Introduction #
The Swin-Expand: Fine-Grained Polyp Segmentation and Classification dataset contain 1126 high-definition images representing the three main histological classes. Colorectal cancer is one of the main causes of cancer death worldwide. Colonoscopy is the gold standard screening tool as it allows lesion detection and removal during the same procedure. During the last decades, several efforts have been made to develop CAD systems to assist clinicians in lesion detection and classification. Regarding the latter, and in order to be used in the exploration room as part of resect and discard or leave-in-situ strategies, these systems must identify correctly all different lesion types. This is a challenging task, as the data used to train these systems presents great inter-class similarity, high class imbalance, and low representation of clinically relevant histology classes such as serrated sessile adenomas.
The authors introduced a new polyp segmentation and classification method, Swin-Expand. Based on Swin-Transformer, it uses a simple and lightweight decoder. The performance of this method has been assessed on a novel dataset, comprising 1126 high-definition images representing the three main histological classes. Results show a clear improvement in both segmentation and classification performance, also achieving competitive results when tested in public datasets. These results confirm that both the method and the data are important to obtain more accurate polyp representations.
Summary #
Swin-Expand: Fine-Grained Polyp Segmentation and Classification is a dataset for semantic segmentation, object detection, and classification tasks. It is used in the medical industry.
The dataset consists of 1126 images with 1126 labeled objects belonging to 1 single class (polyp).
Images in the Fine Grained Polyp dataset have . All images are labeled (i.e. with annotations). There are 3 splits in the dataset: train (788 images), test (225 images), and val (113 images). Alternatively, the dataset could be split into 3 cls: ad (760 images), hp (190 images), and ass (176 images). Additionally, every image marked with its histologia tag. The dataset was released in 2023 by the ES joint research group.
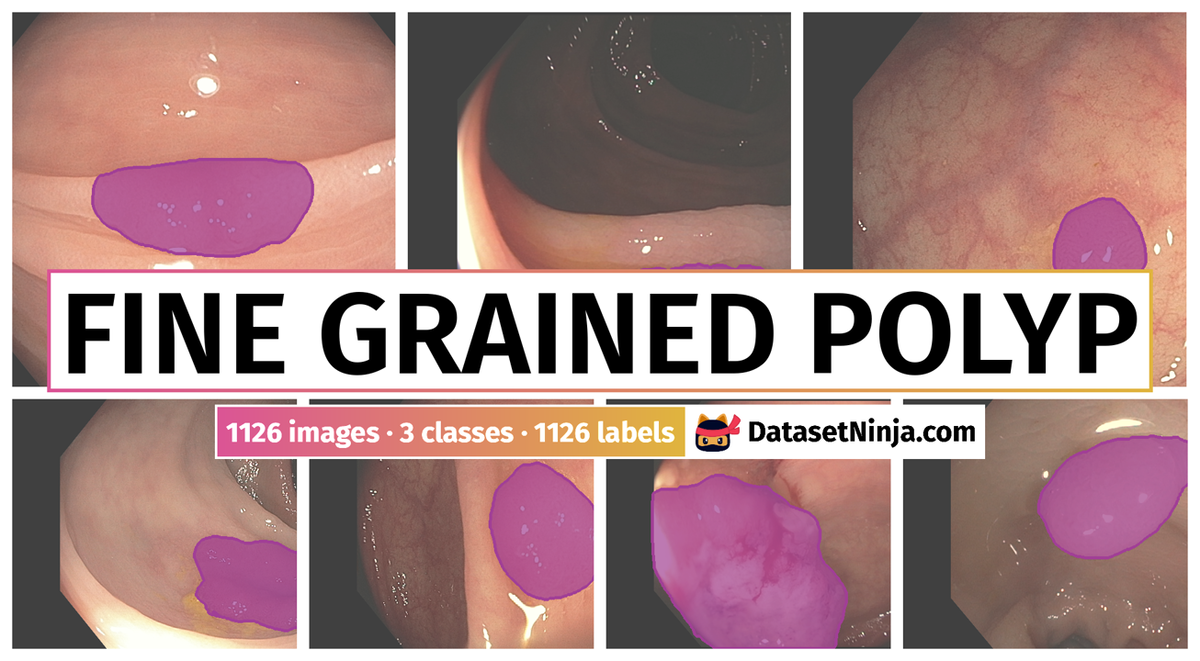
Explore #
Fine Grained Polyp dataset has 1126 images. Click on one of the examples below or open "Explore" tool anytime you need to view dataset images with annotations. This tool has extended visualization capabilities like zoom, translation, objects table, custom filters and more. Hover the mouse over the images to hide or show annotations.
Class balance #
There are 1 annotation classes in the dataset. Find the general statistics and balances for every class in the table below. Click any row to preview images that have labels of the selected class. Sort by column to find the most rare or prevalent classes.
Class ㅤ | Images ㅤ | Objects ㅤ | Count on image average | Area on image average |
---|---|---|---|---|
polyp➔ mask | 1126 | 1126 | 1 | 9.59% |
Images #
Explore every single image in the dataset with respect to the number of annotations of each class it has. Click a row to preview selected image. Sort by any column to find anomalies and edge cases. Use horizontal scroll if the table has many columns for a large number of classes in the dataset.
Object distribution #
Interactive heatmap chart for every class with object distribution shows how many images are in the dataset with a certain number of objects of a specific class. Users can click cell and see the list of all corresponding images.
Class sizes #
The table below gives various size properties of objects for every class. Click a row to see the image with annotations of the selected class. Sort columns to find classes with the smallest or largest objects or understand the size differences between classes.
Class | Object count | Avg area | Max area | Min area | Min height | Min height | Max height | Max height | Avg height | Avg height | Min width | Min width | Max width | Max width |
---|---|---|---|---|---|---|---|---|---|---|---|---|---|---|
polyp mask | 1126 | 9.59% | 53.98% | 0.35% | 89px | 8.24% | 1080px | 100% | 455px | 42.25% | 82px | 4.27% | 1367px | 76.17% |
Spatial Heatmap #
The heatmaps below give the spatial distributions of all objects for every class. These visualizations provide insights into the most probable and rare object locations on the image. It helps analyze objects' placements in a dataset.
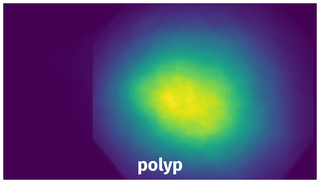
Objects #
Table contains all 1126 objects. Click a row to preview an image with annotations, and use search or pagination to navigate. Sort columns to find outliers in the dataset.
Object ID ㅤ | Class ㅤ | Image name click row to open | Image size height x width | Height ㅤ | Height ㅤ | Width ㅤ | Width ㅤ | Area ㅤ |
---|---|---|---|---|---|---|---|---|
1➔ | polyp mask | 472.tif.tiff | 1080 x 1920 | 193px | 17.87% | 335px | 17.45% | 1.98% |
2➔ | polyp mask | 219.tif.tiff | 1080 x 1920 | 381px | 35.28% | 439px | 22.86% | 6.48% |
3➔ | polyp mask | 559.tif.tiff | 1080 x 1920 | 439px | 40.65% | 570px | 29.69% | 8.78% |
4➔ | polyp mask | 190.tif.tiff | 1080 x 1920 | 360px | 33.33% | 319px | 16.61% | 4.07% |
5➔ | polyp mask | 232.tif.tiff | 1080 x 1920 | 181px | 16.76% | 174px | 9.06% | 1.13% |
6➔ | polyp mask | 582.tif.tiff | 1080 x 1920 | 1006px | 93.15% | 1362px | 70.94% | 50.95% |
7➔ | polyp mask | 332.tif.tiff | 1080 x 1920 | 338px | 31.3% | 155px | 8.07% | 1.31% |
8➔ | polyp mask | 606.tif.tiff | 1080 x 1920 | 266px | 24.63% | 267px | 13.91% | 2.71% |
9➔ | polyp mask | 545.tif.tiff | 1080 x 1920 | 747px | 69.17% | 862px | 44.9% | 18.21% |
10➔ | polyp mask | 318.tif.tiff | 1080 x 1920 | 852px | 78.89% | 1269px | 66.09% | 36.79% |
License #
This dataset is provided for academic and research purposes only. Any commercial use, reproduction, or distribution of this dataset, in whole or in part, is strictly prohibited.
By accessing and using this dataset, you agree to use it solely for non-commercial, research purposes and acknowledge that any commercial exploitation of this dataset is expressly forbidden. Users are required to cite the dataset appropriately in any publications or presentations that result from the use of this data.
Citation #
If you make use of the Fine Grained Polyp data, please cite the following reference:
@InProceedings{10.1007/978-3-031-45249-9_4,
author="Tudela, Yael and Garc{\'i}a-Rodr{\'i}guez, Ana and Fern{\'a}ndez-Esparrach, Gl{\'o}ria and Bernal, Jorge",
title="Towards Fine-Grained Polyp Segmentation and Classification",
booktitle="Clinical Image-Based Procedures, Fairness of AI in Medical Imaging, and Ethical and Philosophical Issues in Medical Imaging ",
year="2023",
publisher="Springer Nature Switzerland",
address="Cham",
pages="32--42",
isbn="978-3-031-45249-9"
}
If you are happy with Dataset Ninja and use provided visualizations and tools in your work, please cite us:
@misc{ visualization-tools-for-fine-grained-polyp-dataset,
title = { Visualization Tools for Fine Grained Polyp Dataset },
type = { Computer Vision Tools },
author = { Dataset Ninja },
howpublished = { \url{ https://datasetninja.com/fine-grained-polyp } },
url = { https://datasetninja.com/fine-grained-polyp },
journal = { Dataset Ninja },
publisher = { Dataset Ninja },
year = { 2025 },
month = { jul },
note = { visited on 2025-07-03 },
}
Download #
Dataset Fine Grained Polyp can be downloaded in Supervisely format:
As an alternative, it can be downloaded with dataset-tools package:
pip install --upgrade dataset-tools
… using following python code:
import dataset_tools as dtools
dtools.download(dataset='Fine Grained Polyp', dst_dir='~/dataset-ninja/')
Make sure not to overlook the python code example available on the Supervisely Developer Portal. It will give you a clear idea of how to effortlessly work with the downloaded dataset.
The data in original format can be downloaded here.
Disclaimer #
Our gal from the legal dep told us we need to post this:
Dataset Ninja provides visualizations and statistics for some datasets that can be found online and can be downloaded by general audience. Dataset Ninja is not a dataset hosting platform and can only be used for informational purposes. The platform does not claim any rights for the original content, including images, videos, annotations and descriptions. Joint publishing is prohibited.
You take full responsibility when you use datasets presented at Dataset Ninja, as well as other information, including visualizations and statistics we provide. You are in charge of compliance with any dataset license and all other permissions. You are required to navigate datasets homepage and make sure that you can use it. In case of any questions, get in touch with us at hello@datasetninja.com.