Introduction #
The dataset for Digital Breakthrough 2022: Ulyanovsk - Car Plate Detection is a collection of 482 meticulously labeled images with a specific emphasis on detecting a car’s plate. Embedded within the Ulyanovsk region (Russia) AI competition, this dataset presents an intriguing challenge for participants: devising an algorithm for real-time assessment of distances between cars depicted in various photos. The primary goal is to integrate this algorithm into navigation systems, serving as a precautionary tool to alert individuals about unsafe distances, thereby advocating and bolstering road safety standards.
About Digital Breakthrough: Season AI (Ulyanovsk Region)
According to the press service of the Moscow Traffic Police, by the end of 2021, non-compliance with the distance between cars has become the most dangerous violation. It is because of non-compliance with the safe distance to the car in front that people most often die and get into accidents on the roads.
Maintaining a safe distance from the vehicle in front is one of the important criteria for road safety. In real-time, such a parameter cannot be monitored with the help of surveillance cameras, and it is also impossible to estimate what distance the driver observes while driving around the city. To control safe driving, a solution is needed that will be able to track the distance between cars in real time. This will reduce the number of accidents and save the lives of drivers and passengers.
The participants of the championship are faced with the task of developing an algorithm that allows determining the distance to the car in front in real-time, using a dataset of photos of cars from different distances. Subsequently, this algorithm can be used in navigation systems to warn of a dangerous approach and to monitor compliance with the distance.
Author’s solution
The author’s solution is based on two datasets:
- Digital Breakthrough 2022: Ulyanovsk - Car Plate Detection (current)
- Digital Breakthrough 2022: Ulyanovsk - Car Object Detection (available on DatasetNinja)
The baseline provided by the organizers of the championship was taken as the basis of the solution, which was improved, namely, in addition to detecting the car, the number, and the car-mating badge were also detected, which gave a greater increase in speed. Additional models were retrained on the competition data train (manual marking using an online service makesense.ai ) using the YOLOv5 (YOLOv5m6) model. CatBoostRegressor with improved parameters was used to predict the distance.
Summary #
Digital Breakthrough 2022: Ulyanovsk - Car Plate Detection is a dataset for an object detection task. Possible applications of the dataset could be in the safety industry.
The dataset consists of 482 images with 482 labeled objects belonging to 1 single class (plate).
Images in the Digital Breakthrough 2022: Car Plate dataset have bounding box annotations. All images are labeled (i.e. with annotations). There is 1 split in the dataset: train (482 images). The dataset was released in 2022 by the Government of the Ulyanovsk Region and NordClan.
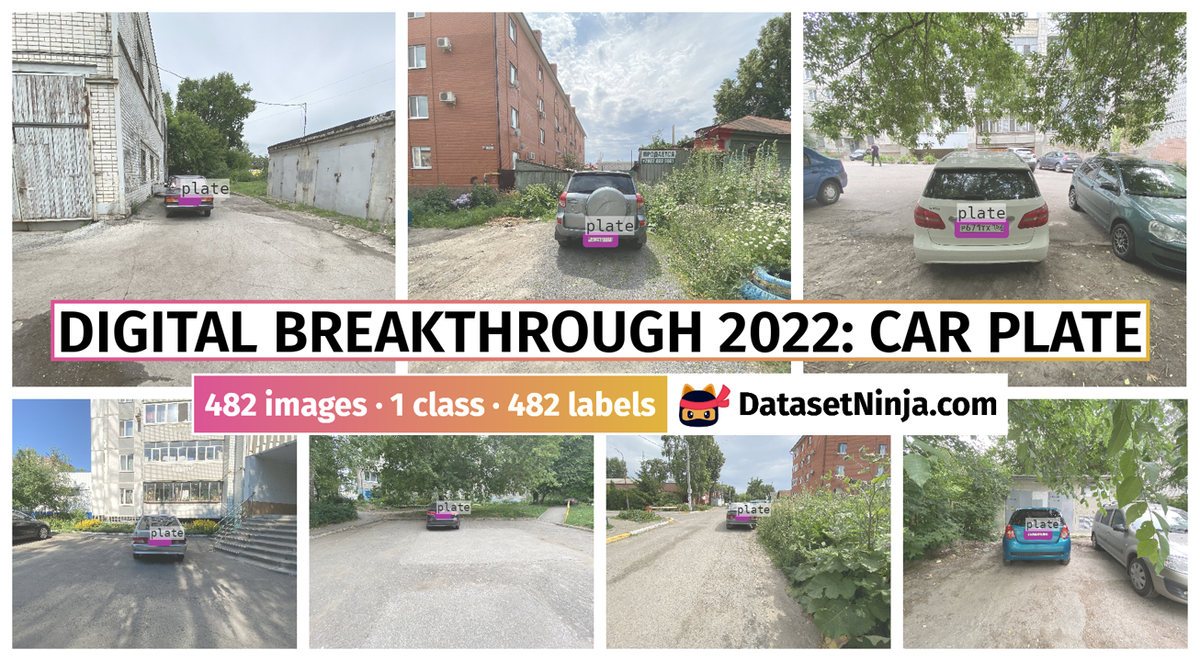
Explore #
Digital Breakthrough 2022: Car Plate dataset has 482 images. Click on one of the examples below or open "Explore" tool anytime you need to view dataset images with annotations. This tool has extended visualization capabilities like zoom, translation, objects table, custom filters and more. Hover the mouse over the images to hide or show annotations.
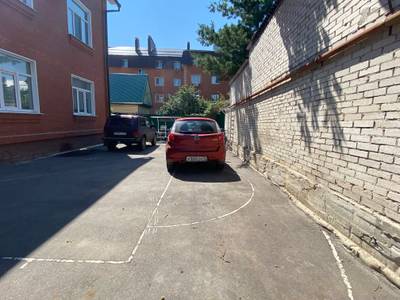
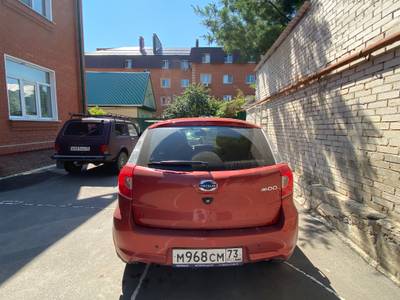

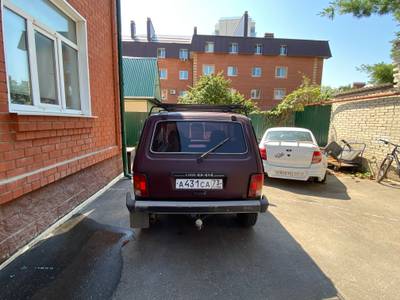

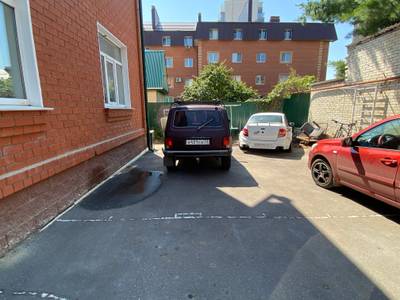
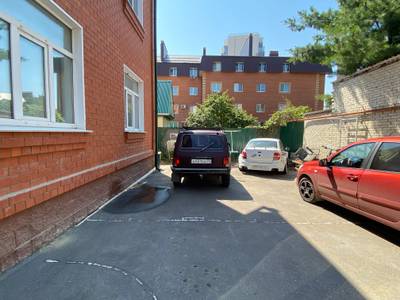
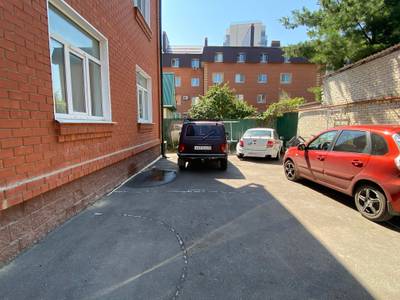
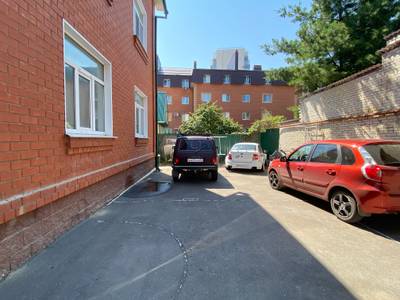

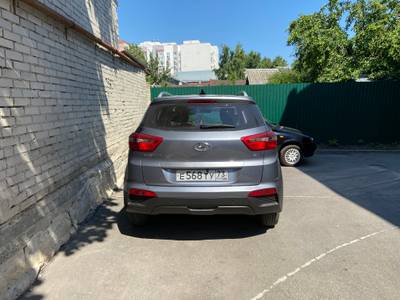
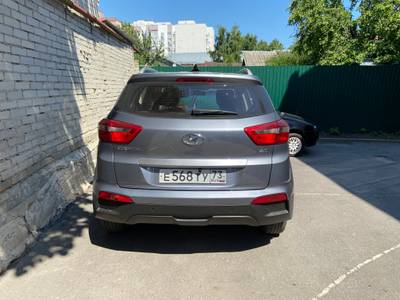
Class balance #
There are 1 annotation classes in the dataset. Find the general statistics and balances for every class in the table below. Click any row to preview images that have labels of the selected class. Sort by column to find the most rare or prevalent classes.
Class ã…¤ | Images ã…¤ | Objects ã…¤ | Count on image average | Area on image average |
---|---|---|---|---|
plateâž” rectangle | 482 | 482 | 1 | 0.21% |
Images #
Explore every single image in the dataset with respect to the number of annotations of each class it has. Click a row to preview selected image. Sort by any column to find anomalies and edge cases. Use horizontal scroll if the table has many columns for a large number of classes in the dataset.
Object distribution #
Interactive heatmap chart for every class with object distribution shows how many images are in the dataset with a certain number of objects of a specific class. Users can click cell and see the list of all corresponding images.
Class sizes #
The table below gives various size properties of objects for every class. Click a row to see the image with annotations of the selected class. Sort columns to find classes with the smallest or largest objects or understand the size differences between classes.
Class | Object count | Avg area | Max area | Min area | Min height | Min height | Max height | Max height | Avg height | Avg height | Min width | Min width | Max width | Max width |
---|---|---|---|---|---|---|---|---|---|---|---|---|---|---|
plate rectangle | 482 | 0.21% | 2.26% | 0.02% | 24px | 0.79% | 274px | 9.06% | 72px | 2.38% | 53px | 1.75% | 1005px | 24.93% |
Spatial Heatmap #
The heatmaps below give the spatial distributions of all objects for every class. These visualizations provide insights into the most probable and rare object locations on the image. It helps analyze objects' placements in a dataset.
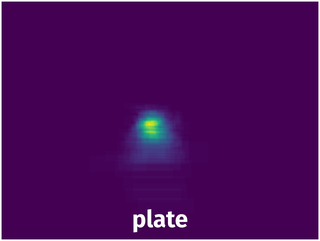
Objects #
Table contains all 482 objects. Click a row to preview an image with annotations, and use search or pagination to navigate. Sort columns to find outliers in the dataset.
Object ID ã…¤ | Class ã…¤ | Image name click row to open | Image size height x width | Height ã…¤ | Height ã…¤ | Width ã…¤ | Width ã…¤ | Area ã…¤ |
---|---|---|---|---|---|---|---|---|
1âž” | plate rectangle | img_2476.jpg | 3024 x 4032 | 77px | 2.55% | 301px | 7.47% | 0.19% |
2âž” | plate rectangle | img_2003.jpg | 3024 x 4032 | 77px | 2.55% | 319px | 7.91% | 0.2% |
3âž” | plate rectangle | img_2492.jpg | 3024 x 4032 | 32px | 1.06% | 139px | 3.45% | 0.04% |
4âž” | plate rectangle | img_1903.jpg | 3024 x 4032 | 82px | 2.71% | 358px | 8.88% | 0.24% |
5âž” | plate rectangle | img_1933.jpg | 3024 x 4032 | 57px | 1.88% | 264px | 6.55% | 0.12% |
6âž” | plate rectangle | img_2458.jpg | 3024 x 4032 | 26px | 0.86% | 125px | 3.1% | 0.03% |
7âž” | plate rectangle | img_2408.jpg | 3024 x 4032 | 95px | 3.14% | 336px | 8.33% | 0.26% |
8âž” | plate rectangle | img_2404.jpg | 3024 x 4032 | 51px | 1.69% | 180px | 4.46% | 0.08% |
9âž” | plate rectangle | img_1909.jpg | 3024 x 4032 | 40px | 1.32% | 157px | 3.89% | 0.05% |
10âž” | plate rectangle | img_2012.jpg | 3024 x 4032 | 60px | 1.98% | 253px | 6.27% | 0.12% |
License #
License is unknown for the Digital Breakthrough 2022: Ulyanovsk - Car Plate Detection dataset.
Citation #
If you make use of the Digital Breakthrough 2022: Car Plate data, please cite the following reference:
@dataset{Digital Breakthrough 2022: Car Plate,
author={Andrew Teplov},
title={Digital Breakthrough 2022: Ulyanovsk - Car Plate Detection},
year={2022},
url={https://www.kaggle.com/datasets/andrewteplov/car-plate-object-detetcion}
}
If you are happy with Dataset Ninja and use provided visualizations and tools in your work, please cite us:
@misc{ visualization-tools-for-digital-breakthrough-2022-ulyanovsk-car-plate-dataset,
title = { Visualization Tools for Digital Breakthrough 2022: Car Plate Dataset },
type = { Computer Vision Tools },
author = { Dataset Ninja },
howpublished = { \url{ https://datasetninja.com/digital-breakthrough-2022-ulyanovsk-car-plate } },
url = { https://datasetninja.com/digital-breakthrough-2022-ulyanovsk-car-plate },
journal = { Dataset Ninja },
publisher = { Dataset Ninja },
year = { 2025 },
month = { jun },
note = { visited on 2025-06-25 },
}
Download #
Please visit dataset homepage to download the data.
Disclaimer #
Our gal from the legal dep told us we need to post this:
Dataset Ninja provides visualizations and statistics for some datasets that can be found online and can be downloaded by general audience. Dataset Ninja is not a dataset hosting platform and can only be used for informational purposes. The platform does not claim any rights for the original content, including images, videos, annotations and descriptions. Joint publishing is prohibited.
You take full responsibility when you use datasets presented at Dataset Ninja, as well as other information, including visualizations and statistics we provide. You are in charge of compliance with any dataset license and all other permissions. You are required to navigate datasets homepage and make sure that you can use it. In case of any questions, get in touch with us at hello@datasetninja.com.