Introduction #
Hacking the Human Body 2022 Dataset is a part of HuBMAP + HPA - Hacking the Human Body competition, where you need to identify and segment functional tissue units (FTUs) across five human organs - prostate, spleen, lung, kidney, and largeintestine. It helps to accelerate the world’s understanding of the relationships between cell and tissue organization. With a better idea of the relationship of cells, researchers will have more insight into the function of cells that impact human health.
This competition uses data from two different consortia, the Human Protein Atlas (HPA) and Human BioMolecular Atlas Program (HuBMAP). The training dataset consists of data from public HPA data, the public test set is a combination of private HPA data and HuBMAP data, and the private test set contains only HuBMAP data. Adapting models to function properly when presented with data that was prepared using a different protocol will be one of the core challenges of this competition. While this is expected to make the problem more difficult, developing models that generalize is a key goal of this endeavor. This competition uses a hidden test.
Dataset includes metadata for the train/test set. Only the first few rows of the test set are available for download:
- id - The image ID.
- organ - The organ that the biopsy sample was taken from.
- data_source - Whether the image was provided by HuBMAP or HPA.
- img_height - The height of the image in pixels.
- img_width - The width of the image in pixels.
- pixel_size - The height/width of a single pixel from this image in micrometers. All HPA images have a pixel size of 0.4 µm. For HuBMAP imagery the pixel size is 0.5 µm for kidney, 0.2290 µm for large intestine, 0.7562 µm for lung, 0.4945 µm for spleen, and 6.263 µm for prostate.
- tissue_thickness - The thickness of the biopsy sample in micrometers. All HPA images have a thickness of 4 µm. The HuBMAP samples have tissue slice thicknesses 10 µm for kidney, 8 µm for large intestine, 4 µm for spleen, 5 µm for lung, and 5 µm for prostate.
- rle - The target column. A run length encoded copy of the annotations. Provided for the training set only.
- age - The patient’s age in years. Provided for the training set only.
- sex - The sex of the patient. Provided for the training set only.
Summary #
Hacking the Human Body 2022 is a dataset for a semantic segmentation task. It is used in the biological research. Possible applications of the dataset could be in the medical industry.
The dataset consists of 352 images with 351 labeled objects belonging to 5 different classes including kidney, prostate, largeintestine, and other: spleen and lung.
Images in the Hacking the Human Body 2022 dataset have pixel-level semantic segmentation annotations. There is 1 unlabeled image (i.e. without annotations). There are 2 splits in the dataset: train (351 images) and test (1 images). Also, the dataset includes age, sex, tissue_thickness for train split, organ and tissue_thickness for test split. The dataset was released in 2022.
Here are the visualized examples for the classes:
Explore #
Hacking the Human Body 2022 dataset has 352 images. Click on one of the examples below or open "Explore" tool anytime you need to view dataset images with annotations. This tool has extended visualization capabilities like zoom, translation, objects table, custom filters and more. Hover the mouse over the images to hide or show annotations.

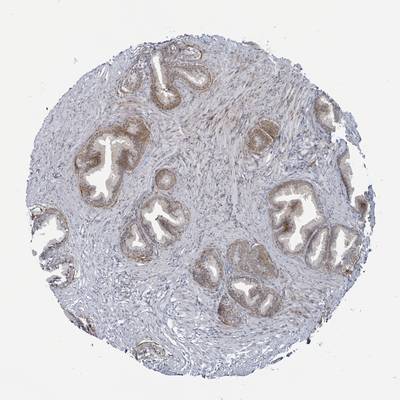




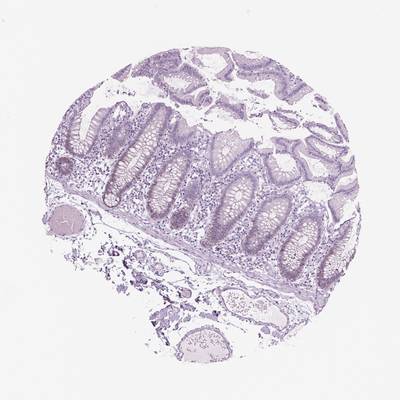
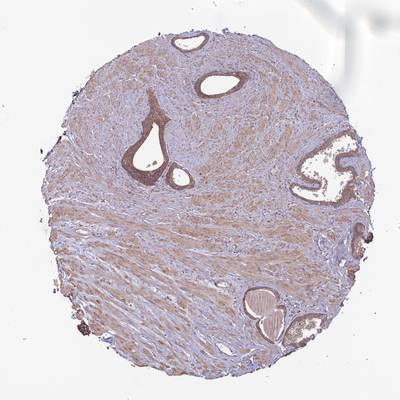
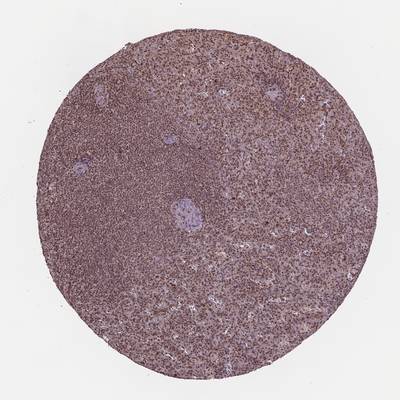
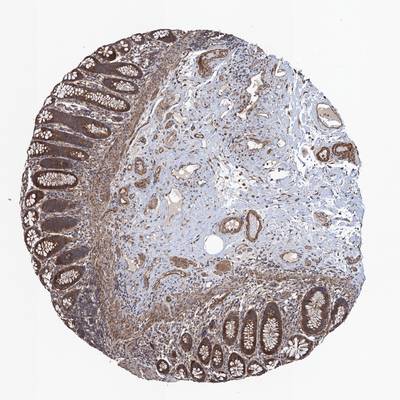
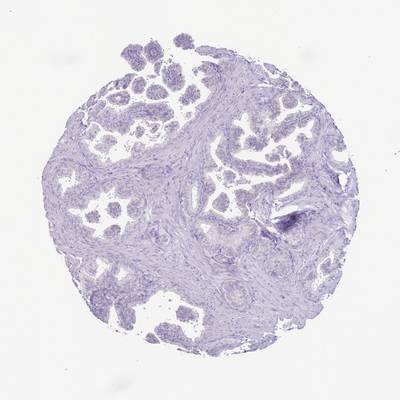
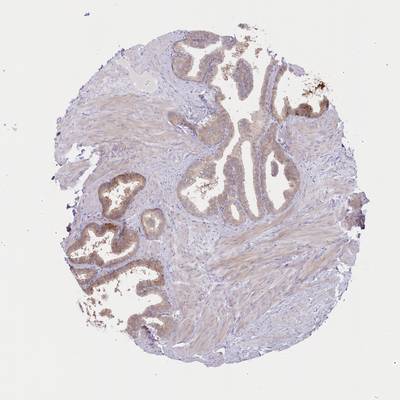
Class balance #
There are 5 annotation classes in the dataset. Find the general statistics and balances for every class in the table below. Click any row to preview images that have labels of the selected class. Sort by column to find the most rare or prevalent classes.
Class ㅤ | Images ㅤ | Objects ㅤ | Count on image average | Area on image average |
---|---|---|---|---|
kidney➔ mask | 99 | 99 | 1 | 3.02% |
prostate➔ mask | 93 | 93 | 1 | 15.4% |
largeintestine➔ mask | 58 | 58 | 1 | 19.34% |
spleen➔ mask | 53 | 53 | 1 | 10.1% |
lung➔ mask | 48 | 48 | 1 | 2.08% |
Co-occurrence matrix #
Co-occurrence matrix is an extremely valuable tool that shows you the images for every pair of classes: how many images have objects of both classes at the same time. If you click any cell, you will see those images. We added the tooltip with an explanation for every cell for your convenience, just hover the mouse over a cell to preview the description.
Images #
Explore every single image in the dataset with respect to the number of annotations of each class it has. Click a row to preview selected image. Sort by any column to find anomalies and edge cases. Use horizontal scroll if the table has many columns for a large number of classes in the dataset.
Object distribution #
Interactive heatmap chart for every class with object distribution shows how many images are in the dataset with a certain number of objects of a specific class. Users can click cell and see the list of all corresponding images.
Class sizes #
The table below gives various size properties of objects for every class. Click a row to see the image with annotations of the selected class. Sort columns to find classes with the smallest or largest objects or understand the size differences between classes.
Class | Object count | Avg area | Max area | Min area | Min height | Min height | Max height | Max height | Avg height | Avg height | Min width | Min width | Max width | Max width |
---|---|---|---|---|---|---|---|---|---|---|---|---|---|---|
kidney mask | 99 | 3.02% | 7.95% | 0.31% | 199px | 6.63% | 2725px | 92.89% | 1436px | 48.56% | 198px | 6.6% | 2796px | 93.2% |
prostate mask | 93 | 15.4% | 42.93% | 0.99% | 487px | 16.23% | 2583px | 96.38% | 1948px | 65.72% | 424px | 14.13% | 2703px | 96.52% |
largeintestine mask | 58 | 19.34% | 34.83% | 2.11% | 558px | 18.6% | 2817px | 93.9% | 2321px | 77.38% | 814px | 27.13% | 2799px | 93.3% |
spleen mask | 53 | 10.1% | 34.33% | 0.81% | 502px | 16.73% | 2792px | 93.07% | 1816px | 60.9% | 397px | 13.23% | 2660px | 90.68% |
lung mask | 48 | 2.08% | 8.89% | 0.07% | 92px | 3.07% | 2489px | 82.97% | 1177px | 39.29% | 93px | 3.1% | 2201px | 73.37% |
Spatial Heatmap #
The heatmaps below give the spatial distributions of all objects for every class. These visualizations provide insights into the most probable and rare object locations on the image. It helps analyze objects' placements in a dataset.
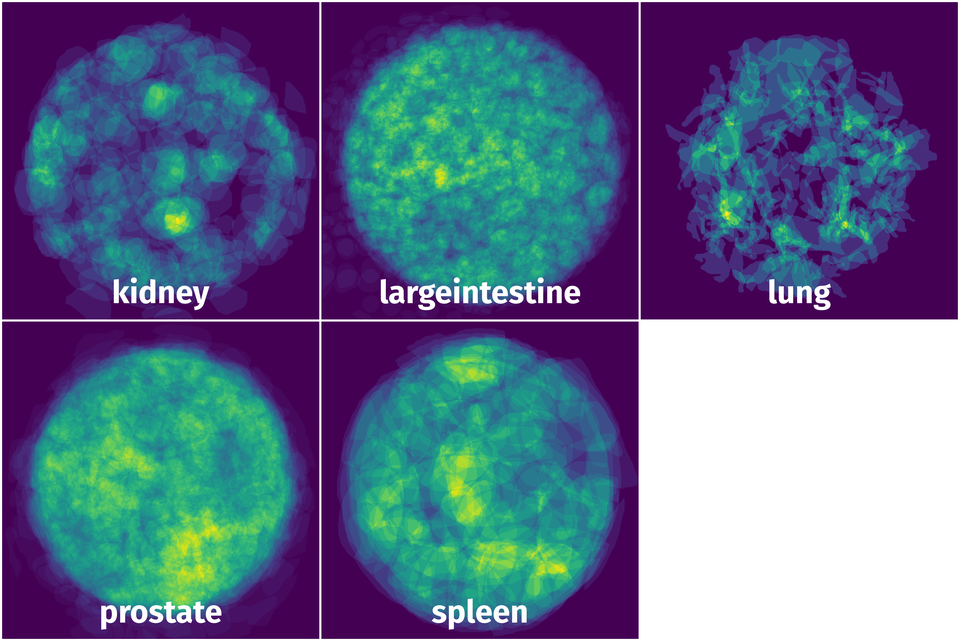
Objects #
Table contains all 351 objects. Click a row to preview an image with annotations, and use search or pagination to navigate. Sort columns to find outliers in the dataset.
Object ID ㅤ | Class ㅤ | Image name click row to open | Image size height x width | Height ㅤ | Height ㅤ | Width ㅤ | Width ㅤ | Area ㅤ |
---|---|---|---|---|---|---|---|---|
1➔ | prostate mask | 435.png | 3000 x 3000 | 2316px | 77.2% | 2400px | 80% | 19.62% |
2➔ | kidney mask | 29238.png | 3000 x 3000 | 245px | 8.17% | 265px | 8.83% | 0.55% |
3➔ | kidney mask | 26174.png | 3000 x 3000 | 199px | 6.63% | 338px | 11.27% | 0.56% |
4➔ | prostate mask | 17143.png | 3000 x 3000 | 2557px | 85.23% | 1837px | 61.23% | 17.53% |
5➔ | largeintestine mask | 31406.png | 3000 x 3000 | 2608px | 86.93% | 2450px | 81.67% | 24.66% |
6➔ | lung mask | 23252.png | 3000 x 3000 | 1542px | 51.4% | 1652px | 55.07% | 4.21% |
7➔ | lung mask | 4412.png | 3000 x 3000 | 663px | 22.1% | 1216px | 40.53% | 2.59% |
8➔ | spleen mask | 8894.png | 3000 x 3000 | 2395px | 79.83% | 2417px | 80.57% | 25.92% |
9➔ | kidney mask | 31709.png | 3000 x 3000 | 2620px | 87.33% | 1427px | 47.57% | 5.92% |
10➔ | prostate mask | 4802.png | 3000 x 3000 | 1178px | 39.27% | 1393px | 46.43% | 5.25% |
License #
License is unknown for the Hacking the Human Body 2022 dataset.
Citation #
If you make use of the Hacking the Human Body 2022 data, please cite the following reference:
@misc{hubmap-organ-segmentation,
author = {Addison Howard, AlmostSohier, Cecilia Lindskog, Emma Lundberg, Katy Borner, Leah Godwin, Shriya, Sohier Dane, Trang Le, Yashvardhan Jain},
title = {HuBMAP + HPA - Hacking the Human Body},
publisher = {Kaggle},
year = {2022},
url = {https://kaggle.com/competitions/hubmap-organ-segmentation}
}
If you are happy with Dataset Ninja and use provided visualizations and tools in your work, please cite us:
@misc{ visualization-tools-for-hacking-the-human-body-dataset,
title = { Visualization Tools for Hacking the Human Body 2022 Dataset },
type = { Computer Vision Tools },
author = { Dataset Ninja },
howpublished = { \url{ https://datasetninja.com/hacking-the-human-body-2022 } },
url = { https://datasetninja.com/hacking-the-human-body-2022 },
journal = { Dataset Ninja },
publisher = { Dataset Ninja },
year = { 2025 },
month = { aug },
note = { visited on 2025-08-08 },
}
Download #
Please visit dataset homepage to download the data.
Disclaimer #
Our gal from the legal dep told us we need to post this:
Dataset Ninja provides visualizations and statistics for some datasets that can be found online and can be downloaded by general audience. Dataset Ninja is not a dataset hosting platform and can only be used for informational purposes. The platform does not claim any rights for the original content, including images, videos, annotations and descriptions. Joint publishing is prohibited.
You take full responsibility when you use datasets presented at Dataset Ninja, as well as other information, including visualizations and statistics we provide. You are in charge of compliance with any dataset license and all other permissions. You are required to navigate datasets homepage and make sure that you can use it. In case of any questions, get in touch with us at hello@datasetninja.com.