspective, showcasing various viewpoints.
Within the dataset, the majority of images feature objects sized between 1-2% of the captured image dimensions (640x360), which is characteristic of UAV-view imagery. This proportion maintains a favorable balance in terms of image brightness and information content. Most images exhibit moderate levels of brightness and information, akin to a Gaussian distribution, with fewer instances of excessively high or low brightness or information content.
Note: the authors did not provide a division of the dataset into training and testing.
Summary #
DAC-SDC: Design Automation Conference System Design Contest 2022 Dataset is a dataset for object detection and identification tasks. It is used in the search and rescue (SAR) industry.
The dataset consists of 93520 images with 93520 labeled objects belonging to 12 different classes including person, car, riding, and other: boat, group, wakeboard, drone, truck, paraglider, whale, building, and horseride.
Images in the DAC-SDC 2022 dataset have bounding box annotations. All images are labeled (i.e. with annotations). There is 1 split in the dataset: train (93520 images). Additionally, every image marked with its sequence tag. The dataset was released in 2022 by the University of Notre Dame, USA, The Chinese University, China, and University of Pittsburgh, USA.
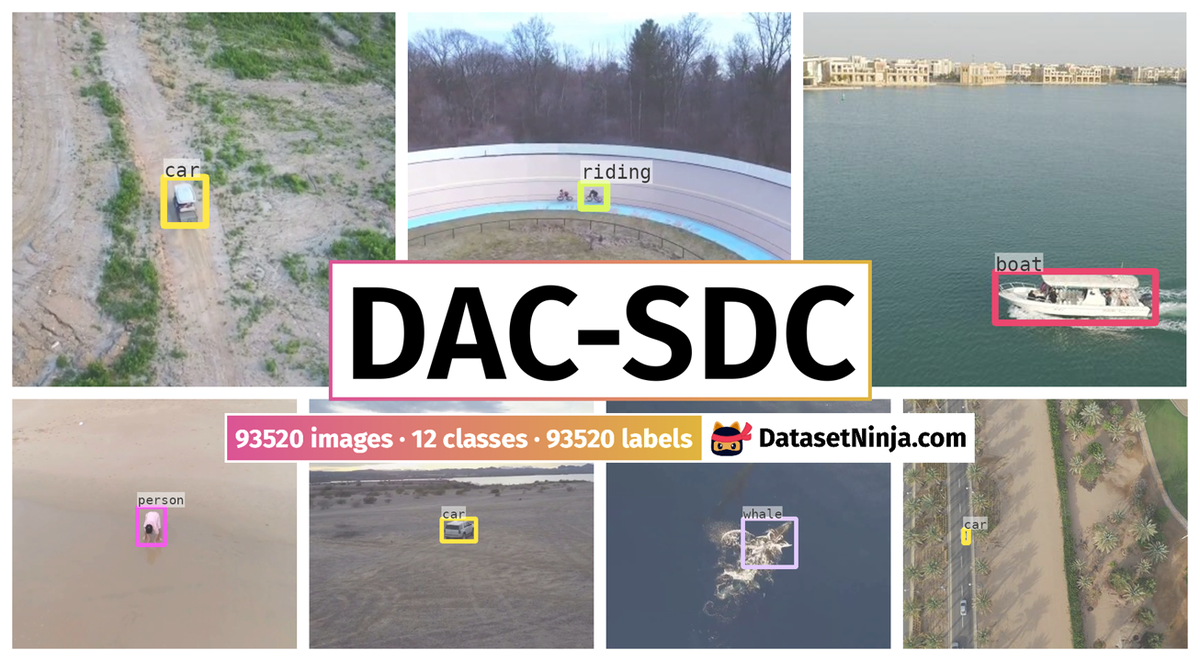
Explore #
DAC-SDC 2022 dataset has 93520 images. Click on one of the examples below or open "Explore" tool anytime you need to view dataset images with annotations. This tool has extended visualization capabilities like zoom, translation, objects table, custom filters and more. Hover the mouse over the images to hide or show annotations.
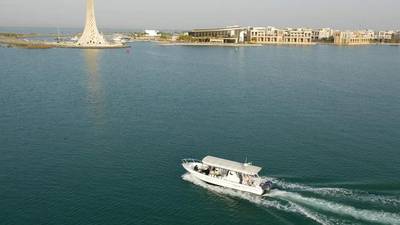
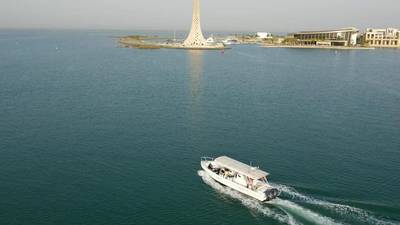
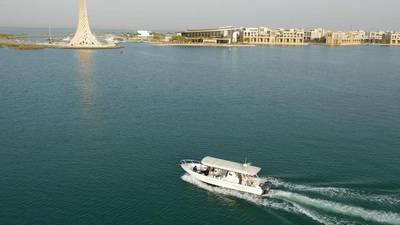




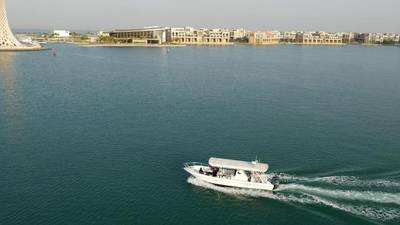
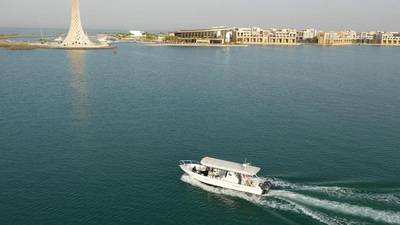

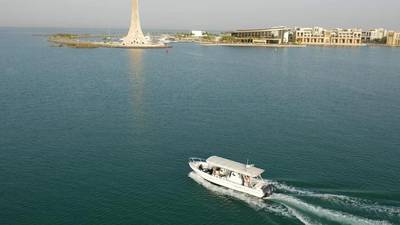
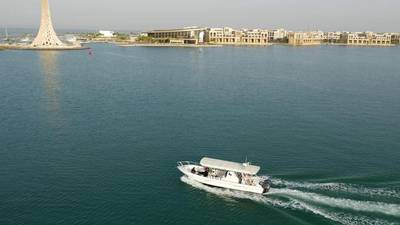

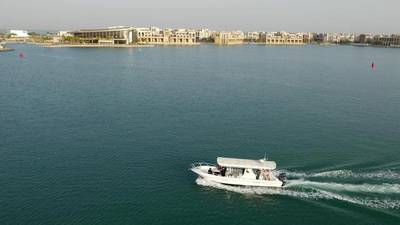
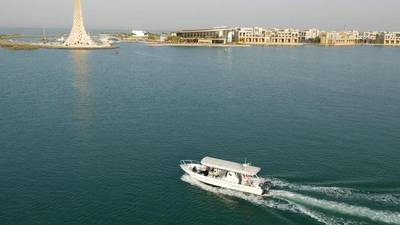

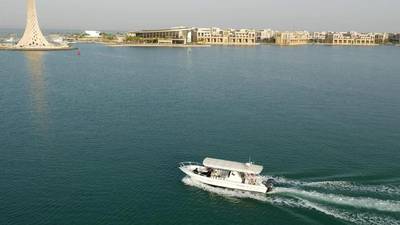
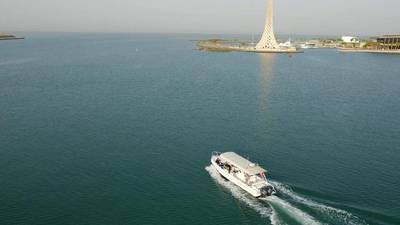

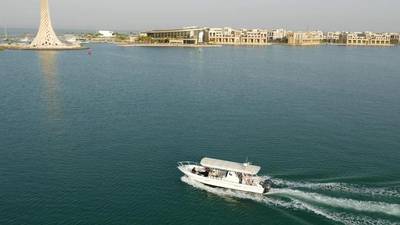
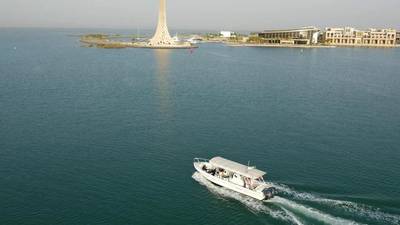
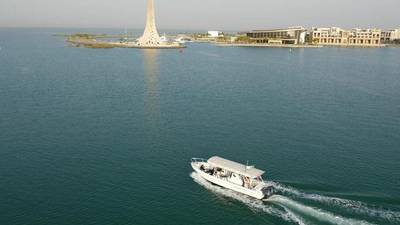
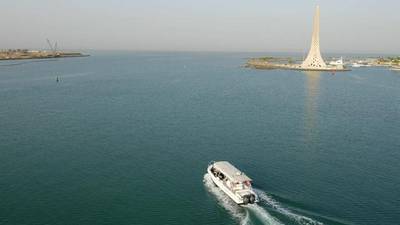



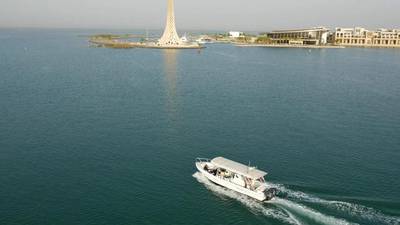

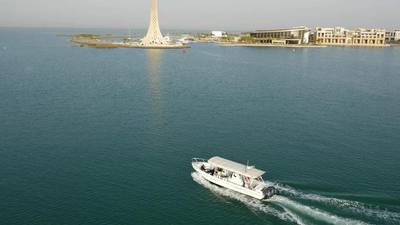
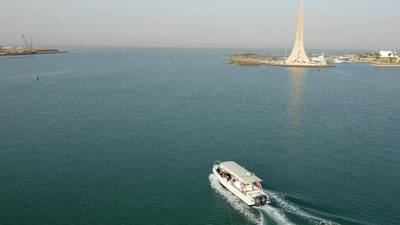
Class balance #
There are 12 annotation classes in the dataset. Find the general statistics and balances for every class in the table below. Click any row to preview images that have labels of the selected class. Sort by column to find the most rare or prevalent classes.
Class ã…¤ | Images ã…¤ | Objects ã…¤ | Count on image average | Area on image average |
---|---|---|---|---|
personâž” rectangle | 27965 | 27965 | 1 | 1.09% |
carâž” rectangle | 25014 | 25014 | 1 | 0.9% |
ridingâž” rectangle | 16999 | 16999 | 1 | 1.37% |
boatâž” rectangle | 5207 | 5207 | 1 | 3.43% |
groupâž” rectangle | 4815 | 4815 | 1 | 0.15% |
wakeboardâž” rectangle | 3300 | 3300 | 1 | 0.22% |
droneâž” rectangle | 2576 | 2576 | 1 | 0.52% |
truckâž” rectangle | 2378 | 2378 | 1 | 1.13% |
paragliderâž” rectangle | 1694 | 1694 | 1 | 2.77% |
whaleâž” rectangle | 1533 | 1533 | 1 | 3.89% |
Co-occurrence matrix #
Co-occurrence matrix is an extremely valuable tool that shows you the images for every pair of classes: how many images have objects of both classes at the same time. If you click any cell, you will see those images. We added the tooltip with an explanation for every cell for your convenience, just hover the mouse over a cell to preview the description.
Images #
Explore every single image in the dataset with respect to the number of annotations of each class it has. Click a row to preview selected image. Sort by any column to find anomalies and edge cases. Use horizontal scroll if the table has many columns for a large number of classes in the dataset.
Object distribution #
Interactive heatmap chart for every class with object distribution shows how many images are in the dataset with a certain number of objects of a specific class. Users can click cell and see the list of all corresponding images.
Class sizes #
The table below gives various size properties of objects for every class. Click a row to see the image with annotations of the selected class. Sort columns to find classes with the smallest or largest objects or understand the size differences between classes.
Class | Object count | Avg area | Max area | Min area | Min height | Min height | Max height | Max height | Avg height | Avg height | Min width | Min width | Max width | Max width |
---|---|---|---|---|---|---|---|---|---|---|---|---|---|---|
person rectangle | 27965 | 1.09% | 7.88% | 0.07% | 10px | 2.78% | 227px | 63.06% | 73px | 20.28% | 10px | 1.56% | 84px | 13.12% |
car rectangle | 25014 | 0.9% | 7.48% | 0.03% | 5px | 1.39% | 133px | 36.94% | 43px | 11.82% | 7px | 1.09% | 146px | 22.81% |
riding rectangle | 16999 | 1.37% | 12.65% | 0.12% | 16px | 4.44% | 192px | 53.33% | 66px | 18.25% | 12px | 1.88% | 155px | 24.22% |
boat rectangle | 5207 | 3.43% | 31.85% | 0.05% | 8px | 2.22% | 239px | 66.39% | 66px | 18.47% | 14px | 2.19% | 307px | 47.97% |
group rectangle | 4815 | 0.15% | 0.37% | 0.02% | 11px | 3.06% | 45px | 12.5% | 33px | 9.2% | 2px | 0.31% | 23px | 3.59% |
wakeboard rectangle | 3300 | 0.22% | 1.23% | 0.01% | 6px | 1.67% | 66px | 18.33% | 25px | 6.83% | 4px | 0.62% | 51px | 7.97% |
drone rectangle | 2576 | 0.52% | 2.23% | 0.19% | 17px | 4.72% | 62px | 17.22% | 30px | 8.31% | 20px | 3.12% | 83px | 12.97% |
truck rectangle | 2378 | 1.13% | 2.76% | 0.01% | 4px | 1.11% | 91px | 25.28% | 48px | 13.28% | 5px | 0.78% | 94px | 14.69% |
paraglider rectangle | 1694 | 2.77% | 10.2% | 0.55% | 51px | 14.17% | 169px | 46.94% | 91px | 25.33% | 25px | 3.91% | 149px | 23.28% |
whale rectangle | 1533 | 3.89% | 12.95% | 0.65% | 52px | 14.44% | 162px | 45% | 95px | 26.45% | 29px | 4.53% | 190px | 29.69% |
Spatial Heatmap #
The heatmaps below give the spatial distributions of all objects for every class. These visualizations provide insights into the most probable and rare object locations on the image. It helps analyze objects' placements in a dataset.

Objects #
Table contains all 93520 objects. Click a row to preview an image with annotations, and use search or pagination to navigate. Sort columns to find outliers in the dataset.
Object ID ã…¤ | Class ã…¤ | Image name click row to open | Image size height x width | Height ã…¤ | Height ã…¤ | Width ã…¤ | Width ã…¤ | Area ã…¤ |
---|---|---|---|---|---|---|---|---|
1âž” | riding rectangle | riding13_1271.jpg | 360 x 640 | 77px | 21.39% | 33px | 5.16% | 1.1% |
2âž” | person rectangle | person20_1119.jpg | 360 x 640 | 75px | 20.83% | 31px | 4.84% | 1.01% |
3âž” | riding rectangle | riding4_0321.jpg | 360 x 640 | 29px | 8.06% | 24px | 3.75% | 0.3% |
4âž” | car rectangle | car22_720 (6)_0239.jpg | 360 x 640 | 47px | 13.06% | 45px | 7.03% | 0.92% |
5âž” | car rectangle | car10_1869.jpg | 360 x 640 | 70px | 19.44% | 56px | 8.75% | 1.7% |
6âž” | person rectangle | person18_000709.jpg | 360 x 640 | 171px | 47.5% | 53px | 8.28% | 3.93% |
7âž” | car rectangle | car17_720 (1)_0035.jpg | 360 x 640 | 57px | 15.83% | 56px | 8.75% | 1.39% |
8âž” | car rectangle | car22_720 (6)_0494.jpg | 360 x 640 | 41px | 11.39% | 39px | 6.09% | 0.69% |
9âž” | person rectangle | person17_001155.jpg | 360 x 640 | 38px | 10.56% | 12px | 1.88% | 0.2% |
10âž” | riding rectangle | riding14_7283.jpg | 360 x 640 | 104px | 28.89% | 54px | 8.44% | 2.44% |
License #
Citation #
If you make use of the DAC-SDC data, please cite the following reference:
@dataset{DAC-SDC,
author={Xiaowei Xu and Xinyi Zhang and Bei Yu and Xiaobo Sharon Hu and Christopher Rowen and Jingtong Hu and Yiyu Shi},
title={DAC-SDC: Design Automation Conference System Design Contest 2022 Dataset},
year={2022},
url={https://byuccl.github.io/dac_sdc_2022/}
}
If you are happy with Dataset Ninja and use provided visualizations and tools in your work, please cite us:
@misc{ visualization-tools-for-dac-sdc-dataset,
title = { Visualization Tools for DAC-SDC 2022 Dataset },
type = { Computer Vision Tools },
author = { Dataset Ninja },
howpublished = { \url{ https://datasetninja.com/dac-sdc-2022 } },
url = { https://datasetninja.com/dac-sdc-2022 },
journal = { Dataset Ninja },
publisher = { Dataset Ninja },
year = { 2025 },
month = { feb },
note = { visited on 2025-02-22 },
}
Download #
Dataset DAC-SDC 2022 can be downloaded in Supervisely format:
As an alternative, it can be downloaded with dataset-tools package:
pip install --upgrade dataset-tools
… using following python code:
import dataset_tools as dtools
dtools.download(dataset='DAC-SDC 2022', dst_dir='~/dataset-ninja/')
Make sure not to overlook the python code example available on the Supervisely Developer Portal. It will give you a clear idea of how to effortlessly work with the downloaded dataset.
The data in original format can be downloaded here.
Disclaimer #
Our gal from the legal dep told us we need to post this:
Dataset Ninja provides visualizations and statistics for some datasets that can be found online and can be downloaded by general audience. Dataset Ninja is not a dataset hosting platform and can only be used for informational purposes. The platform does not claim any rights for the original content, including images, videos, annotations and descriptions. Joint publishing is prohibited.
You take full responsibility when you use datasets presented at Dataset Ninja, as well as other information, including visualizations and statistics we provide. You are in charge of compliance with any dataset license and all other permissions. You are required to navigate datasets homepage and make sure that you can use it. In case of any questions, get in touch with us at hello@datasetninja.com.