Introduction #
The authors of the Magnetic Tile Surface Defect Dataset explore the significance of magnetic tiles in engines, where maintaining a productive automatic assembly line for magnetic tile manufacturing remains a fundamental challenge. It consists of 1344 images with the following defect types: blowhole, crack, fray, break, uneven (caused by the grinding process), and images with no defects.
Surface defect detection is crucial for filtering out subpar products, yet this process often involves significant manual effort. In Zhejiang Province, China, the largest magnetic tile production base globally, approximately three-quarters of the workforce is engaged in manual product quality inspections. In response to this, various image processing techniques, such as those based on grayscale value, gradient edge, wavelet, curvelet, and shearlet transformations, have been proposed to automate inspection tasks. Recently, deep neural network models have gained prominence for surface defect detection, demonstrating state-of-the-art performance in classification tasks. These advancements underscore the feasibility of employing computer vision for surface examinations and the potential to reduce reliance on human labor.
To delve deeper into the possibilities, authors suggest a real-time model called MCuePush U-Net, specifically designed for saliency detection of surface defect. Magnetic tiles typically feature 4β6 curved surfaces, causing image distortion. Challenges in automatic damage detection include the complexity of texture, the variety of defect shapes, and the randomness of illumination conditions, contributing to grayscale image noise. Target defects, such as blowholes, cracks, breaks, and frays, often manifest on curved surfaces without fixed patterns, introducing randomness to image detection tasks. Overcoming these challenges is essential for building robust computer vision models, particularly when aiming for real-time performance in the magnetic tile industry.
In image-based surface detection for magnetic tiles, the focus is primarily on minimizing the interference of product texture. While embedding denoising techniques like wavelet, curvelet, and shearlet transformations can effectively extract desirable features, they also lead to extended processing times, far from meeting real-time standards. Notably, the performance of experienced maintenance workers still surpasses machinery in terms of detection accuracy and efficiency. Transitioning from manual detection to automated surface inspection (ASI) requires substantial improvements in computer vision model performance.
For the dataset and evaluation metrics, a total of 1344 images are captured, and the region of interest (ROI) of magnetic tiles is cropped and classified into six datasets based on defect types: blowhole, crack, fray, break, uneven (caused by the grinding process), and free (no defects), each with pixel-level labels. To simulate real assembly line conditions, images of a given magnetic tile are collected under multiple illumination conditions.
Summary #
Magnetic Tile Surface Defect Dataset is a dataset for instance segmentation, semantic segmentation, and object detection tasks. It is used in the manufacturing industry, and in the surface defect detection domain.
The dataset consists of 1344 images with 459 labeled objects belonging to 5 different classes including blowhole, uneven, break, and other: crack and fray.
Images in the Magnetic Tile Surface Defect dataset have pixel-level instance segmentation annotations. Due to the nature of the instance segmentation task, it can be automatically transformed into a semantic segmentation (only one mask for every class) or object detection (bounding boxes for every object) tasks. There are 956 (71% of the total) unlabeled images (i.e. without annotations). There are no pre-defined train/val/test splits in the dataset. Additionally, every image has experiment tag. The dataset was released in 2018 by the Chinese Academy of Sciences and Columbia University, USA.

Explore #
Magnetic Tile Surface Defect dataset has 1344 images. Click on one of the examples below or open "Explore" tool anytime you need to view dataset images with annotations. This tool has extended visualization capabilities like zoom, translation, objects table, custom filters and more. Hover the mouse over the images to hide or show annotations.


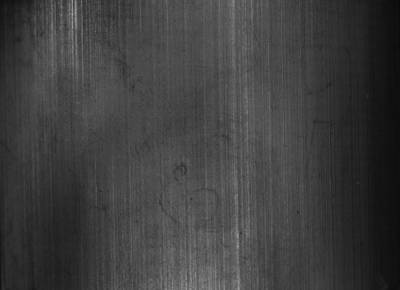
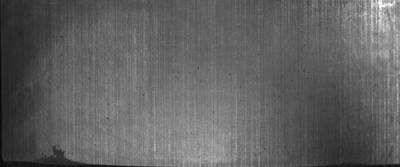

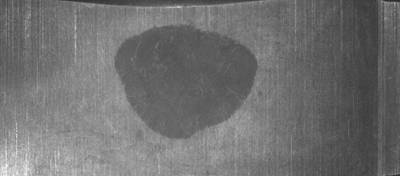


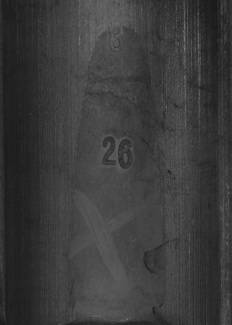




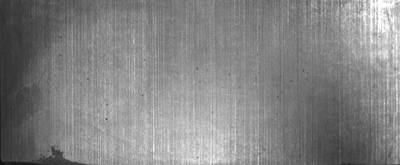

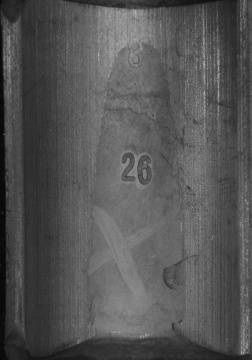


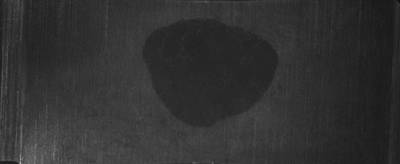





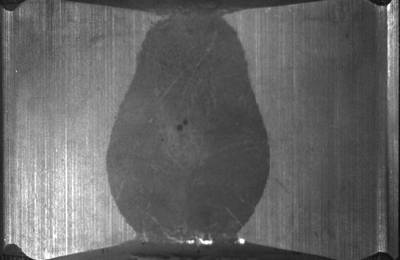



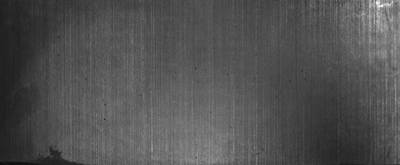

Class balance #
There are 5 annotation classes in the dataset. Find the general statistics and balances for every class in the table below. Click any row to preview images that have labels of the selected class. Sort by column to find the most rare or prevalent classes.
Class γ
€ | Images γ
€ | Objects γ
€ | Count on image average | Area on image average |
---|---|---|---|---|
blowholeβ mask | 115 | 116 | 1.01 | 0.23% |
unevenβ mask | 99 | 108 | 1.09 | 25.24% |
breakβ mask | 85 | 128 | 1.51 | 4.53% |
crackβ mask | 57 | 70 | 1.23 | 0.72% |
frayβ mask | 32 | 37 | 1.16 | 17.47% |
Co-occurrence matrix #
Co-occurrence matrix is an extremely valuable tool that shows you the images for every pair of classes: how many images have objects of both classes at the same time. If you click any cell, you will see those images. We added the tooltip with an explanation for every cell for your convenience, just hover the mouse over a cell to preview the description.
Images #
Explore every single image in the dataset with respect to the number of annotations of each class it has. Click a row to preview selected image. Sort by any column to find anomalies and edge cases. Use horizontal scroll if the table has many columns for a large number of classes in the dataset.
Object distribution #
Interactive heatmap chart for every class with object distribution shows how many images are in the dataset with a certain number of objects of a specific class. Users can click cell and see the list of all corresponding images.
Class sizes #
The table below gives various size properties of objects for every class. Click a row to see the image with annotations of the selected class. Sort columns to find classes with the smallest or largest objects or understand the size differences between classes.
Class | Object count | Avg area | Max area | Min area | Min height | Min height | Max height | Max height | Avg height | Avg height | Min width | Min width | Max width | Max width |
---|---|---|---|---|---|---|---|---|---|---|---|---|---|---|
break mask | 128 | 3.01% | 23.55% | 0.01% | 2px | 0.64% | 263px | 100% | 59px | 20.46% | 3px | 0.66% | 263px | 100% |
blowhole mask | 116 | 0.23% | 0.72% | 0.03% | 7px | 2.13% | 33px | 9.38% | 15px | 4.85% | 5px | 1.76% | 34px | 17.54% |
uneven mask | 108 | 23.14% | 49.81% | 0.01% | 3px | 0.85% | 195px | 63.11% | 94px | 30.68% | 5px | 1.15% | 620px | 100% |
crack mask | 70 | 0.58% | 3.36% | 0.07% | 6px | 1.54% | 268px | 98.11% | 78px | 26.39% | 6px | 0.99% | 40px | 32.79% |
fray mask | 37 | 15.11% | 44.5% | 0.11% | 18px | 4.84% | 355px | 100% | 172px | 59.55% | 16px | 3.28% | 423px | 100% |
Spatial Heatmap #
The heatmaps below give the spatial distributions of all objects for every class. These visualizations provide insights into the most probable and rare object locations on the image. It helps analyze objects' placements in a dataset.
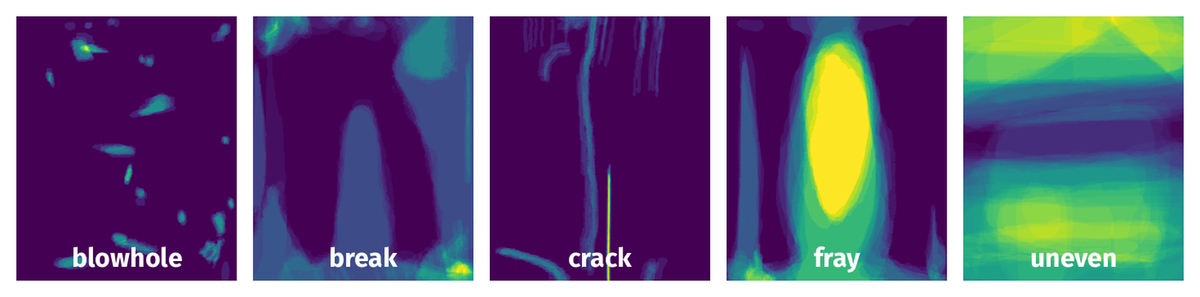
Objects #
Table contains all 459 objects. Click a row to preview an image with annotations, and use search or pagination to navigate. Sort columns to find outliers in the dataset.
Object ID γ
€ | Class γ
€ | Image name click row to open | Image size height x width | Height γ
€ | Height γ
€ | Width γ
€ | Width γ
€ | Area γ
€ |
---|---|---|---|---|---|---|---|---|
1β | uneven mask | exp3_num_186885.jpg | 317 x 195 | 187px | 58.99% | 177px | 90.77% | 46.68% |
2β | break mask | exp2_num_356745.jpg | 378 x 504 | 20px | 5.29% | 61px | 12.1% | 0.43% |
3β | blowhole mask | exp3_num_40438.jpg | 290 x 116 | 10px | 3.45% | 16px | 13.79% | 0.39% |
4β | break mask | exp4_num_348673.jpg | 378 x 516 | 28px | 7.41% | 35px | 6.78% | 0.21% |
5β | uneven mask | exp3_num_24829.jpg | 336 x 524 | 4px | 1.19% | 6px | 1.15% | 0.01% |
6β | uneven mask | exp3_num_24829.jpg | 336 x 524 | 3px | 0.89% | 6px | 1.15% | 0.01% |
7β | uneven mask | exp1_num_28341.jpg | 333 x 522 | 83px | 24.92% | 174px | 33.33% | 3.89% |
8β | blowhole mask | exp5_num_291077.jpg | 356 x 295 | 33px | 9.27% | 34px | 11.53% | 0.46% |
9β | blowhole mask | exp6_num_54366.jpg | 276 x 125 | 21px | 7.61% | 13px | 10.4% | 0.54% |
10β | blowhole mask | exp1_num_108889.jpg | 373 x 248 | 23px | 6.17% | 9px | 3.63% | 0.15% |
License #
It is welcomed to use our dataset. And if it is used in your research, please cite our paper.
A toolbox for surface defects saliency detection can be reach at https://github.com/abin24/Saliency-detection-toolbox. In which our MCue and 14 other saliency detection models are available.
Citation #
If you make use of the Magnetic Tile Surface Defect data, please cite the following reference:
@dataset{Magnetic Tile Surface Defect,
author={Yibin Huang and Congying Qiu and Kui Yuan},
title={Magnetic Tile Surface Defect Dataset},
year={2018},
url={https://github.com/abin24/Magnetic-tile-defect-datasets.}
}
If you are happy with Dataset Ninja and use provided visualizations and tools in your work, please cite us:
@misc{ visualization-tools-for-magnetic-tile-surface-defect-dataset,
title = { Visualization Tools for Magnetic Tile Surface Defect Dataset },
type = { Computer Vision Tools },
author = { Dataset Ninja },
howpublished = { \url{ https://datasetninja.com/magnetic-tile-surface-defect } },
url = { https://datasetninja.com/magnetic-tile-surface-defect },
journal = { Dataset Ninja },
publisher = { Dataset Ninja },
year = { 2025 },
month = { apr },
note = { visited on 2025-04-04 },
}
Download #
Dataset Magnetic Tile Surface Defect can be downloaded in Supervisely format:
As an alternative, it can be downloaded with dataset-tools package:
pip install --upgrade dataset-tools
β¦ using following python code:
import dataset_tools as dtools
dtools.download(dataset='Magnetic Tile Surface Defect', dst_dir='~/dataset-ninja/')
Make sure not to overlook the python code example available on the Supervisely Developer Portal. It will give you a clear idea of how to effortlessly work with the downloaded dataset.
The data in original format can be downloaded here.
Disclaimer #
Our gal from the legal dep told us we need to post this:
Dataset Ninja provides visualizations and statistics for some datasets that can be found online and can be downloaded by general audience. Dataset Ninja is not a dataset hosting platform and can only be used for informational purposes. The platform does not claim any rights for the original content, including images, videos, annotations and descriptions. Joint publishing is prohibited.
You take full responsibility when you use datasets presented at Dataset Ninja, as well as other information, including visualizations and statistics we provide. You are in charge of compliance with any dataset license and all other permissions. You are required to navigate datasets homepage and make sure that you can use it. In case of any questions, get in touch with us at hello@datasetninja.com.