Introduction #
The authors present the first maritime panoptic obstacle detection benchmark LaRS: Lakes Rivers and Seas dataset , featuring scenes from lakes, rivers and seas. The progress in maritime obstacle detection is hindered by the lack of a diverse dataset that adequately captures the complexity of general maritime environments. Today over 90% of goods being moved over water, substantial efforts are being invested in development of autonomous unmanned surface vessels (USV). The autonomy of USVs critically depends on obstacle detection capability for timely collision avoidance. There are several challenges associated with maritime obstacle detection. The appearance of the navigable surface (water) is dynamic and reflects the environment, often containing strong mirroring and sun glitter.
LaRS features diverse and challenging USV-centric scenes with per-pixel panoptic annotations (right).
Although modern detectors can accurately detect common dynamic obstacles such as ships and boats, the appearance of obstacles such as buoys, people and animals can vary significantly, bringing the task closer to anomaly detection. Furthermore, background static obstacles, such as shorelines and piers, cannot be addressed by these methods. The currently dominant approach instead employs semantic segmentation to decompose the scene into three semantic classes (water, obstacles and sky), which jointly address static and dynamic obstacles. The recent detection benchmark indicates that segmentation methods could benefit from the detection approach. A natural approach that combines these two principles is panoptic segmentation, which has proven highly effective in the related field of autonomous ground vehicles. Unfortunately, panoptic segmentation has not been fully explored for maritime perception, primarily due to the lack of a diverse, publicly available, curated panoptic dataset.
Dataset creation and description
The authors proposes the first maritime panoptic obstacle detection benchmark. LaRS surpasses existing datasets in terms of diversity, obstacle types and acquisition conditions. The dataset is composed of over 4000 key frames with panoptic labels for 3 stuff and 8 thing categories, and 19 global scene attributes. Each key frame is equipped with the preceding nine frames to facilitate the development of methods that exploit temporal texture. To ensure equal attribute distribution, the training, validation, and test splits were carefully constructed.
A wide range of sources was considered to ensure the visual diversity of LaRS. Specifically, the authors collected scenes from public online videos featuring various activities captured from boats around the world, recorded new sequences in a number of different geographic locations ourselves and included the most challenging scenes from existing maritime datasets. The collection of public videos was guided using search prompts related to underrepresented scenes in the existing datasets. This includes canals (e.g. canal tour), exotic locations (e.g. tropic boat tour, polar kayaking), crowded scenes (e.g. boat parade), strong reflections (e.g. still lake), and poor visibility conditions (e.g. boat ride in the rain, night-time boat ride). At least one key frame was extracted from each of the collected 396 sequences, to ensure visual diversity. The authors manually inspected the predicted segmentation and included examples with failures such as false negative obstacle segmentation and false positives on reflections to increase the difficulty level. In this way, a set of 897 representative key frames spanning diverse and challenging scenes was selected. Next, they manually recorded videos at various locations on lakes, rivers and seas. From these, they identified 494 challenging sequences, and using the same process as for online videos, the authors identified 1354 diverse and challenging key frames. The total number of images in LaRS is thus over 40k. Faces were de-identified in all frames by running a face detector and blurring, followed by manual inspection.
Dataset annotation
All 4k selected key frames were manually annotated with per-pixel panoptic labels by a professional labeling company. In particular, water, sky and
static obstacles like shores and piers were annotated as stuff classes, while the dynamic obstacles instances were segmented and classified into 8 different object categories: boat, row boat, paddle board, buoy, swimmer, animal, float and an open-world other class to cover the remaining obstacles. Following a standard practice group labels were used to group multiple hard-to-delineate neighbouring instances of the same category. Regions that could not be reliably manually segmented were labeled with the ignore class.
LaRS frames are labeled with 19 global attributes relevant for navigation. Mutually exclusive and mutually nonexclusive groups are indicated in blue and green, respectively. The numbers indicate the amount of frames in the dataset.
Statistics of dynamic obstacle classes in LaRS (left) with respect to their size (right)…
Global attributes were assigned to key frames, to indicate environment type, illumination conditions, presence of reflections, surface roughness and scene conditions: scene type, lighting, reflections, waves, extra dark, extra bright, glitter, dirty lens, wakes, rain, fog, plants debris images tags.
Annotation correctness was further analyzed to ensure the highest quality of the dataset. In the first pass, state-ofthe-art semantic segmentation and panoptic segmentatation methods were trained and run on the entire dataset to identify major annotation errors. The authors manually inspected all ground truth instance labels of the dynamic obstacles and identified and corrected approximately 3600 annotation errors.
Summary #
LaRS: Lakes Rivers and Seas dataset is a dataset for instance segmentation, semantic segmentation, and object detection tasks. It is used in the marine industry.
The dataset consists of 53301 images with 41852 labeled objects belonging to 12 different classes including water, obstacle, static obstacle, and other: sky, boat/ship, buoy, row boats, other, swimmer, paddle board, animal, and float.
Images in the LaRS dataset have pixel-level instance segmentation and bounding box annotations. Due to the nature of the instance segmentation task, it can be automatically transformed into a semantic segmentation task (only one mask for every class). There are 50498 (95% of the total) unlabeled images (i.e. without annotations). There are 3 splits in the dataset: train (32375 images), test (17966 images), and val (2960 images). Additionally, the images have seq name and seq id tags, that help associate every image with a parent sequence. Each of 8 dynamic obstacle labels has supercategory tag. The dataset was released in 2023 by the University of Ljubljana, Slovenia.
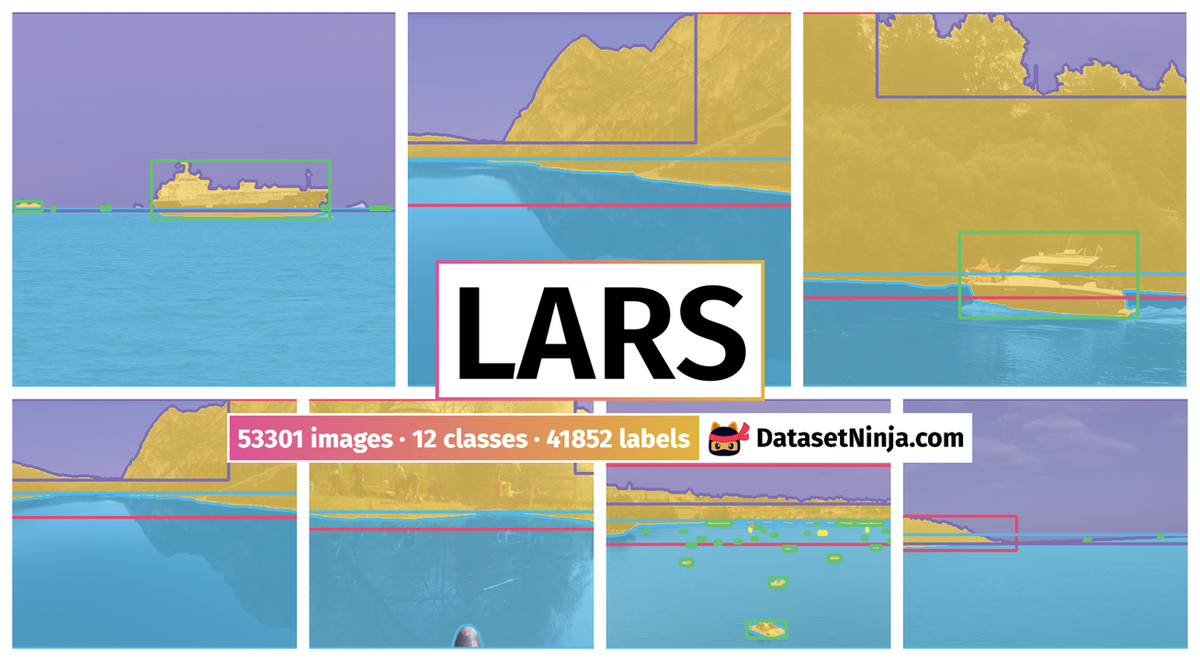
Explore #
LaRS dataset has 53301 images. Click on one of the examples below or open "Explore" tool anytime you need to view dataset images with annotations. This tool has extended visualization capabilities like zoom, translation, objects table, custom filters and more. Hover the mouse over the images to hide or show annotations.
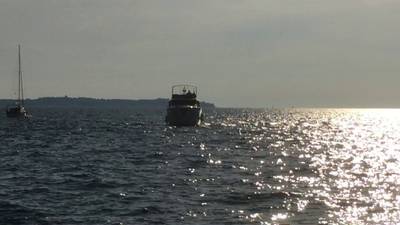
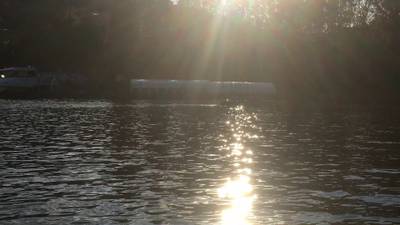
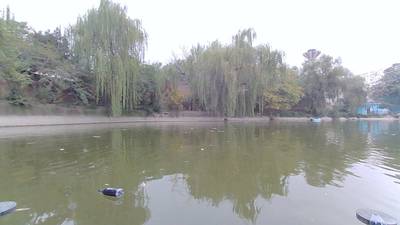
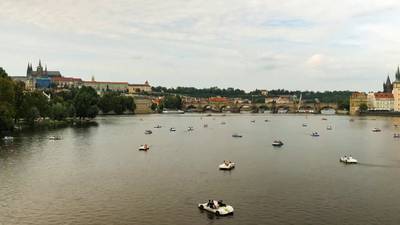
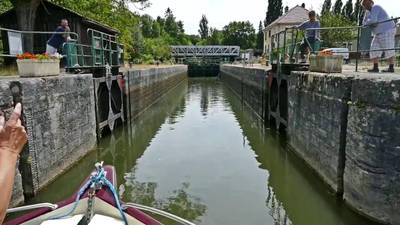
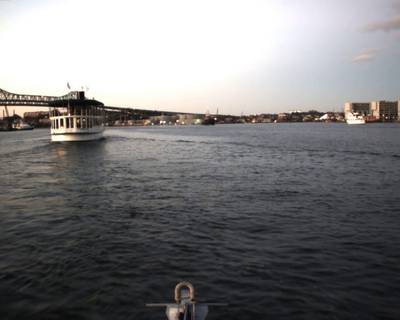

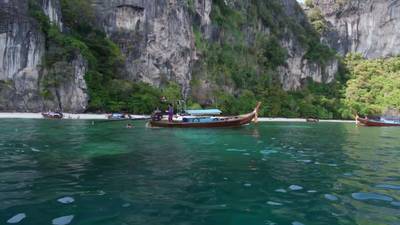
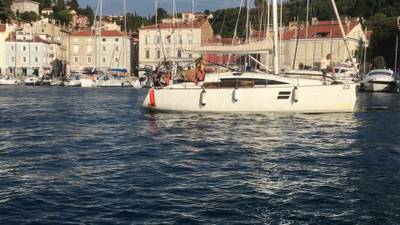
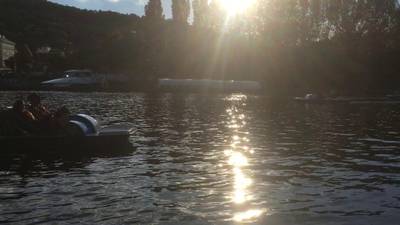
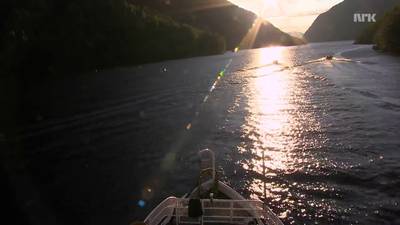
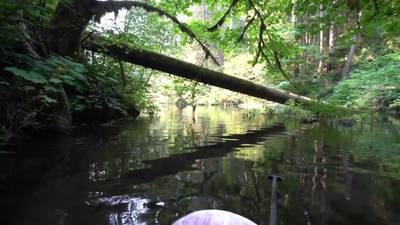
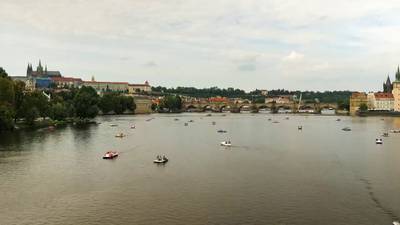
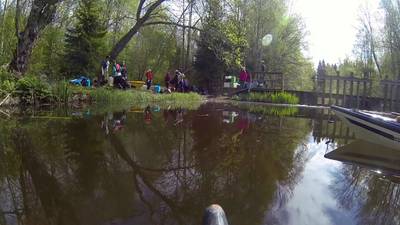
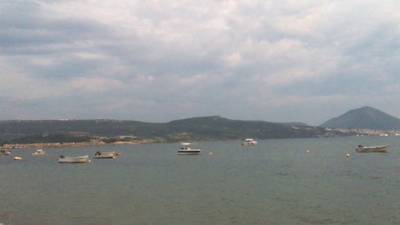
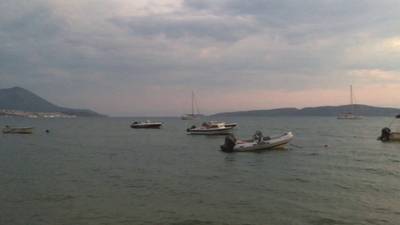
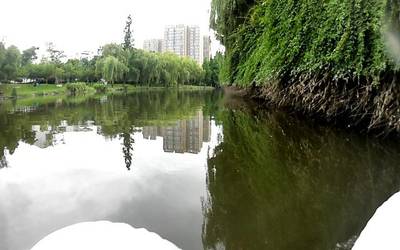
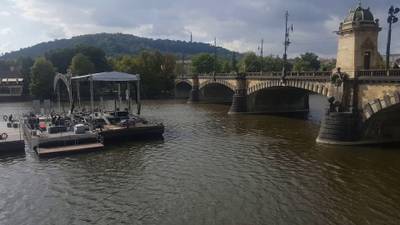
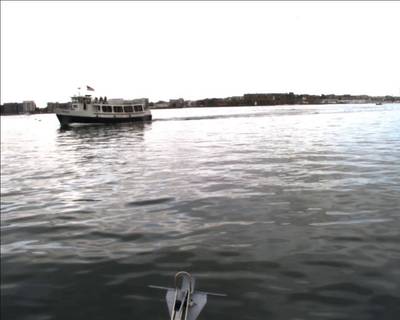
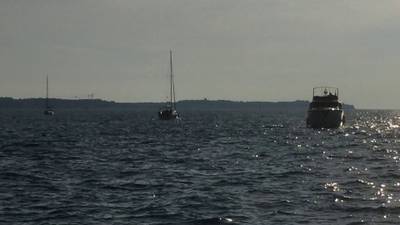


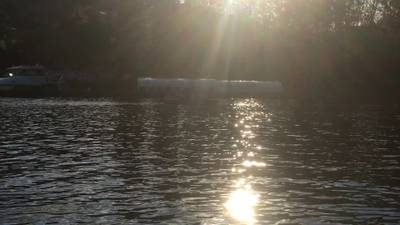
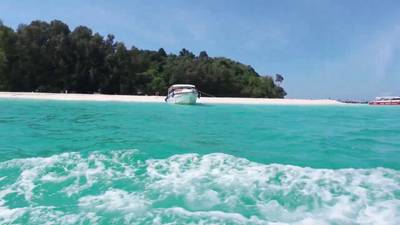
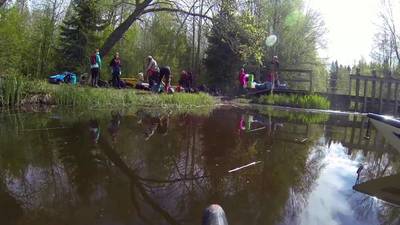

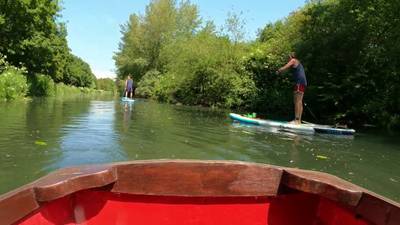
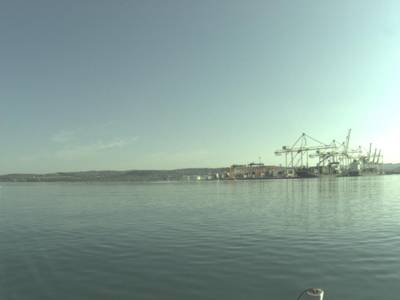
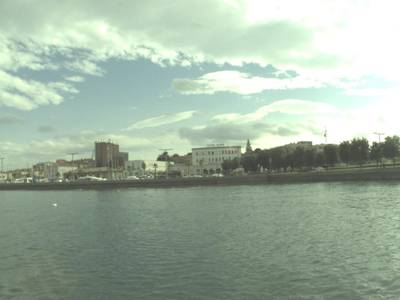
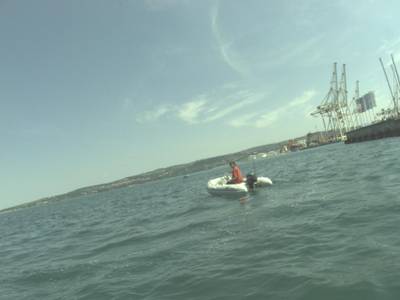
Class balance #
There are 12 annotation classes in the dataset. Find the general statistics and balances for every class in the table below. Click any row to preview images that have labels of the selected class. Sort by column to find the most rare or prevalent classes.
Class ㅤ | Images ㅤ | Objects ㅤ | Count on image average | Area on image average |
---|---|---|---|---|
water➔ any | 2803 | 9282 | 3.31 | 49.32% |
obstacle➔ any | 2791 | 8504 | 3.05 | 20.06% |
static obstacle➔ rectangle | 2718 | 2718 | 1 | 43.84% |
sky➔ any | 2668 | 10773 | 4.04 | 45.49% |
boat/ship➔ rectangle | 1666 | 6846 | 4.11 | 6.32% |
buoy➔ rectangle | 844 | 1757 | 2.08 | 0.29% |
row boats➔ rectangle | 256 | 480 | 1.88 | 1.51% |
other➔ rectangle | 253 | 463 | 1.83 | 0.07% |
swimmer➔ rectangle | 138 | 414 | 3 | 0.32% |
paddle board➔ rectangle | 126 | 184 | 1.46 | 1.14% |
Co-occurrence matrix #
Co-occurrence matrix is an extremely valuable tool that shows you the images for every pair of classes: how many images have objects of both classes at the same time. If you click any cell, you will see those images. We added the tooltip with an explanation for every cell for your convenience, just hover the mouse over a cell to preview the description.
Images #
Explore every single image in the dataset with respect to the number of annotations of each class it has. Click a row to preview selected image. Sort by any column to find anomalies and edge cases. Use horizontal scroll if the table has many columns for a large number of classes in the dataset.
Class sizes #
The table below gives various size properties of objects for every class. Click a row to see the image with annotations of the selected class. Sort columns to find classes with the smallest or largest objects or understand the size differences between classes.
Class | Object count | Avg area | Max area | Min area | Min height | Min height | Max height | Max height | Avg height | Avg height | Min width | Min width | Max width | Max width |
---|---|---|---|---|---|---|---|---|---|---|---|---|---|---|
sky any | 10773 | 20.61% | 95.72% | 0% | 1px | 0.09% | 995px | 95.72% | 251px | 26.69% | 1px | 0.05% | 2208px | 100% |
water any | 9282 | 28.06% | 100% | 0% | 1px | 0.14% | 1204px | 100% | 291px | 31.32% | 1px | 0.08% | 2208px | 100% |
obstacle any | 8504 | 6.58% | 97.8% | 0% | 1px | 0.14% | 1242px | 100% | 139px | 15.52% | 1px | 0.05% | 2208px | 100% |
boat/ship rectangle | 6846 | 1.66% | 100% | 0% | 2px | 0.21% | 1080px | 100% | 77px | 8.2% | 2px | 0.16% | 1920px | 100% |
static obstacle rectangle | 2718 | 43.84% | 100% | 0.04% | 11px | 1.2% | 1080px | 100% | 405px | 45.49% | 31px | 1.61% | 2208px | 100% |
buoy rectangle | 1757 | 0.14% | 48.32% | 0% | 2px | 0.21% | 945px | 98.64% | 25px | 2.58% | 3px | 0.16% | 1007px | 52.45% |
row boats rectangle | 480 | 0.82% | 23.22% | 0% | 5px | 0.48% | 412px | 37.69% | 49px | 4.82% | 5px | 0.23% | 1202px | 93.91% |
other rectangle | 463 | 0.04% | 1.55% | 0% | 2px | 0.21% | 112px | 16.25% | 13px | 1.46% | 3px | 0.16% | 218px | 17.06% |
swimmer rectangle | 414 | 0.11% | 8.24% | 0% | 3px | 0.28% | 260px | 26.39% | 25px | 2.56% | 3px | 0.21% | 657px | 34.22% |
animal rectangle | 396 | 0.03% | 1.72% | 0% | 3px | 0.28% | 143px | 13.24% | 13px | 1.24% | 3px | 0.21% | 250px | 13.02% |
Spatial Heatmap #
The heatmaps below give the spatial distributions of all objects for every class. These visualizations provide insights into the most probable and rare object locations on the image. It helps analyze objects' placements in a dataset.
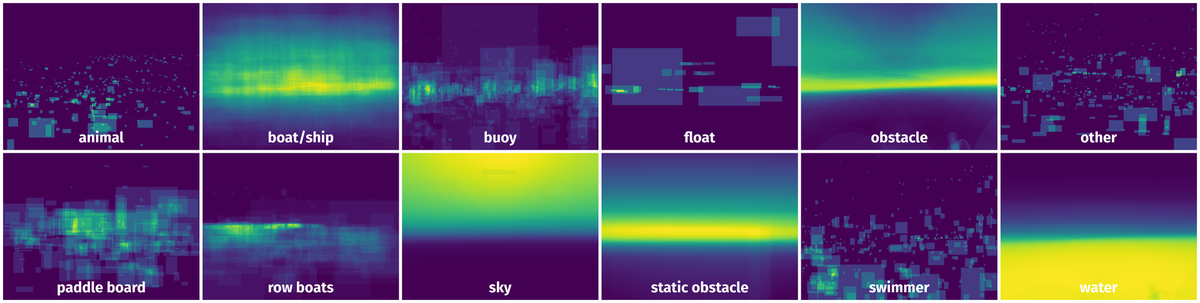
Objects #
Table contains all 41852 objects. Click a row to preview an image with annotations, and use search or pagination to navigate. Sort columns to find outliers in the dataset.
Object ID ㅤ | Class ㅤ | Image name click row to open | Image size height x width | Height ㅤ | Height ㅤ | Width ㅤ | Width ㅤ | Area ㅤ |
---|---|---|---|---|---|---|---|---|
1➔ | obstacle any | inhouse_seq_381_00123.jpg | 1080 x 1920 | 263px | 24.35% | 1920px | 100% | 19.16% |
2➔ | obstacle any | inhouse_seq_381_00123.jpg | 1080 x 1920 | 379px | 35.09% | 841px | 43.8% | 7.01% |
3➔ | obstacle any | inhouse_seq_381_00123.jpg | 1080 x 1920 | 21px | 1.94% | 29px | 1.51% | 0.02% |
4➔ | obstacle any | inhouse_seq_381_00123.jpg | 1080 x 1920 | 41px | 3.8% | 36px | 1.88% | 0.05% |
5➔ | water any | inhouse_seq_381_00123.jpg | 1080 x 1920 | 14px | 1.3% | 24px | 1.25% | 0.01% |
6➔ | water any | inhouse_seq_381_00123.jpg | 1080 x 1920 | 848px | 78.52% | 1920px | 100% | 68.58% |
7➔ | water any | inhouse_seq_381_00123.jpg | 1080 x 1920 | 115px | 10.65% | 154px | 8.02% | 0.58% |
8➔ | water any | inhouse_seq_381_00123.jpg | 1080 x 1920 | 27px | 2.5% | 26px | 1.35% | 0.01% |
9➔ | water any | inhouse_seq_381_00123.jpg | 1080 x 1920 | 18px | 1.67% | 19px | 0.99% | 0% |
10➔ | water any | inhouse_seq_381_00123.jpg | 1080 x 1920 | 141px | 13.06% | 113px | 5.89% | 0.35% |
License #
LaRS: Lakes Rivers and Seas dataset is under CC BY-NC 4.0 license.
Citation #
If you make use of the LaRS data, please cite the following reference:
@InProceedings{Zust2023LaRS,
title={LaRS: A Diverse Panoptic Maritime Obstacle Detection Dataset and Benchmark},
author={{\v{Z}}ust, Lojze and Per{\v{s}}, Janez and Kristan, Matej},
booktitle={International Conference on Computer Vision (ICCV)},
year={2023}
}
If you are happy with Dataset Ninja and use provided visualizations and tools in your work, please cite us:
@misc{ visualization-tools-for-lars-dataset,
title = { Visualization Tools for LaRS Dataset },
type = { Computer Vision Tools },
author = { Dataset Ninja },
howpublished = { \url{ https://datasetninja.com/lars } },
url = { https://datasetninja.com/lars },
journal = { Dataset Ninja },
publisher = { Dataset Ninja },
year = { 2025 },
month = { feb },
note = { visited on 2025-02-22 },
}
Download #
Dataset LaRS can be downloaded in Supervisely format:
As an alternative, it can be downloaded with dataset-tools package:
pip install --upgrade dataset-tools
… using following python code:
import dataset_tools as dtools
dtools.download(dataset='LaRS', dst_dir='~/dataset-ninja/')
Make sure not to overlook the python code example available on the Supervisely Developer Portal. It will give you a clear idea of how to effortlessly work with the downloaded dataset.
The data in original format can be downloaded here:
Disclaimer #
Our gal from the legal dep told us we need to post this:
Dataset Ninja provides visualizations and statistics for some datasets that can be found online and can be downloaded by general audience. Dataset Ninja is not a dataset hosting platform and can only be used for informational purposes. The platform does not claim any rights for the original content, including images, videos, annotations and descriptions. Joint publishing is prohibited.
You take full responsibility when you use datasets presented at Dataset Ninja, as well as other information, including visualizations and statistics we provide. You are in charge of compliance with any dataset license and all other permissions. You are required to navigate datasets homepage and make sure that you can use it. In case of any questions, get in touch with us at hello@datasetninja.com.