Introduction #
The creators of the GWHD: Global Wheat Head Dataset are working towards automating the wheat head counting process through Deep Learning. However, this task is visually intricate, given factors like plant overlap, wind-induced blurriness, and variations in appearance based on factors such as maturity, color, genotype, and orientation. The dataset itself is extensive, containing over 6000 images, each with dimensions of 1024x1024 pixels, encompassing more than 300,000 distinct wheat heads, each paired with its corresponding bounding boxes. These images were taken across 11 different countries and span 44 unique measurement sessions. A “measurement session” here means a set of images captured at a consistent location over a few hours.
Diverse ground-based phenotyping platforms and cameras captured these images at heights ranging from 1.8 m to 3 m above the ground. They utilized various camera setups, resulting in Ground Sampling Distances (GSDs) that range between 0.10 and 0.62 mm, along with different field of view angles. Although these images are acquired in a nadir-viewing direction, occasional geometric distortions may appear in some sub-datasets due to distinct camera lens characteristics.
The authors of the GWHD dataset emphasized the need to harmonize diverse sub-datasets. To achieve this, they conducted a manual inspection, removing images captured too early in the growth stage when wheat heads were not clearly visible and avoiding those acquired during head senescence to prevent overlapping. Object scale, which is crucial for object detection methods, was influenced by both the object’s size (in mm) and image resolution. While wheat head dimensions generally measure around 1.5 cm in diameter and 10 cm in length, the image resolution varied significantly across sub-datasets. To address this, images were rescaled using bilinear interpolation to maintain a more uniform resolution at the head level. To align with the requirements of deep learning algorithms, the authors of the dataset divided the original images into square patches. These patches were carefully sized at 1024 x 1024 pixels to minimize the possibility of wheat heads intersecting patch boundaries and being partially cropped. Each patch typically contained approximately 20 to 60 heads, with only a few heads extending beyond the patch boundaries. The number of patches generated from each original image ranged from 1 to 6, depending on the specific sub-dataset.
The authors applied interactive labeling to wheat heads, creating bounding boxes encompassing the entire head pixel area. Labeling posed challenges when heads were not clearly visible due to obstruction by leaves or other heads. Partially hidden heads were only labeled if at least one spikelet was visible, typically occurring in images from the early growth stage when heads did not fully emerge. Head overlap was more common in images from cameras with a wider field of view, particularly along the image borders with oblique view angles. In cases where the bounding box was too large to include the awns, it was limited to covering the head itself. Additionally, heads that were cropped at the image edges were labeled only if at least 30% of their basal part was visible. The initial labeling results underwent an independent review by two individuals unaffiliated with the sub-dataset institution. In cases where significant discrepancies between reviewers were identified, an additional round of labeling and reviewing was initiated. Approximately 20 individuals participated in this labeling process, promoting collaboration and repeated reviews to maintain a high level of accuracy and consistency across the sub-datasets.
Summary #
GWHD: Global Wheat Head Dataset 2021 v1 is a dataset for an object detection task. It is used in the agricultural industry.
The dataset consists of 4948 images with 188500 labeled objects belonging to 1 single class (wheat head).
Images in the GWHD 2021 dataset have bounding box annotations. There are 124 (3% of the total) unlabeled images (i.e. without annotations). There are 3 splits in the dataset: train (2091 images), val (1476 images), and test (1381 images). The dataset was released in 2021 by the FR-AUS-CN-USA-MEX-NW-SUD-JAP-CH-UK-BEL-CND joint research group.
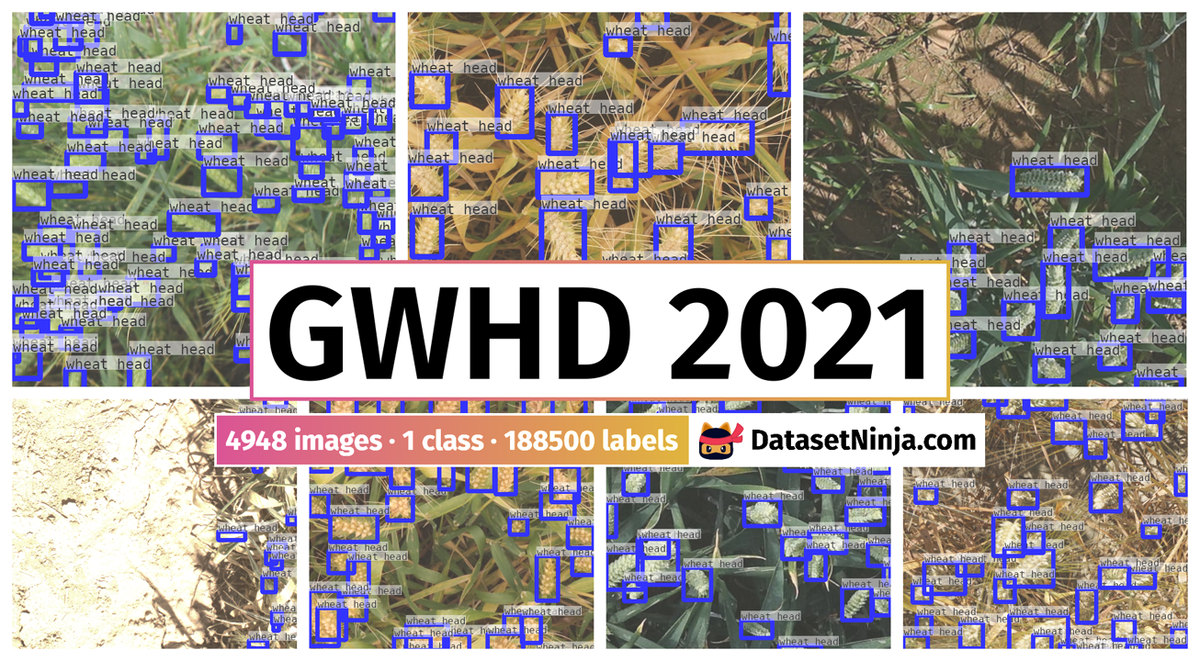
Explore #
GWHD 2021 dataset has 4948 images. Click on one of the examples below or open "Explore" tool anytime you need to view dataset images with annotations. This tool has extended visualization capabilities like zoom, translation, objects table, custom filters and more. Hover the mouse over the images to hide or show annotations.
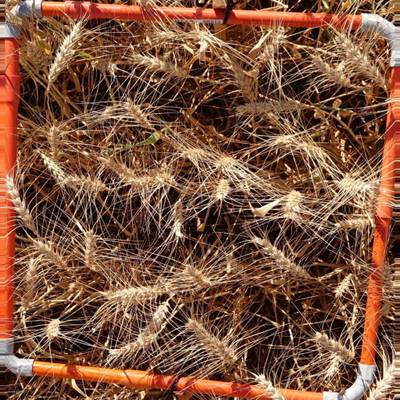

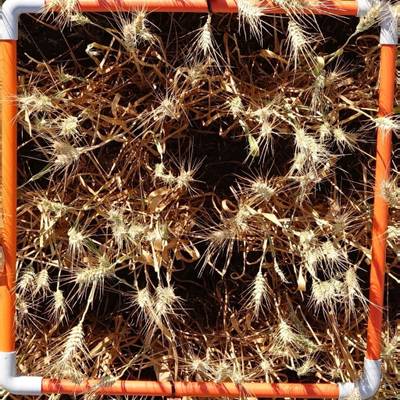
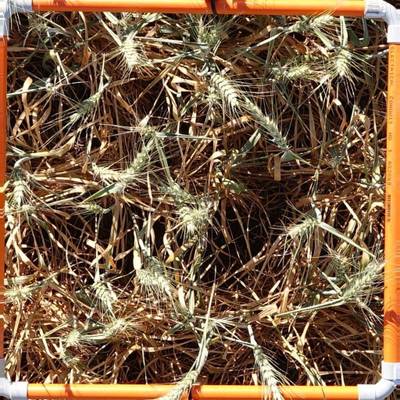
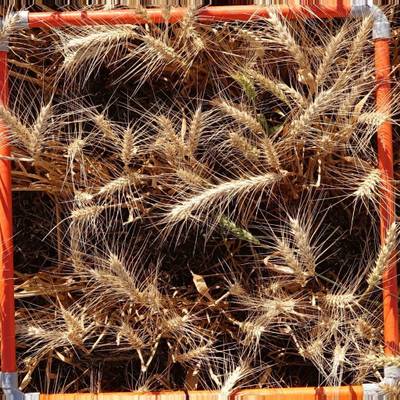
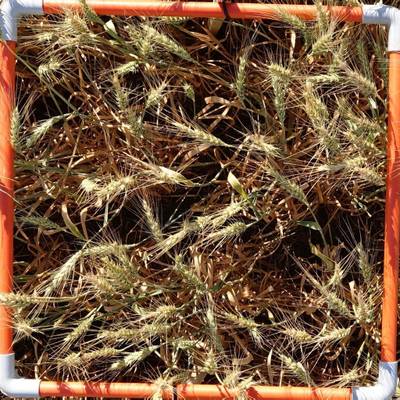
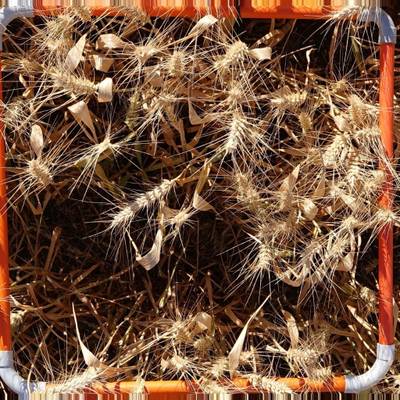
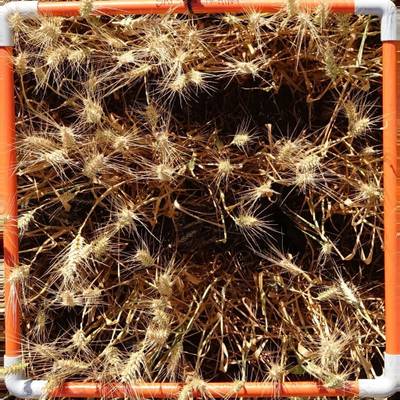

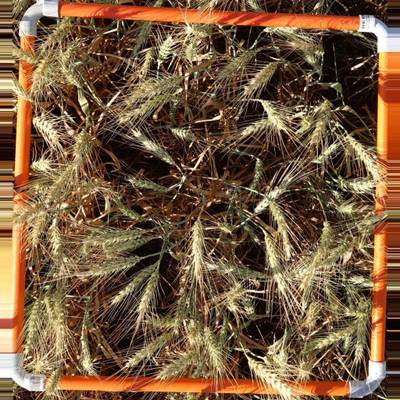
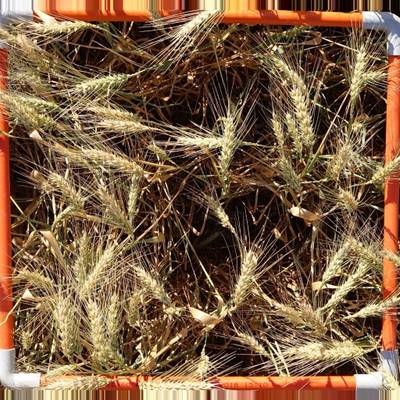
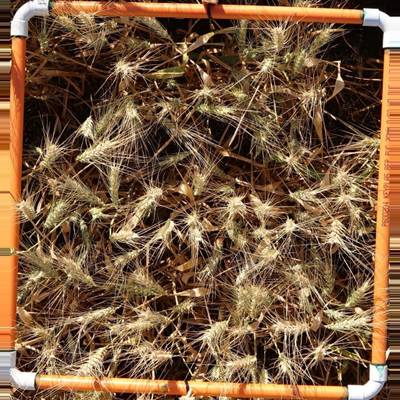
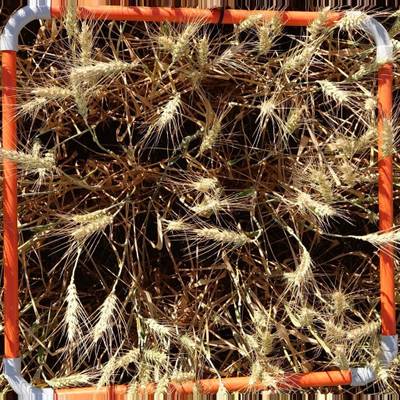
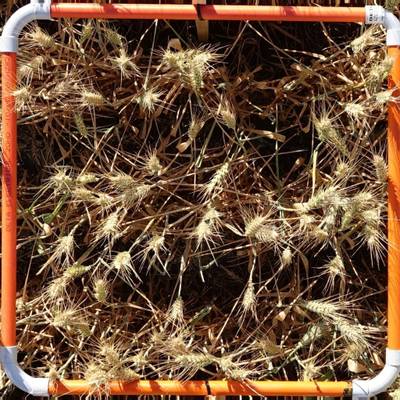
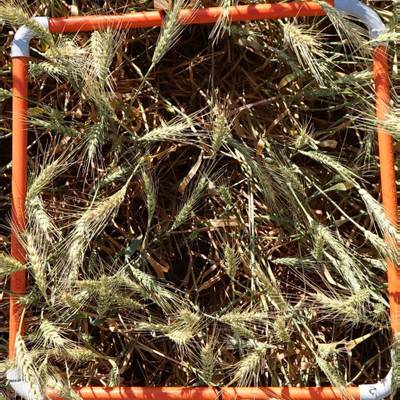
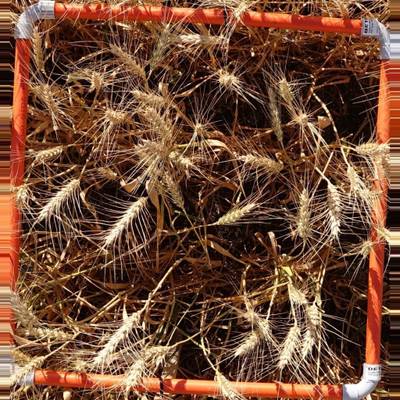
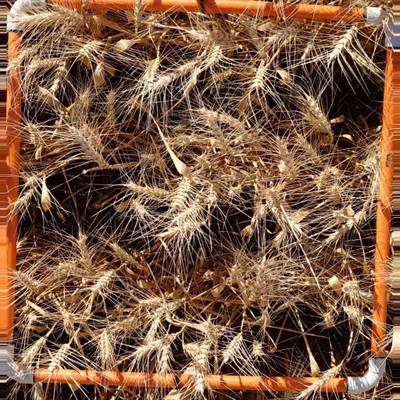
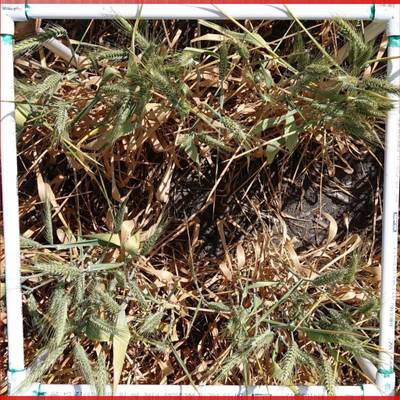
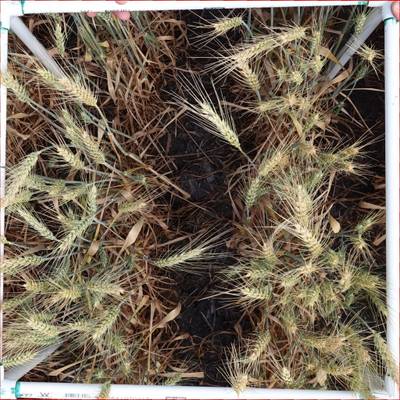

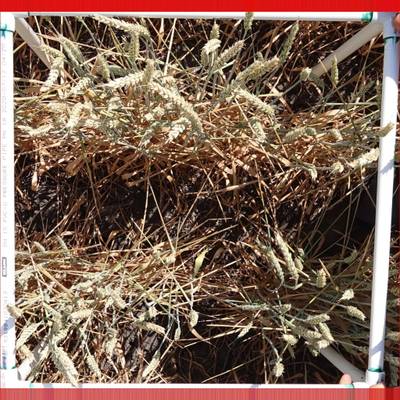
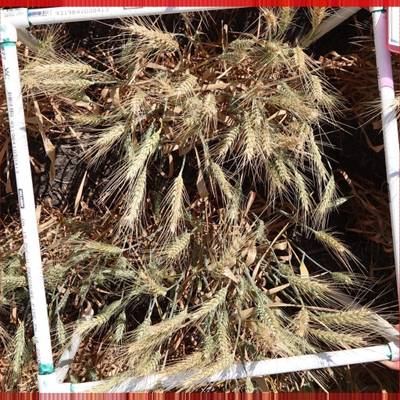
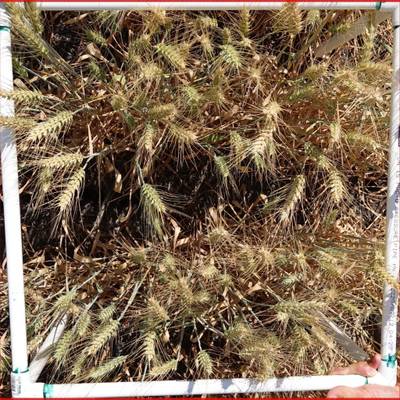
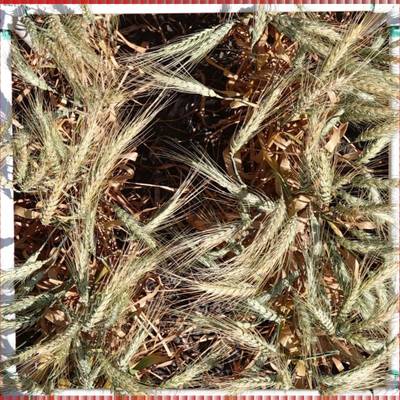
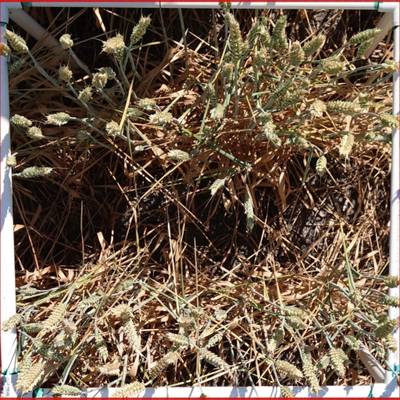
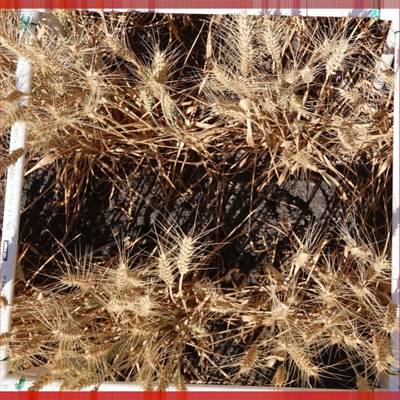
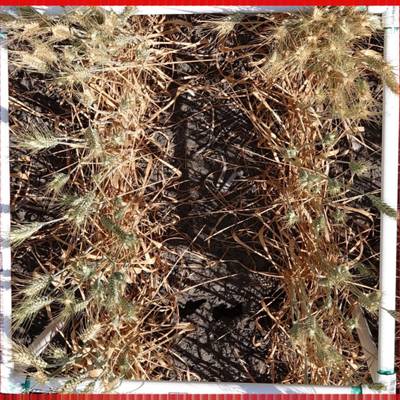
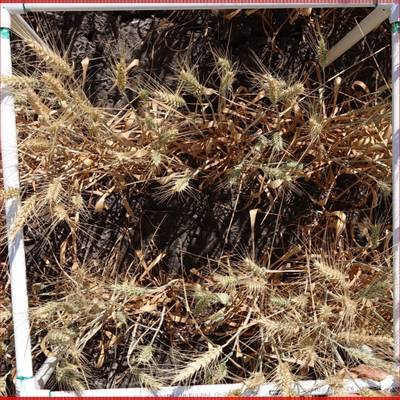
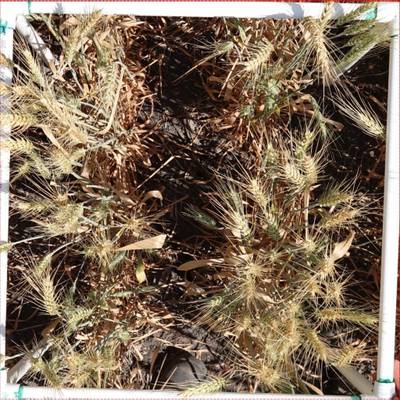
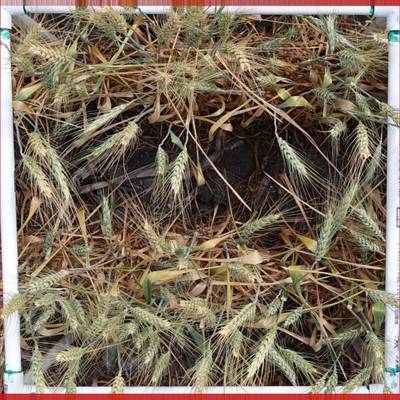
Class balance #
There are 1 annotation classes in the dataset. Find the general statistics and balances for every class in the table below. Click any row to preview images that have labels of the selected class. Sort by column to find the most rare or prevalent classes.
Class ㅤ | Images ㅤ | Objects ㅤ | Count on image average | Area on image average |
---|---|---|---|---|
wheat head➔ rectangle | 4824 | 188500 | 39.08 | 22.24% |
Images #
Explore every single image in the dataset with respect to the number of annotations of each class it has. Click a row to preview selected image. Sort by any column to find anomalies and edge cases. Use horizontal scroll if the table has many columns for a large number of classes in the dataset.
Class sizes #
The table below gives various size properties of objects for every class. Click a row to see the image with annotations of the selected class. Sort columns to find classes with the smallest or largest objects or understand the size differences between classes.
Class | Object count | Avg area | Max area | Min area | Min height | Min height | Max height | Max height | Avg height | Avg height | Min width | Min width | Max width | Max width |
---|---|---|---|---|---|---|---|---|---|---|---|---|---|---|
wheat head rectangle | 188500 | 0.62% | 37.06% | 0% | 1px | 0.1% | 611px | 59.67% | 75px | 7.29% | 2px | 0.2% | 706px | 68.95% |
Spatial Heatmap #
The heatmaps below give the spatial distributions of all objects for every class. These visualizations provide insights into the most probable and rare object locations on the image. It helps analyze objects' placements in a dataset.
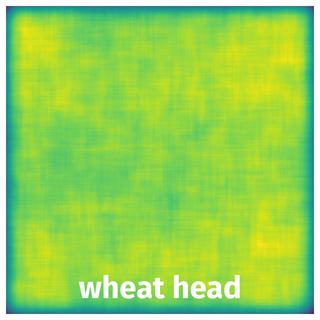
Objects #
Table contains all 97628 objects. Click a row to preview an image with annotations, and use search or pagination to navigate. Sort columns to find outliers in the dataset.
Object ID ㅤ | Class ㅤ | Image name click row to open | Image size height x width | Height ㅤ | Height ㅤ | Width ㅤ | Width ㅤ | Area ㅤ |
---|---|---|---|---|---|---|---|---|
1➔ | wheat head rectangle | 71f35a33f0ef40dd4295b8dac4fd17b902477c14daf5494474c1727c2e661381.png.jpeg | 1024 x 1024 | 53px | 5.18% | 39px | 3.81% | 0.2% |
2➔ | wheat head rectangle | 71f35a33f0ef40dd4295b8dac4fd17b902477c14daf5494474c1727c2e661381.png.jpeg | 1024 x 1024 | 124px | 12.11% | 31px | 3.03% | 0.37% |
3➔ | wheat head rectangle | 71f35a33f0ef40dd4295b8dac4fd17b902477c14daf5494474c1727c2e661381.png.jpeg | 1024 x 1024 | 131px | 12.79% | 62px | 6.05% | 0.77% |
4➔ | wheat head rectangle | 71f35a33f0ef40dd4295b8dac4fd17b902477c14daf5494474c1727c2e661381.png.jpeg | 1024 x 1024 | 241px | 23.54% | 220px | 21.48% | 5.06% |
5➔ | wheat head rectangle | 71f35a33f0ef40dd4295b8dac4fd17b902477c14daf5494474c1727c2e661381.png.jpeg | 1024 x 1024 | 171px | 16.7% | 72px | 7.03% | 1.17% |
6➔ | wheat head rectangle | 71f35a33f0ef40dd4295b8dac4fd17b902477c14daf5494474c1727c2e661381.png.jpeg | 1024 x 1024 | 138px | 13.48% | 161px | 15.72% | 2.12% |
7➔ | wheat head rectangle | 71f35a33f0ef40dd4295b8dac4fd17b902477c14daf5494474c1727c2e661381.png.jpeg | 1024 x 1024 | 82px | 8.01% | 79px | 7.71% | 0.62% |
8➔ | wheat head rectangle | 71f35a33f0ef40dd4295b8dac4fd17b902477c14daf5494474c1727c2e661381.png.jpeg | 1024 x 1024 | 90px | 8.79% | 50px | 4.88% | 0.43% |
9➔ | wheat head rectangle | 71f35a33f0ef40dd4295b8dac4fd17b902477c14daf5494474c1727c2e661381.png.jpeg | 1024 x 1024 | 141px | 13.77% | 102px | 9.96% | 1.37% |
10➔ | wheat head rectangle | 71f35a33f0ef40dd4295b8dac4fd17b902477c14daf5494474c1727c2e661381.png.jpeg | 1024 x 1024 | 67px | 6.54% | 56px | 5.47% | 0.36% |
License #
Citation #
If you make use of the GWHD 2021 data, please cite the following reference:
@article{david2020global,
title={Global Wheat Head Detection (GWHD) dataset: a large and diverse dataset of high-resolution RGB-labelled images to develop and benchmark wheat head detection methods},
author={David, Etienne and Madec, Simon and Sadeghi-Tehran, Pouria and Aasen, Helge and Zheng, Bangyou and Liu, Shouyang and Kirchgessner, Norbert and Ishikawa, Goro and Nagasawa, Koichi and Badhon, Minhajul A and others},
journal={Plant Phenomics},
volume={2020},
year={2020},
publisher={Science Partner Journal}
}
If you are happy with Dataset Ninja and use provided visualizations and tools in your work, please cite us:
@misc{ visualization-tools-for-gwhd-dataset,
title = { Visualization Tools for GWHD 2021 Dataset },
type = { Computer Vision Tools },
author = { Dataset Ninja },
howpublished = { \url{ https://datasetninja.com/gwhd } },
url = { https://datasetninja.com/gwhd },
journal = { Dataset Ninja },
publisher = { Dataset Ninja },
year = { 2024 },
month = { sep },
note = { visited on 2024-09-08 },
}
Download #
Dataset GWHD 2021 can be downloaded in Supervisely format:
As an alternative, it can be downloaded with dataset-tools package:
pip install --upgrade dataset-tools
… using following python code:
import dataset_tools as dtools
dtools.download(dataset='GWHD 2021', dst_dir='~/dataset-ninja/')
Make sure not to overlook the python code example available on the Supervisely Developer Portal. It will give you a clear idea of how to effortlessly work with the downloaded dataset.
The data in original format can be downloaded here.
Disclaimer #
Our gal from the legal dep told us we need to post this:
Dataset Ninja provides visualizations and statistics for some datasets that can be found online and can be downloaded by general audience. Dataset Ninja is not a dataset hosting platform and can only be used for informational purposes. The platform does not claim any rights for the original content, including images, videos, annotations and descriptions. Joint publishing is prohibited.
You take full responsibility when you use datasets presented at Dataset Ninja, as well as other information, including visualizations and statistics we provide. You are in charge of compliance with any dataset license and all other permissions. You are required to navigate datasets homepage and make sure that you can use it. In case of any questions, get in touch with us at hello@datasetninja.com.