Introduction #
The Labeled Surgical Tools and Images was created for a master’s thesis project focused on sorting surgical tools from a cluttered tray. The goal was to address object detection and occlusion reasoning. The dataset comprises a total of 3009 images, each accompanied by corresponding labels. These labels categorize the objects into four types: scalpel nº4, straight dissection clamp, straight mayo scissor, or curved mayo scissor. Additionally, each tool is classified as either “on top” (occlusion=False
) or “at the bottom” (occlusion=True
).
The dataset’s inspiration arose from an interview with the Chief Nurse of the Main Operating Room at the Hospital of the University of Coimbra. During the interview, concerns were raised about the time nurses spend sorting surgical tools after disinfection, which could be better utilized for patient care. This dataset was created as part of an effort to develop a robotic system that could streamline this process.
To ensure a balanced division for object detection, the dataset is split into training and test groups. While the standard split ratio in machine learning is 70% for train and 30% for test, the authors stressed the importance of maintaining a balanced proportion of every class within the dataset. To achieve this, they employed a more nuanced approach to determine the division percentages, taking into account the number of images for each combination of tools.
To construct the dataset, the authors took multiple photos of each surgical instrument individually, varying the tray’s rotations, inclinations, and lighting conditions. They used BBox-Label-Tool and YoloMark for this purpose. To account for occlusion, each tool was paired with another of a different class, and the same photographic process was repeated, with one instrument occluding the other. Finally, additional photos were taken with tools from all classes without occlusion.
The division of the dataset into train and test groups was carefully considered to maintain balance, especially for images with two instruments. This involved calculating the optimal number of images for each instrument combination and ensuring that occlusion reasoning data was also appropriately divided.
To facilitate this complex division process, a Python script was developed, randomly sorting the dataset multiple times and selecting images for the test and train groups based on the calculated values.
Overall, the dataset was meticulously created to support the development of robotic systems for surgical tool sorting, addressing the challenges of object detection and occlusion reasoning in this context.
Summary #
Labeled Surgical Tools and Images is a dataset for an object detection task. It is used in the medical industry.
The dataset consists of 2620 images with 3639 labeled objects belonging to 4 different classes including curved mayo scissor, scalpel nº4, straight dissection clamp, and other: straight mayo scissor.
Images in the Labeled Surgical Tools and Images dataset have bounding box annotations. All images are labeled (i.e. with annotations). There are 2 splits in the dataset: train (1834 images) and test (786 images). Additionally, objects with occlusion are marked. Explore them in supervisely labeling tool. The dataset was released in 2018 by the University of Coimbra, Portugal.
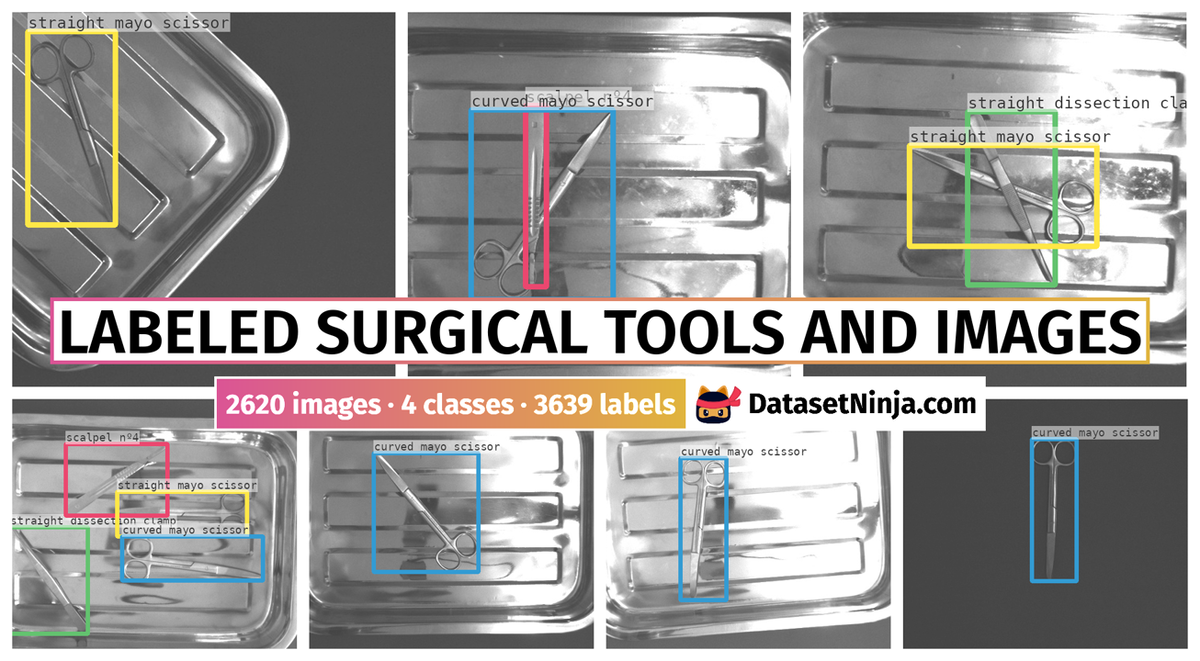
Explore #
Labeled Surgical Tools and Images dataset has 2620 images. Click on one of the examples below or open "Explore" tool anytime you need to view dataset images with annotations. This tool has extended visualization capabilities like zoom, translation, objects table, custom filters and more. Hover the mouse over the images to hide or show annotations.
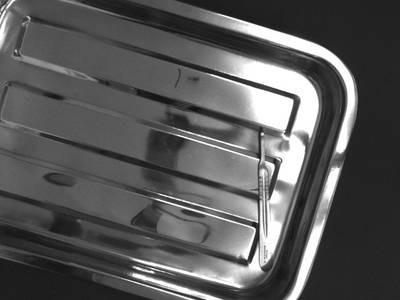
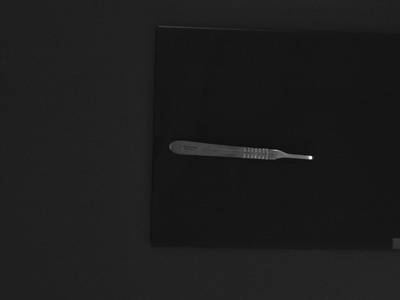
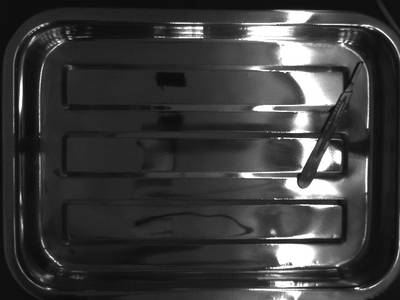
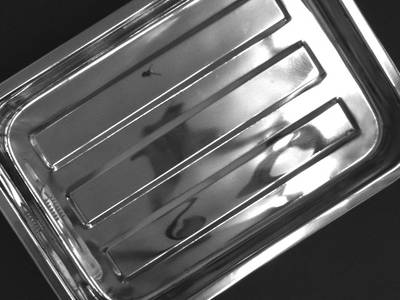
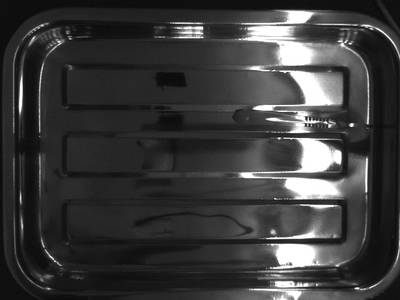
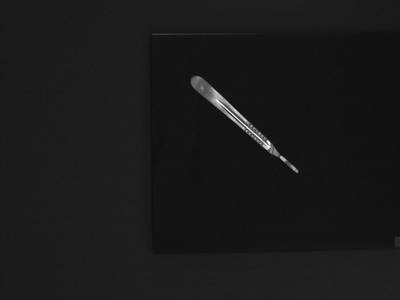
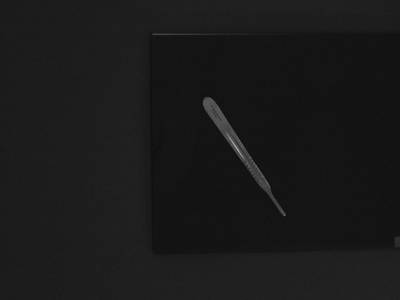
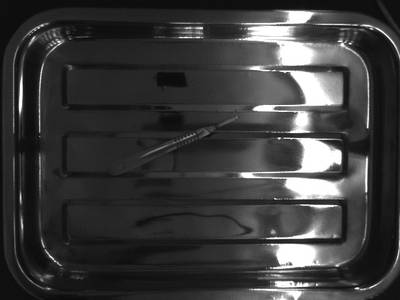
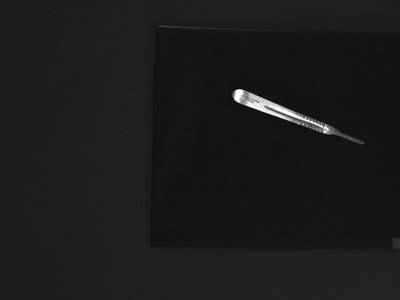
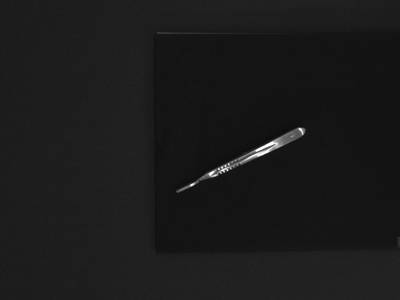
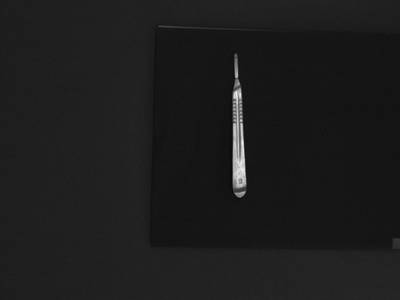
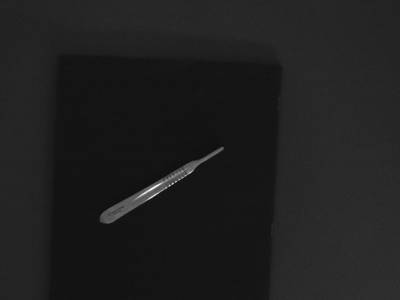
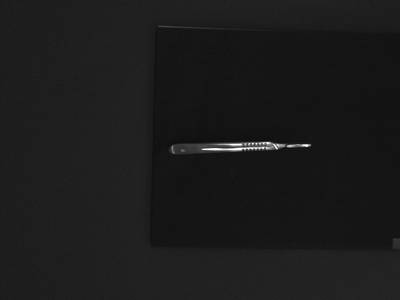
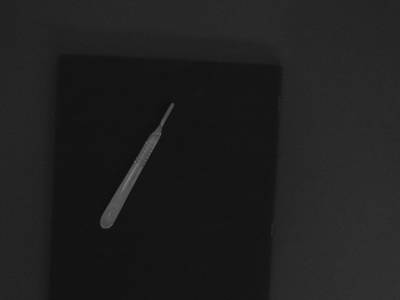
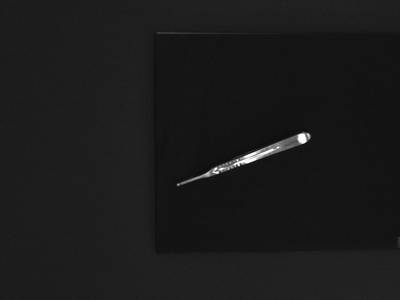

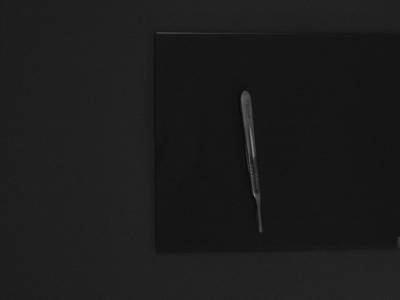
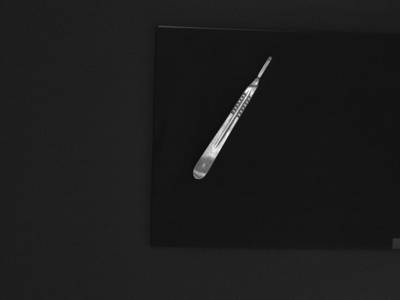
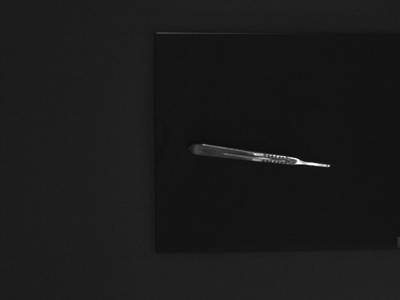
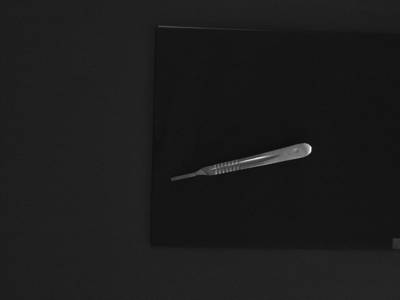

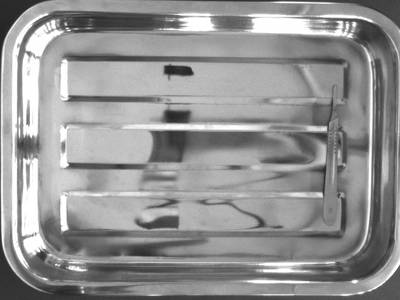
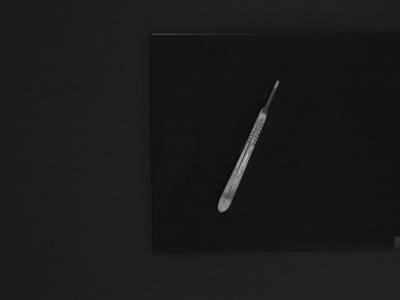
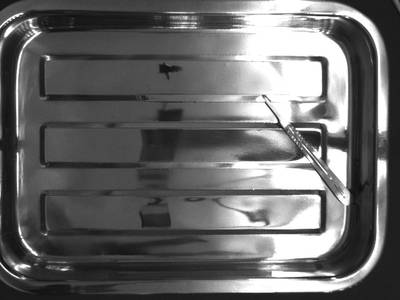
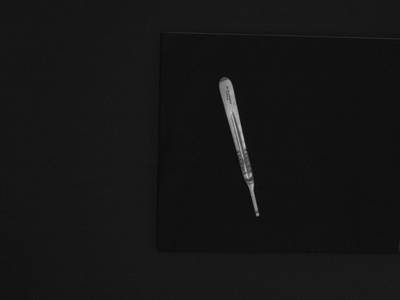
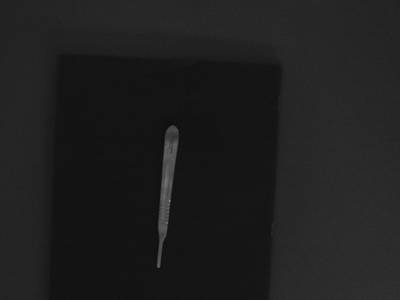
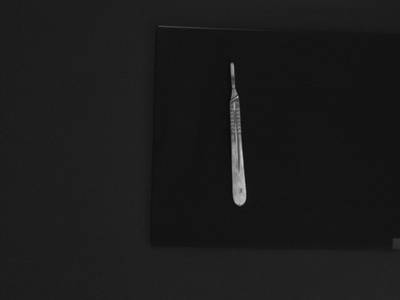

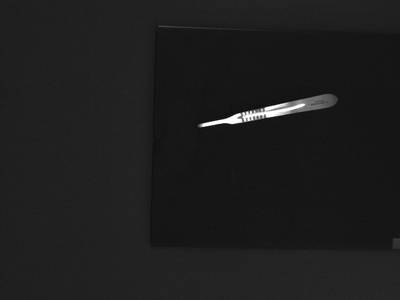
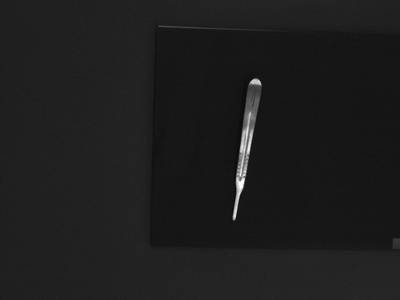
Class balance #
There are 4 annotation classes in the dataset. Find the general statistics and balances for every class in the table below. Click any row to preview images that have labels of the selected class. Sort by column to find the most rare or prevalent classes.
Class ㅤ | Images ㅤ | Objects ㅤ | Count on image average | Area on image average |
---|---|---|---|---|
curved mayo scissor➔ rectangle | 911 | 911 | 1 | 11.78% |
scalpel nº4➔ rectangle | 910 | 910 | 1 | 6.1% |
straight mayo scissor➔ rectangle | 909 | 909 | 1 | 10.1% |
straight dissection clamp➔ rectangle | 909 | 909 | 1 | 7.35% |
Co-occurrence matrix #
Co-occurrence matrix is an extremely valuable tool that shows you the images for every pair of classes: how many images have objects of both classes at the same time. If you click any cell, you will see those images. We added the tooltip with an explanation for every cell for your convenience, just hover the mouse over a cell to preview the description.
Images #
Explore every single image in the dataset with respect to the number of annotations of each class it has. Click a row to preview selected image. Sort by any column to find anomalies and edge cases. Use horizontal scroll if the table has many columns for a large number of classes in the dataset.
Object distribution #
Interactive heatmap chart for every class with object distribution shows how many images are in the dataset with a certain number of objects of a specific class. Users can click cell and see the list of all corresponding images.
Class sizes #
The table below gives various size properties of objects for every class. Click a row to see the image with annotations of the selected class. Sort columns to find classes with the smallest or largest objects or understand the size differences between classes.
Class | Object count | Avg area | Max area | Min area | Min height | Min height | Max height | Max height | Avg height | Avg height | Min width | Min width | Max width | Max width |
---|---|---|---|---|---|---|---|---|---|---|---|---|---|---|
curved mayo scissor rectangle | 911 | 11.79% | 34.25% | 6.85% | 85px | 17.71% | 415px | 86.46% | 209px | 43.59% | 80px | 12.5% | 409px | 63.91% |
scalpel nº4 rectangle | 910 | 6.1% | 20.1% | 1.34% | 18px | 3.75% | 346px | 72.08% | 153px | 31.86% | 19px | 2.97% | 343px | 53.59% |
straight mayo scissor rectangle | 909 | 10.1% | 28.91% | 6.59% | 83px | 17.29% | 376px | 78.33% | 188px | 39.17% | 82px | 12.81% | 376px | 58.75% |
straight dissection clamp rectangle | 909 | 7.35% | 24.43% | 1.24% | 16px | 3.33% | 363px | 75.62% | 169px | 35.17% | 21px | 3.28% | 362px | 56.56% |
Spatial Heatmap #
The heatmaps below give the spatial distributions of all objects for every class. These visualizations provide insights into the most probable and rare object locations on the image. It helps analyze objects' placements in a dataset.

Objects #
Table contains all 3639 objects. Click a row to preview an image with annotations, and use search or pagination to navigate. Sort columns to find outliers in the dataset.
Object ID ㅤ | Class ㅤ | Image name click row to open | Image size height x width | Height ㅤ | Height ㅤ | Width ㅤ | Width ㅤ | Area ㅤ |
---|---|---|---|---|---|---|---|---|
1➔ | straight mayo scissor rectangle | pinca612.jpg | 480 x 640 | 103px | 21.46% | 243px | 37.97% | 8.15% |
2➔ | straight dissection clamp rectangle | pinca612.jpg | 480 x 640 | 235px | 48.96% | 68px | 10.62% | 5.2% |
3➔ | straight dissection clamp rectangle | bisturi552.jpg | 480 x 640 | 91px | 18.96% | 241px | 37.66% | 7.14% |
4➔ | scalpel nº4 rectangle | bisturi552.jpg | 480 x 640 | 208px | 43.33% | 113px | 17.66% | 7.65% |
5➔ | scalpel nº4 rectangle | bisturi88.jpg | 480 x 640 | 165px | 34.38% | 173px | 27.03% | 9.29% |
6➔ | scalpel nº4 rectangle | bisturi272.jpg | 480 x 640 | 180px | 37.5% | 155px | 24.22% | 9.08% |
7➔ | scalpel nº4 rectangle | bisturi654.jpg | 480 x 640 | 35px | 7.29% | 229px | 35.78% | 2.61% |
8➔ | straight mayo scissor rectangle | bisturi654.jpg | 480 x 640 | 221px | 46.04% | 173px | 27.03% | 12.45% |
9➔ | straight mayo scissor rectangle | tesourareta550.jpg | 480 x 640 | 255px | 53.12% | 94px | 14.69% | 7.8% |
10➔ | scalpel nº4 rectangle | tesourareta550.jpg | 480 x 640 | 53px | 11.04% | 231px | 36.09% | 3.99% |
License #
Citation #
If you make use of the Labeled Surgical Tools and Images data, please cite the following reference:
@dataset{Labeled Surgical Tools and Images,
author={Diana Lavado and Joaquim da Silva and Francisco Caramelo},
title={Labeled Surgical Tools and Images},
year={2018},
url={https://www.kaggle.com/datasets/dilavado/labeled-surgical-tools}
}
If you are happy with Dataset Ninja and use provided visualizations and tools in your work, please cite us:
@misc{ visualization-tools-for-labeled-surgical-tools-and-images-dataset,
title = { Visualization Tools for Labeled Surgical Tools and Images Dataset },
type = { Computer Vision Tools },
author = { Dataset Ninja },
howpublished = { \url{ https://datasetninja.com/labeled-surgical-tools-and-images } },
url = { https://datasetninja.com/labeled-surgical-tools-and-images },
journal = { Dataset Ninja },
publisher = { Dataset Ninja },
year = { 2025 },
month = { jul },
note = { visited on 2025-07-24 },
}
Download #
Dataset Labeled Surgical Tools and Images can be downloaded in Supervisely format:
As an alternative, it can be downloaded with dataset-tools package:
pip install --upgrade dataset-tools
… using following python code:
import dataset_tools as dtools
dtools.download(dataset='Labeled Surgical Tools and Images', dst_dir='~/dataset-ninja/')
Make sure not to overlook the python code example available on the Supervisely Developer Portal. It will give you a clear idea of how to effortlessly work with the downloaded dataset.
The data in original format can be downloaded here.
Disclaimer #
Our gal from the legal dep told us we need to post this:
Dataset Ninja provides visualizations and statistics for some datasets that can be found online and can be downloaded by general audience. Dataset Ninja is not a dataset hosting platform and can only be used for informational purposes. The platform does not claim any rights for the original content, including images, videos, annotations and descriptions. Joint publishing is prohibited.
You take full responsibility when you use datasets presented at Dataset Ninja, as well as other information, including visualizations and statistics we provide. You are in charge of compliance with any dataset license and all other permissions. You are required to navigate datasets homepage and make sure that you can use it. In case of any questions, get in touch with us at hello@datasetninja.com.