Introduction #
The authors’ research addresses the challenging task of detecting and localizing human hands in still images, with a two-stage framework and a curated Hand dataset containing 9,163 train, 1,856 val, and 2,031 test hand instances, along with a subset of “bigger hand instances” for evaluation. The goal is to facilitate human visual recognition, including sign language and gesture interpretation, and temporal analysis.
The objective of this work is to detect and localize human hands in still images. This is a tremendously challenging task as hands can be very varied in shape and viewpoint, can be closed or open, can be partially occluded, can have different articulations of the fingers, can be grasping other objects or other hands, etc. The authors’ motivation for this is that having a reliable hand detector facilitates many other tasks in human visual recognition, such as determining human layout and actions from static images. It also benefits human temporal analysis, such as recognizing sign language, gestures and activities in video.
In their work, authors propose a detector using a two-stage hypothesize and classify framework. First, hand hypotheses are proposed from three independent methods: a sliding window hand-shape detector, a sliding window context-based detector, and a skin-based detector. The sliding window detectors employ the Felzenszwalb et al. Object detection with discriminatively trained part’s partly based deformable model with three components. Then, the proposals are scored by all three methods and a discriminatively trained model is used to verify them. The three proposal mechanisms ensure good recall, and the discriminative classification ensures good precision. In addition, authors develop a new method of non-maximum suppression based on super-pixels. Figure below overviews the detector.

Overview of the method. First row - Original image. Second - some of the hypotheses proposed by hand and context detector. Bounding boxes in ‘Red’ are proposed by the hand detector and ‘Green’ bounding boxes are proposed by the context detector. Third row - skin detection and hypotheses generation. Next row - Super-pixel segmentation of the image with combined hypothesized bounding boxes from the three proposal schemes. Using super-pixel-based non-maximum suppression (NMS), overlapping bounding boxes are suppressed. Last row - final detection after post-processing.
Authors have collected a comprehensive dataset of hand images from various public image sources. In each image, all the hands that can be perceived clearly by humans are annotated. The annotations consist of a bounding rectangle, which does not have to be axis aligned, or oriented with respect to the wrist.
train Set | val Set | test Set | |
---|---|---|---|
Hand Instances | 9163 | 1856 | 2031 |
Bigger Hand Instances | 2861 | 649 | 660 |
Statistics of hand dataset. A hand instance is ‘big’ if area of the bounding box is greater than 1500 sq. pixels. Bigger hand instances are used for experimental evaluations.
Summary #
Hand is a dataset for an object detection task. It is used in the entertainment industry.
The dataset consists of 5628 images with 13050 labeled objects belonging to 1 single class (hand).
Images in the Hand dataset have bounding box annotations. All images are labeled (i.e. with annotations). There are 3 splits in the dataset: train (4069 images), test (821 images), and val (738 images). Also, the objects have right and left tags. Explore them in the supervisely labeling tool. The dataset was released in 2011 by the University of Oxford and Oxford Brookes University, UK.
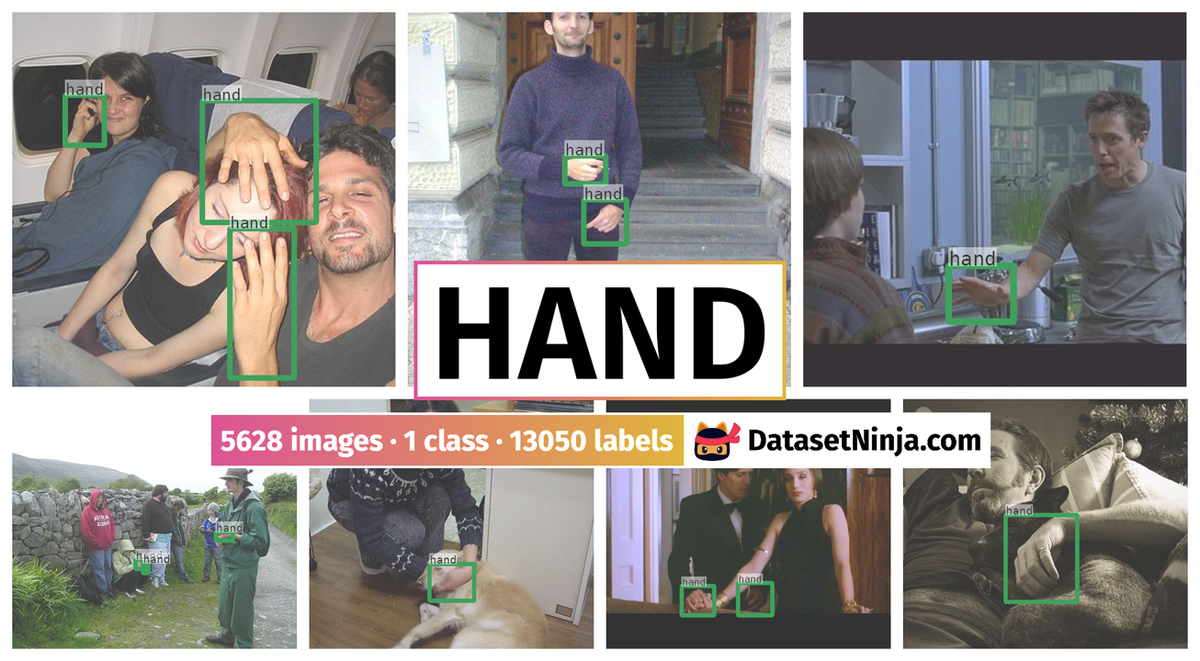
Explore #
Hand dataset has 5628 images. Click on one of the examples below or open "Explore" tool anytime you need to view dataset images with annotations. This tool has extended visualization capabilities like zoom, translation, objects table, custom filters and more. Hover the mouse over the images to hide or show annotations.
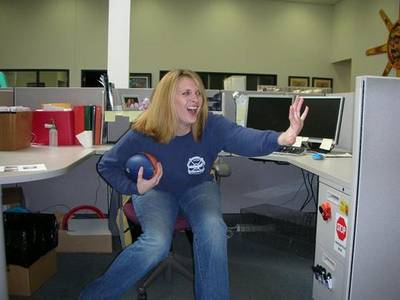
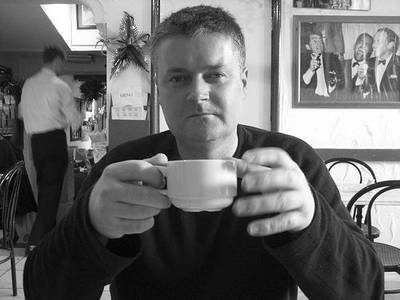
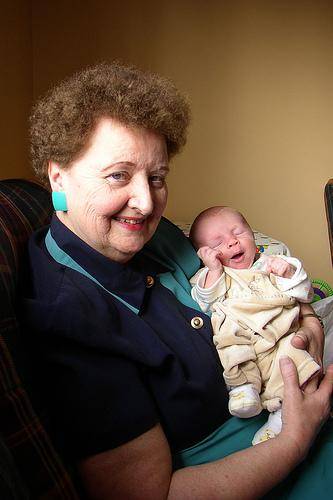
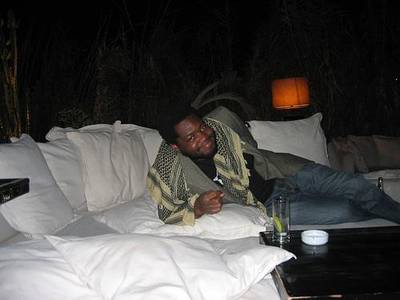
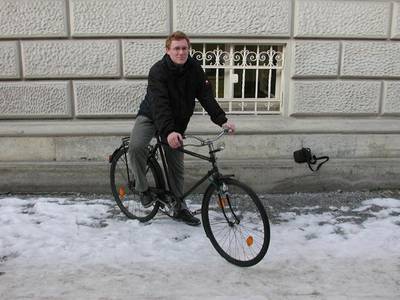
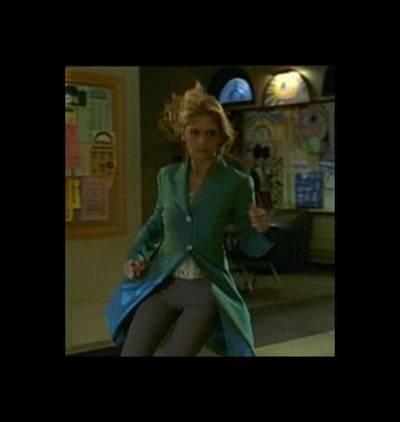

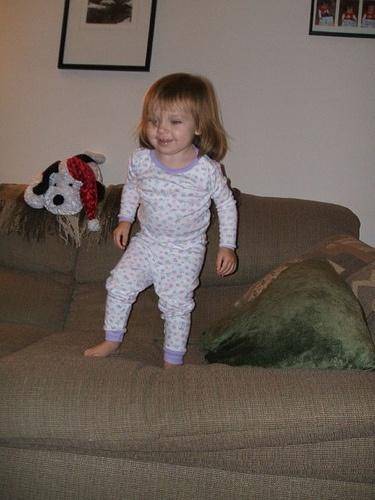

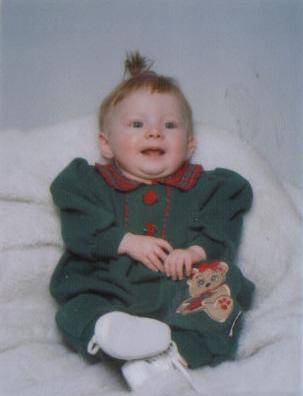
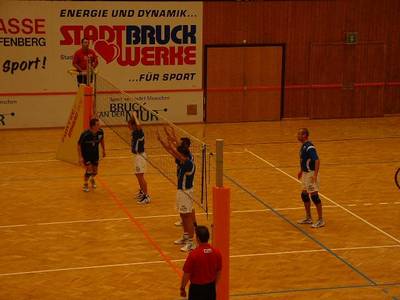
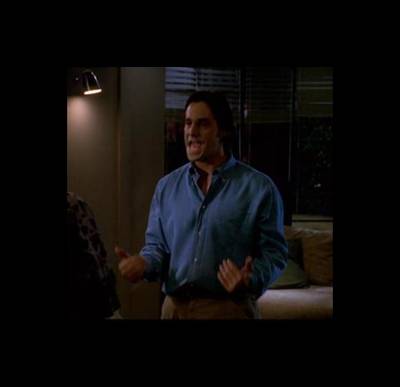
Class balance #
There are 1 annotation classes in the dataset. Find the general statistics and balances for every class in the table below. Click any row to preview images that have labels of the selected class. Sort by column to find the most rare or prevalent classes.
Class ㅤ | Images ㅤ | Objects ㅤ | Count on image average | Area on image average |
---|---|---|---|---|
hand➔ polygon | 5628 | 13050 | 2.32 | 1.59% |
Images #
Explore every single image in the dataset with respect to the number of annotations of each class it has. Click a row to preview selected image. Sort by any column to find anomalies and edge cases. Use horizontal scroll if the table has many columns for a large number of classes in the dataset.
Object distribution #
Interactive heatmap chart for every class with object distribution shows how many images are in the dataset with a certain number of objects of a specific class. Users can click cell and see the list of all corresponding images.
Class sizes #
The table below gives various size properties of objects for every class. Click a row to see the image with annotations of the selected class. Sort columns to find classes with the smallest or largest objects or understand the size differences between classes.
Class | Object count | Avg area | Max area | Min area | Min height | Min height | Max height | Max height | Avg height | Avg height | Min width | Min width | Max width | Max width |
---|---|---|---|---|---|---|---|---|---|---|---|---|---|---|
hand polygon | 13050 | 0.67% | 26.89% | 0.01% | 8px | 1.46% | 315px | 75.6% | 46px | 10.2% | 6px | 0.7% | 312px | 68.53% |
Spatial Heatmap #
The heatmaps below give the spatial distributions of all objects for every class. These visualizations provide insights into the most probable and rare object locations on the image. It helps analyze objects' placements in a dataset.
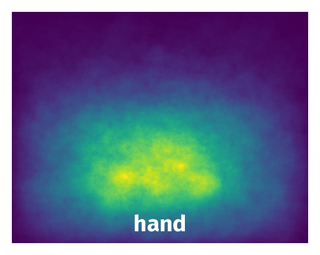
Objects #
Table contains all 13050 objects. Click a row to preview an image with annotations, and use search or pagination to navigate. Sort columns to find outliers in the dataset.
Object ID ㅤ | Class ㅤ | Image name click row to open | Image size height x width | Height ㅤ | Height ㅤ | Width ㅤ | Width ㅤ | Area ㅤ |
---|---|---|---|---|---|---|---|---|
1➔ | hand polygon | Buffy_448.jpg | 639 x 619 | 46px | 7.2% | 47px | 7.59% | 0.26% |
2➔ | hand polygon | Buffy_448.jpg | 639 x 619 | 34px | 5.32% | 48px | 7.75% | 0.34% |
3➔ | hand polygon | Skin_208.jpg | 504 x 756 | 38px | 7.54% | 38px | 5.03% | 0.27% |
4➔ | hand polygon | Skin_208.jpg | 504 x 756 | 28px | 5.56% | 72px | 9.52% | 0.5% |
5➔ | hand polygon | Skin_208.jpg | 504 x 756 | 33px | 6.55% | 34px | 4.5% | 0.18% |
6➔ | hand polygon | VOC2007_819.jpg | 332 x 500 | 73px | 21.99% | 75px | 15% | 1.62% |
7➔ | hand polygon | VOC2010_1113.jpg | 460 x 460 | 68px | 14.78% | 61px | 13.26% | 0.81% |
8➔ | hand polygon | Skin_204.jpg | 300 x 200 | 50px | 16.67% | 40px | 20% | 1.62% |
9➔ | hand polygon | Buffy_527.jpg | 577 x 577 | 38px | 6.59% | 53px | 9.19% | 0.51% |
10➔ | hand polygon | Buffy_527.jpg | 577 x 577 | 49px | 8.49% | 46px | 7.97% | 0.41% |
License #
You must not use the content if you do not agree to these terms.
What does content mean?
We mean the images and videos which are curated by the Visual Geometry Group (VGG), and which we are making available to you to download from a link provided by the University hosting and managing the content (or in any other way we decide). We do not guarantee that the link or content will always be available, or that it is accurate, free of viruses and bugs, or the information in it can be relied upon. You use the content entirely at your own risk.
What can I use it for?
Provided you keep to these rules, the University grants you (the researcher) a non-exclusive and non-transferable licence to use the content free of charge strictly for non-commercial research (i.e., whose output artefacts are not incorporated in commercial products) for 12 months. You are responsible for your own costs in using the content. You must delete the content when this permission ends. You may download the content again, and renew the licence, if it is still available.
You must not:
- use the content other than for the Permitted Purpose in strict conformity with these terms and any other reasonable instructions of the University.
- except as may be strictly necessary for carrying out the Permitted Purpose, provide or otherwise make available content to any third party or allow use of it or them by or on behalf of any third party, in whole or in part, whether by way of sale, resale, loan, transfer, hire or any other form of exploitation; or
- attempt to identify any living or deceased individual from the content.
You shall:
- comply with all applicable laws and regulations in relation to the use of the content, including without limitation the Data Protection Act 2018 and the UK GDPR; and
- not participate or encourage the participation in any illegal, deceptive, misleading or unethical practice, including, but without limitation, disparagement of the content or any other practices which may be detrimental to the same or the University.
You shall notify the University promptly of:
- any inaccuracies, errors or malfunctions in the content which come (or should reasonably come) to its attention.
- any unlicensed or other unauthorised use of the whole or any part of any of the content which comes to (or should reasonably come to) your attention; and
- any allegation by any person that the use of the whole or any part of any of our site infringes the rights of any third party.
Citation #
If you make use of the Hand data, please cite the following reference:
@inproceedings{Mittal2011HandDU,
title={Hand detection using multiple proposals},
author={Arpit Mittal and Andrew Zisserman and Philip H. S. Torr},
booktitle={British Machine Vision Conference},
year={2011},
url={https://api.semanticscholar.org/CorpusID:8162487}
}
If you are happy with Dataset Ninja and use provided visualizations and tools in your work, please cite us:
@misc{ visualization-tools-for-hand-dataset,
title = { Visualization Tools for Hand Dataset },
type = { Computer Vision Tools },
author = { Dataset Ninja },
howpublished = { \url{ https://datasetninja.com/hand } },
url = { https://datasetninja.com/hand },
journal = { Dataset Ninja },
publisher = { Dataset Ninja },
year = { 2024 },
month = { jul },
note = { visited on 2024-07-27 },
}
Download #
Please visit dataset homepage to download the data.
Disclaimer #
Our gal from the legal dep told us we need to post this:
Dataset Ninja provides visualizations and statistics for some datasets that can be found online and can be downloaded by general audience. Dataset Ninja is not a dataset hosting platform and can only be used for informational purposes. The platform does not claim any rights for the original content, including images, videos, annotations and descriptions. Joint publishing is prohibited.
You take full responsibility when you use datasets presented at Dataset Ninja, as well as other information, including visualizations and statistics we provide. You are in charge of compliance with any dataset license and all other permissions. You are required to navigate datasets homepage and make sure that you can use it. In case of any questions, get in touch with us at hello@datasetninja.com.