Introduction #
The authors of the dataset introduced the MVTec D2S: MVTec Densely Segmented Supermarket Dataset as a novel benchmark for instance-aware semantic segmentation in an industrial domain. The benchmark was meticulously designed to mimic real-world settings of automatic checkout, inventory, or warehouse systems. In the training images, only objects of a single class appeared against a homogeneous background, while the validation and test sets were more complex and diverse. The scenes were captured under different lighting conditions, rotations, and backgrounds to evaluate the robustness of instance segmentation methods.
Care was taken to ensure unambiguous labeling of every instance, resulting in pixel-precise annotations. The comprehensive labeling allowed for the use of individual instance crops for artificial data augmentation. The dataset presented various challenges relevant to the field, including limited training data and high diversity in the validation and test sets.
The instance segmentation dataset offered high-resolution images representing a real-world, industrial environment. Annotations for 60 different object categories were obtained through meticulous labeling, ensuring high-quality annotations. The authors emphasized that the training set’s high-quality region annotations could be effectively used for artificial data augmentation, leading to a significant improvement in average precision (AP) on the test set.
The dataset was designed to realistically represent applications such as automatic checkout, inventory, or warehouse systems. In these scenarios, isolated products on a conveyor belt were identified, but external influences and partly occluded objects posed challenges. The dataset’s object categories included common everyday products like fruits, vegetables, cereal packets, pasta, and bottles, organized in a class hierarchy tree. The dataset provided scenes captured from various angles and under different lighting settings to evaluate the robustness of instance segmentation methods.
split | all | train | val | test |
---|---|---|---|---|
scenes | 700 | 146 | 120 | 434 |
images | 21000 | 4380 | 3600 | 13020 |
objects | 72447 | 6900 | 15654 | 49893 |
objects/image | 3.45 | 1.58 | 4.35 | 3.83 |
scenes w. occlusion | 393 | 10 | 84 | 299 |
scenes w. clutter | 86 | 0 | 18 | 68 |
rotations | ✓ | ✓ | ✓ | |
lighting variation | ✓ | ✓ | ✓ | |
background variation | ✓ | ✓ | ||
clutter | ✓ | ✓ |
To meet the mentioned industrial requirements, the training scenes are selected to be as simple as possible: They have a homogeneous background, mostly contain only one object and the amount of occlusions is reduced to a minimum.
train split properties:
- contain only objects of one category
- provide new views of an object
- only contain objects with no or marginal overlap
- have no clutter and a homogeneous background
The remaining scenes are split between the validation and the test set. They consist of scenes with:
- single or multiple objects of different classes
- touching or objects with occlusion
- clutter objects
- random background
The dataset also included ablation study which is performed to evaluate the importance of different variations including all rotations and lightings for one placement of objects in the scene, and the ability of methods to learn invariance with respect to rotations and illumination. For this purpose, the authors created three subsets of the full training set train. The train rot0 set contains all three lightings, but only the first rotation of each scene. The train light0 set contains only the default lighting, but all ten rotations of each scene. The train rot0_light0 set contains only the default lighting and the first rotation for each scene.
The data collection utilized a high-resolution industrial color camera with 1920 × 1440 pixels mounted above a turntable. The camera’s intentional off-centered mounting introduced more variations in the rotated images. The scenes were rotated ten times in increments of 36 degrees, ensuring precise rotation angles. To assess the robustness to illumination changes and reflection, each scene and rotation were captured under three different lighting settings using an LED ring light attached to the camera, spanning a wide spectrum of possible lightings.
Note, that this is a version 1.1 of D2S annotations - with new subsets containing specific difficulties (not mentioned in paper), such as occlusion, clutter or random background.
Summary #
MVTec D2S: MVTec Densely Segmented Supermarket Dataset is a dataset for instance segmentation, semantic segmentation, and object detection tasks. It is used in the retail industry.
The dataset consists of 53114 images with 258748 labeled objects belonging to 60 different classes including dr_oetker_vitalis_knuspermuesli_klassisch, clementine_single, salad_iceberg, and other: pasta_reggia_fusilli, gepa_italienischer_bio_espresso, adelholzener_gourmet_mineralwasser_02, cocoba_cocoa, douwe_egberts_professional_ground_coffee, zucchini, vine_tomatoes, franken_tafelreiniger, cafe_wunderbar_espresso, corny_schoko_banane_single, clementine, corny_nussvoll, kilimanjaro_tea_earl_grey, augustiner_weissbier_05, apple_braeburn_bundle, corny_nussvoll_single, gepa_bio_caffe_crema, rocket, pasta_reggia_spaghetti, koelln_muesli_schoko, coca_cola_light_05, gepa_bio_und_fair_kraeuterteemischung, koelln_muesli_fruechte, caona_cocoa, kiwi, and 32 more.
Images in the MVTec D2S dataset have pixel-level instance segmentation and bounding box annotations. Due to the nature of the instance segmentation task, it can be automatically transformed into a semantic segmentation task (only one mask for every class). There are 28740 (54% of the total) unlabeled images (i.e. without annotations). There are 4 splits in the dataset: test (28740 images), augmented (10000 images), validation (7950 images), and training (6424 images). Alternatively, the dataset could be split into 3 train ablation study sets: light0 (1460 images), rot0 (438 images), and rot0_light0 (146 images), or into 6 test specific difficulties: info (13020 images), info_random_background (4650 images), info_occlusion (3930 images), info_random_background_wo_clutter (2640 images), info_wo_occlusion (2490 images), and info_clutter (2010 images), or into 5 validation specific difficulties: random_background (1350 images), occlusion (990 images), random_background_wo_clutter (810 images), wo_occlusion (660 images), and clutter (540 images). The dataset was released in 2018 by the MVTec Software GmbH, Germany and Technical University of Munich.
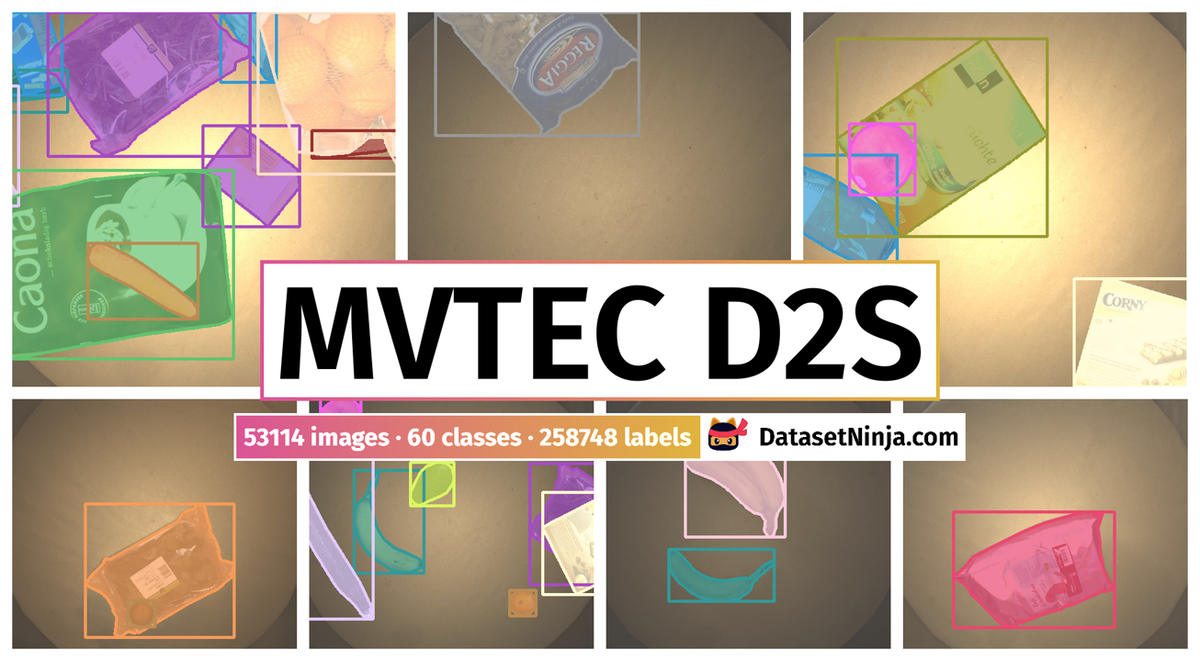
Explore #
MVTec D2S dataset has 53114 images. Click on one of the examples below or open "Explore" tool anytime you need to view dataset images with annotations. This tool has extended visualization capabilities like zoom, translation, objects table, custom filters and more. Hover the mouse over the images to hide or show annotations.
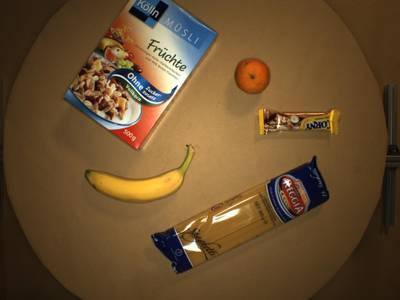

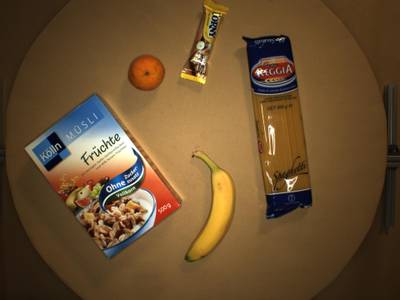
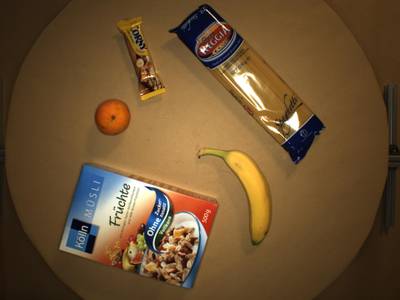
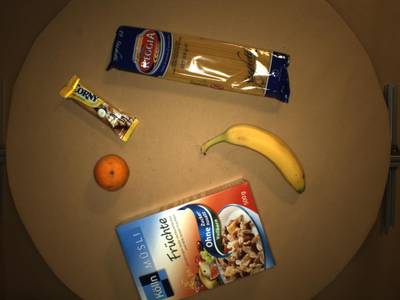
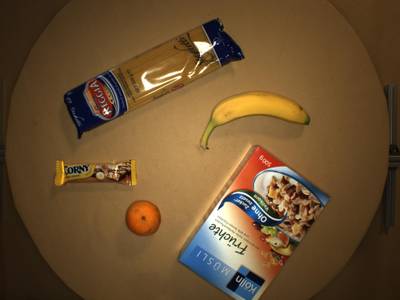
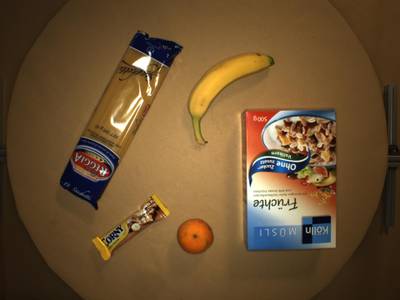
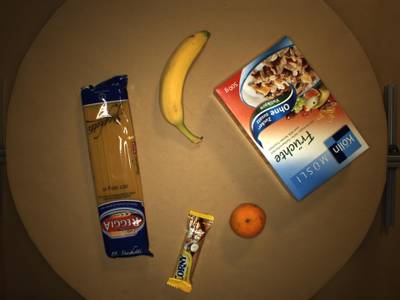
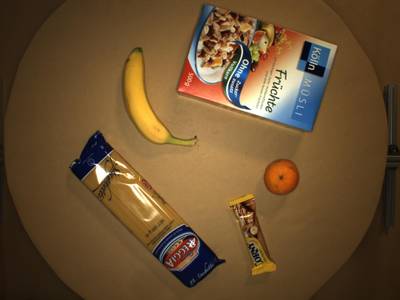
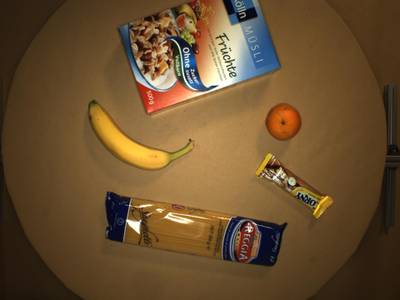

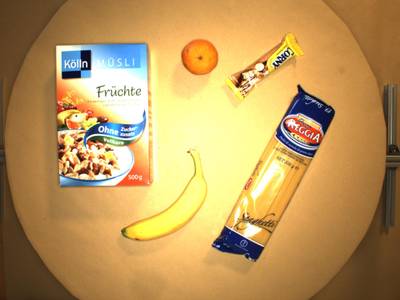
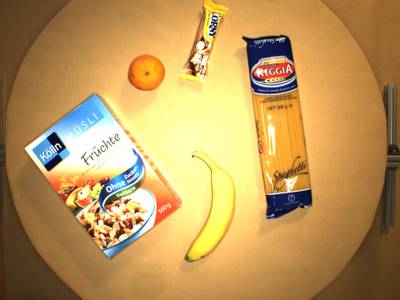
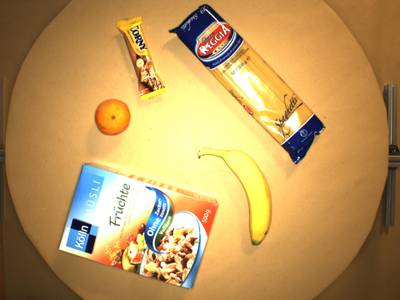
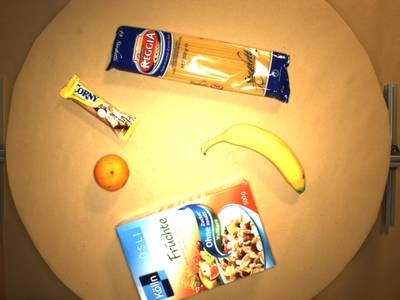
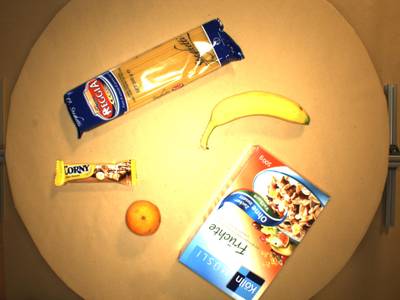
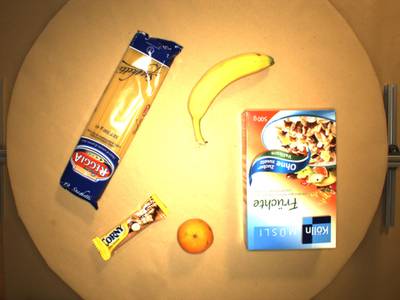
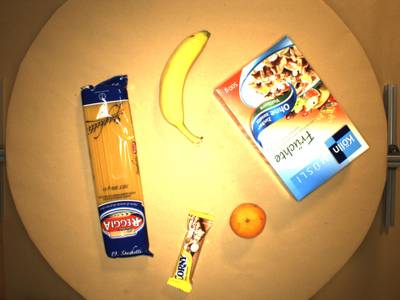
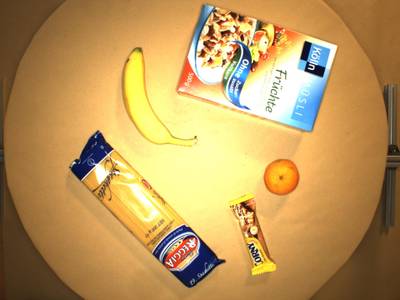
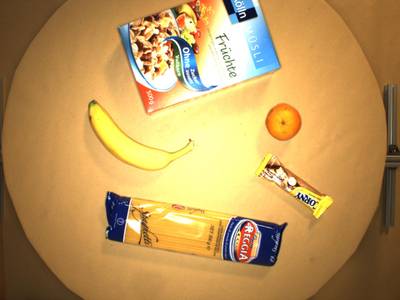
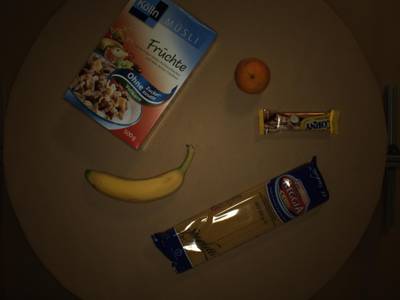
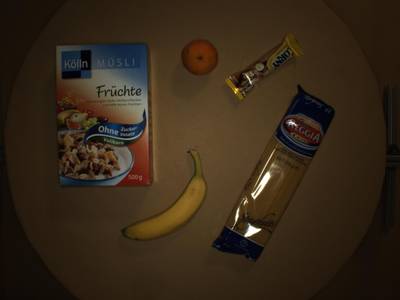
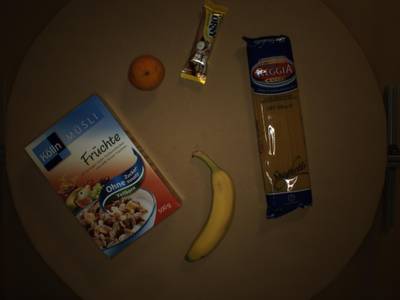
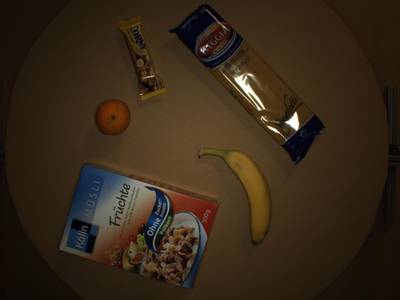
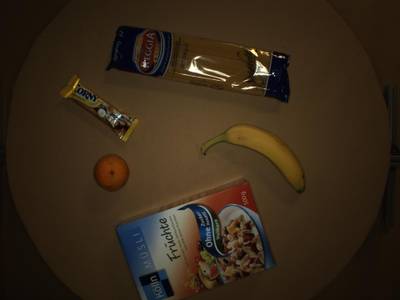
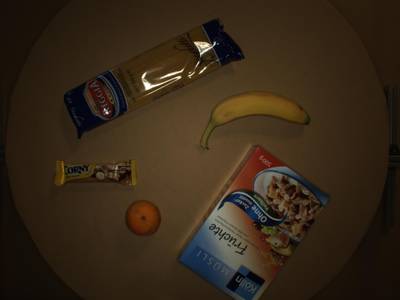
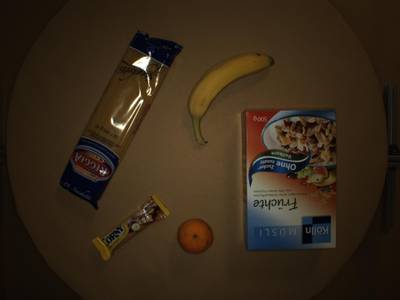

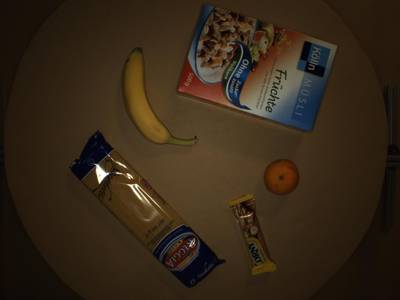
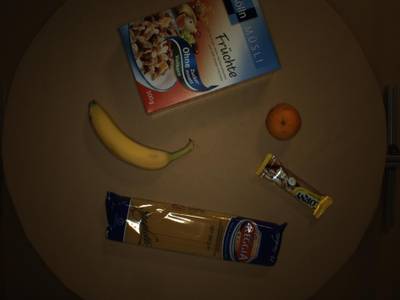
Class balance #
There are 60 annotation classes in the dataset. Find the general statistics and balances for every class in the table below. Click any row to preview images that have labels of the selected class. Sort by column to find the most rare or prevalent classes.
Class ㅤ | Images ㅤ | Objects ㅤ | Count on image average | Area on image average |
---|---|---|---|---|
dr_oetker_vitalis_knuspermuesli_klassisch➔ any | 1167 | 2658 | 2.28 | 13.63% |
salad_iceberg➔ any | 1136 | 2456 | 2.16 | 11.29% |
clementine_single➔ any | 1134 | 2499 | 2.2 | 0.86% |
pasta_reggia_fusilli➔ any | 1128 | 2545 | 2.26 | 16.39% |
gepa_italienischer_bio_espresso➔ any | 1104 | 2484 | 2.25 | 9.63% |
adelholzener_gourmet_mineralwasser_02➔ any | 1086 | 2535 | 2.33 | 3.92% |
vine_tomatoes➔ any | 1078 | 2485 | 2.31 | 18.82% |
corny_nussvoll➔ any | 1070 | 2312 | 2.16 | 8.41% |
cocoba_cocoa➔ any | 1069 | 2525 | 2.36 | 8.91% |
cafe_wunderbar_espresso➔ any | 1053 | 2377 | 2.26 | 21.23% |
Images #
Explore every single image in the dataset with respect to the number of annotations of each class it has. Click a row to preview selected image. Sort by any column to find anomalies and edge cases. Use horizontal scroll if the table has many columns for a large number of classes in the dataset.
Object distribution #
Interactive heatmap chart for every class with object distribution shows how many images are in the dataset with a certain number of objects of a specific class. Users can click cell and see the list of all corresponding images.
Class sizes #
The table below gives various size properties of objects for every class. Click a row to see the image with annotations of the selected class. Sort columns to find classes with the smallest or largest objects or understand the size differences between classes.
Class | Object count | Avg area | Max area | Min area | Min height | Min height | Max height | Max height | Avg height | Avg height | Min width | Min width | Max width | Max width |
---|---|---|---|---|---|---|---|---|---|---|---|---|---|---|
dr_oetker_vitalis_knuspermuesli_klassisch any | 5405 | 9.24% | 22.4% | 0% | 2px | 0.14% | 819px | 56.88% | 539px | 37.43% | 2px | 0.1% | 821px | 42.76% |
corny_schoko_banane_single any | 5289 | 2.24% | 6.2% | 0% | 2px | 0.14% | 472px | 32.78% | 294px | 20.41% | 2px | 0.1% | 472px | 24.58% |
cocoba_cocoa any | 5075 | 5.9% | 17.5% | 0% | 2px | 0.14% | 719px | 49.93% | 440px | 30.52% | 2px | 0.1% | 748px | 38.96% |
adelholzener_gourmet_mineralwasser_02 any | 5048 | 2.6% | 10.37% | 0% | 2px | 0.14% | 661px | 45.9% | 291px | 20.2% | 2px | 0.1% | 661px | 34.43% |
pasta_reggia_fusilli any | 4965 | 11.07% | 31.11% | 0% | 2px | 0.14% | 950px | 65.97% | 587px | 40.76% | 2px | 0.1% | 951px | 49.53% |
gepa_italienischer_bio_espresso any | 4958 | 6.65% | 17.44% | 0% | 2px | 0.14% | 799px | 55.49% | 472px | 32.77% | 2px | 0.1% | 799px | 41.61% |
clementine_single any | 4926 | 0.68% | 2.02% | 0% | 8px | 0.56% | 237px | 16.46% | 143px | 9.95% | 6px | 0.31% | 238px | 12.4% |
douwe_egberts_professional_ground_coffee any | 4909 | 6.12% | 13.02% | 0% | 2px | 0.14% | 605px | 42.01% | 441px | 30.63% | 2px | 0.1% | 606px | 31.56% |
augustiner_weissbier_05 any | 4889 | 4.31% | 12.61% | 0% | 2px | 0.14% | 743px | 51.6% | 383px | 26.61% | 2px | 0.1% | 738px | 38.44% |
salad_iceberg any | 4850 | 8.79% | 16.07% | 0% | 2px | 0.14% | 712px | 49.44% | 514px | 35.72% | 2px | 0.1% | 744px | 38.75% |
Spatial Heatmap #
The heatmaps below give the spatial distributions of all objects for every class. These visualizations provide insights into the most probable and rare object locations on the image. It helps analyze objects' placements in a dataset.
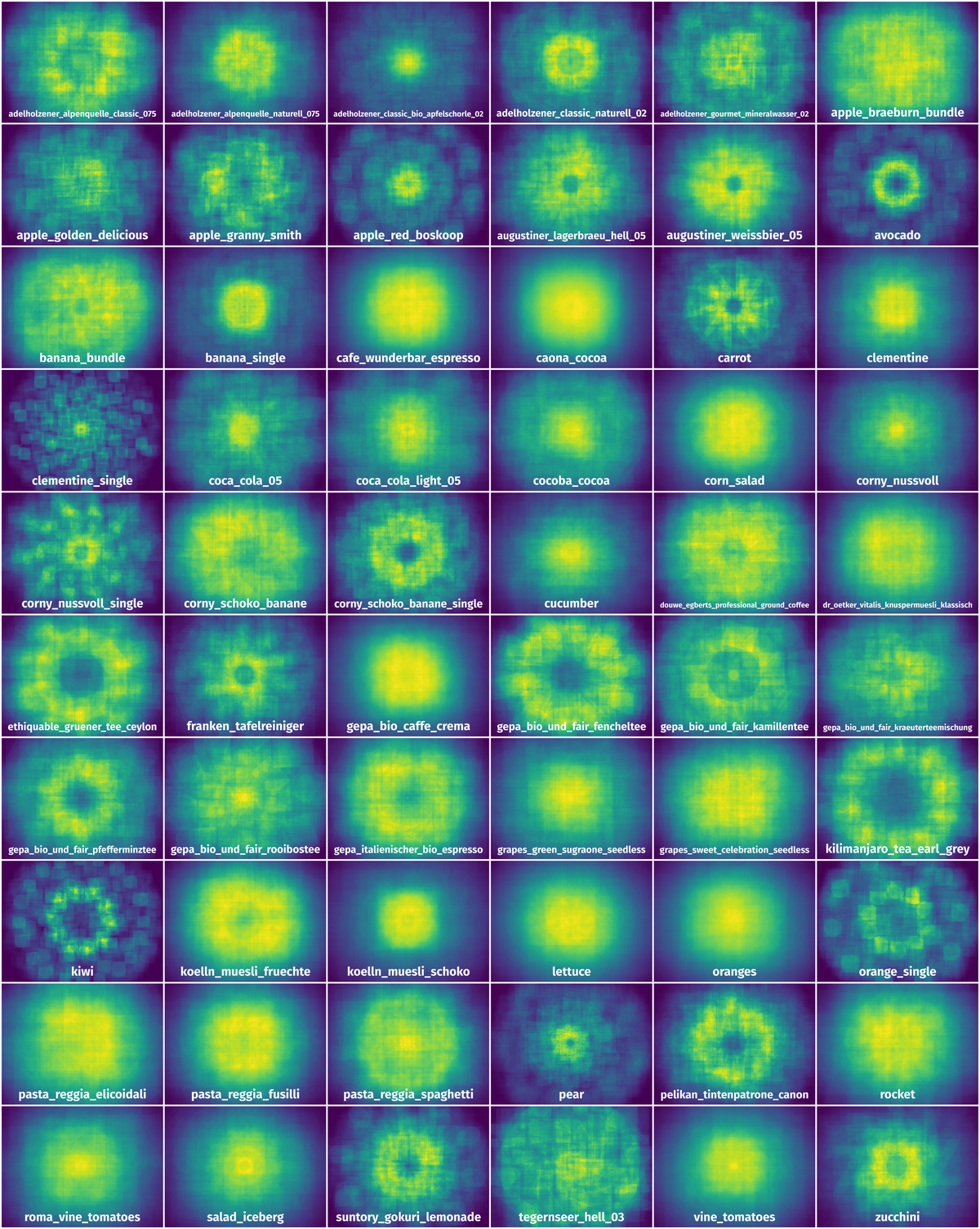
Objects #
Table contains all 258748 objects. Click a row to preview an image with annotations, and use search or pagination to navigate. Sort columns to find outliers in the dataset.
Object ID ㅤ | Class ㅤ | Image name click row to open | Image size height x width | Height ㅤ | Height ㅤ | Width ㅤ | Width ㅤ | Area ㅤ |
---|---|---|---|---|---|---|---|---|
1➔ | zucchini any | D2S_057307_D2S_validation_random_background.jpg | 1440 x 1920 | 289px | 20.07% | 568px | 29.58% | 2.48% |
2➔ | zucchini any | D2S_057307_D2S_validation_random_background.jpg | 1440 x 1920 | 290px | 20.14% | 569px | 29.64% | 5.97% |
3➔ | vine_tomatoes any | D2S_057307_D2S_validation_random_background.jpg | 1440 x 1920 | 830px | 57.64% | 1045px | 54.43% | 15.07% |
4➔ | vine_tomatoes any | D2S_057307_D2S_validation_random_background.jpg | 1440 x 1920 | 831px | 57.71% | 1046px | 54.48% | 31.44% |
5➔ | gepa_bio_caffe_crema any | D2S_039728_D2S_validation_occlusion.jpg | 1440 x 1920 | 975px | 67.71% | 876px | 45.62% | 15.62% |
6➔ | gepa_bio_caffe_crema any | D2S_039728_D2S_validation_occlusion.jpg | 1440 x 1920 | 976px | 67.78% | 877px | 45.68% | 30.96% |
7➔ | avocado any | D2S_039728_D2S_validation_occlusion.jpg | 1440 x 1920 | 234px | 16.25% | 265px | 13.8% | 1.51% |
8➔ | avocado any | D2S_039728_D2S_validation_occlusion.jpg | 1440 x 1920 | 235px | 16.32% | 266px | 13.85% | 2.26% |
9➔ | salad_iceberg any | D2S_055321_D2S_validation.jpg | 1440 x 1920 | 601px | 41.74% | 561px | 29.22% | 9.04% |
10➔ | salad_iceberg any | D2S_055321_D2S_validation.jpg | 1440 x 1920 | 602px | 41.81% | 562px | 29.27% | 12.24% |
License #
MVTec D2S: MVTec D2S Densely Segmented Supermarket Dataset is under CC BY-NC-SA 4.0 license.
Citation #
If you make use of the MVTEC D2S data, please cite the following reference:
Patrick Follmann, Tobias Böttger, Philipp Härtinger, Rebecca König, Markus Ulrich;
MVTec D2S: Densely Segmented Supermarket Dataset;
European Conference on Computer Vision (ECCV), 569-585, 2018
If you are happy with Dataset Ninja and use provided visualizations and tools in your work, please cite us:
@misc{ visualization-tools-for-mvtec-d2s-dataset,
title = { Visualization Tools for MVTec D2S Dataset },
type = { Computer Vision Tools },
author = { Dataset Ninja },
howpublished = { \url{ https://datasetninja.com/mvtec-d2s } },
url = { https://datasetninja.com/mvtec-d2s },
journal = { Dataset Ninja },
publisher = { Dataset Ninja },
year = { 2025 },
month = { may },
note = { visited on 2025-05-19 },
}
Download #
Dataset MVTec D2S can be downloaded in Supervisely format:
As an alternative, it can be downloaded with dataset-tools package:
pip install --upgrade dataset-tools
… using following python code:
import dataset_tools as dtools
dtools.download(dataset='MVTec D2S', dst_dir='~/dataset-ninja/')
Make sure not to overlook the python code example available on the Supervisely Developer Portal. It will give you a clear idea of how to effortlessly work with the downloaded dataset.
The data in original format can be downloaded here.
Disclaimer #
Our gal from the legal dep told us we need to post this:
Dataset Ninja provides visualizations and statistics for some datasets that can be found online and can be downloaded by general audience. Dataset Ninja is not a dataset hosting platform and can only be used for informational purposes. The platform does not claim any rights for the original content, including images, videos, annotations and descriptions. Joint publishing is prohibited.
You take full responsibility when you use datasets presented at Dataset Ninja, as well as other information, including visualizations and statistics we provide. You are in charge of compliance with any dataset license and all other permissions. You are required to navigate datasets homepage and make sure that you can use it. In case of any questions, get in touch with us at hello@datasetninja.com.