Introduction #
The authors of the Multi-topography Dataset for Wind Turbine Detection have compiled the land remote sensing wind turbine dataset, comprising 1270 remote sensing images containing a total of 4459 individual wind turbines. These images were captured over an extensive time span, showing the same wind farm at different times. The dataset includes both YOLO and VOC tagging formats to accommodate different model requirements.
The dataset varies in 5 different land backgrounds: sandy land, forest land, grassland, snow land, and wasteland. Having diverse land backgrounds enhances the model’s robustness, while the presence of various marker formats and a substantial number of wind turbine instances caters to the object detection needs of different models.
Due to variations in power wind turbine sizes, the multi-angle imaging characteristics of remote sensing satellites, as well as the varying solar radiation angles in different seasons and vegetation shading, wind turbines exhibit significant differences in the images. The image features of the wind turbine shadow are more distinct than those of the wind turbine body. In light of this, to improve the wind turbine detection model’s accuracy, the authors opted to label the wind turbine body and its shadow together as a single unit while using the labelImg tool for target labeling.
Summary #
Multi-topography Dataset for Wind Turbine Detection from Remote Sensing Image is a dataset for an object detection task. Possible applications of the dataset could be in the energy industry.
The dataset consists of 1270 images with 4458 labeled objects belonging to 1 single class (wind turbine).
Images in the Multi-topography Dataset for Wind Turbine Detection dataset have bounding box annotations. All images are labeled (i.e. with annotations). There are no pre-defined train/val/test splits in the dataset. Alternatively, the dataset could be split into 13 locations: Baicheng (258 images), Tongliao (195 images), Tieling (134 images), Yilan (131 images), Ximeng (112 images), Ürümqi (93 images), Hami (92 images), Wulanchabu (75 images), Baqi (51 images), Chifeng (46 images), Jiamusi (45 images), Huiteng (33 images), and Mudanjiang (5 images). The dataset was released in 2023 by the Shandong University of Science and Technology.
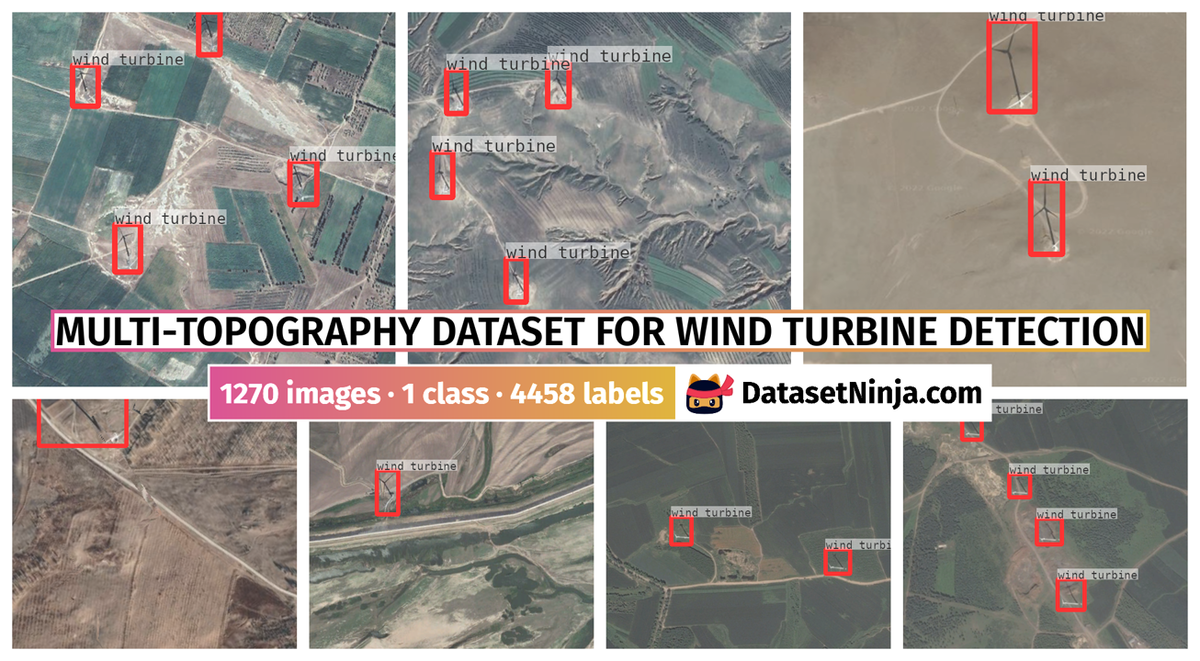
Explore #
Multi-topography Dataset for Wind Turbine Detection dataset has 1270 images. Click on one of the examples below or open "Explore" tool anytime you need to view dataset images with annotations. This tool has extended visualization capabilities like zoom, translation, objects table, custom filters and more. Hover the mouse over the images to hide or show annotations.











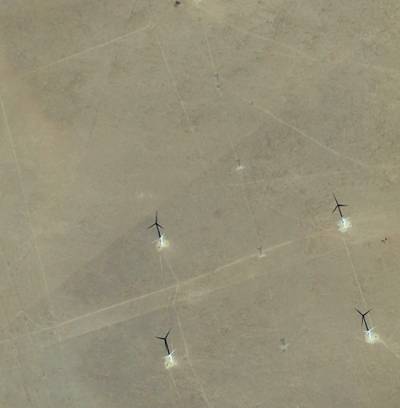

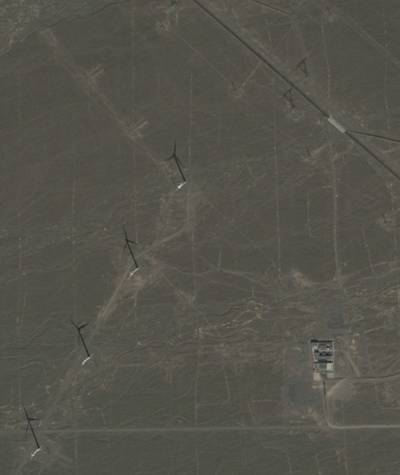










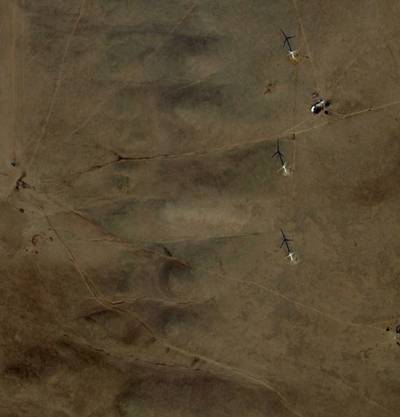




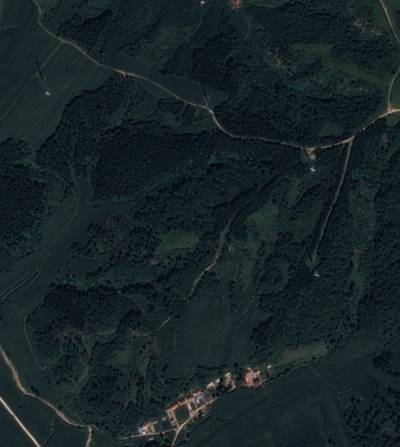
Class balance #
There are 1 annotation classes in the dataset. Find the general statistics and balances for every class in the table below. Click any row to preview images that have labels of the selected class. Sort by column to find the most rare or prevalent classes.
Class ã…¤ | Images ã…¤ | Objects ã…¤ | Count on image average | Area on image average |
---|---|---|---|---|
wind turbineâž” rectangle | 1270 | 4458 | 3.51 | 2.71% |
Images #
Explore every single image in the dataset with respect to the number of annotations of each class it has. Click a row to preview selected image. Sort by any column to find anomalies and edge cases. Use horizontal scroll if the table has many columns for a large number of classes in the dataset.
Object distribution #
Interactive heatmap chart for every class with object distribution shows how many images are in the dataset with a certain number of objects of a specific class. Users can click cell and see the list of all corresponding images.
Class sizes #
The table below gives various size properties of objects for every class. Click a row to see the image with annotations of the selected class. Sort columns to find classes with the smallest or largest objects or understand the size differences between classes.
Class | Object count | Avg area | Max area | Min area | Min height | Min height | Max height | Max height | Avg height | Avg height | Min width | Min width | Max width | Max width |
---|---|---|---|---|---|---|---|---|---|---|---|---|---|---|
wind turbine rectangle | 4458 | 0.77% | 7.69% | 0.1% | 13px | 2.35% | 217px | 38.91% | 59px | 10.76% | 13px | 2.55% | 136px | 34.84% |
Spatial Heatmap #
The heatmaps below give the spatial distributions of all objects for every class. These visualizations provide insights into the most probable and rare object locations on the image. It helps analyze objects' placements in a dataset.
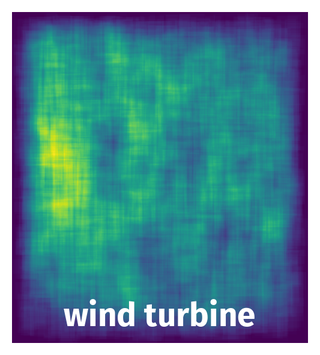
Objects #
Table contains all 4458 objects. Click a row to preview an image with annotations, and use search or pagination to navigate. Sort columns to find outliers in the dataset.
Object ID ã…¤ | Class ã…¤ | Image name click row to open | Image size height x width | Height ã…¤ | Height ã…¤ | Width ã…¤ | Width ã…¤ | Area ã…¤ |
---|---|---|---|---|---|---|---|---|
1âž” | wind turbine rectangle | tieling22.png | 542 x 553 | 73px | 13.47% | 50px | 9.04% | 1.22% |
2âž” | wind turbine rectangle | tieling22.png | 542 x 553 | 75px | 13.84% | 50px | 9.04% | 1.25% |
3âž” | wind turbine rectangle | tieling28.png | 562 x 522 | 25px | 4.45% | 40px | 7.66% | 0.34% |
4âž” | wind turbine rectangle | tieling28.png | 562 x 522 | 29px | 5.16% | 44px | 8.43% | 0.43% |
5âž” | wind turbine rectangle | tieling28.png | 562 x 522 | 33px | 5.87% | 48px | 9.2% | 0.54% |
6âž” | wind turbine rectangle | ximeng73.png | 644 x 627 | 70px | 10.87% | 34px | 5.42% | 0.59% |
7âž” | wind turbine rectangle | ximeng73.png | 644 x 627 | 61px | 9.47% | 32px | 5.1% | 0.48% |
8âž” | wind turbine rectangle | ximeng73.png | 644 x 627 | 66px | 10.25% | 35px | 5.58% | 0.57% |
9âž” | wind turbine rectangle | ximeng73.png | 644 x 627 | 62px | 9.63% | 31px | 4.94% | 0.48% |
10âž” | wind turbine rectangle | ximeng73.png | 644 x 627 | 62px | 9.63% | 31px | 4.94% | 0.48% |
License #
Citation #
If you make use of the Multi-topography dataset for wind turbine detection data, please cite the following reference:
@dataset{hao_mi_2023_7808269,
author = {Hao Mi},
title = {{Multi-topography dataset for wind turbine
detection from remote sensing image}},
month = apr,
year = 2023,
publisher = {Zenodo},
doi = {10.5281/zenodo.7808269},
url = {https://doi.org/10.5281/zenodo.7808269}
}
If you are happy with Dataset Ninja and use provided visualizations and tools in your work, please cite us:
@misc{ visualization-tools-for-multi-topography-dataset-for-wind-turbine-detection-dataset,
title = { Visualization Tools for Multi-topography Dataset for Wind Turbine Detection Dataset },
type = { Computer Vision Tools },
author = { Dataset Ninja },
howpublished = { \url{ https://datasetninja.com/multi-topography-dataset-for-wind-turbine-detection } },
url = { https://datasetninja.com/multi-topography-dataset-for-wind-turbine-detection },
journal = { Dataset Ninja },
publisher = { Dataset Ninja },
year = { 2025 },
month = { mar },
note = { visited on 2025-03-27 },
}
Download #
Dataset Multi-topography Dataset for Wind Turbine Detection can be downloaded in Supervisely format:
As an alternative, it can be downloaded with dataset-tools package:
pip install --upgrade dataset-tools
… using following python code:
import dataset_tools as dtools
dtools.download(dataset='Multi-topography Dataset for Wind Turbine Detection', dst_dir='~/dataset-ninja/')
Make sure not to overlook the python code example available on the Supervisely Developer Portal. It will give you a clear idea of how to effortlessly work with the downloaded dataset.
The data in original format can be downloaded here.
Disclaimer #
Our gal from the legal dep told us we need to post this:
Dataset Ninja provides visualizations and statistics for some datasets that can be found online and can be downloaded by general audience. Dataset Ninja is not a dataset hosting platform and can only be used for informational purposes. The platform does not claim any rights for the original content, including images, videos, annotations and descriptions. Joint publishing is prohibited.
You take full responsibility when you use datasets presented at Dataset Ninja, as well as other information, including visualizations and statistics we provide. You are in charge of compliance with any dataset license and all other permissions. You are required to navigate datasets homepage and make sure that you can use it. In case of any questions, get in touch with us at hello@datasetninja.com.