Introduction #
The authors created HuTics: Human Deictic Gestures Dataset, consisting of 2040 images collected from 170 people that include various deictic gestures and objects with segmentation mask annotations. The technical evaluation shows that their object highlights can achieve the accuracy of 0.718 (mean Intersection over Union; ππΌππ) and can run at 28.3 fps. It covers four kinds of deictic gestures to objects: exhibit, pointing, present and touch.
Motivation
Interactive Machine Teaching (IMT) endeavors to enhance usersβ teaching involvement in Machine Learning (ML) model creation. Tailored for non-ML experts, IMT systems enable users to contribute training data through demonstrations. Among these, Vision-based IMT (V-IMT) systems utilize cameras to capture usersβ demonstrations. For instance, Teachable Machine allows users to construct a computer vision classification model by presenting various perspectives of each object (class) to a camera.
Despite the minimal effort required to provide training samples, prior research has indicated that ML models trained via V-IMT systems might identify objects based on irrelevant visual features. For instance, during a demonstration of a book, the model may focus on background visual elements. Failure to rectify this issue could lead to diminished model performance in real-world applications. Hence, users should be empowered to specify the image portions that the model must prioritize for accurate classification. One strategy to address this is through object annotations, which can be incorporated into the modelβs training process.
Advancements in annotation tools streamline user tasks, simplifying interactions to clicks or sketches. Despite their reduced workload, existing annotation tools are not optimized for V-IMT systems, necessitating users to conduct annotations post hoc. This undermines the overall user experience with V-IMT systems. Consequently, exploring annotation methods more seamlessly integrated into V-IMT systems is crucial.
The backend model responsible for identifying object highlights requires training data illustrating how individuals gesture towards objects in front of a camera. Among the available datasets focusing on human-object interactions, TEgO stands out as the most suitable for the authorsβ task. TEgO comprises 5758 labeled egocentric images capturing hand-object interactions. Each image includes a hand segmentation mask and precise point-level annotations indicating the objectβs location. However, these annotations alone do not fulfill the requirement for object segmentation. To address this, an attempt was made to infer the segmentation mask of the object using a click-based interactive segmentation approach. Subsequently, all generated results underwent manual inspection to eliminate data samples where the inferred segmentation masks proved to be significantly inaccurate. This process led to the creation of a custom dataset, named TEgO-Syn, comprising 5232 instances with automatically synthesized object segmentation masks.
The authors observations showed that the model was not robust enough. They then summarized three main reasons why TEgO still cannot fit their target task:
- A limited set of gestures. All data in TEgO were collected from two participants, which is insufficient to cover how different people interact with the object using gestures.
- A limited set of objects. TEgO-Syn includes 5232 images of 19 objects. Training on a small set of objects repeatedly enables the model to over-fit the features of these specific objects, which is harmful to our target task, i.e., object-agnostic segmentation.
- Egocentric images. The images in the TEgO dataset are taken from the egocentric view. Our system uses a front-facing camera, which is a common configuration in V-IMT.
Dataset description
The authors created the HuTics dataset consisting of 2040 images collected from 170 people that include various deictic gestures and objects with segmentation
mask annotations. They leveraged crowd-workers from Amazon Mechanical Turk to enrich the datasetβs diversity, following approval from their university for data collection. Each task entailed uploading 12 images depicting how deictic gestures are utilized to reference objects clearly. To ensure a varied image set, the authors categorized deictic gestures into four types: pointing, present, touch, and exhibit. Workers were instructed to capture three distinct photos for each gesture category, supplemented with example images for clarity. In total, 2040 qualifying images were amassed from 170 crowd-workers, averaging 34 years in age. Unacceptable submissions included excessively blurry images or those devoid of any discernible gestures.
On average, crowd-workers dedicated 15 minutes per task, receiving $2 for their participation. Additionally, the authors engaged five individuals through a local crowdsourcing platform to annotate object segmentation masks on the gathered images. Each annotation worker labeled approximately 408 images and received an average compensation of $78 in local currency. AnnoFab, an online polygon-based tool, facilitated the annotation process for delineating segmentation masks.
Example images in HuTics dataset. HuTics covers four kinds of deictic gestures to objects: exhibiting (top-left), pointing (top-right), presenting (bottom-left) and touching (bottom-right). The hands and objects of interest are highlighted in blue and green, respectively.
Summary #
HuTics: Human Deictic Gestures Dataset is a dataset for instance segmentation, semantic segmentation, and object detection tasks. It is used in the robotics industry.
The dataset consists of 2040 images with 6428 labeled objects belonging to 2 different classes including object of interest and hand.
Images in the HuTics dataset have pixel-level instance segmentation annotations. Due to the nature of the instance segmentation task, it can be automatically transformed into a semantic segmentation (only one mask for every class) or object detection (bounding boxes for every object) tasks. There are 5 (0% of the total) unlabeled images (i.e. without annotations). There are 2 splits in the dataset: train (1632 images) and test (408 images). Alternatively, the dataset could be split into 4 4 taxonomy categories: present (1728 objects), pointing (1645 objects), touch (1563 objects), and exhibit (1492 objects). Additionally, every image marked with its sequence tag. The dataset was released in 2022 by the University of Tokyo, Japan.
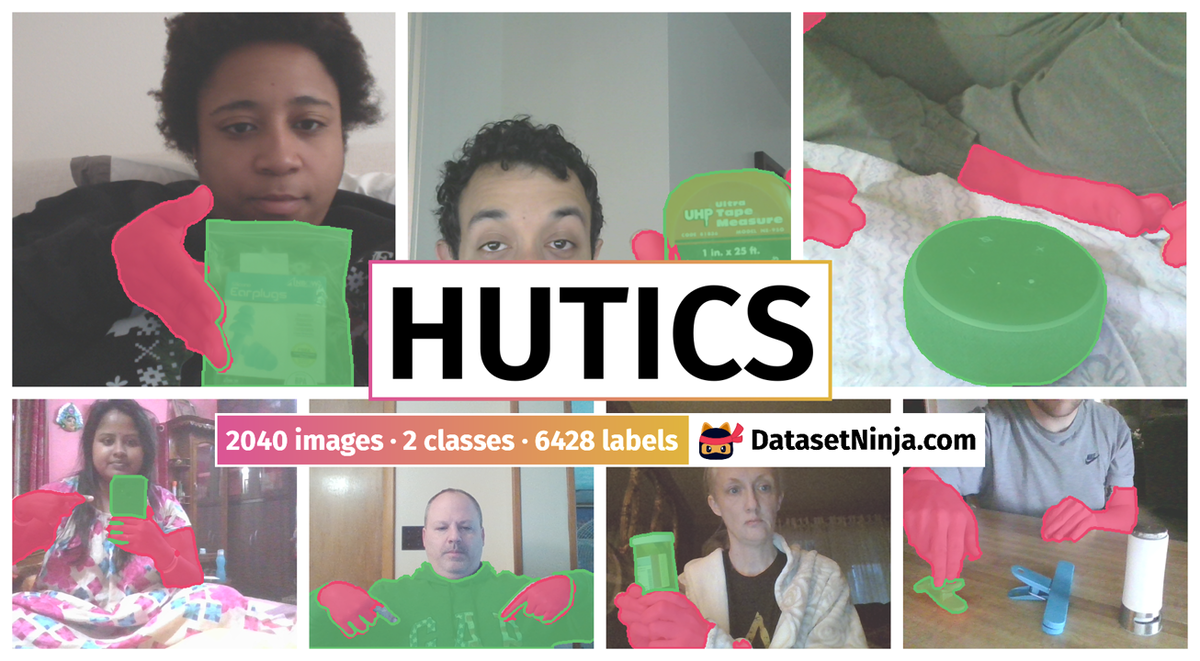
Explore #
HuTics dataset has 2040 images. Click on one of the examples below or open "Explore" tool anytime you need to view dataset images with annotations. This tool has extended visualization capabilities like zoom, translation, objects table, custom filters and more. Hover the mouse over the images to hide or show annotations.

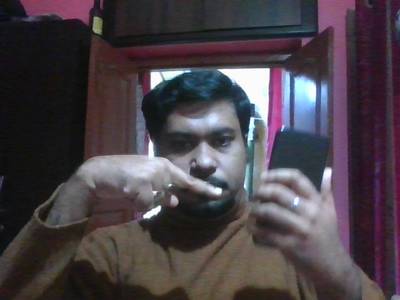
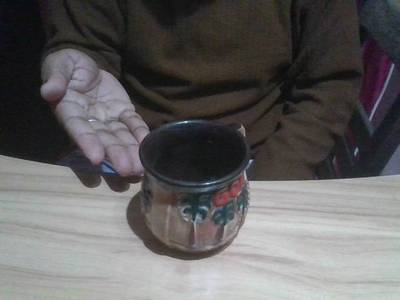


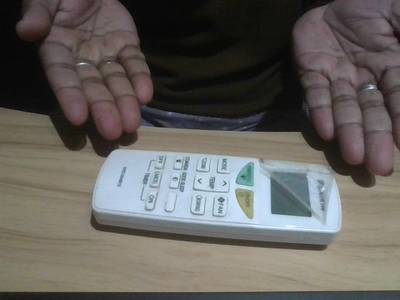


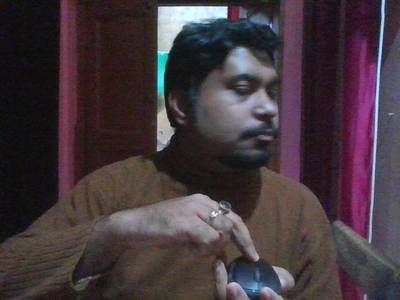

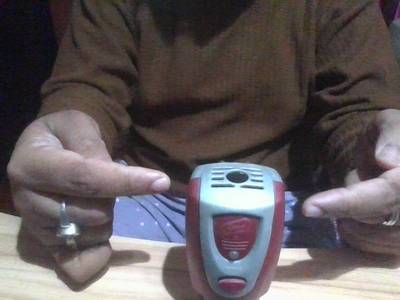
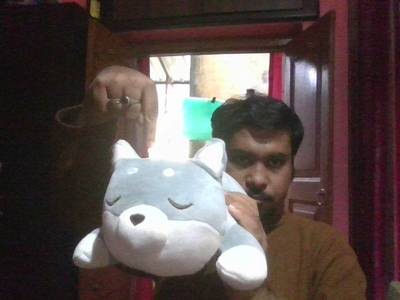
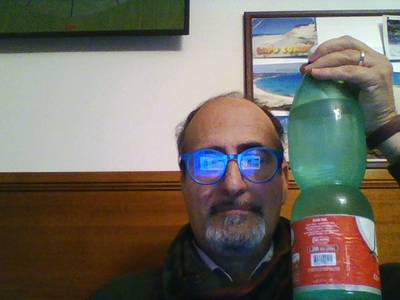
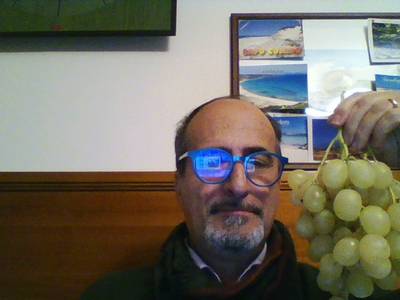
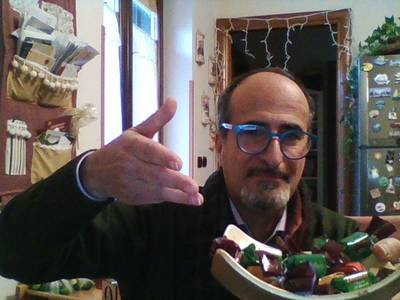
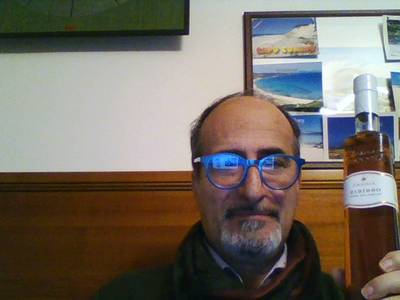
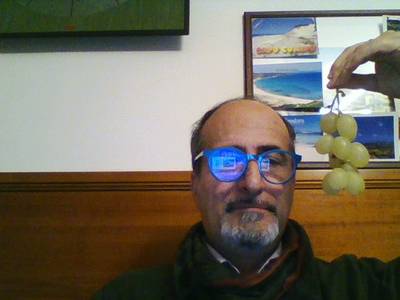
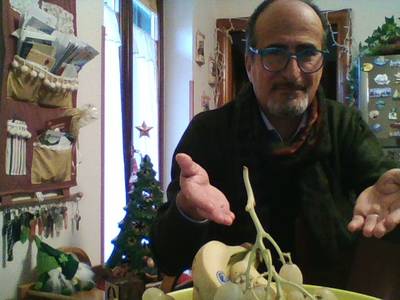


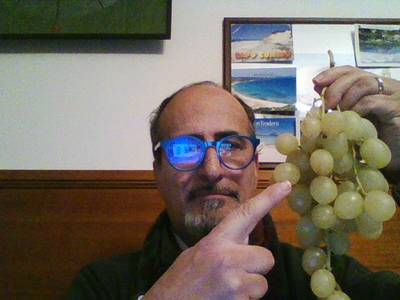
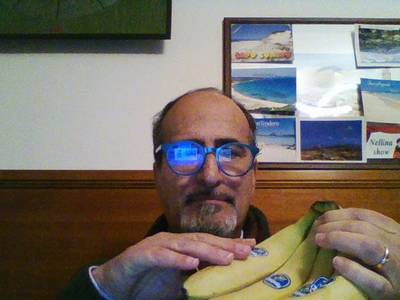
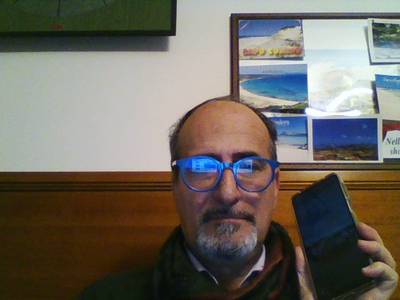
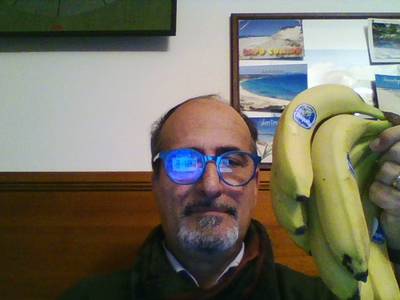
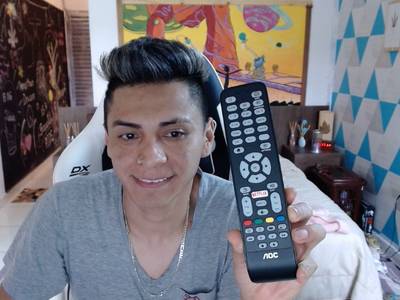

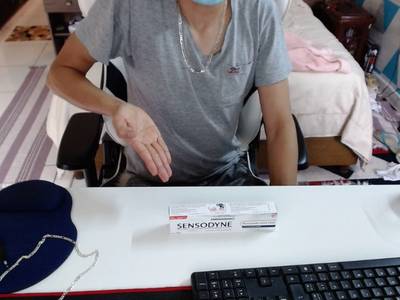
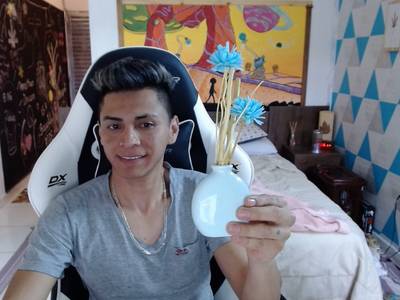
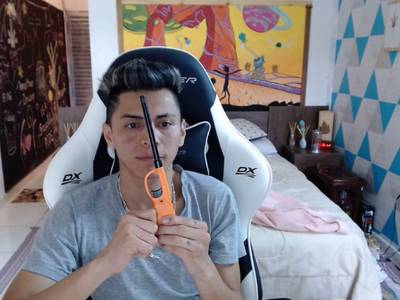
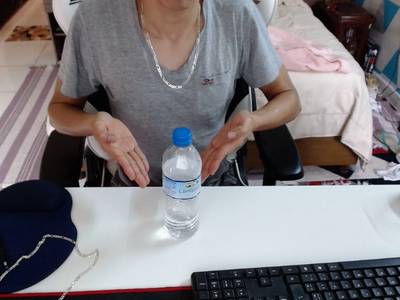
Class balance #
There are 2 annotation classes in the dataset. Find the general statistics and balances for every class in the table below. Click any row to preview images that have labels of the selected class. Sort by column to find the most rare or prevalent classes.
Class γ
€ | Images γ
€ | Objects γ
€ | Count on image average | Area on image average |
---|---|---|---|---|
object of interestβ mask | 2023 | 2023 | 1 | 7.08% |
handβ mask | 2021 | 4405 | 2.18 | 9.97% |
Co-occurrence matrix #
Co-occurrence matrix is an extremely valuable tool that shows you the images for every pair of classes: how many images have objects of both classes at the same time. If you click any cell, you will see those images. We added the tooltip with an explanation for every cell for your convenience, just hover the mouse over a cell to preview the description.
Images #
Explore every single image in the dataset with respect to the number of annotations of each class it has. Click a row to preview selected image. Sort by any column to find anomalies and edge cases. Use horizontal scroll if the table has many columns for a large number of classes in the dataset.
Object distribution #
Interactive heatmap chart for every class with object distribution shows how many images are in the dataset with a certain number of objects of a specific class. Users can click cell and see the list of all corresponding images.
Class sizes #
The table below gives various size properties of objects for every class. Click a row to see the image with annotations of the selected class. Sort columns to find classes with the smallest or largest objects or understand the size differences between classes.
Class | Object count | Avg area | Max area | Min area | Min height | Min height | Max height | Max height | Avg height | Avg height | Min width | Min width | Max width | Max width |
---|---|---|---|---|---|---|---|---|---|---|---|---|---|---|
hand mask | 4405 | 4.58% | 51.48% | 0.01% | 2px | 0.42% | 480px | 100% | 148px | 30.77% | 2px | 0.31% | 640px | 100% |
object of interest mask | 2023 | 7.08% | 53.03% | 0.03% | 10px | 2.08% | 480px | 100% | 168px | 34.93% | 18px | 2.81% | 640px | 100% |
Spatial Heatmap #
The heatmaps below give the spatial distributions of all objects for every class. These visualizations provide insights into the most probable and rare object locations on the image. It helps analyze objects' placements in a dataset.
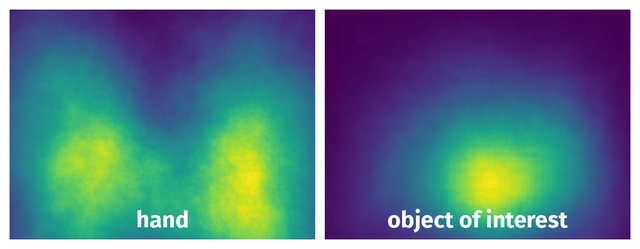
Objects #
Table contains all 6428 objects. Click a row to preview an image with annotations, and use search or pagination to navigate. Sort columns to find outliers in the dataset.
Object ID γ
€ | Class γ
€ | Image name click row to open | Image size height x width | Height γ
€ | Height γ
€ | Width γ
€ | Width γ
€ | Area γ
€ |
---|---|---|---|---|---|---|---|---|
1β | hand mask | 00087_exhibit-2.png | 480 x 640 | 61px | 12.71% | 26px | 4.06% | 0.25% |
2β | hand mask | 00087_exhibit-2.png | 480 x 640 | 9px | 1.88% | 7px | 1.09% | 0.01% |
3β | hand mask | 00087_exhibit-2.png | 480 x 640 | 72px | 15% | 105px | 16.41% | 1.59% |
4β | object of interest mask | 00087_exhibit-2.png | 480 x 640 | 206px | 42.92% | 333px | 52.03% | 17.63% |
5β | hand mask | 00107_touch-2.png | 480 x 640 | 144px | 30% | 248px | 38.75% | 7.36% |
6β | hand mask | 00107_touch-2.png | 480 x 640 | 136px | 28.33% | 329px | 51.41% | 8.31% |
7β | object of interest mask | 00107_touch-2.png | 480 x 640 | 109px | 22.71% | 260px | 40.62% | 6.8% |
8β | hand mask | 00135_present-1.png | 480 x 640 | 291px | 60.62% | 84px | 13.12% | 3.87% |
9β | hand mask | 00135_present-1.png | 480 x 640 | 283px | 58.96% | 104px | 16.25% | 3.81% |
10β | hand mask | 00135_present-1.png | 480 x 640 | 12px | 2.5% | 9px | 1.41% | 0.02% |
License #
Citation #
If you make use of the HuTics data, please cite the following reference:
@misc{zhou2022gesture,
doi = {10.48550/ARXIV.2208.01211},
url = {https://arxiv.org/abs/2208.01211},
author = {Zhou, Zhongyi and Yatani, Koji},
title = {Gesture-aware Interactive Machine Teaching with In-situ Object Annotations},
publisher = {arXiv},
year = {2022}
}
@inproceedings{zhou2021enhancing,
author = {Zhou, Zhongyi and Yatani, Koji},
title = {Enhancing Model Assessment in Vision-Based Interactive Machine Teaching through Real-Time Saliency Map Visualization},
year = {2021},
isbn = {9781450386555},
publisher = {Association for Computing Machinery},
address = {New York, NY, USA},
url = {https://doi.org/10.1145/3474349.3480194},
doi = {10.1145/3474349.3480194},
pages = {112β114},
numpages = {3},
keywords = {Visualization, Saliency Map, Interactive Machine Teaching},
location = {Virtual Event, USA},
series = {UIST '21}
}
If you are happy with Dataset Ninja and use provided visualizations and tools in your work, please cite us:
@misc{ visualization-tools-for-hu-tics-dataset,
title = { Visualization Tools for HuTics Dataset },
type = { Computer Vision Tools },
author = { Dataset Ninja },
howpublished = { \url{ https://datasetninja.com/hu-tics } },
url = { https://datasetninja.com/hu-tics },
journal = { Dataset Ninja },
publisher = { Dataset Ninja },
year = { 2025 },
month = { jul },
note = { visited on 2025-07-04 },
}
Download #
Dataset HuTics can be downloaded in Supervisely format:
As an alternative, it can be downloaded with dataset-tools package:
pip install --upgrade dataset-tools
β¦ using following python code:
import dataset_tools as dtools
dtools.download(dataset='HuTics', dst_dir='~/dataset-ninja/')
Make sure not to overlook the python code example available on the Supervisely Developer Portal. It will give you a clear idea of how to effortlessly work with the downloaded dataset.
The data in original format can be downloaded here.
Disclaimer #
Our gal from the legal dep told us we need to post this:
Dataset Ninja provides visualizations and statistics for some datasets that can be found online and can be downloaded by general audience. Dataset Ninja is not a dataset hosting platform and can only be used for informational purposes. The platform does not claim any rights for the original content, including images, videos, annotations and descriptions. Joint publishing is prohibited.
You take full responsibility when you use datasets presented at Dataset Ninja, as well as other information, including visualizations and statistics we provide. You are in charge of compliance with any dataset license and all other permissions. You are required to navigate datasets homepage and make sure that you can use it. In case of any questions, get in touch with us at hello@datasetninja.com.