Introduction #
The authors of the Goat Images Dataset emphasize the significance of automatic individual animal identification in the context of livestock farming, with its potential to enhance breeding histories and contribute substantially to breeding and genetic management programs. In the current livestock identification practices, such as the utilization of various tags, tattoos, paint brands, and microchips, manual identification processes prevail, characterized by their time-consuming, expensive, and unreliable nature. In their research, authors present a deep learning-based solution, offering a fully automated pipeline for the detection and recognition of goats’ faces, a task more complex than human face recognition due to the high similarity among individual goats.
In the broader context of livestock farming, the automatic identification of individual animals has become a pivotal element, enabling comprehensive data collection and report generation to support farm management. While historical methods like tag identification have been employed, they pose challenges when applied to animals kept in groups, such as goats, as individual observation and data collection become arduous. To overcome this challenge, researchers are turning to deep learning techniques, which have found application in various aspects of animal farming, including biometric identification, activity monitoring, body condition scoring, and behavioral analysis.
The use of deep learning algorithms is gaining traction across the animal industry, addressing identification challenges by focusing on specific body parts, such as sheep retinas, cattle body patterns, and pig faces. However, challenges persist in obtaining clear and comprehensive datasets for these methods in real-world, uncontrolled environments. As of January 2022, the performance of CNNs for individual goat identification using facial images remains unexplored. The paper aims to extract robust features that enhance the distinguishability between individual goats in facial images. The proposed methodology comprises two primary steps: face detection and identity recognition. Given an input image, the first step involves the detection of faces and facial landmarks using a Yolo-based method, followed by cropping these regions into fixed segments. Facial landmarks serve to geometrically normalize the face. Ultimately, a custom CNN model extracts features for the detected regions and predicts classification probabilities. Notably, the approach covers not only face recognition but also automatic face detection and alignment based on the eye centroid, akin to human face recognition.
Two different types of image datasets are uploaded. The first dataset (dataset_1) is useful for detection purpose. This consists of a total 1680 goat image where the face, eye, mouth and ear bounding boxes are given in YOLO format. On the other hand, the second dataset (dataset_2) is for face recognition and facial expression analysis. In total 1311 images are captured from 10 individuals of a Chinese farm (34.2721° N, 108.0847° E).
Summary #
Goat Image Dataset is a dataset for object detection and identification tasks. It is used in the livestock industry, and in the biological research.
The dataset consists of 1767 images with 13761 labeled objects belonging to 4 different classes including face, ear, eye, and other: mouth.
Images in the Goat Image dataset have bounding box annotations. There are 87 (5% of the total) unlabeled images (i.e. without annotations). There are 2 splits in the dataset: dataset_1 (1680 images) and dataset_2 (87 images). Additionally, every image in dataset_2 contains information about goat_id. The dataset was released in 2020 by the Northwest A&F University, China.
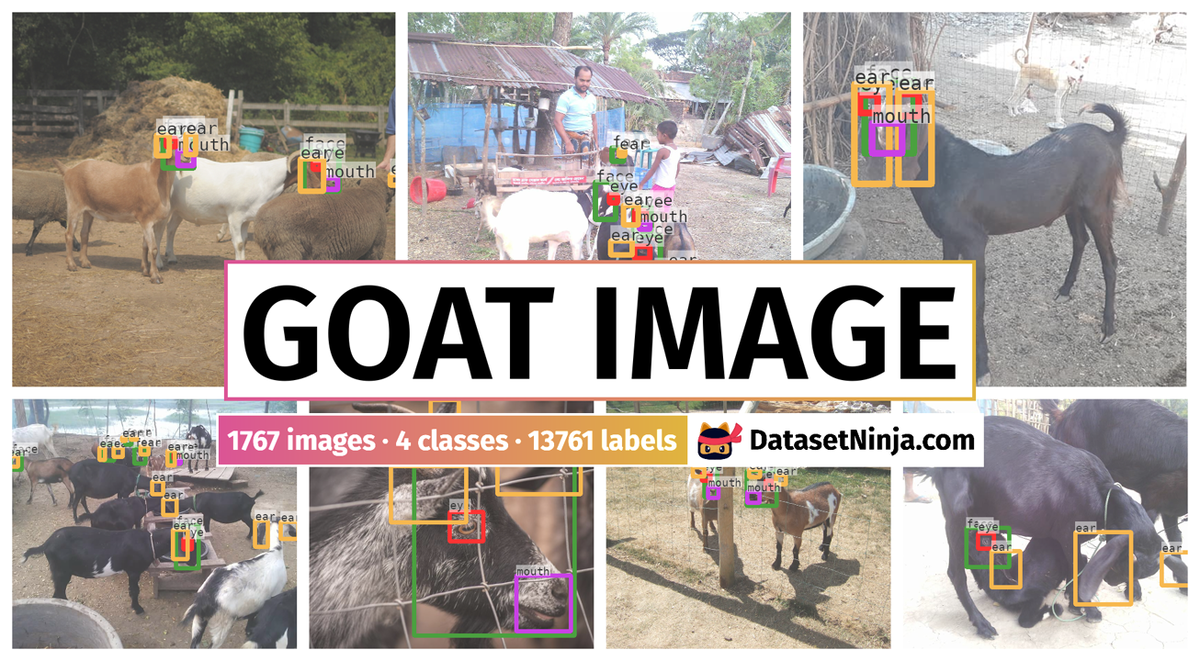
Explore #
Goat Image dataset has 1767 images. Click on one of the examples below or open "Explore" tool anytime you need to view dataset images with annotations. This tool has extended visualization capabilities like zoom, translation, objects table, custom filters and more. Hover the mouse over the images to hide or show annotations.
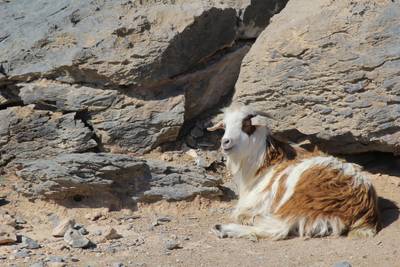
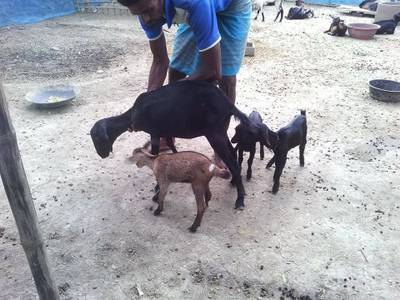
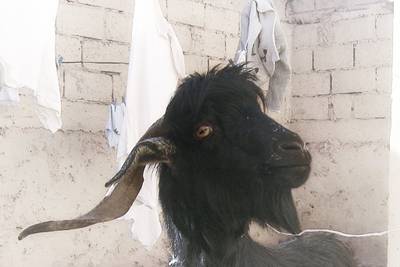


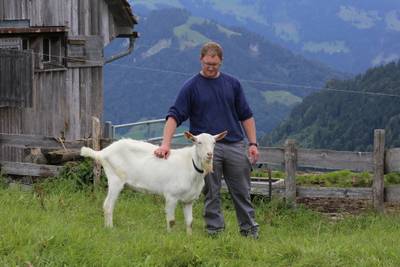

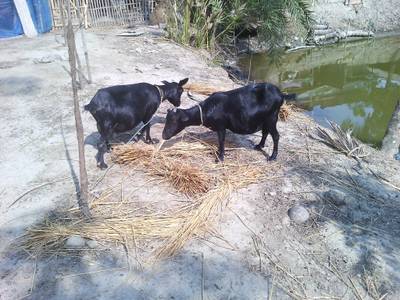
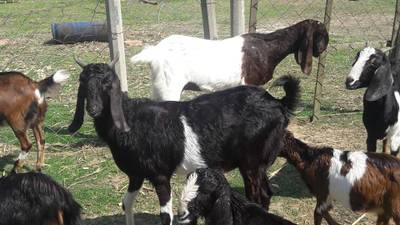
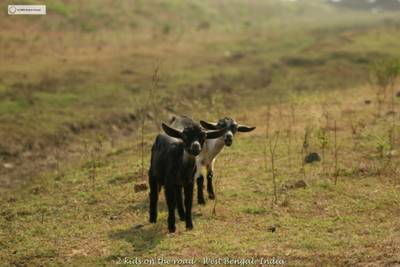
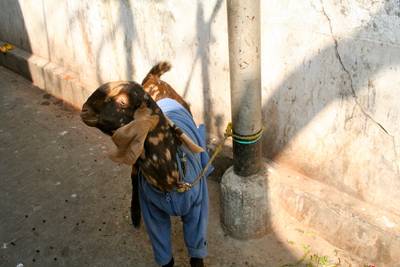
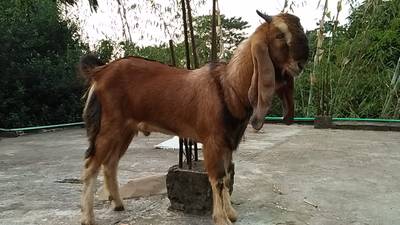

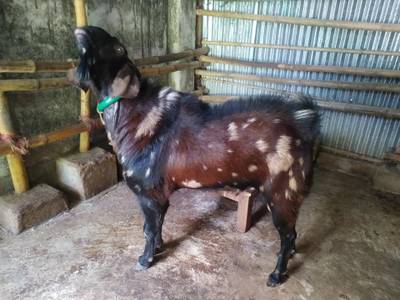


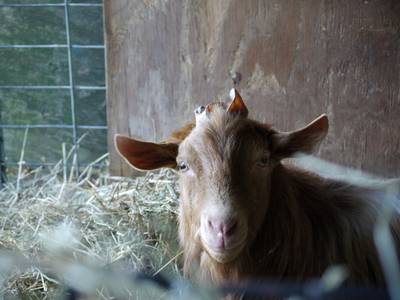
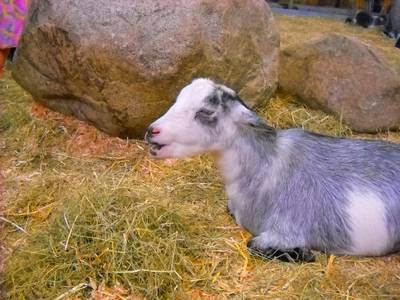
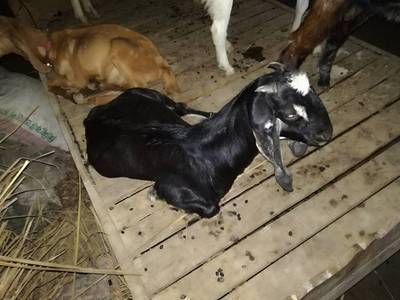

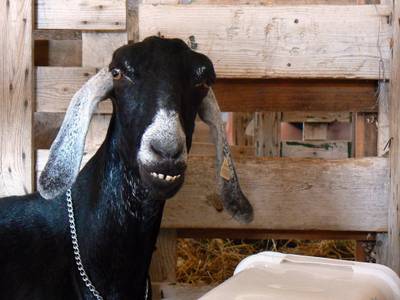
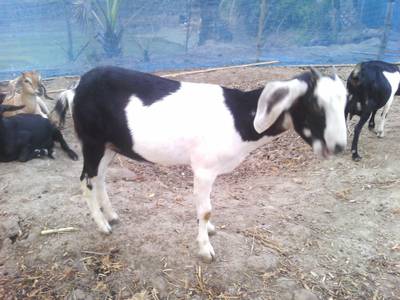
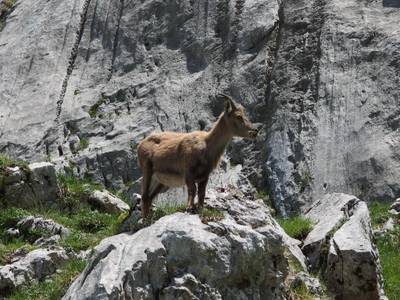
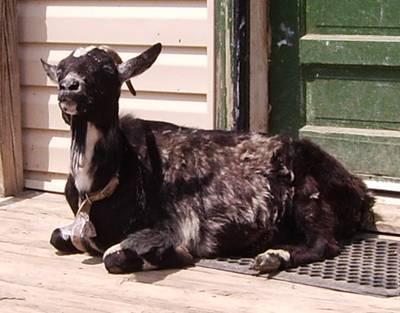
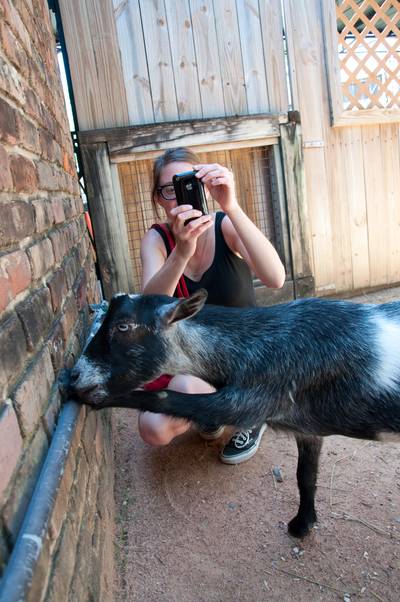
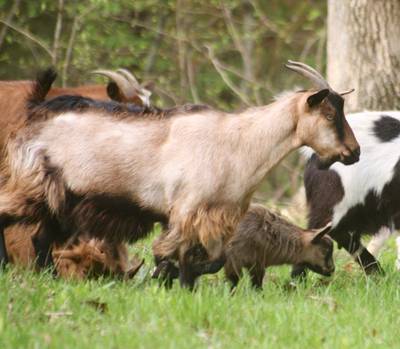
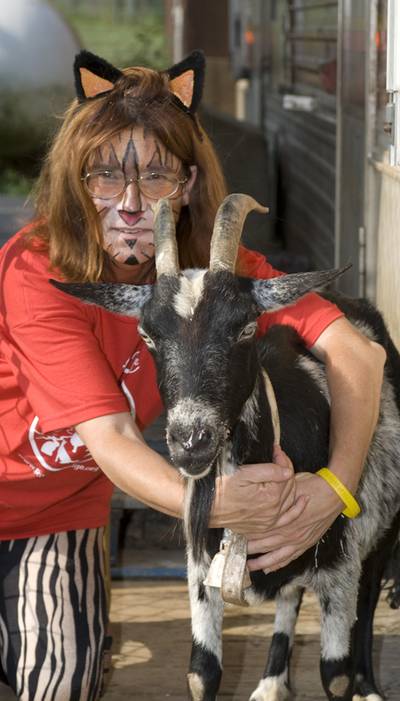
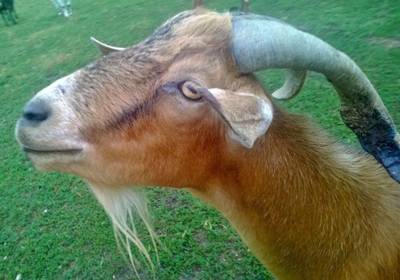
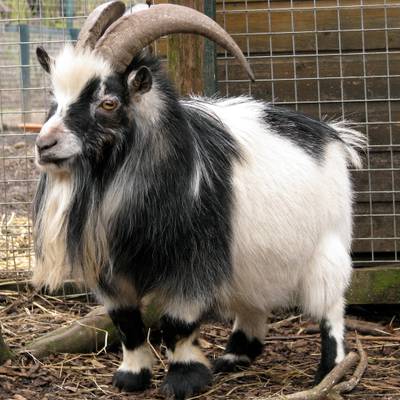

Class balance #
There are 4 annotation classes in the dataset. Find the general statistics and balances for every class in the table below. Click any row to preview images that have labels of the selected class. Sort by column to find the most rare or prevalent classes.
Class ㅤ | Images ㅤ | Objects ㅤ | Count on image average | Area on image average |
---|---|---|---|---|
face➔ rectangle | 1680 | 3078 | 1.83 | 6.56% |
ear➔ rectangle | 1655 | 4771 | 2.88 | 3.09% |
eye➔ rectangle | 1632 | 3326 | 2.04 | 0.57% |
mouth➔ rectangle | 1628 | 2586 | 1.59 | 1.13% |
Co-occurrence matrix #
Co-occurrence matrix is an extremely valuable tool that shows you the images for every pair of classes: how many images have objects of both classes at the same time. If you click any cell, you will see those images. We added the tooltip with an explanation for every cell for your convenience, just hover the mouse over a cell to preview the description.
Images #
Explore every single image in the dataset with respect to the number of annotations of each class it has. Click a row to preview selected image. Sort by any column to find anomalies and edge cases. Use horizontal scroll if the table has many columns for a large number of classes in the dataset.
Object distribution #
Interactive heatmap chart for every class with object distribution shows how many images are in the dataset with a certain number of objects of a specific class. Users can click cell and see the list of all corresponding images.
Class sizes #
The table below gives various size properties of objects for every class. Click a row to see the image with annotations of the selected class. Sort columns to find classes with the smallest or largest objects or understand the size differences between classes.
Class | Object count | Avg area | Max area | Min area | Min height | Min height | Max height | Max height | Avg height | Avg height | Min width | Min width | Max width | Max width |
---|---|---|---|---|---|---|---|---|---|---|---|---|---|---|
ear rectangle | 4771 | 1.08% | 43.97% | 0.01% | 6px | 0.82% | 801px | 83.07% | 94px | 11.11% | 6px | 0.68% | 542px | 52.93% |
eye rectangle | 3326 | 0.28% | 19.67% | 0.01% | 6px | 0.94% | 482px | 62.76% | 41px | 5.02% | 7px | 0.73% | 321px | 31.35% |
face rectangle | 3078 | 3.61% | 85.28% | 0.01% | 8px | 1.11% | 860px | 100% | 152px | 18.62% | 6px | 0.62% | 881px | 92.99% |
mouth rectangle | 2586 | 0.71% | 22.59% | 0.01% | 5px | 0.78% | 445px | 65.15% | 58px | 7.15% | 6px | 0.77% | 380px | 39.97% |
Spatial Heatmap #
The heatmaps below give the spatial distributions of all objects for every class. These visualizations provide insights into the most probable and rare object locations on the image. It helps analyze objects' placements in a dataset.

Objects #
Table contains all 13761 objects. Click a row to preview an image with annotations, and use search or pagination to navigate. Sort columns to find outliers in the dataset.
Object ID ㅤ | Class ㅤ | Image name click row to open | Image size height x width | Height ㅤ | Height ㅤ | Width ㅤ | Width ㅤ | Area ㅤ |
---|---|---|---|---|---|---|---|---|
1➔ | face rectangle | 384.jpg | 720 x 960 | 206px | 28.61% | 184px | 19.17% | 5.48% |
2➔ | face rectangle | 384.jpg | 720 x 960 | 95px | 13.19% | 130px | 13.54% | 1.79% |
3➔ | face rectangle | 384.jpg | 720 x 960 | 78px | 10.83% | 124px | 12.92% | 1.4% |
4➔ | eye rectangle | 384.jpg | 720 x 960 | 62px | 8.61% | 58px | 6.04% | 0.52% |
5➔ | eye rectangle | 384.jpg | 720 x 960 | 35px | 4.86% | 29px | 3.02% | 0.15% |
6➔ | eye rectangle | 384.jpg | 720 x 960 | 31px | 4.31% | 39px | 4.06% | 0.17% |
7➔ | mouth rectangle | 384.jpg | 720 x 960 | 65px | 9.03% | 71px | 7.4% | 0.67% |
8➔ | ear rectangle | 384.jpg | 720 x 960 | 176px | 24.44% | 85px | 8.85% | 2.16% |
9➔ | mouth rectangle | 384.jpg | 720 x 960 | 32px | 4.44% | 36px | 3.75% | 0.17% |
10➔ | mouth rectangle | 384.jpg | 720 x 960 | 46px | 6.39% | 44px | 4.58% | 0.29% |
License #
Citation #
If you make use of the Goat Image data, please cite the following reference:
Billah, Masum; Jiantao, Yu; Jiang, Yu (2020),
“Goat Image Dataset”,
Mendeley Data, V2,
doi: 10.17632/4skwhnrscr.2
If you are happy with Dataset Ninja and use provided visualizations and tools in your work, please cite us:
@misc{ visualization-tools-for-goat-image-dataset-dataset,
title = { Visualization Tools for Goat Image Dataset },
type = { Computer Vision Tools },
author = { Dataset Ninja },
howpublished = { \url{ https://datasetninja.com/goat-image-dataset } },
url = { https://datasetninja.com/goat-image-dataset },
journal = { Dataset Ninja },
publisher = { Dataset Ninja },
year = { 2025 },
month = { may },
note = { visited on 2025-05-15 },
}
Download #
Dataset Goat Image can be downloaded in Supervisely format:
As an alternative, it can be downloaded with dataset-tools package:
pip install --upgrade dataset-tools
… using following python code:
import dataset_tools as dtools
dtools.download(dataset='Goat Image', dst_dir='~/dataset-ninja/')
Make sure not to overlook the python code example available on the Supervisely Developer Portal. It will give you a clear idea of how to effortlessly work with the downloaded dataset.
The data in original format can be downloaded here.
Disclaimer #
Our gal from the legal dep told us we need to post this:
Dataset Ninja provides visualizations and statistics for some datasets that can be found online and can be downloaded by general audience. Dataset Ninja is not a dataset hosting platform and can only be used for informational purposes. The platform does not claim any rights for the original content, including images, videos, annotations and descriptions. Joint publishing is prohibited.
You take full responsibility when you use datasets presented at Dataset Ninja, as well as other information, including visualizations and statistics we provide. You are in charge of compliance with any dataset license and all other permissions. You are required to navigate datasets homepage and make sure that you can use it. In case of any questions, get in touch with us at hello@datasetninja.com.