Introduction #
In the past decade, significant progress in object detection has been made in natural images, but authors of the DOTA v2.0: Dataset of Object deTection in Aerial images note that this progress hasn’t extended to aerial images. The main reason for this discrepancy is the substantial variations in object scale and orientation caused by the bird’s-eye view of aerial images. One major obstacle to the development of object detection in aerial images (ODAI) is the lack of large-scale benchmark datasets. The DOTA dataset contains 1,793,658 object instances spanning 18 different categories, all annotated with oriented bounding box annotations (OBB). These annotations were collected from a total of 11,268 aerial images. Using this extensive and meticulously annotated dataset, the authors establish baselines covering ten state-of-the-art algorithms, each with over 70 different configurations. These configurations are evaluated for both speed and accuracy performance.
Regarding the construction of DOTA, the authors emphasize the importance of collecting images from various sensors and platforms to address dataset biases. They describe the acquisition of such as Google Earth, the Gaofen-2 Satellite, Jilin-1 Satellite, and CycloMedia airborne images. These images vary in resolution and sensor type, reflecting real-world conditions. Additionally, they detail the selection of 18 object categories for annotation based on their relevance and frequency in real-world applications.
An example image taken from DOTA. (a) A typical image in DOTA consisting of many instances from multiple categories. (b), ©, (d), (e) are cropped from the source image. We can see that instances such as small vehicles have arbitrary orientations. There is also a massive scale variation across different instances. Moreover, the instances are not distributed uniformly. The instances are sparse in most areas but crowded in some local areas. Large vehicles and ships have large ARs. (f) and (g) exhibit the size and orientation histograms, respectively, for all instances.
The images used in DOTA-v2.0 are from three distinct image source: Google Earth images, GF-2 and JL-1 (GF&JL) satellite images, and the CycloMedia airborne images. Statistical data, as provided in Table 3, outlines aspects like image area, object area, and foreground ratio. Notably, Google Earth images, thoughtfully selected, constitute the majority of positive samples, although negative samples also play a crucial role in mitigating sample bias. The distributions of objects in GF&JL satellite images and CycloMedia airborne images closely resemble real-world scenarios. It’s worth noting that DOTA-v2.0 includes both RGB and grayscale images, and the images from different sources undergo specific spectral rendering and bit-length optimization processes. These processes ensure consistency in structure and appearance information, making the images suitable for recognition-oriented tasks. While acquisition date is available for images from GF-2, JL-1, and CycloMedia, only 27% of Google Earth images include this information. Given that the primary objective of the task is object recognition in aerial images based on visual cues, the geolocation of images is considered insignificant, and thus, DOTA-v2.0 does not provide geolocation data for its images.
Google Earth | GF&JL | Aerial | All | |
---|---|---|---|---|
# of images | 10186 | 516 | 566 | 11268 |
Images Area (106) | 29,991 | 75,854 | 20,462 | 126,306 |
Objects Area (1066) | 1,111 | 243 | 673 | 2,027 |
Foreground Ratio | 0.037 | 0.003 | 0.033 | 0.016 |
Another valuable meta inforamtion is ground sample distance (GSD), which measures the distance between pixel centers on Earth. GSD is valuable for calculating actual object sizes, which, in turn, can be employed for identifying mislabeled or misclassified instances. Additionally, GSD can be integrated directly into object detectors to enhance the accuracy of category classification for objects with less physical size variation. The authors highlight that GSDs vary across the dataset, with different values for images from GF-2, JL-1, CycloMedia, and Google Earth. Moreover, it’s noted that GSD information is missing in 70% of the images within DOTA-v2.0. However, the absence of GSD data does not significantly impact applications that rely on GSD, as machine learning-based methods can be utilized to estimate it.
A distinct characteristic of DOTA is the diverse orientations of objects in overhead view images. Unlike other object detection tasks, these objects aren’t constrained by gravity, resulting in a wide range of possible angles for object orientation. The authors emphasize that this unique distribution of object angles in DOTA makes it an ideal dataset for research on rotation-invariant feature extraction and oriented object detection.
The aspect ratio (AR) of instances is essential for anchor-based models. DOTA considers two ARs for instances: one based on the original Oriented Bounding Boxes (OBBs) and another based on Horizontal Bounding Boxes (HBBs). The distribution of these two ARs is explored in the dataset. Instances exhibit significant variation in aspect ratio, with many instances having a large aspect ratio.
The number of instances per image varies widely in DOTA, with some images containing up to 1000 instances while others have just one instance. This property is compared to other object detection datasets. The density of instances varies across categories, with some categories having significantly denser instances than others. The authors provide quantitative analysis by measuring the distance between instances within the same category and binning them into three density categories: dense, normal, and sparse. The density is measured by calculating the distance to the closest instance.
The authors also note significant improvements in DOTA from earlier versions (DOTA v1.0 and DOTA v1.5), which included addressing challenges related to tiny objects, large-scale images, and multi-source overhead images. In DOTA-v2.0, there are 18 common categories, 11,268 images, and 1,793,658 instances, with the addition of new categories like airport and helipad. The dataset is divided into train, val, test-dev, and test-challenge (not available at download source - comm. dninja) subsets, each with specific proportions to avoid overfitting. Additionally, two test subsets, test-dev and test-challenge, have been introduced for evaluation, following a similar structure to the MS COCO dataset.
In summary, the authors of the dataset have made significant contributions to the field of object detection in aerial images by providing a comprehensive dataset, baselines, and tools to facilitate research and development in this domain. They have addressed various challenges and limitations to create a more robust benchmark dataset for oriented object detection in aerial images.
Summary #
DOTA v2.0: Dataset of Object deTection in Aerial images is a dataset for an object detection task. It is used in the geospatial domain.
The dataset consists of 5215 images with 349589 labeled objects belonging to 18 different classes including small vehicle, large vehicle, ship, and other: harbor, tennis court, airport, bridge, swimming pool, ground track field, roundabout, storage tank, plane, soccer ball field, baseball diamond, basketball court, helicopter, container crane, and helipad.
Images in the DOTA dataset have bounding box annotations. There are 2793 (54% of the total) unlabeled images (i.e. without annotations). There are 3 splits in the dataset: test-dev (2792 images), train (1830 images), and val (593 images). Additionally, images contain meta-info about acquisition date, image source, and ground sample distance, while every OBB has boolean difficult tag. The dataset was released in 2021 by the CHI-NLD-USA-GER-ITL joint research group.
Here are the visualized examples for the classes:
Explore #
DOTA dataset has 5215 images. Click on one of the examples below or open "Explore" tool anytime you need to view dataset images with annotations. This tool has extended visualization capabilities like zoom, translation, objects table, custom filters and more. Hover the mouse over the images to hide or show annotations.
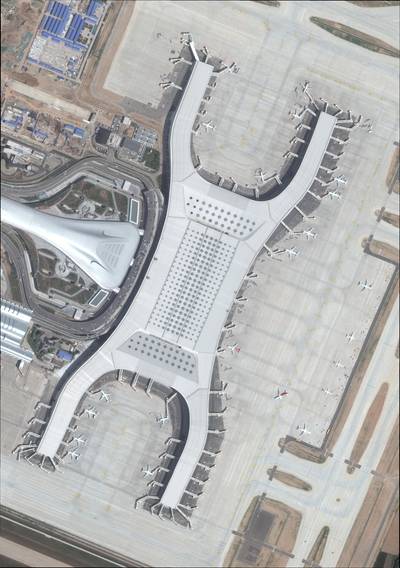













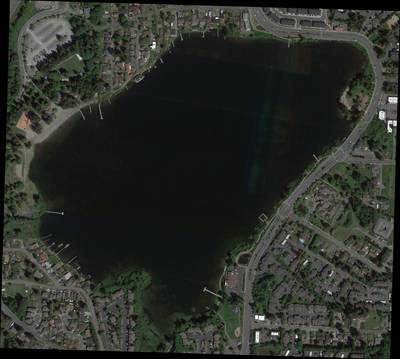





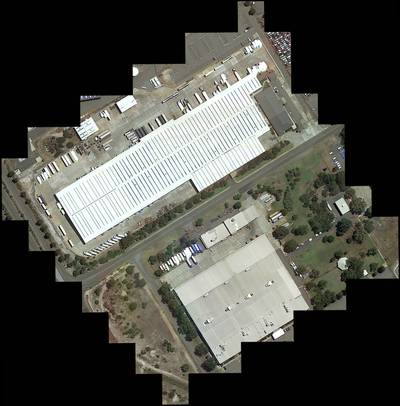








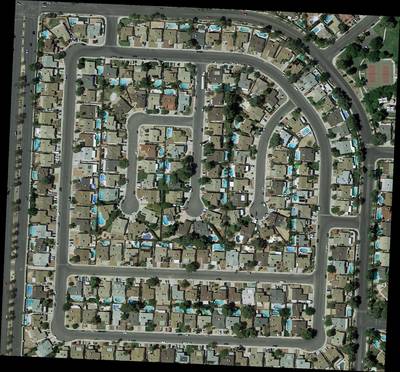
Class balance #
There are 18 annotation classes in the dataset. Find the general statistics and balances for every class in the table below. Click any row to preview images that have labels of the selected class. Sort by column to find the most rare or prevalent classes.
Class ㅤ | Images ㅤ | Objects ㅤ | Count on image average | Area on image average |
---|---|---|---|---|
small vehicle➔ polygon | 1285 | 219328 | 170.68 | 1.09% |
large vehicle➔ polygon | 777 | 29941 | 38.53 | 2.32% |
ship➔ polygon | 641 | 54353 | 84.79 | 1.78% |
harbor➔ polygon | 529 | 8902 | 16.83 | 5.22% |
tennis court➔ polygon | 422 | 3560 | 8.44 | 6.94% |
airport➔ polygon | 407 | 410 | 1.01 | 14.52% |
bridge➔ polygon | 382 | 3043 | 7.97 | 0.21% |
swimming pool➔ polygon | 348 | 3230 | 9.28 | 0.41% |
ground track field➔ polygon | 335 | 684 | 2.04 | 3.13% |
roundabout➔ polygon | 317 | 885 | 2.79 | 0.69% |
Co-occurrence matrix #
Co-occurrence matrix is an extremely valuable tool that shows you the images for every pair of classes: how many images have objects of both classes at the same time. If you click any cell, you will see those images. We added the tooltip with an explanation for every cell for your convenience, just hover the mouse over a cell to preview the description.
Images #
Explore every single image in the dataset with respect to the number of annotations of each class it has. Click a row to preview selected image. Sort by any column to find anomalies and edge cases. Use horizontal scroll if the table has many columns for a large number of classes in the dataset.
Class sizes #
The table below gives various size properties of objects for every class. Click a row to see the image with annotations of the selected class. Sort columns to find classes with the smallest or largest objects or understand the size differences between classes.
Class | Object count | Avg area | Max area | Min area | Min height | Min height | Max height | Max height | Avg height | Avg height | Min width | Min width | Max width | Max width |
---|---|---|---|---|---|---|---|---|---|---|---|---|---|---|
small vehicle polygon | 219328 | 0.01% | 0.43% | 0% | 3px | 0.06% | 204px | 14.26% | 20px | 0.79% | 3px | 0.04% | 208px | 12.19% |
ship polygon | 54353 | 0.02% | 25.28% | 0% | 3px | 0.01% | 1751px | 92.71% | 40px | 1.68% | 4px | 0.01% | 1329px | 85.43% |
large vehicle polygon | 29941 | 0.06% | 0.88% | 0% | 4px | 0.03% | 457px | 19.38% | 48px | 2.98% | 4px | 0.02% | 1727px | 27.05% |
plane polygon | 11281 | 0.1% | 15.31% | 0% | 8px | 0.05% | 843px | 47.89% | 102px | 3.26% | 6px | 0.04% | 886px | 52.79% |
storage tank polygon | 10617 | 0.01% | 1.78% | 0% | 3px | 0.02% | 443px | 11.11% | 29px | 0.91% | 4px | 0.02% | 477px | 16.46% |
harbor polygon | 8902 | 0.3% | 22.92% | 0% | 5px | 0.04% | 1703px | 87.44% | 144px | 8.55% | 7px | 0.03% | 3571px | 87.18% |
tennis court polygon | 3560 | 0.81% | 6.34% | 0% | 18px | 0.07% | 376px | 40.29% | 134px | 11.69% | 10px | 0.04% | 453px | 42.4% |
swimming pool polygon | 3230 | 0.04% | 3.12% | 0% | 5px | 0.04% | 531px | 28.94% | 43px | 1.89% | 5px | 0.04% | 542px | 28.71% |
bridge polygon | 3043 | 0.02% | 3.88% | 0% | 4px | 0.02% | 1182px | 100% | 54px | 1.62% | 4px | 0.02% | 1308px | 72.76% |
baseball diamond polygon | 965 | 0.42% | 13.27% | 0% | 10px | 0.06% | 1002px | 45.16% | 97px | 4.3% | 10px | 0.06% | 983px | 43.07% |
Spatial Heatmap #
The heatmaps below give the spatial distributions of all objects for every class. These visualizations provide insights into the most probable and rare object locations on the image. It helps analyze objects' placements in a dataset.
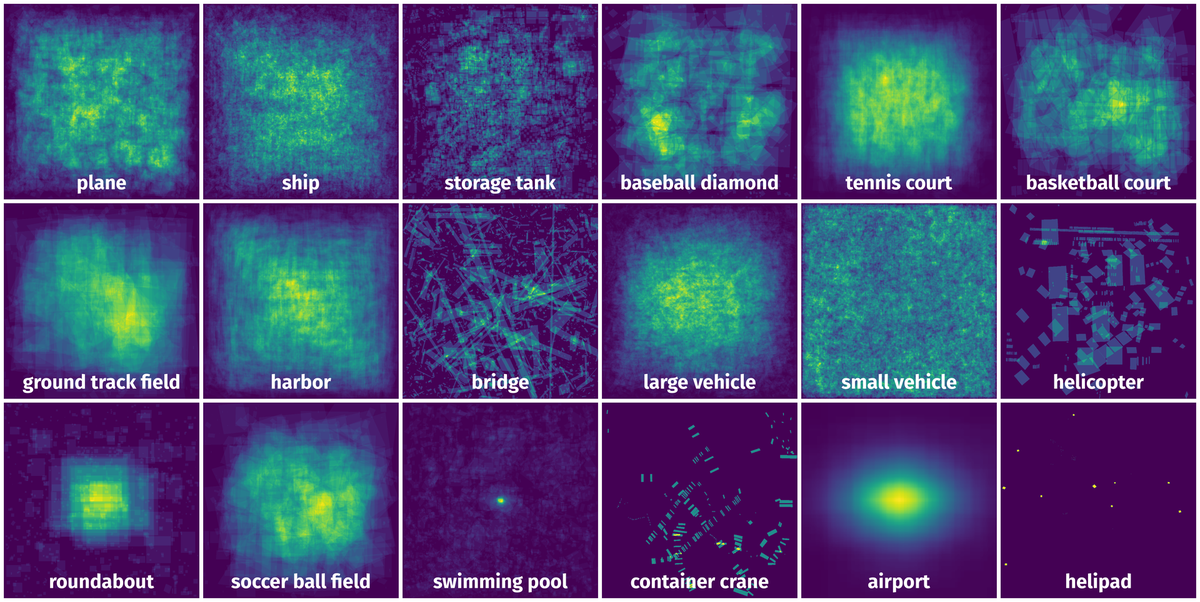
Objects #
Table contains all 93911 objects. Click a row to preview an image with annotations, and use search or pagination to navigate. Sort columns to find outliers in the dataset.
Object ID ㅤ | Class ㅤ | Image name click row to open | Image size height x width | Height ㅤ | Height ㅤ | Width ㅤ | Width ㅤ | Area ㅤ |
---|---|---|---|---|---|---|---|---|
1➔ | small vehicle polygon | P0190.png | 1065 x 3018 | 18px | 1.69% | 8px | 0.27% | 0% |
2➔ | small vehicle polygon | P0190.png | 1065 x 3018 | 16px | 1.5% | 8px | 0.27% | 0% |
3➔ | small vehicle polygon | P0190.png | 1065 x 3018 | 19px | 1.78% | 10px | 0.33% | 0% |
4➔ | small vehicle polygon | P0190.png | 1065 x 3018 | 16px | 1.5% | 9px | 0.3% | 0% |
5➔ | small vehicle polygon | P0190.png | 1065 x 3018 | 17px | 1.6% | 9px | 0.3% | 0% |
6➔ | small vehicle polygon | P0190.png | 1065 x 3018 | 18px | 1.69% | 10px | 0.33% | 0% |
7➔ | small vehicle polygon | P0190.png | 1065 x 3018 | 17px | 1.6% | 9px | 0.3% | 0% |
8➔ | small vehicle polygon | P0190.png | 1065 x 3018 | 17px | 1.6% | 8px | 0.27% | 0% |
9➔ | small vehicle polygon | P0190.png | 1065 x 3018 | 17px | 1.6% | 9px | 0.3% | 0% |
10➔ | small vehicle polygon | P0190.png | 1065 x 3018 | 18px | 1.69% | 8px | 0.27% | 0% |
License #
The DOTA images are collected from the Google Earth, GF-2 and JL-1 satellite provided by the China Centre for Resources Satellite Data and Application, and aerial images provided by CycloMedia B.V. DOTA consists of RGB images and grayscale images. The RGB images are from Google Earth and CycloMedia, while the grayscale images are from the panchromatic band of GF-2 and JL-1 satellite images. All the images are stored in ‘png’ formats.
Use of the Google Earth images must respect the “Google Earth” terms of use.
All images and their associated annotations in DOTA can be used for academic purposes only, but any commercial use is prohibited.
Citation #
If you make use of the DOTA data, please cite the following reference:
@ARTICLE{9560031,
author={Ding, Jian and Xue, Nan and Xia, Gui-Song and Bai, Xiang and Yang, Wen and Yang, Michael and Belongie, Serge and Luo, Jiebo and Datcu, Mihai and Pelillo, Marcello and Zhang, Liangpei},
journal={IEEE Transactions on Pattern Analysis and Machine Intelligence},
title={Object Detection in Aerial Images: A Large-Scale Benchmark and Challenges},
year={2021},
volume={},
number={},
pages={1-1},
doi={10.1109/TPAMI.2021.3117983}
}
@InProceedings{Xia_2018_CVPR,
author = {Xia, Gui-Song and Bai, Xiang and Ding, Jian and Zhu, Zhen and Belongie, Serge and Luo, Jiebo and Datcu, Mihai and Pelillo, Marcello and Zhang, Liangpei},
title = {DOTA: A Large-Scale Dataset for Object Detection in Aerial Images},
booktitle = {The IEEE Conference on Computer Vision and Pattern Recognition (CVPR)},
month = {June},
year = {2018}
}
@InProceedings{Ding_2019_CVPR,
author = {Jian Ding, Nan Xue, Yang Long, Gui-Song Xia, Qikai Lu},
title = {Learning RoI Transformer for Detecting Oriented Objects in Aerial Images},
booktitle = {The IEEE Conference on Computer Vision and Pattern Recognition (CVPR)},
month = {June},
year = {2019}
}
If you are happy with Dataset Ninja and use provided visualizations and tools in your work, please cite us:
@misc{ visualization-tools-for-dota-dataset,
title = { Visualization Tools for DOTA Dataset },
type = { Computer Vision Tools },
author = { Dataset Ninja },
howpublished = { \url{ https://datasetninja.com/dota } },
url = { https://datasetninja.com/dota },
journal = { Dataset Ninja },
publisher = { Dataset Ninja },
year = { 2024 },
month = { oct },
note = { visited on 2024-10-31 },
}
Download #
Dataset DOTA can be downloaded in Supervisely format:
As an alternative, it can be downloaded with dataset-tools package:
pip install --upgrade dataset-tools
… using following python code:
import dataset_tools as dtools
dtools.download(dataset='DOTA', dst_dir='~/dataset-ninja/')
Make sure not to overlook the python code example available on the Supervisely Developer Portal. It will give you a clear idea of how to effortlessly work with the downloaded dataset.
The data in original format can be downloaded here.
Disclaimer #
Our gal from the legal dep told us we need to post this:
Dataset Ninja provides visualizations and statistics for some datasets that can be found online and can be downloaded by general audience. Dataset Ninja is not a dataset hosting platform and can only be used for informational purposes. The platform does not claim any rights for the original content, including images, videos, annotations and descriptions. Joint publishing is prohibited.
You take full responsibility when you use datasets presented at Dataset Ninja, as well as other information, including visualizations and statistics we provide. You are in charge of compliance with any dataset license and all other permissions. You are required to navigate datasets homepage and make sure that you can use it. In case of any questions, get in touch with us at hello@datasetninja.com.