Introduction #
The HIT-UAV: A High-Altitude Infrared Thermal Dataset for Unmanned Aerial Vehicle-Based Object Detection dataset consists of 2,898 infrared thermal images. These images were extracted from a larger pool of 43,470 frames sourced from numerous videos, all of which were publicly available and had undergone desensitization for privacy reasons. In order to enhance the dataset’s utility for various tasks, the HIT-UAV10 dataset includes two types of annotated bounding boxes for each object within the images: oriented bounding boxes, designed to address the challenge of significant overlap between object instances in aerial images, and standard bounding boxes, aimed at facilitating efficient dataset utilization. This comprehensive dataset encompasses five distinct object categories: person, car, bicycle, other vehicle, and dontcare, totaling 24,899 annotated objects. The DontCare category encompasses objects that proved difficult for annotators to categorize accurately, with additional details provided in the Methods section.
The dataset is composed of 2,029 training images, 579 test images, and 290 validation images. To evaluate the HIT-UAV10 dataset, the authors conducted training and testing using established object detection algorithms, specifically YOLOv422, YOLOv4-tiny, Faster R-CNN23, and SSD24. The results demonstrated that these algorithms exhibited outstanding performance on the HIT-UAV10 dataset in comparison to other visual light datasets. This suggests the significant potential of infrared thermal datasets in enhancing object detection applications in Unmanned Aerial Vehicles (UAVs). Furthermore, the authors conducted a performance analysis of YOLOv4 and YOLOv4-tiny at various altitudes and camera perspectives, yielding valuable insights to assist users in comprehending UAV-based object detection.
The authors opted for the DJI Matrice M210 V2 UAV platform for image acquisition, which comes with an approximate price tag of 10,000 US dollars. The configuration of the DJI Matrice M210 V2 used is elaborated upon in Table 3. To capture the images, the authors equipped the UAV with the DJI Zenmuse XT2 camera26. This camera boasts a FLIR longwave infrared thermal camera with a thermal infrared resolution of 640 × 512 pixels and a 25 mm lens. Additionally, it includes a visual camera capable of capturing 4K videos and 12MP photos. The cost associated with the DJI Zenmuse XT2 camera is approximately 8,000 US dollars.
The dataset generation pipeline consists of four stages: video capture, frame extraction and data cleaning, object annotation, and dataset generation.
Videos were recorded under diverse conditions, encompassing locations such as schools, parking lots, roads, playgrounds, and others. The flight altitude spanned from 60 to 130 meters, and the camera perspective ranged from 30 to 90 degrees. Flights were conducted during both daylight and nighttime settings. For each video, essential information, including flight altitude, camera perspective, flight date, and daylight intensity, was meticulously recorded.
The sample images were captured from a distance of 80 meters, with different camera angles. When the camera was set at a 30-degree angle, distant objects seemed smaller due to the broader field of view. Conversely, when the camera angle was increased to 50 degrees, objects appeared larger. However, when the camera angle reached 90 degrees, objects once again appeared smaller, this time due to the reduced visible surface area of the objects.
The image files are systematically named following this format: T_HH(H)_AA_W_NNNNN. Here, T signifies the shooting time (0 for day and 1 for night), HH(H) indicates the flight altitude (which varies from 60 to 130 meters), AA represents the camera perspective (ranging from 30 to 90 degrees), W denotes the prevailing weather condition (with only images taken under non-rainy conditions being included in the dataset), and NNNNN signifies the unique serial number assigned to each image. Each of those parameters were added as an image tag.
Summary #
HIT-UAV: A High-Altitude Infrared Thermal Dataset for Unmanned Aerial Vehicle-Based Object Detection is a dataset for an object detection task. It is used in the drone inspection domain.
The dataset consists of 2898 images with 24899 labeled objects belonging to 5 different classes including person, car, bicycle, and other: other vehicle and dontcare.
Images in the HIT-UAV dataset have bounding box annotations. There are 32 (1% of the total) unlabeled images (i.e. without annotations). There are 3 splits in the dataset: train (2029 images), test (579 images), and val (290 images). The dataset was released in 2023 by the Pegasus Project, China.
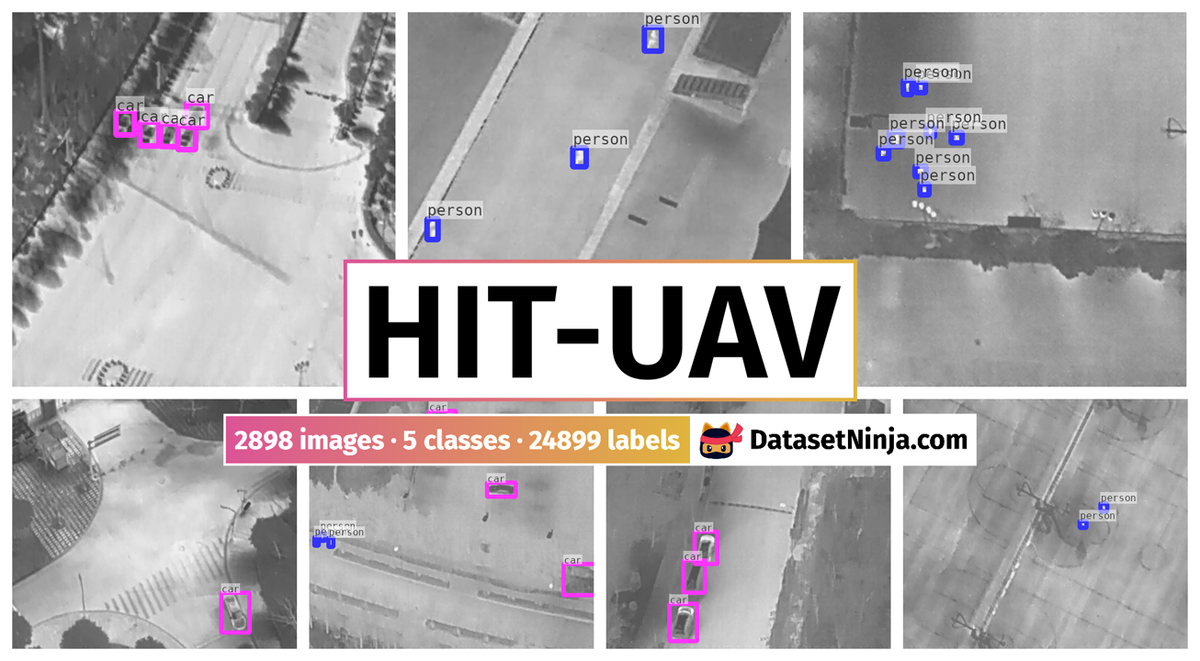
Explore #
HIT-UAV dataset has 2898 images. Click on one of the examples below or open "Explore" tool anytime you need to view dataset images with annotations. This tool has extended visualization capabilities like zoom, translation, objects table, custom filters and more. Hover the mouse over the images to hide or show annotations.
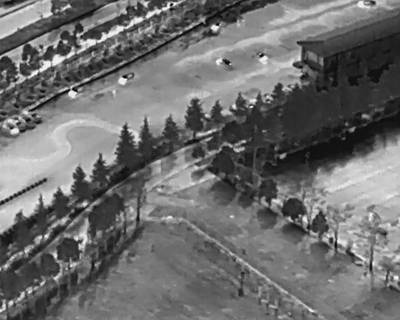
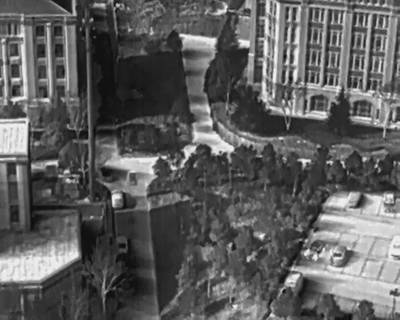
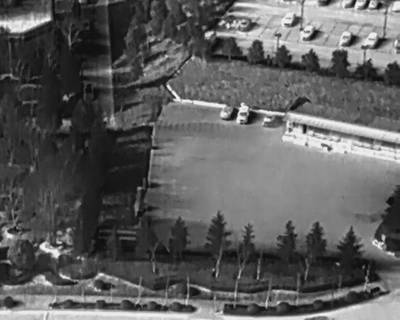
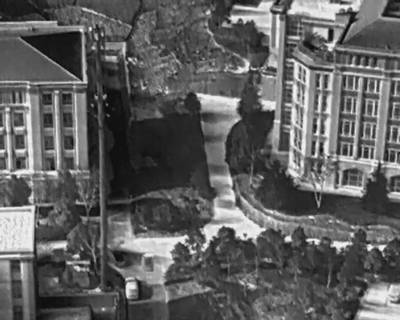
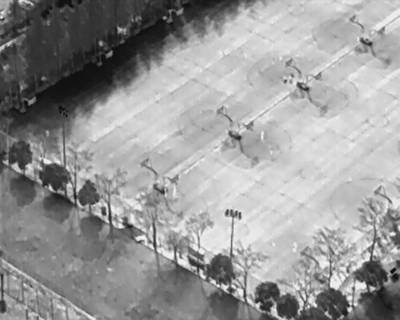
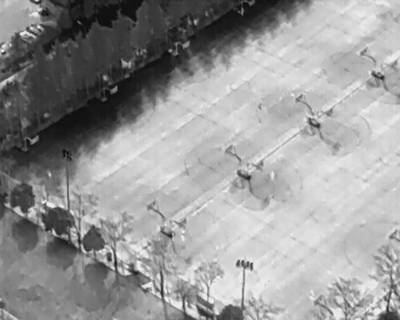
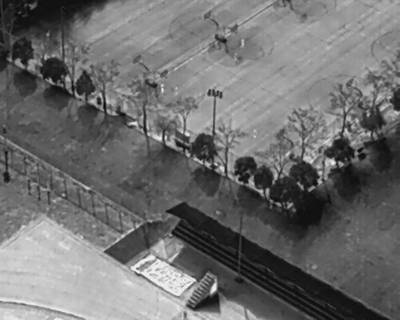
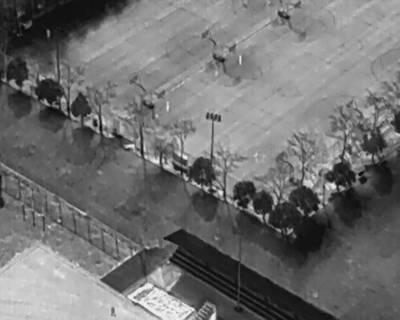
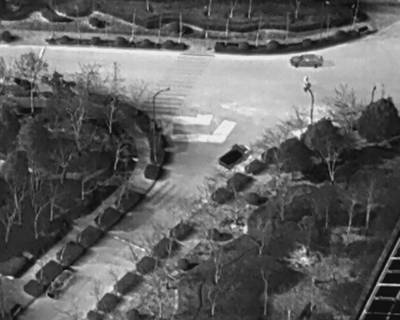
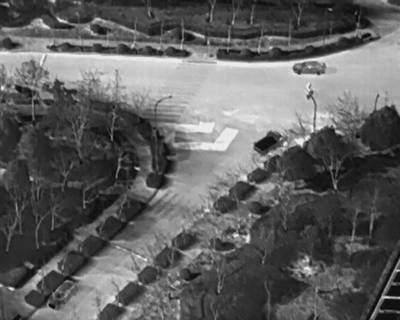
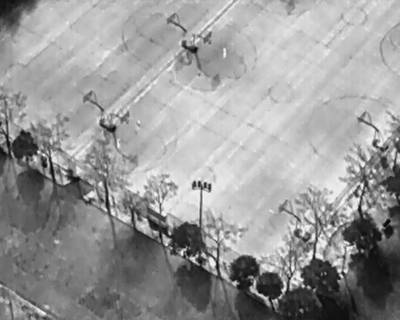
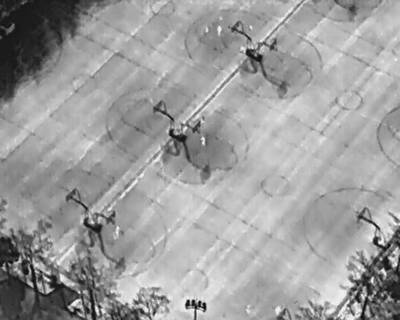
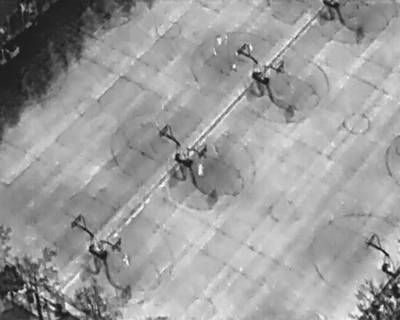
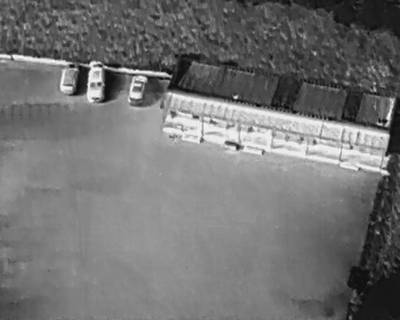
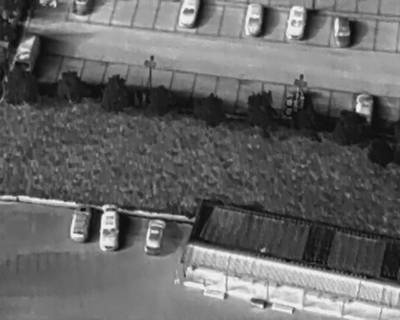
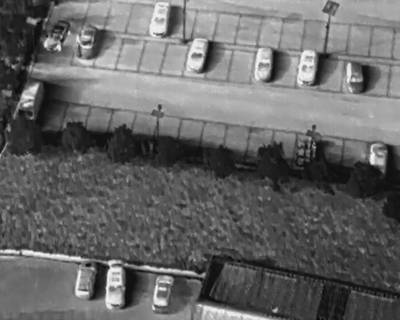

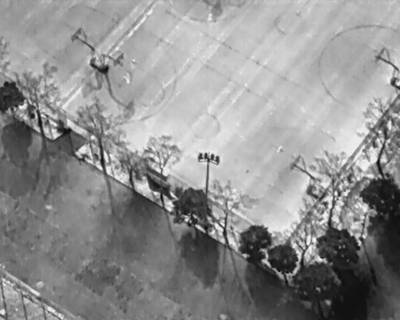
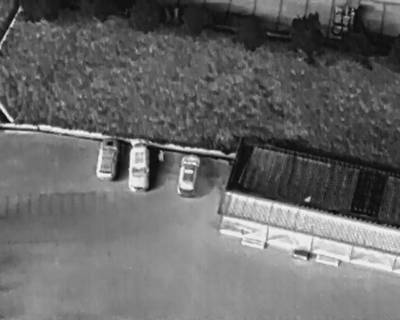
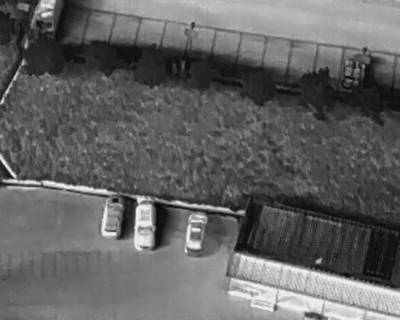
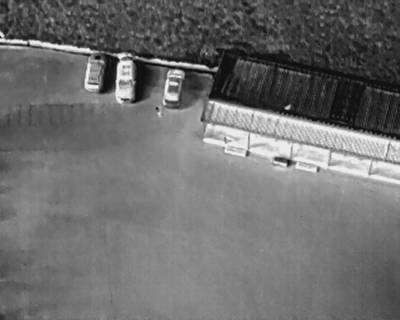
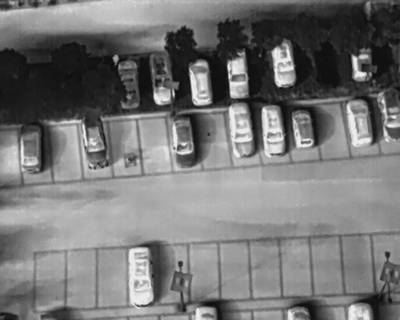

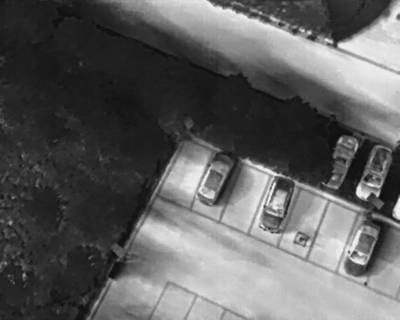
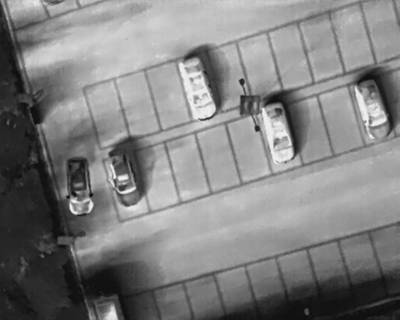
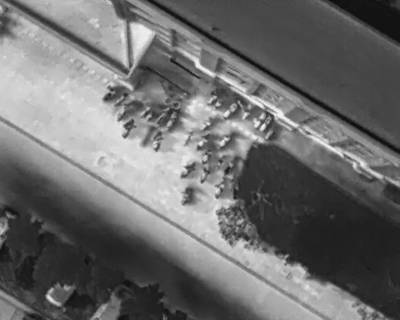
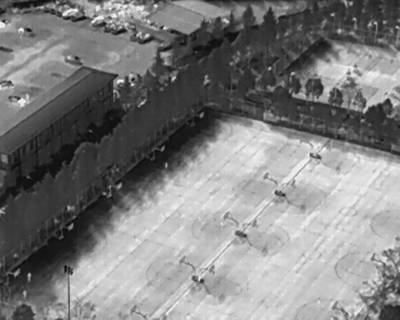
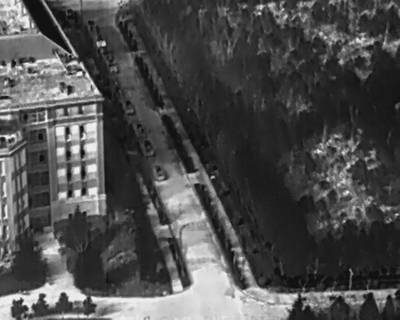
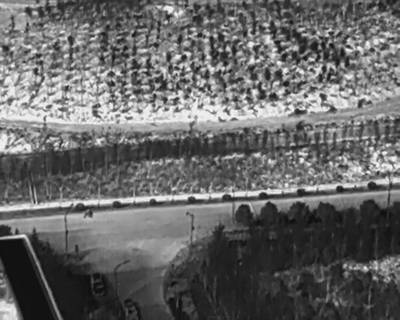

Class balance #
There are 5 annotation classes in the dataset. Find the general statistics and balances for every class in the table below. Click any row to preview images that have labels of the selected class. Sort by column to find the most rare or prevalent classes.
Class ã…¤ | Images ã…¤ | Objects ã…¤ | Count on image average | Area on image average |
---|---|---|---|---|
personâž” rectangle | 1691 | 12312 | 7.28 | 0.67% |
carâž” rectangle | 1398 | 7311 | 5.23 | 4.94% |
bicycleâž” rectangle | 460 | 4980 | 10.83 | 2.95% |
other vehicleâž” rectangle | 109 | 148 | 1.36 | 2.08% |
dontcareâž” rectangle | 103 | 148 | 1.44 | 1.09% |
Co-occurrence matrix #
Co-occurrence matrix is an extremely valuable tool that shows you the images for every pair of classes: how many images have objects of both classes at the same time. If you click any cell, you will see those images. We added the tooltip with an explanation for every cell for your convenience, just hover the mouse over a cell to preview the description.
Images #
Explore every single image in the dataset with respect to the number of annotations of each class it has. Click a row to preview selected image. Sort by any column to find anomalies and edge cases. Use horizontal scroll if the table has many columns for a large number of classes in the dataset.
Object distribution #
Interactive heatmap chart for every class with object distribution shows how many images are in the dataset with a certain number of objects of a specific class. Users can click cell and see the list of all corresponding images.
Class sizes #
The table below gives various size properties of objects for every class. Click a row to see the image with annotations of the selected class. Sort columns to find classes with the smallest or largest objects or understand the size differences between classes.
Class | Object count | Avg area | Max area | Min area | Min height | Min height | Max height | Max height | Avg height | Avg height | Min width | Min width | Max width | Max width |
---|---|---|---|---|---|---|---|---|---|---|---|---|---|---|
person rectangle | 12312 | 0.09% | 2.01% | 0.01% | 4px | 0.78% | 75px | 14.65% | 21px | 4.09% | 4px | 0.62% | 88px | 13.75% |
car rectangle | 7311 | 1.02% | 8.65% | 0.02% | 4px | 0.78% | 182px | 35.55% | 53px | 10.39% | 7px | 1.09% | 192px | 30% |
bicycle rectangle | 4980 | 0.33% | 1.64% | 0.02% | 7px | 1.37% | 82px | 16.02% | 31px | 6.06% | 6px | 0.94% | 87px | 13.59% |
other vehicle rectangle | 148 | 1.54% | 12.43% | 0.1% | 20px | 3.91% | 166px | 32.42% | 70px | 13.63% | 12px | 1.88% | 304px | 47.5% |
dontcare rectangle | 148 | 0.76% | 6.81% | 0.06% | 15px | 2.93% | 186px | 36.33% | 45px | 8.77% | 10px | 1.56% | 155px | 24.22% |
Spatial Heatmap #
The heatmaps below give the spatial distributions of all objects for every class. These visualizations provide insights into the most probable and rare object locations on the image. It helps analyze objects' placements in a dataset.

Objects #
Table contains all 24899 objects. Click a row to preview an image with annotations, and use search or pagination to navigate. Sort columns to find outliers in the dataset.
Object ID ã…¤ | Class ã…¤ | Image name click row to open | Image size height x width | Height ã…¤ | Height ã…¤ | Width ã…¤ | Width ã…¤ | Area ã…¤ |
---|---|---|---|---|---|---|---|---|
1âž” | person rectangle | 1_100_90_0_02520.jpg | 512 x 640 | 21px | 4.1% | 19px | 2.97% | 0.12% |
2âž” | person rectangle | 1_100_90_0_02520.jpg | 512 x 640 | 14px | 2.73% | 16px | 2.5% | 0.07% |
3âž” | person rectangle | 1_100_90_0_02520.jpg | 512 x 640 | 15px | 2.93% | 11px | 1.72% | 0.05% |
4âž” | person rectangle | 1_100_90_0_02520.jpg | 512 x 640 | 13px | 2.54% | 12px | 1.88% | 0.05% |
5âž” | person rectangle | 1_100_90_0_02520.jpg | 512 x 640 | 12px | 2.34% | 15px | 2.34% | 0.05% |
6âž” | person rectangle | 1_100_90_0_02520.jpg | 512 x 640 | 12px | 2.34% | 15px | 2.34% | 0.05% |
7âž” | person rectangle | 1_100_90_0_02520.jpg | 512 x 640 | 19px | 3.71% | 14px | 2.19% | 0.08% |
8âž” | person rectangle | 1_100_90_0_02520.jpg | 512 x 640 | 14px | 2.73% | 15px | 2.34% | 0.06% |
9âž” | person rectangle | 1_100_90_0_02520.jpg | 512 x 640 | 20px | 3.91% | 19px | 2.97% | 0.12% |
10âž” | person rectangle | 1_100_90_0_02520.jpg | 512 x 640 | 18px | 3.52% | 20px | 3.12% | 0.11% |
License #
Citation #
If you make use of the HIT-UAV data, please cite the following reference:
@dataset{HIT-UAV,
author={Suo, Jiashun and Wang, Tianyi and Zhang, Xingzhou and Chen, Haiyang and Zhou, Wei and Shi, Weisong},
title={HIT-UAV: A High-altitude Infrared Thermal Dataset for Unmanned Aerial Vehicle-based Object Detection},
year={2023},
url={https://zenodo.org/record/7633134}
}
If you are happy with Dataset Ninja and use provided visualizations and tools in your work, please cite us:
@misc{ visualization-tools-for-hit-uav-dataset,
title = { Visualization Tools for HIT-UAV Dataset },
type = { Computer Vision Tools },
author = { Dataset Ninja },
howpublished = { \url{ https://datasetninja.com/hit-uav } },
url = { https://datasetninja.com/hit-uav },
journal = { Dataset Ninja },
publisher = { Dataset Ninja },
year = { 2024 },
month = { jul },
note = { visited on 2024-07-27 },
}
Download #
Dataset HIT-UAV can be downloaded in Supervisely format:
As an alternative, it can be downloaded with dataset-tools package:
pip install --upgrade dataset-tools
… using following python code:
import dataset_tools as dtools
dtools.download(dataset='HIT-UAV', dst_dir='~/dataset-ninja/')
Make sure not to overlook the python code example available on the Supervisely Developer Portal. It will give you a clear idea of how to effortlessly work with the downloaded dataset.
The data in original format can be downloaded here.
Disclaimer #
Our gal from the legal dep told us we need to post this:
Dataset Ninja provides visualizations and statistics for some datasets that can be found online and can be downloaded by general audience. Dataset Ninja is not a dataset hosting platform and can only be used for informational purposes. The platform does not claim any rights for the original content, including images, videos, annotations and descriptions. Joint publishing is prohibited.
You take full responsibility when you use datasets presented at Dataset Ninja, as well as other information, including visualizations and statistics we provide. You are in charge of compliance with any dataset license and all other permissions. You are required to navigate datasets homepage and make sure that you can use it. In case of any questions, get in touch with us at hello@datasetninja.com.