Introduction #
The authors collected and labeled a detection dataset named Human Parts Dataset which contains annotations of three categories, including person, hand and
face. The proposed dataset contains high-resolution images which are randomly selected from AI-challenger dataset. The authors added the missed person body annotations and labeled hand and face additionally in each image.
Motivation
Detecting the human body, face, and hand robustly in natural settings is fundamental to general object detection. This capability is essential for a variety of tasks centered around individuals, including pedestrian detection, person re-identification, facial landmarking, and driver behavior monitoring. Over decades, considerable attention has been devoted to addressing the challenges of detecting human body parts in natural environments, leading to significant advancements in recent detection algorithms. This progress is largely attributed to the evolution of deep Convolutional Neural Networks (CNNs), which have greatly enhanced person, face, and hand detection. Human body parts constitute multi-level objects, with faces and hands being sub-components of the body. Similar multi-level object structures are prevalent in everyday scenarios, such as laptops and keyboards, lungs and lung nodules, or buses and wheels. Despite this, many detection frameworks overlook the inherent correlations between these multi-level objects. Instead, they tend to treat sub-objects and objects uniformly, neglecting the nuanced relationships between them in solving multi-level object detection challenges.
In tasks involving multi-level objects using general detection algorithms, detecting large objects such as the human body typically yields relatively high performance. However, significant challenges arise in real-world applications, particularly when training detectors for small objects like faces and hands. These challenges stem from the considerable pose variations and frequent occlusions encountered, which hinder the attainment of detection capabilities comparable to those of humans. The substantial scale disparity between the body (objects) and its smaller parts (sub-objects) results in the predominance of large objects, such as the human body, occupying the majority of the image. Conversely, small objects like hands and faces typically occupy a comparatively smaller area. Consequently, during training, there tends to be an abundance of background information relative to the small objects, leading to significant interference during small object detection.
Examples of multi-level objects. Boxes in green are sub-objects of boxes in blue.
Dataset description
In their work, the authors perform the person, face and hand detection tasks together to explore the more efficient detection methods for the multi-level objects. They collected and labeled a detection Human Parts Dataset. The proposed dataset contains high-resolution images which were randomly selected from AI-challenger dataset. person category has already been labeled in this dataset. However, the small human whose body parts are hard to distinguish or the vague ones whose body contours are hard to recognize are missed-labeled in this dataset. The authors added the missed person body annotations and labeled hand and face additionally in each image. The number of persons in each image range from 1 to 11. In total, dataset consists of 14,962 images (12,000 for train, 2,962 for testing) with 10,6879 annotations (35,306 persons, 27,821 faces and 43,752 hands). They have labeled every visible person, hand or face with xmin, ymin, xmax and ymax coordinates and ensured that annotations cover the entire objects including the blocked parts but without extra background.
Samples of annotated images in the Human Parts Dataset.
DataSet | Images | Person | Hand | Face | Total Instance |
---|---|---|---|---|---|
Caltech | 42,782 | X | - | - | 13,674 |
CityPersons | 2,975 | X | - | - | 19,238 |
VGG Hand | 4,800 | - | X | - | 15,053 |
EgoHands | 11,194 | - | X | - | 13,050 |
FDDB | 2,854 | - | - | X | 5,171 |
Wider Face | 32,203 | - | - | X | 393,703 |
Human Parts | 14,962 | X | X | X | 106,879 |
Comparison of different human parts detection datasets.
Summary #
Human Parts Dataset is a dataset for object detection and identification tasks. It is used in the entertainment industry.
The dataset consists of 14962 images with 106879 labeled objects belonging to 3 different classes including person, face, and hand.
Images in the Human Parts dataset have bounding box annotations. All images are labeled (i.e. with annotations). There are 2 splits in the dataset: train (12000 images) and val (2962 images). The dataset was released in 2019 by the Beihang University, China and Beijing University of Posts and Telecommunications, China.
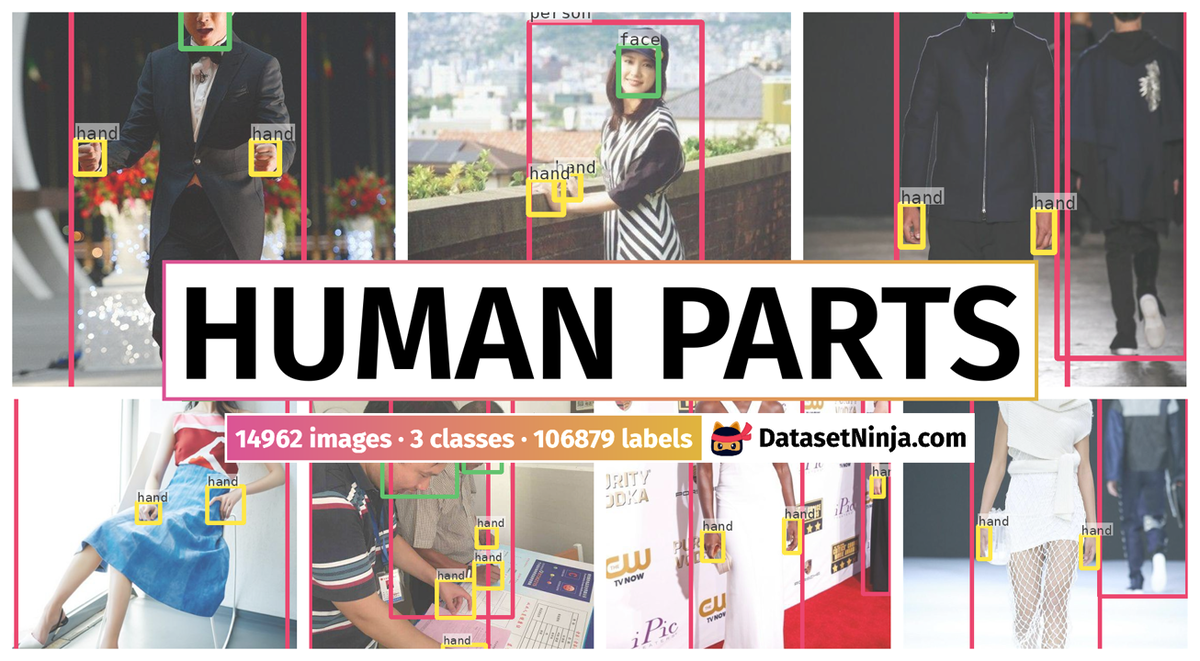
Explore #
Human Parts dataset has 14962 images. Click on one of the examples below or open "Explore" tool anytime you need to view dataset images with annotations. This tool has extended visualization capabilities like zoom, translation, objects table, custom filters and more. Hover the mouse over the images to hide or show annotations.
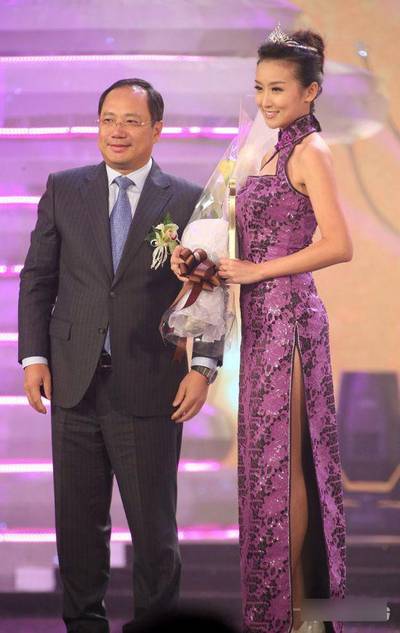
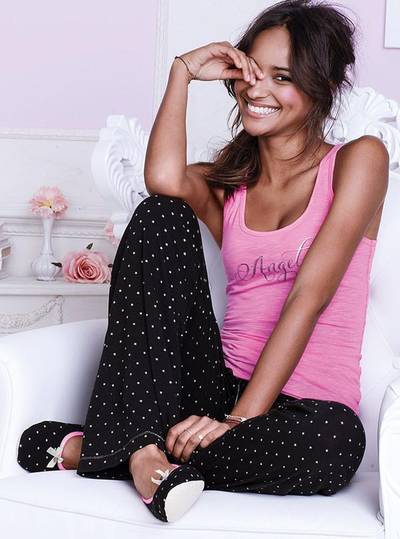
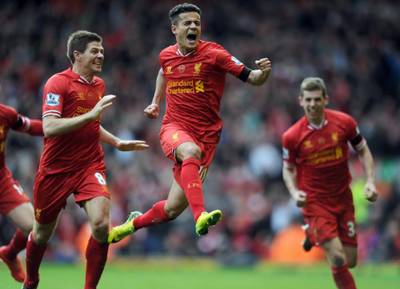
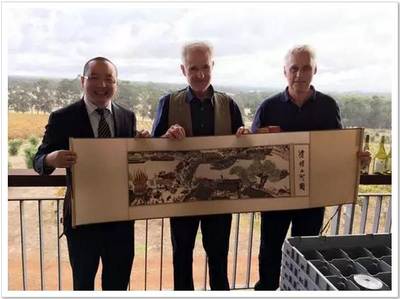
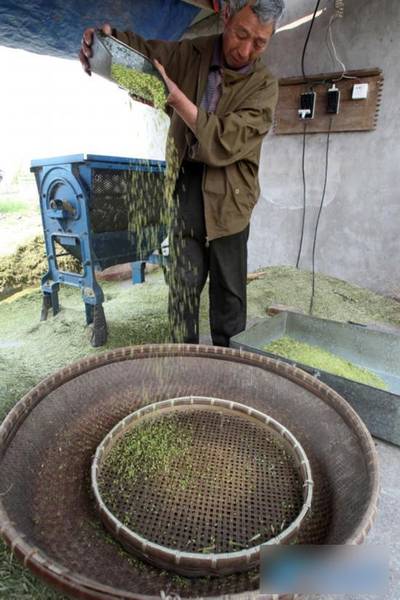

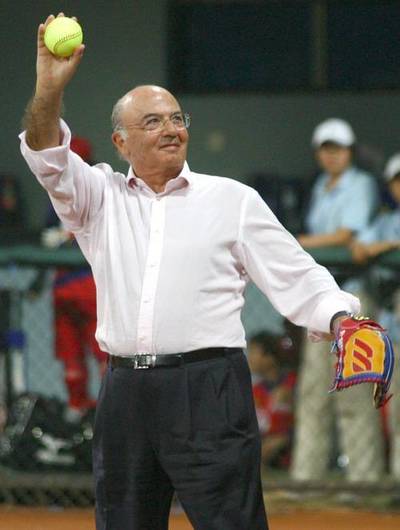
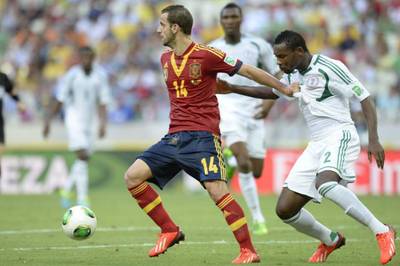
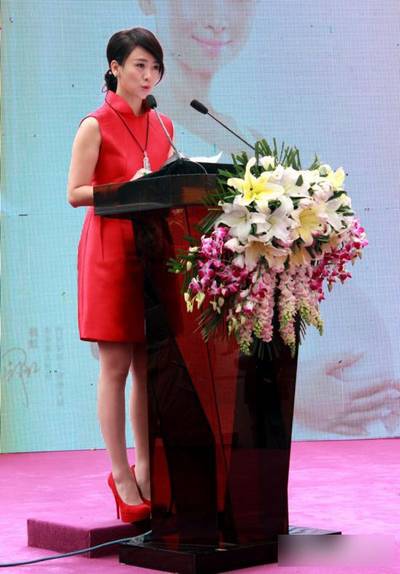
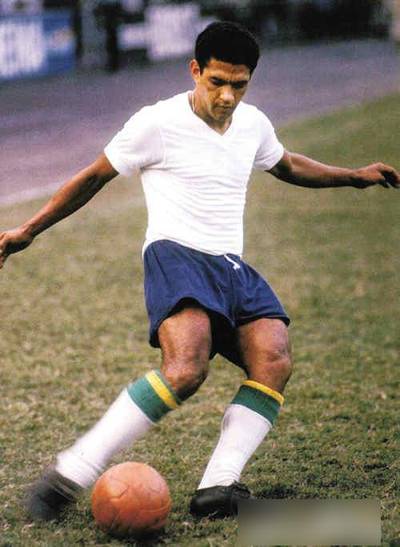
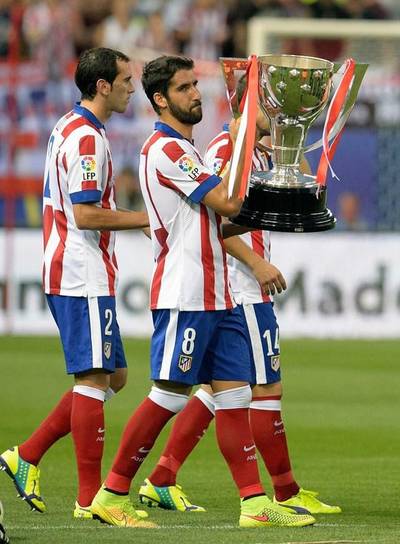
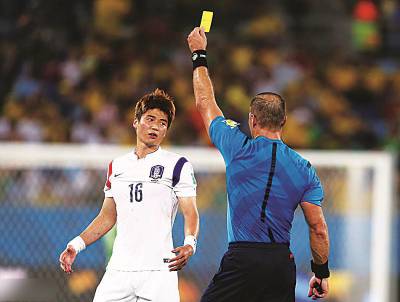
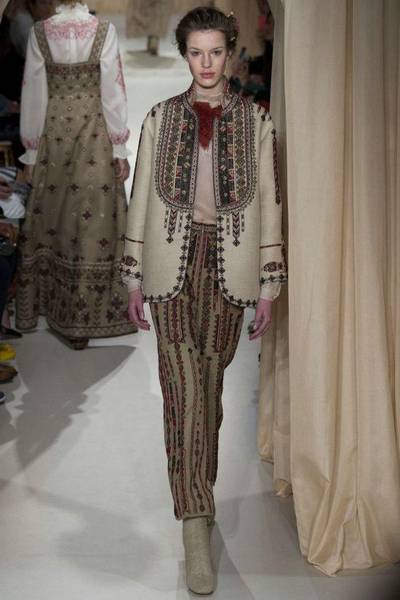
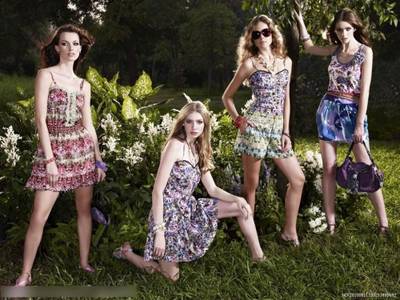
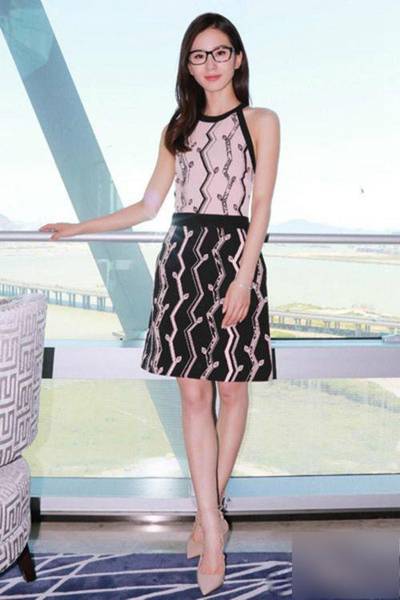
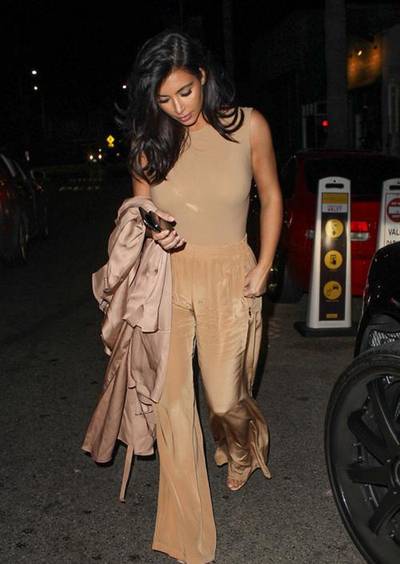
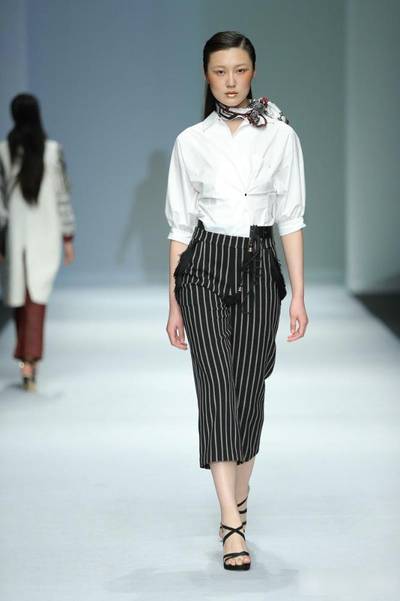
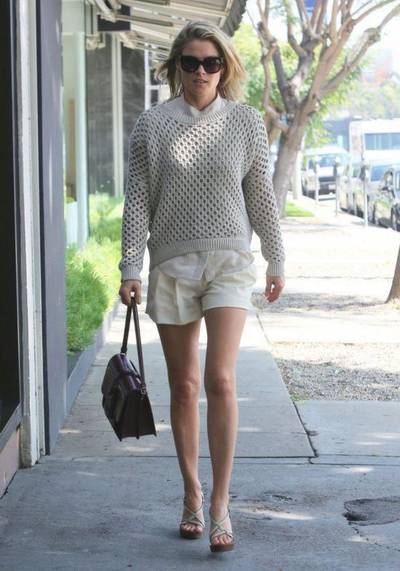

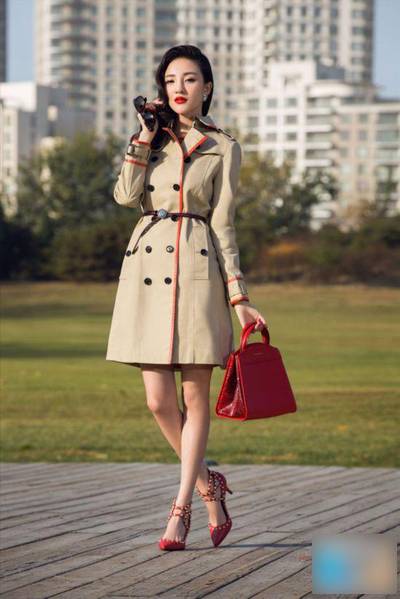
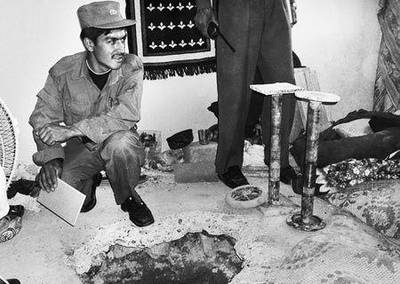
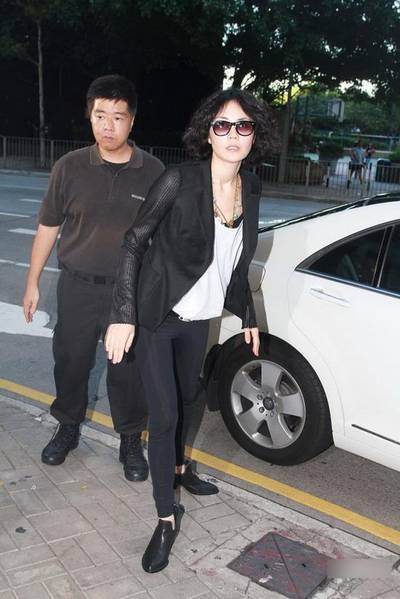
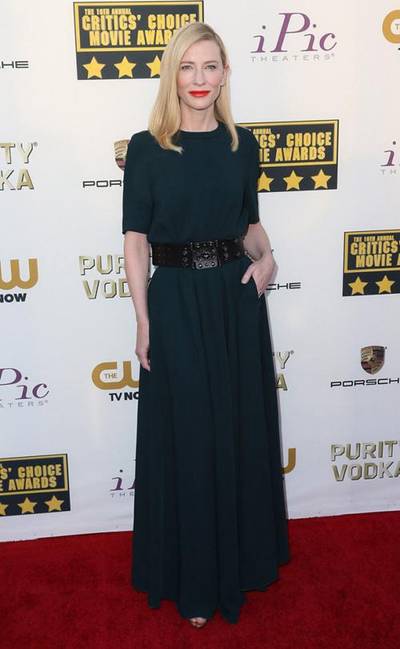
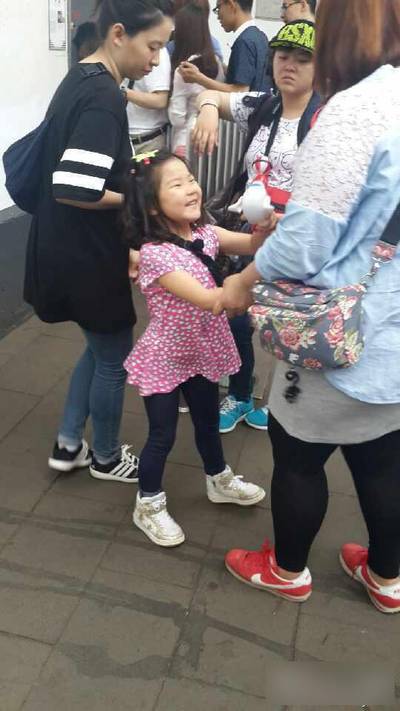
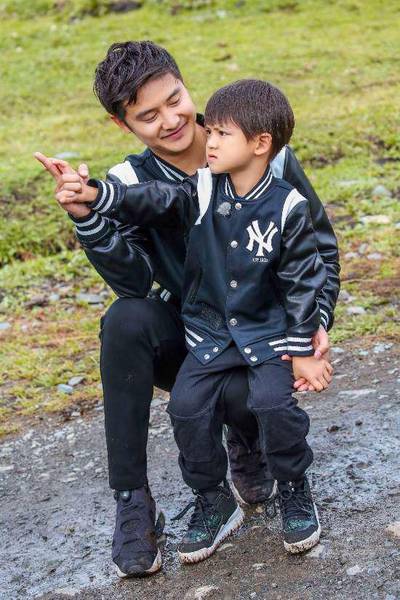
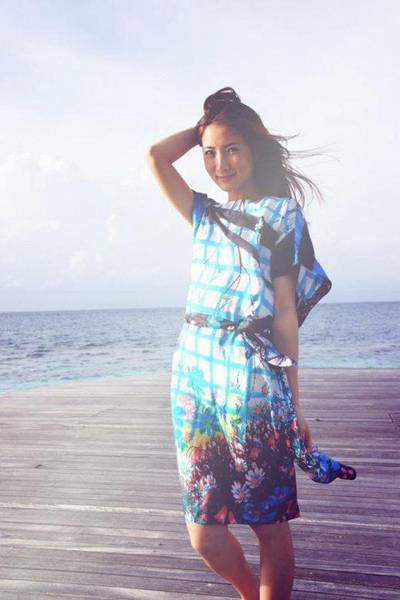
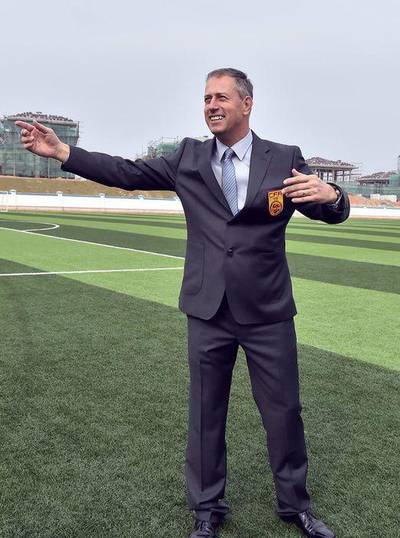
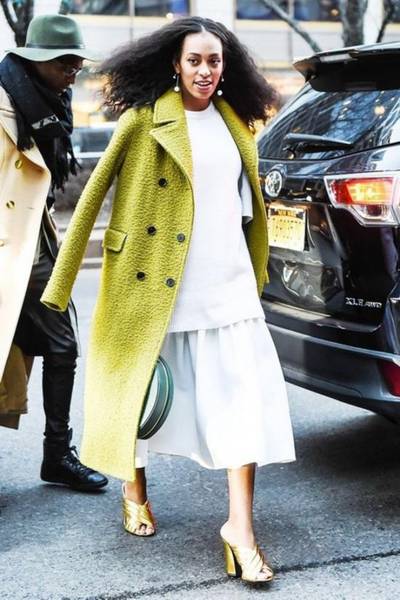
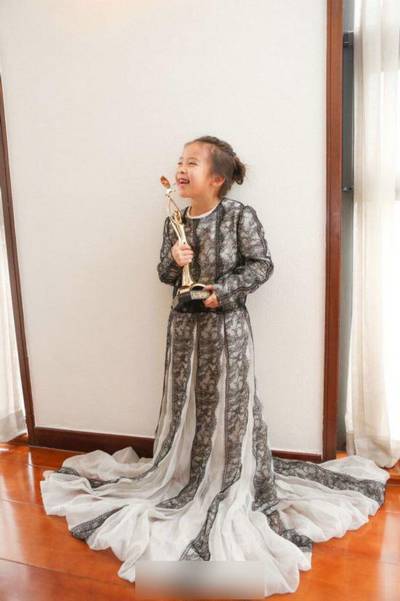
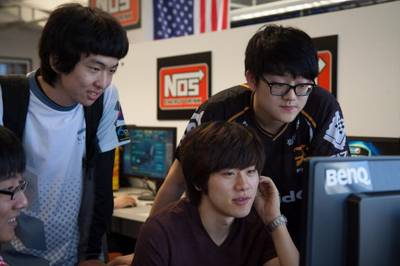
Class balance #
There are 3 annotation classes in the dataset. Find the general statistics and balances for every class in the table below. Click any row to preview images that have labels of the selected class. Sort by column to find the most rare or prevalent classes.
Class ã…¤ | Images ã…¤ | Objects ã…¤ | Count on image average | Area on image average |
---|---|---|---|---|
personâž” rectangle | 14962 | 35306 | 2.36 | 60.53% |
faceâž” rectangle | 14859 | 27821 | 1.87 | 2.26% |
handâž” rectangle | 14334 | 43752 | 3.05 | 1.78% |
Co-occurrence matrix #
Co-occurrence matrix is an extremely valuable tool that shows you the images for every pair of classes: how many images have objects of both classes at the same time. If you click any cell, you will see those images. We added the tooltip with an explanation for every cell for your convenience, just hover the mouse over a cell to preview the description.
Images #
Explore every single image in the dataset with respect to the number of annotations of each class it has. Click a row to preview selected image. Sort by any column to find anomalies and edge cases. Use horizontal scroll if the table has many columns for a large number of classes in the dataset.
Object distribution #
Interactive heatmap chart for every class with object distribution shows how many images are in the dataset with a certain number of objects of a specific class. Users can click cell and see the list of all corresponding images.
Class sizes #
The table below gives various size properties of objects for every class. Click a row to see the image with annotations of the selected class. Sort columns to find classes with the smallest or largest objects or understand the size differences between classes.
Class | Object count | Avg area | Max area | Min area | Min height | Min height | Max height | Max height | Avg height | Avg height | Min width | Min width | Max width | Max width |
---|---|---|---|---|---|---|---|---|---|---|---|---|---|---|
hand rectangle | 43752 | 0.6% | 13.41% | 0.02% | 5px | 1.09% | 361px | 57.03% | 55px | 7.38% | 1px | 0.2% | 327px | 47.82% |
person rectangle | 35306 | 31.28% | 99.77% | 0.03% | 14px | 2% | 999px | 99.9% | 552px | 72.74% | 8px | 1.33% | 960px | 99.89% |
face rectangle | 27821 | 1.21% | 92.37% | 0.02% | 8px | 1.45% | 940px | 97.85% | 83px | 11.3% | 2px | 0.39% | 490px | 94.4% |
Spatial Heatmap #
The heatmaps below give the spatial distributions of all objects for every class. These visualizations provide insights into the most probable and rare object locations on the image. It helps analyze objects' placements in a dataset.
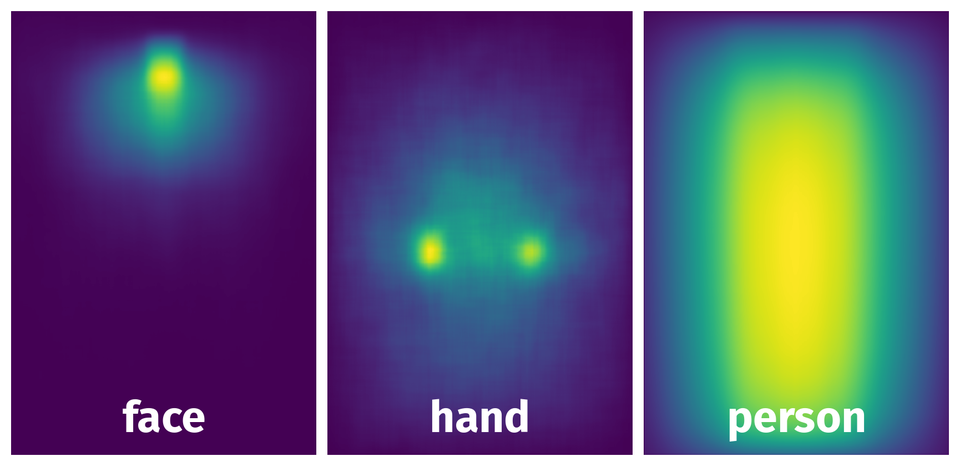
Objects #
Table contains all 100156 objects. Click a row to preview an image with annotations, and use search or pagination to navigate. Sort columns to find outliers in the dataset.
Object ID ã…¤ | Class ã…¤ | Image name click row to open | Image size height x width | Height ã…¤ | Height ã…¤ | Width ã…¤ | Width ã…¤ | Area ã…¤ |
---|---|---|---|---|---|---|---|---|
1âž” | person rectangle | 11a60681255b059e5ca23acfb0011f4f42f40c0b.jpeg | 725 x 500 | 613px | 84.55% | 189px | 37.8% | 31.96% |
2âž” | person rectangle | 11a60681255b059e5ca23acfb0011f4f42f40c0b.jpeg | 725 x 500 | 596px | 82.21% | 151px | 30.2% | 24.83% |
3âž” | face rectangle | 11a60681255b059e5ca23acfb0011f4f42f40c0b.jpeg | 725 x 500 | 66px | 9.1% | 49px | 9.8% | 0.89% |
4âž” | face rectangle | 11a60681255b059e5ca23acfb0011f4f42f40c0b.jpeg | 725 x 500 | 58px | 8% | 50px | 10% | 0.8% |
5âž” | hand rectangle | 11a60681255b059e5ca23acfb0011f4f42f40c0b.jpeg | 725 x 500 | 40px | 5.52% | 43px | 8.6% | 0.47% |
6âž” | hand rectangle | 11a60681255b059e5ca23acfb0011f4f42f40c0b.jpeg | 725 x 500 | 50px | 6.9% | 34px | 6.8% | 0.47% |
7âž” | hand rectangle | 11a60681255b059e5ca23acfb0011f4f42f40c0b.jpeg | 725 x 500 | 49px | 6.76% | 50px | 10% | 0.68% |
8âž” | hand rectangle | 11a60681255b059e5ca23acfb0011f4f42f40c0b.jpeg | 725 x 500 | 42px | 5.79% | 47px | 9.4% | 0.54% |
9âž” | person rectangle | b0807f6ff343c3e4f7644901fe0981c81b0bcd18.jpeg | 695 x 520 | 598px | 86.04% | 266px | 51.15% | 44.01% |
10âž” | face rectangle | b0807f6ff343c3e4f7644901fe0981c81b0bcd18.jpeg | 695 x 520 | 89px | 12.81% | 68px | 13.08% | 1.67% |
License #
Citation #
If you make use of the Human Parts data, please cite the following reference:
@article{didnet,
title={Detector-in-Detector: Multi-Level Analysis for Human-Parts},
author={Xiaojie Li, Lu yang, Qing Song, Fuqiang Zhou},
journal={arXiv preprint arXiv:****},
year={2019}
}
If you are happy with Dataset Ninja and use provided visualizations and tools in your work, please cite us:
@misc{ visualization-tools-for-human-parts-dataset,
title = { Visualization Tools for Human Parts Dataset },
type = { Computer Vision Tools },
author = { Dataset Ninja },
howpublished = { \url{ https://datasetninja.com/human-parts } },
url = { https://datasetninja.com/human-parts },
journal = { Dataset Ninja },
publisher = { Dataset Ninja },
year = { 2025 },
month = { may },
note = { visited on 2025-05-11 },
}
Download #
Dataset Human Parts can be downloaded in Supervisely format:
As an alternative, it can be downloaded with dataset-tools package:
pip install --upgrade dataset-tools
… using following python code:
import dataset_tools as dtools
dtools.download(dataset='Human Parts', dst_dir='~/dataset-ninja/')
Make sure not to overlook the python code example available on the Supervisely Developer Portal. It will give you a clear idea of how to effortlessly work with the downloaded dataset.
The data in original format can be downloaded here.
Disclaimer #
Our gal from the legal dep told us we need to post this:
Dataset Ninja provides visualizations and statistics for some datasets that can be found online and can be downloaded by general audience. Dataset Ninja is not a dataset hosting platform and can only be used for informational purposes. The platform does not claim any rights for the original content, including images, videos, annotations and descriptions. Joint publishing is prohibited.
You take full responsibility when you use datasets presented at Dataset Ninja, as well as other information, including visualizations and statistics we provide. You are in charge of compliance with any dataset license and all other permissions. You are required to navigate datasets homepage and make sure that you can use it. In case of any questions, get in touch with us at hello@datasetninja.com.