Introduction #
The ISIC 2017: Part 1 - Lesion Segmentation dataset is specifically designed for a semantic segmentation task focused on dermatology. Comprising 2750 images, each image in the dataset is associated with 1 single class, namely lesion. The primary objective of this dataset is to challenge participants to generate automated predictions of lesion segmentation boundaries from dermoscopic images. Each image is accompanied by expert manual tracings of lesion boundaries represented as binary masks, providing a ground truth for the segmentation task. This dataset serves as a valuable resource for advancing the development and evaluation of algorithms in the field of dermatological image analysis.
About ISIC 2017 Challenge
The International Skin Imaging Collaboration (ISIC) has begun to aggregate a large-scale publicly accessible dataset of dermoscopy images. Currently, the dataset houses more than 20,000 images from leading clinical centers internationally, acquired from a variety of devices used at each center. The ISIC dataset was the foundation for the first public benchmark challenge on dermoscopic image analysis in 2016.
The goal of the challenge is to help participants develop image analysis tools to enable the automated diagnosis of melanoma from dermoscopic images. Image analysis of skin lesions is composed of 3 parts:
- Part 1: Lesion Segmentation (current)
- Part 2: Detection and Localization of Visual Dermoscopic Features/Patterns
- Part 3: Disease Classification (Available on DatasetNinja)
For each, data consisted of images and corresponding ground truth annotations, split into training (n=2000), validation (n=150), and holdout test (n=600) datasets. Predictions could be submitted on validation and test datasets. The validation submissions provided instantaneous feedback in the form of performance evaluations, as well as ranking in comparison to other participants. Test submissions only provided feedback after the submission deadline.
About Lesion Segmentation
Participants were asked to submit automated predictions of lesion segmentations from dermoscopic images in the form of binary masks. Lesion segmentation training data included the original image, paired with the expert manual tracing of the lesion boundaries also in the form of a binary mask, where pixel values of 255 were considered inside the area of the lesion, and pixel values of 0 were outside.
Images from “Part 1: Lesion Segmentation.” Top: Original images. Bottom: Segmentation masks.
Summary #
ISIC 2017: Part 1 - Lesion Segmentation is a dataset for a semantic segmentation task. It is used in the medical industry.
The dataset consists of 2750 images with 2750 labeled objects belonging to 1 single class (lesion).
Images in the ISIC 2017: Part 1 - Lesion Segmentation dataset have pixel-level semantic segmentation annotations. All images are labeled (i.e. with annotations). There are 3 splits in the dataset: train (2000 images), test (600 images), and valid (150 images). Also, the dataset contains age_approximate and sex tags. The dataset was released in 2017 by the IBM T. J. Watson Research Center, Emory University, University of Central Arkansas, Kitware, Memorial Sloan-Kettering Cancer Center, Missouri University of Science and Technology, USA, and Medical University of Vienna, Austria.
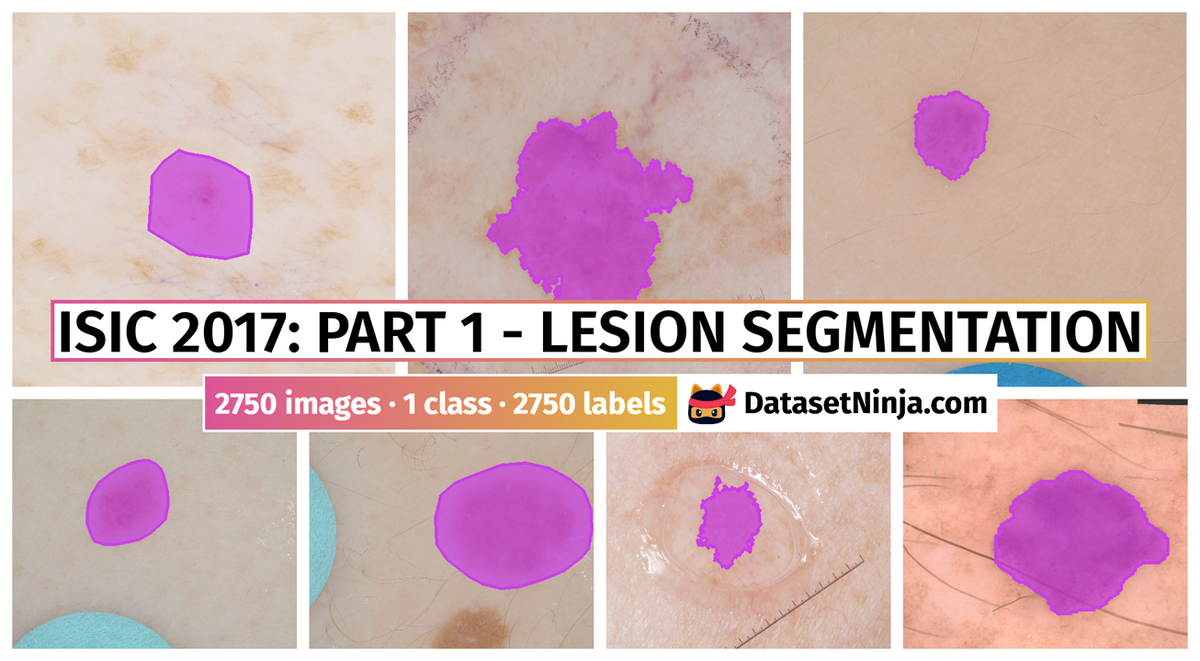
Explore #
ISIC 2017: Part 1 - Lesion Segmentation dataset has 2750 images. Click on one of the examples below or open "Explore" tool anytime you need to view dataset images with annotations. This tool has extended visualization capabilities like zoom, translation, objects table, custom filters and more. Hover the mouse over the images to hide or show annotations.
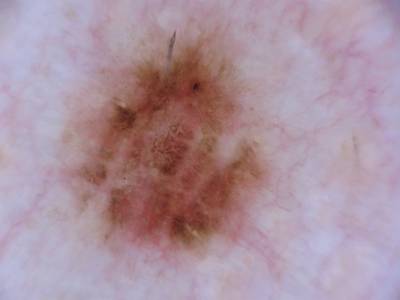
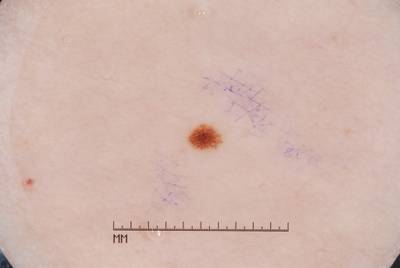
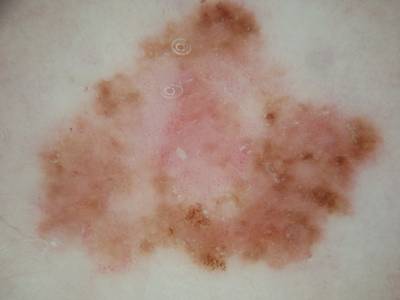
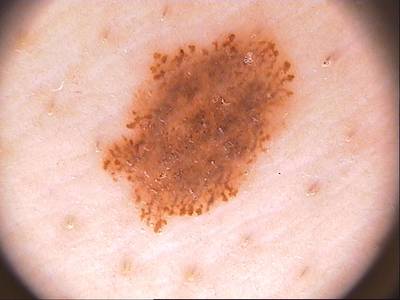
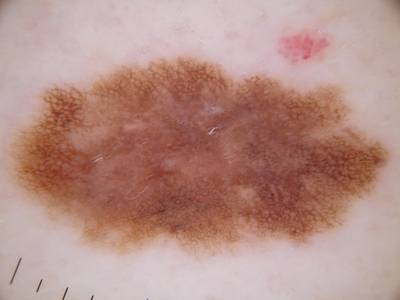
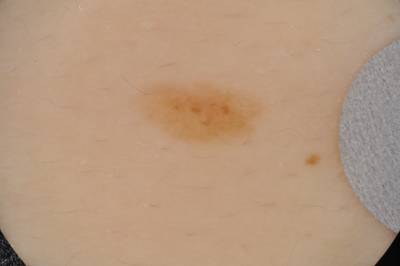
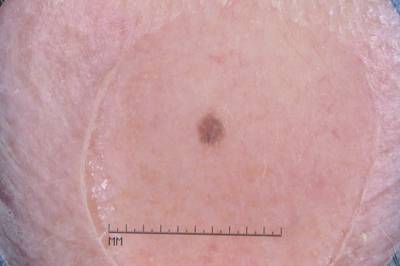
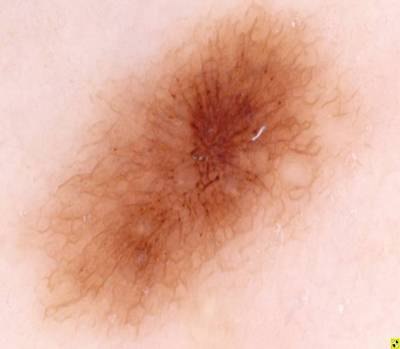
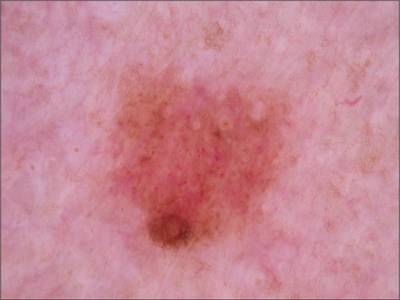
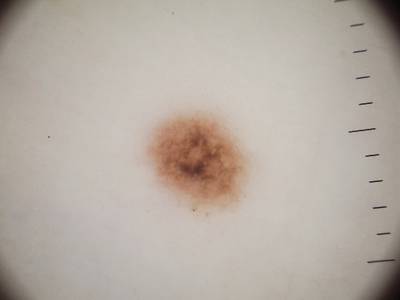
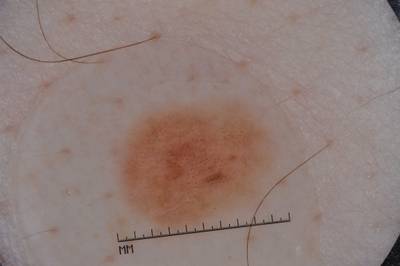
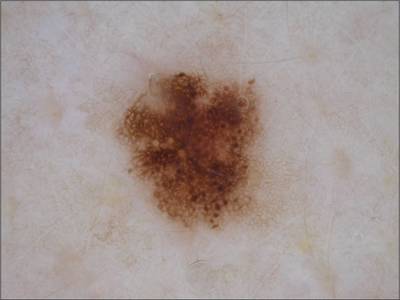
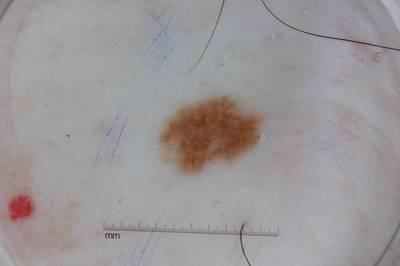

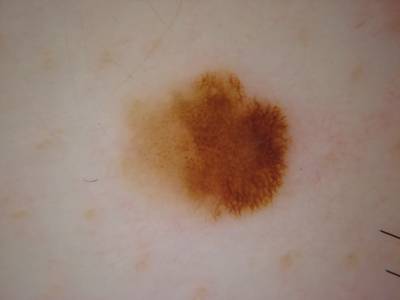
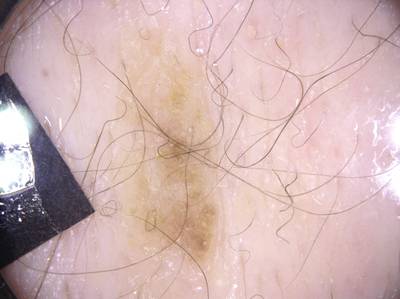
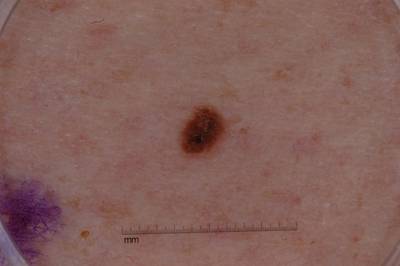
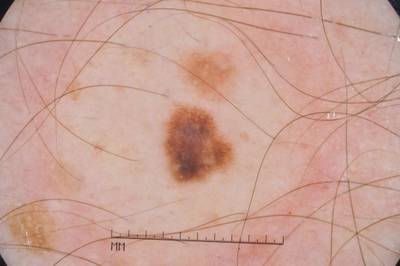

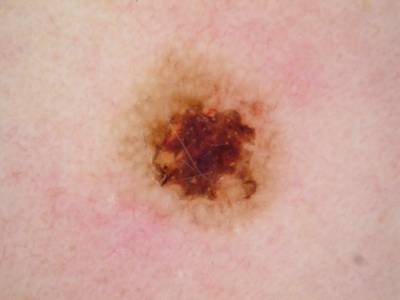
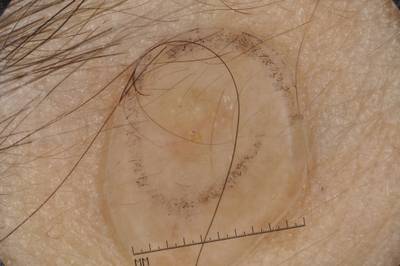
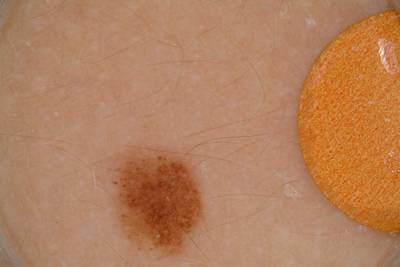
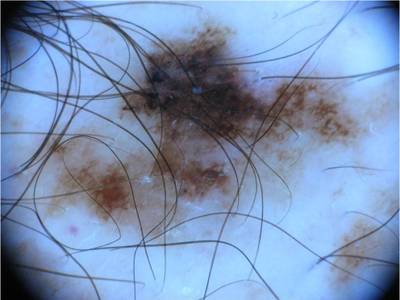

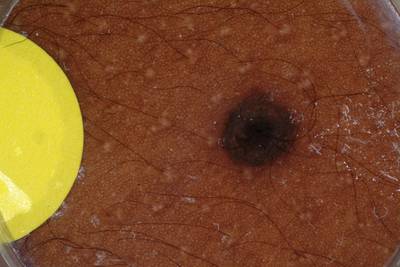
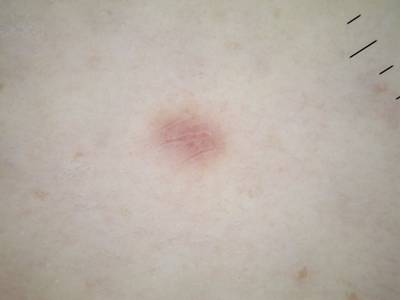
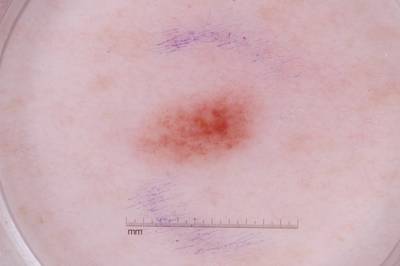
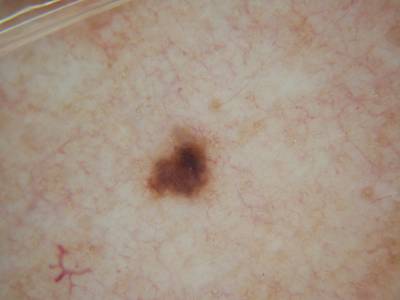

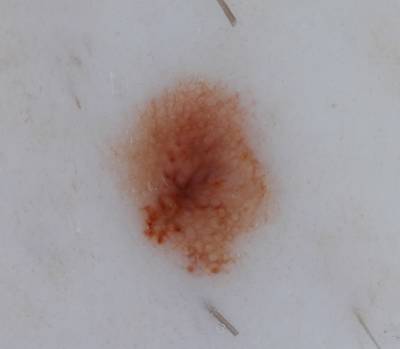
Class balance #
There are 1 annotation classes in the dataset. Find the general statistics and balances for every class in the table below. Click any row to preview images that have labels of the selected class. Sort by column to find the most rare or prevalent classes.
Class ㅤ | Images ㅤ | Objects ㅤ | Count on image average | Area on image average |
---|---|---|---|---|
lesion➔ mask | 2750 | 2750 | 1 | 20.2% |
Images #
Explore every single image in the dataset with respect to the number of annotations of each class it has. Click a row to preview selected image. Sort by any column to find anomalies and edge cases. Use horizontal scroll if the table has many columns for a large number of classes in the dataset.
Object distribution #
Interactive heatmap chart for every class with object distribution shows how many images are in the dataset with a certain number of objects of a specific class. Users can click cell and see the list of all corresponding images.
Class sizes #
The table below gives various size properties of objects for every class. Click a row to see the image with annotations of the selected class. Sort columns to find classes with the smallest or largest objects or understand the size differences between classes.
Class | Object count | Avg area | Max area | Min area | Min height | Min height | Max height | Max height | Avg height | Avg height | Min width | Min width | Max width | Max width |
---|---|---|---|---|---|---|---|---|---|---|---|---|---|---|
lesion mask | 2750 | 20.2% | 95.82% | 0.3% | 97px | 7.83% | 4479px | 100% | 1196px | 53.46% | 114px | 4.62% | 6708px | 100% |
Spatial Heatmap #
The heatmaps below give the spatial distributions of all objects for every class. These visualizations provide insights into the most probable and rare object locations on the image. It helps analyze objects' placements in a dataset.
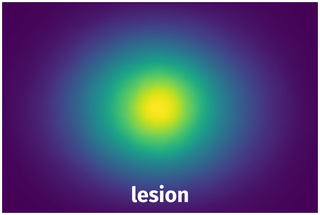
Objects #
Table contains all 2750 objects. Click a row to preview an image with annotations, and use search or pagination to navigate. Sort columns to find outliers in the dataset.
Object ID ㅤ | Class ㅤ | Image name click row to open | Image size height x width | Height ㅤ | Height ㅤ | Width ㅤ | Width ㅤ | Area ㅤ |
---|---|---|---|---|---|---|---|---|
1➔ | lesion mask | ISIC_0013674.jpg | 2848 x 4288 | 2139px | 75.11% | 1919px | 44.75% | 21.77% |
2➔ | lesion mask | ISIC_0013065.jpg | 2592 x 3872 | 415px | 16.01% | 570px | 14.72% | 1.42% |
3➔ | lesion mask | ISIC_0014061.jpg | 2848 x 4288 | 889px | 31.21% | 937px | 21.85% | 4.47% |
4➔ | lesion mask | ISIC_0000245.jpg | 768 x 1024 | 618px | 80.47% | 789px | 77.05% | 49.74% |
5➔ | lesion mask | ISIC_0010219.jpg | 768 x 1024 | 407px | 52.99% | 492px | 48.05% | 19.99% |
6➔ | lesion mask | ISIC_0013554.jpg | 2848 x 4288 | 556px | 19.52% | 503px | 11.73% | 1.86% |
7➔ | lesion mask | ISIC_0000141.jpg | 1536 x 2048 | 683px | 44.47% | 1133px | 55.32% | 17.17% |
8➔ | lesion mask | ISIC_0010011.jpg | 768 x 1024 | 539px | 70.18% | 506px | 49.41% | 25.64% |
9➔ | lesion mask | ISIC_0000501.jpg | 802 x 919 | 443px | 55.24% | 422px | 45.92% | 17.5% |
10➔ | lesion mask | ISIC_0014316.jpg | 2848 x 4288 | 1402px | 49.23% | 1173px | 27.36% | 9.04% |
License #
Citation #
If you make use of the ISIC 2017: Part 1 - Lesion Segmentation data, please cite the following reference:
@misc{codella2018skin,
title={Skin Lesion Analysis Toward Melanoma Detection: A Challenge at the 2017 International Symposium on Biomedical Imaging (ISBI), Hosted by the International Skin Imaging Collaboration (ISIC)},
author={Noel C. F. Codella and David Gutman and M. Emre Celebi and Brian Helba and Michael A. Marchetti and Stephen W. Dusza and Aadi Kalloo and Konstantinos Liopyris and Nabin Mishra and Harald Kittler and Allan Halpern},
year={2018},
eprint={1710.05006},
archivePrefix={arXiv},
primaryClass={cs.CV}
}
If you are happy with Dataset Ninja and use provided visualizations and tools in your work, please cite us:
@misc{ visualization-tools-for-isic-2017-part-dataset,
title = { Visualization Tools for ISIC 2017: Part 1 - Lesion Segmentation Dataset },
type = { Computer Vision Tools },
author = { Dataset Ninja },
howpublished = { \url{ https://datasetninja.com/isic-2017-part-1 } },
url = { https://datasetninja.com/isic-2017-part-1 },
journal = { Dataset Ninja },
publisher = { Dataset Ninja },
year = { 2025 },
month = { may },
note = { visited on 2025-05-15 },
}
Download #
Dataset ISIC 2017: Part 1 - Lesion Segmentation can be downloaded in Supervisely format:
As an alternative, it can be downloaded with dataset-tools package:
pip install --upgrade dataset-tools
… using following python code:
import dataset_tools as dtools
dtools.download(dataset='ISIC 2017: Part 1 - Lesion Segmentation', dst_dir='~/dataset-ninja/')
Make sure not to overlook the python code example available on the Supervisely Developer Portal. It will give you a clear idea of how to effortlessly work with the downloaded dataset.
The data in original format can be downloaded here.
Disclaimer #
Our gal from the legal dep told us we need to post this:
Dataset Ninja provides visualizations and statistics for some datasets that can be found online and can be downloaded by general audience. Dataset Ninja is not a dataset hosting platform and can only be used for informational purposes. The platform does not claim any rights for the original content, including images, videos, annotations and descriptions. Joint publishing is prohibited.
You take full responsibility when you use datasets presented at Dataset Ninja, as well as other information, including visualizations and statistics we provide. You are in charge of compliance with any dataset license and all other permissions. You are required to navigate datasets homepage and make sure that you can use it. In case of any questions, get in touch with us at hello@datasetninja.com.