Introduction #
The authors of the MVTec LOCO AD: MVTec Logical Constraints Anomaly Detection dataset introduce a benchmark corpus aimed at addressing the unsupervised detection and localization of anomalies in natural images. This challenging problem involves the identification of anomalies that can manifest in diverse ways. The authors offer pixel-precise ground truth annotations for each anomalous region and introduce an evaluation metric tailored to address localization ambiguities that can arise with logical anomalies. To create an effective benchmark, the authors assert that a dataset should encompass representative examples of various anomaly types. They observe that existing datasets tend to focus on local structural anomalies, such as scratches or dents, while overlooking anomalies involving violations of logical constraints, such as objects appearing in invalid locations. To bridge this gap, the authors contribute a novel dataset based on industrial inspection contexts. This dataset is meticulously designed to provide a balanced representation of both structural and logical anomalies. Additionally, they present a new algorithm that outperforms existing approaches in jointly detecting structural and logical anomalies. This algorithm comprises local and global network branches, each with distinct responsibilities.
The authors highlight that existing datasets often focus solely on structural anomalies within industrial inspection scenarios. They note the scarcity of datasets that include both structural and logical anomalies, which are prevalent in real-world manufacturing processes. In response, the authors introduce a dataset inspired by industrial inspection scenarios, carefully balancing the presence of logical and structural anomalies.
This new dataset, termed MVTec LOCO AD, is composed of five object categories relevant to industrial inspection contexts. The authors selected these objects to closely mimic real-world applications. The dataset includes 1772 train images, 304 validation images, and 1568 test images. The authors provide sample images for each category and stress that the training and validation sets exclusively contain anomaly-free images. The test set encompasses both anomaly-free images and images featuring diverse types of logical and structural anomalies. The authors emphasize the independence of the three sets from one another, ensuring distinct physical objects and no overlap between sets.
Each category in the dataset adheres to specific logical constraints, and the authors detail the characteristics of each category and the logical rules governing them. The authors illustrate logical defects and provide examples of how violations of these constraints manifest within the dataset’s images.
Category | # Training images | # Validation images | # Test images (anomaly-free) | # Test images (structural) | # Test images (logical) | # Defect types | Image width | Image height |
---|---|---|---|---|---|---|---|---|
Breakfast Box | 351 | 62 | 102 | 90 | 83 | 22 | 1600 | 1280 |
Screw Bag | 360 | 60 | 122 | 82 | 137 | 20 | 1600 | 1100 |
Pushpins | 372 | 69 | 138 | 81 | 91 | 8 | 1700 | 1000 |
Splicing Connectors | 354 | 59 | 119 | 85 | 108 | 21 | 1700 | 850 |
Juice Bottle | 335 | 54 | 94 | 94 | 142 | 18 | 800 | 1600 |
Total | 1772 | 304 | 575 | 432 | 561 | 89 | – | – |
For model evaluation purposes, authors selected suitable saturation thresholds for each of the 89 individual defect types that occur in the dataset.
For an annotated anomaly A, a saturation_threshold s is selected. Once the overlap of the predicted region with the ground truth A exceeds s, the anomaly segmentation task is considered as solved. A relative_saturation=True
indicates that the whole area of the ground truth region is taken as the saturation threshold. In particular, this is the case for all structural anomalies in the dataset.
Summary #
MVTec LOCO AD: MVTec Logical Constraints Anomaly Detection is a dataset for instance segmentation, semantic segmentation, object detection, classification, and unsupervised learning tasks. It is used in the anomaly detection research. Possible applications of the dataset could be in the industrial domain.
The dataset consists of 3651 images with 1969 labeled objects belonging to 83 different classes including contamination, missing_pushpin, color, and other: 1_additional_pushpin, fruit_damaged, broken, wrong_cable_location, part_broken, missing_top_label, missing_bottom_label, wrong_fill_level_not_enough, wrong_juice_type, damaged_label, screw_too_long, screw_too_short, 1_very_short_screw, bag_broken, front_bent, juice_color, missing_separator, swapped_labels, empty_bottle, wrong_fill_level_too_much, missing_connector, missing_cable, extra_cable, cable_color, broken_cable, and 55 more.
Images in the MVTec LOCO AD dataset have pixel-level instance segmentation annotations. Due to the nature of the instance segmentation task, it can be automatically transformed into a semantic segmentation (only one mask for every class) or object detection (bounding boxes for every object) tasks. There are 2672 (73% of the total) unlabeled images (i.e. without annotations). There are 3 splits in the dataset: train (1778 images), test (1568 images), and validation (305 images). Alternatively, the dataset could be split into 5 categories: screw_bag (761 images), pushpins (751 images), splicing_connectors (732 images), juice_bottle (719 images), and breakfast_box (688 images), or into 3 classification sets: good (2658 images), logical_anomaly (561 images), and structural_anomaly (432 images). Additionaly, each image has tags for model evaluation: saturation_threshold and relative_saturation. The dataset was released in 2021 by the MVTec Software GmbH, Germany.
Here is a visualized example for randomly selected sample classes:
Explore #
MVTec LOCO AD dataset has 3651 images. Click on one of the examples below or open "Explore" tool anytime you need to view dataset images with annotations. This tool has extended visualization capabilities like zoom, translation, objects table, custom filters and more. Hover the mouse over the images to hide or show annotations.
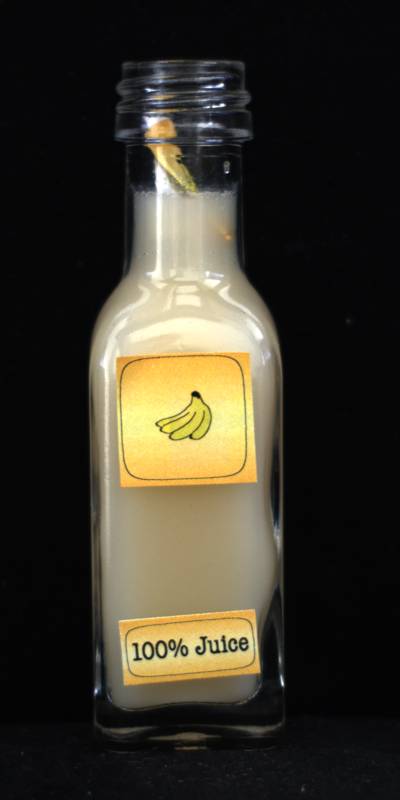
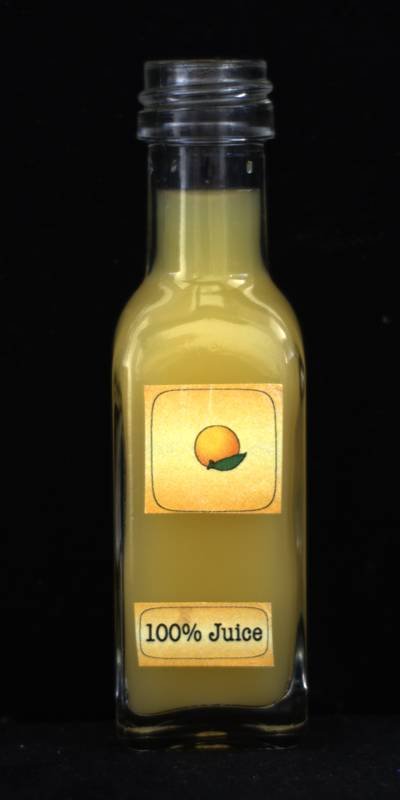
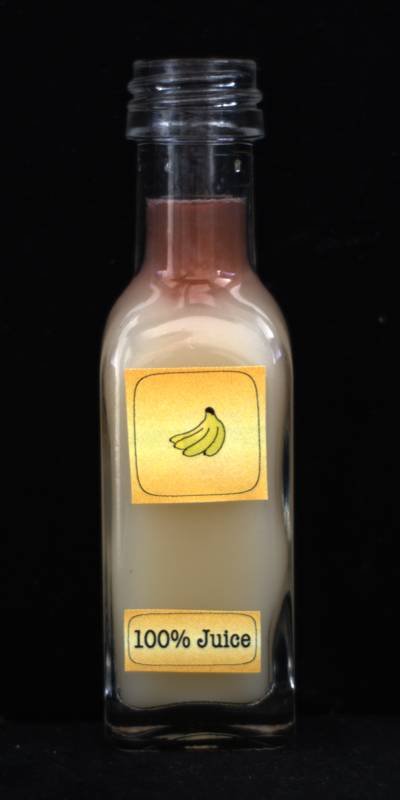
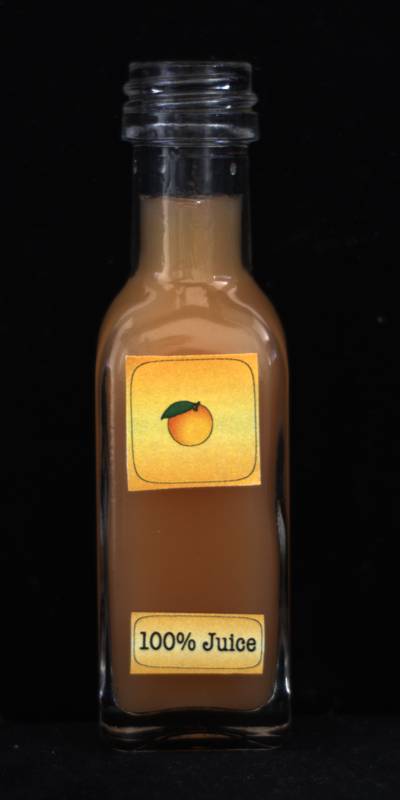

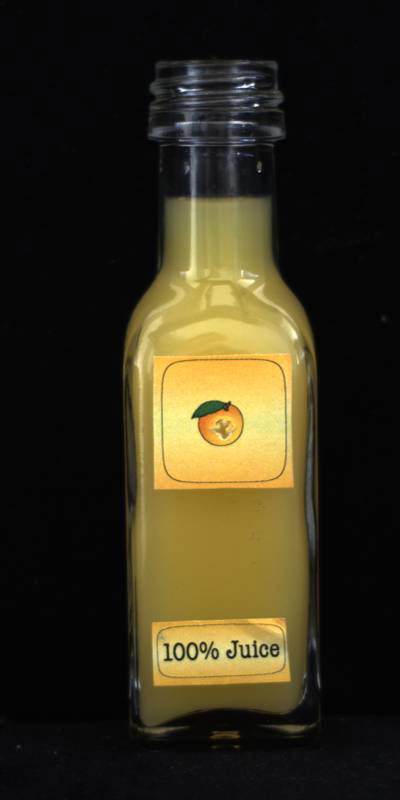
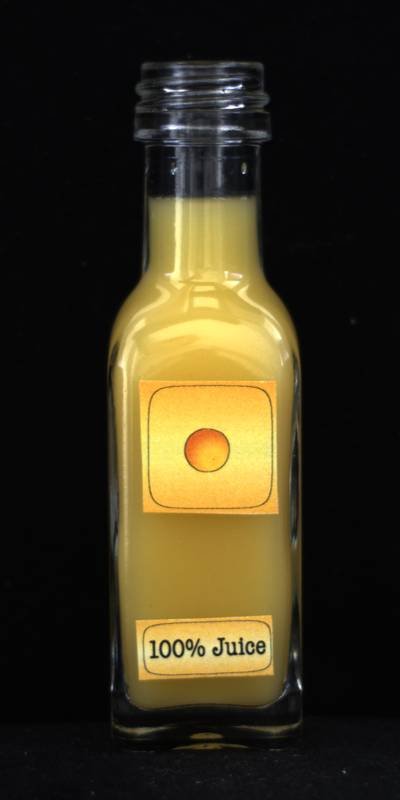
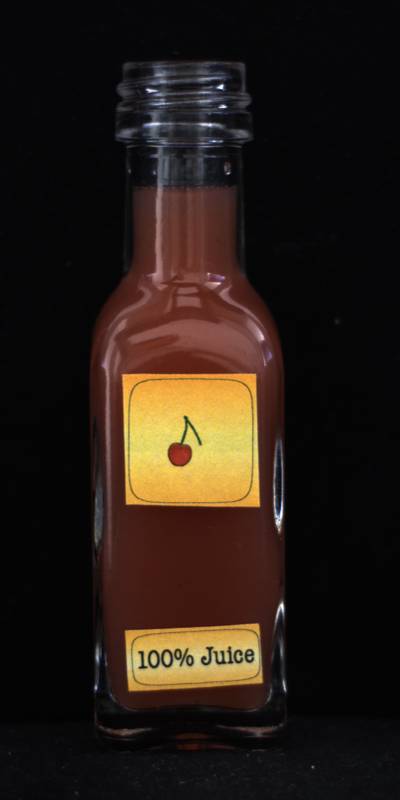
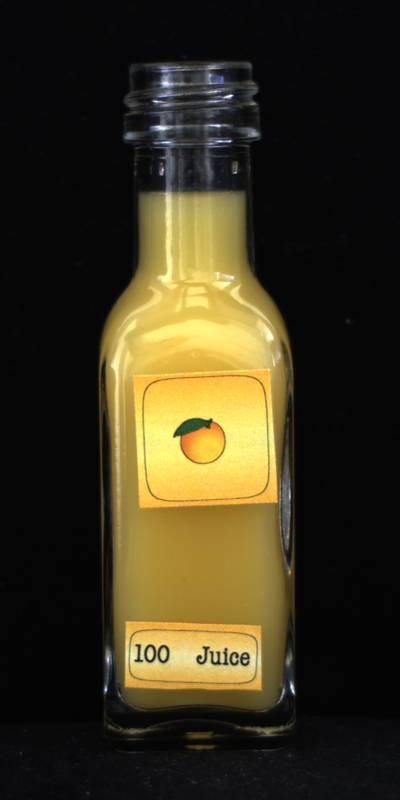
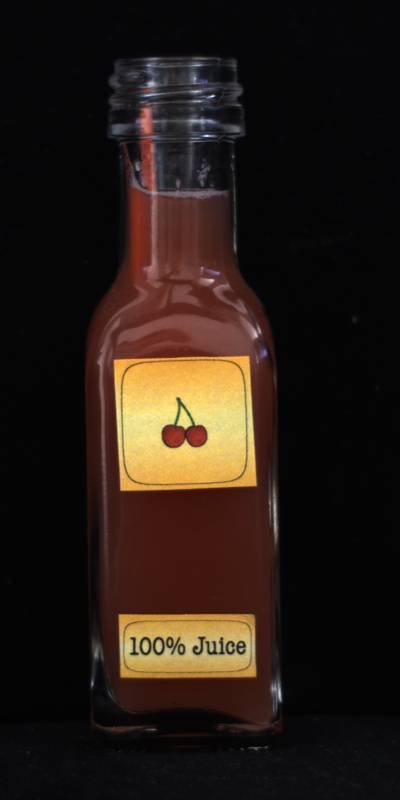
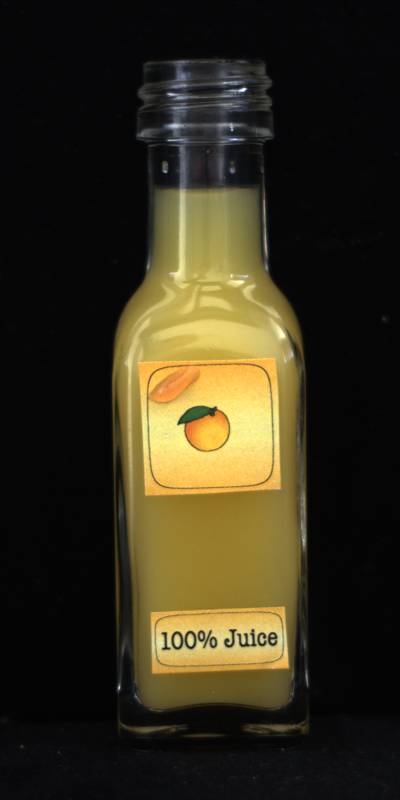
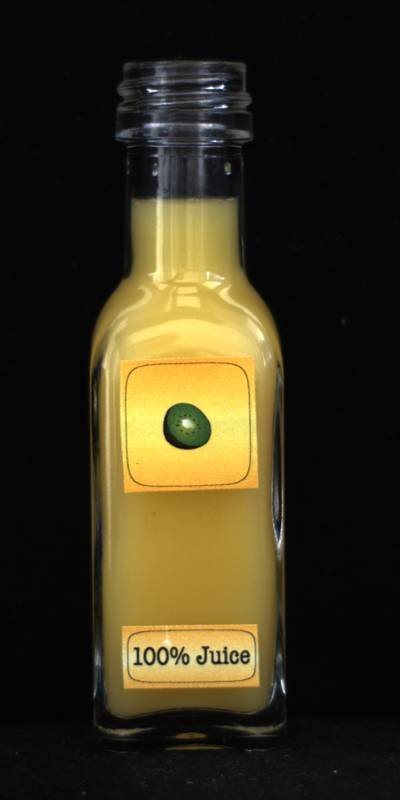
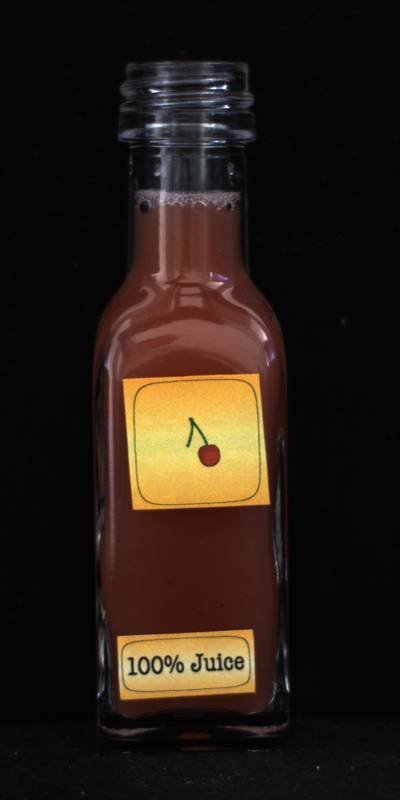
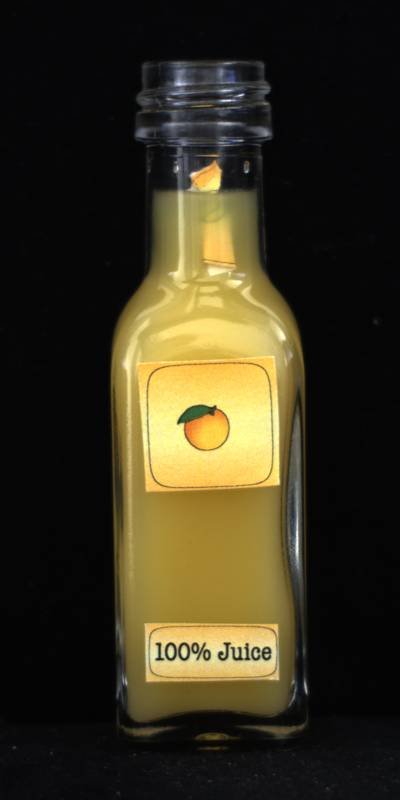
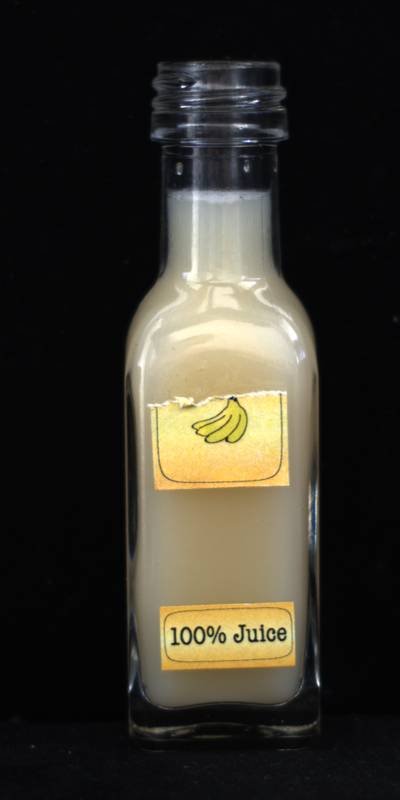
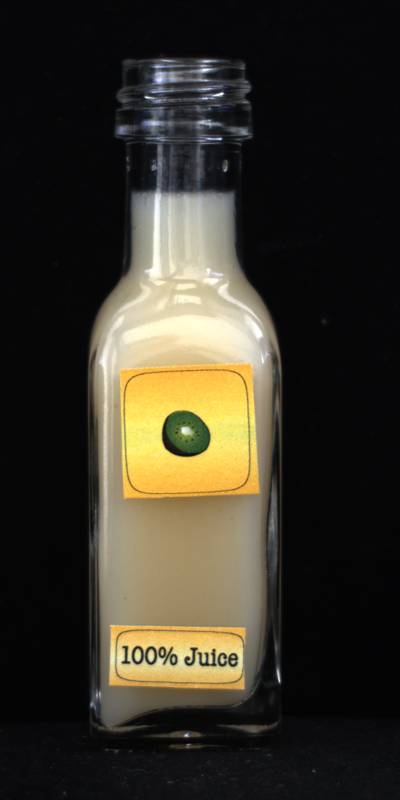
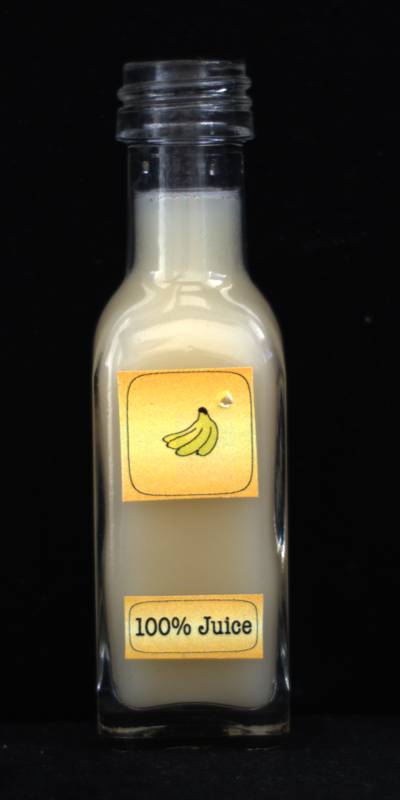



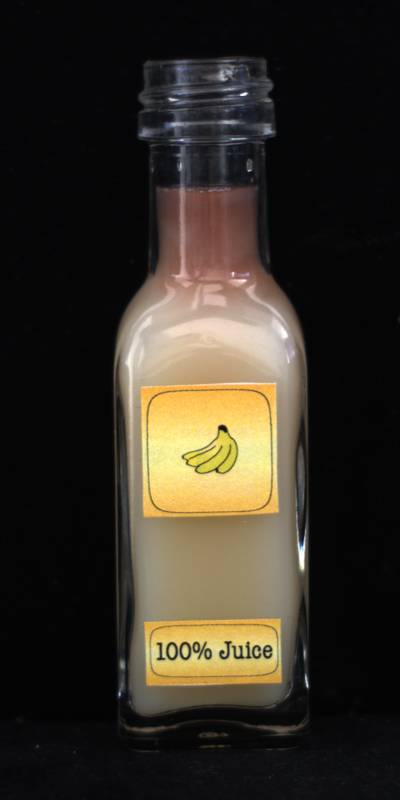


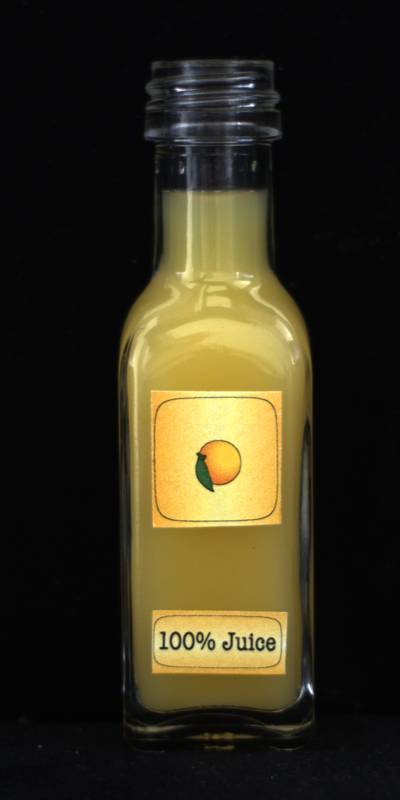
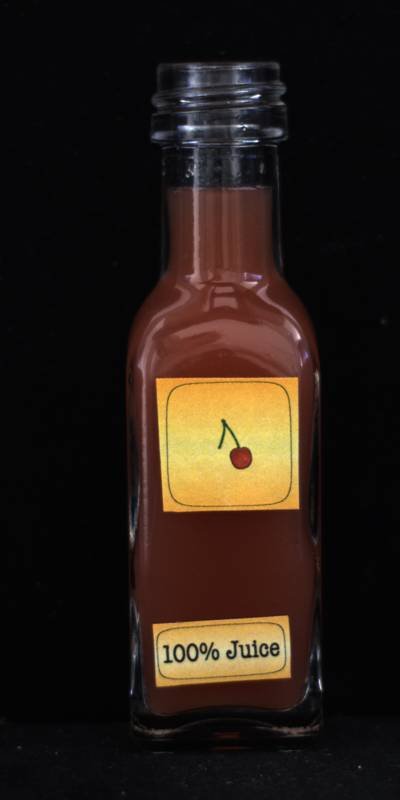

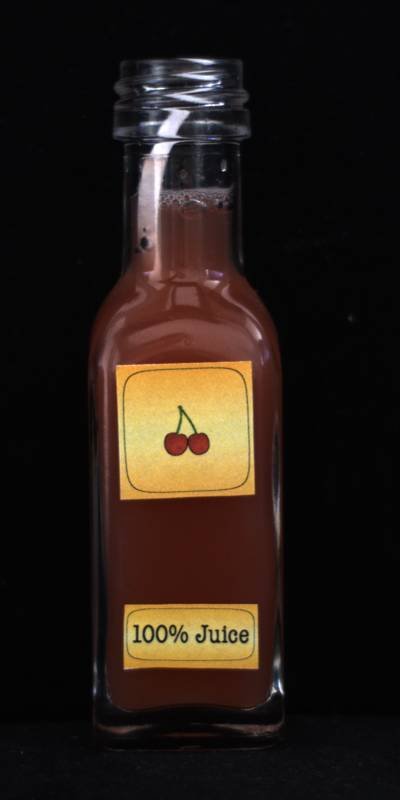
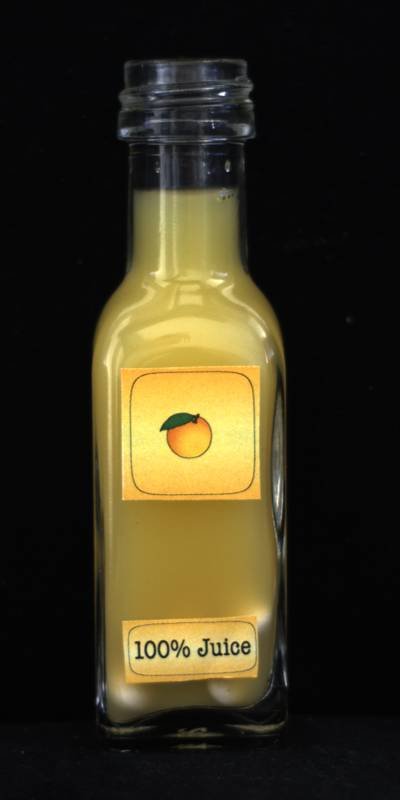
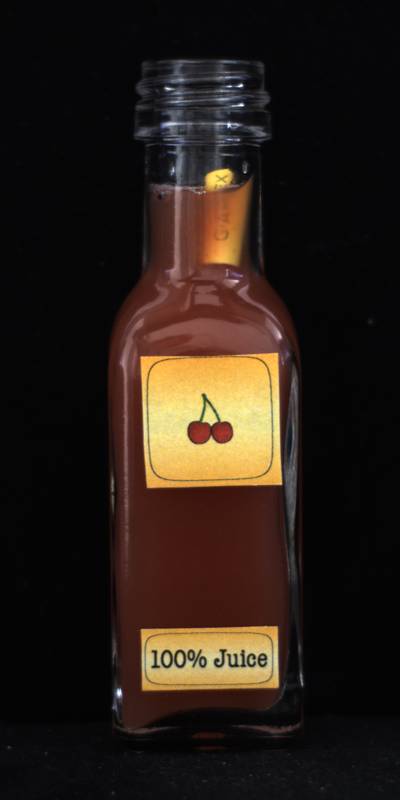
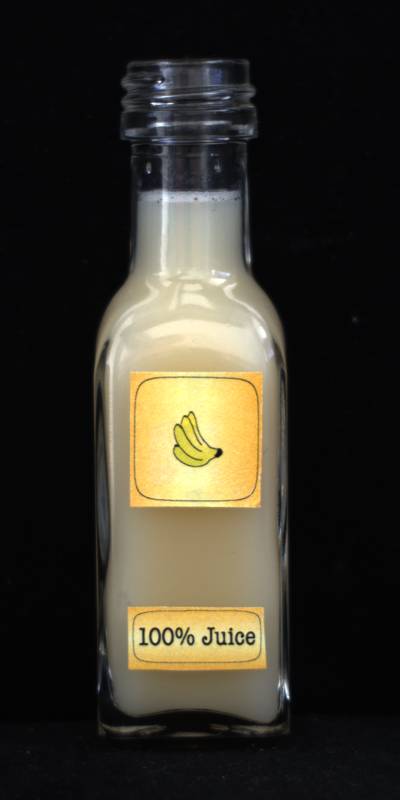
Class balance #
There are 83 annotation classes in the dataset. Find the general statistics and balances for every class in the table below. Click any row to preview images that have labels of the selected class. Sort by column to find the most rare or prevalent classes.
Class ã…¤ | Images ã…¤ | Objects ã…¤ | Count on image average | Area on image average |
---|---|---|---|---|
contaminationâž” mask | 123 | 158 | 1.28 | 1.3% |
missing_pushpinâž” mask | 47 | 143 | 3.04 | 15.6% |
colorâž” mask | 43 | 57 | 1.33 | 0.18% |
1_additional_pushpinâž” mask | 36 | 247 | 6.86 | 3.25% |
fruit_damagedâž” mask | 31 | 35 | 1.13 | 0.55% |
brokenâž” mask | 29 | 30 | 1.03 | 0.12% |
wrong_cable_locationâž” mask | 25 | 125 | 5 | 11.69% |
part_brokenâž” mask | 25 | 25 | 1 | 0.28% |
wrong_fill_level_not_enoughâž” mask | 24 | 24 | 1 | 6.33% |
missing_top_labelâž” mask | 24 | 24 | 1 | 8.12% |
Images #
Explore every single image in the dataset with respect to the number of annotations of each class it has. Click a row to preview selected image. Sort by any column to find anomalies and edge cases. Use horizontal scroll if the table has many columns for a large number of classes in the dataset.
Object distribution #
Interactive heatmap chart for every class with object distribution shows how many images are in the dataset with a certain number of objects of a specific class. Users can click cell and see the list of all corresponding images.
Class sizes #
The table below gives various size properties of objects for every class. Click a row to see the image with annotations of the selected class. Sort columns to find classes with the smallest or largest objects or understand the size differences between classes.
Class | Object count | Avg area | Max area | Min area | Min height | Min height | Max height | Max height | Avg height | Avg height | Min width | Min width | Max width | Max width |
---|---|---|---|---|---|---|---|---|---|---|---|---|---|---|
1_additional_pushpin mask | 247 | 0.47% | 0.9% | 0% | 7px | 0.7% | 281px | 28.1% | 141px | 14.07% | 8px | 0.47% | 306px | 18% |
contamination mask | 158 | 1.01% | 8.63% | 0.02% | 7px | 0.44% | 507px | 39.61% | 163px | 13.18% | 27px | 1.59% | 525px | 37.62% |
missing_pushpin mask | 143 | 5.13% | 5.42% | 4.91% | 276px | 27.6% | 299px | 29.9% | 288px | 28.8% | 302px | 17.76% | 325px | 19.12% |
wrong_cable_location mask | 125 | 2.34% | 14.78% | 0.1% | 23px | 2.71% | 348px | 40.94% | 79px | 9.27% | 72px | 4.24% | 988px | 58.12% |
missing_cable mask | 92 | 1.98% | 21.97% | 0.1% | 23px | 2.71% | 499px | 58.71% | 67px | 7.86% | 73px | 4.29% | 775px | 45.59% |
cable_color mask | 76 | 1.97% | 5.03% | 0.09% | 22px | 2.59% | 308px | 36.24% | 118px | 13.93% | 68px | 4% | 959px | 56.41% |
extra_cable mask | 71 | 0.59% | 2.88% | 0.07% | 21px | 2.47% | 136px | 16% | 40px | 4.76% | 59px | 3.47% | 962px | 56.59% |
color mask | 57 | 0.13% | 0.66% | 0% | 10px | 0.91% | 187px | 17% | 54px | 5.25% | 9px | 0.56% | 224px | 14% |
wrong_juice_type mask | 44 | 11.66% | 23% | 0.55% | 101px | 6.31% | 1129px | 70.56% | 610px | 38.13% | 108px | 13.5% | 398px | 49.75% |
screw_too_long mask | 42 | 0.5% | 0.56% | 0.45% | 72px | 6.55% | 145px | 13.18% | 108px | 9.85% | 82px | 5.12% | 158px | 9.88% |
Spatial Heatmap #
The heatmaps below give the spatial distributions of all objects for every class. These visualizations provide insights into the most probable and rare object locations on the image. It helps analyze objects' placements in a dataset.
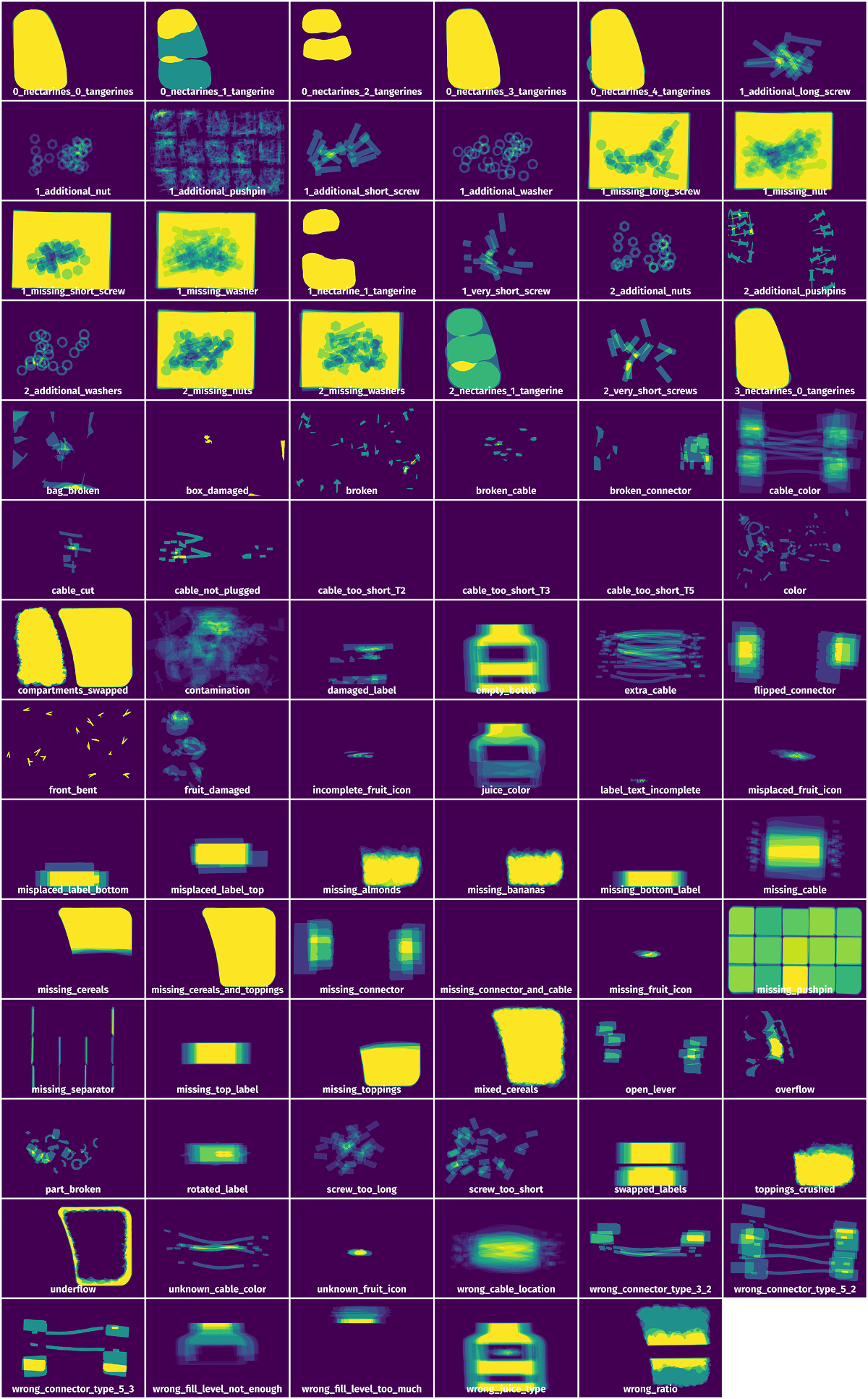
Objects #
Table contains all 1969 objects. Click a row to preview an image with annotations, and use search or pagination to navigate. Sort columns to find outliers in the dataset.
Object ID ã…¤ | Class ã…¤ | Image name click row to open | Image size height x width | Height ã…¤ | Height ã…¤ | Width ã…¤ | Width ã…¤ | Area ã…¤ |
---|---|---|---|---|---|---|---|---|
1âž” | screw_too_short mask | screw_bag_logical_anomalies_037.png | 1100 x 1600 | 113px | 10.27% | 148px | 9.25% | 0.47% |
2âž” | screw_too_short mask | screw_bag_logical_anomalies_037.png | 1100 x 1600 | 148px | 13.45% | 108px | 6.75% | 0.5% |
3âž” | swapped_labels mask | juice_bottle_logical_anomalies_065.png | 1600 x 800 | 344px | 21.5% | 303px | 37.88% | 8.12% |
4âž” | swapped_labels mask | juice_bottle_logical_anomalies_065.png | 1600 x 800 | 323px | 20.19% | 330px | 41.25% | 7.92% |
5âž” | bag_broken mask | screw_bag_structural_anomalies_007.png | 1100 x 1600 | 253px | 23% | 175px | 10.94% | 1.47% |
6âž” | color mask | screw_bag_structural_anomalies_051.png | 1100 x 1600 | 83px | 7.55% | 70px | 4.38% | 0.21% |
7âž” | wrong_ratio mask | breakfast_box_logical_anomalies_075.png | 1280 x 1600 | 402px | 31.41% | 677px | 42.31% | 10.97% |
8âž” | swapped_labels mask | juice_bottle_logical_anomalies_063.png | 1600 x 800 | 344px | 21.5% | 303px | 37.88% | 8.11% |
9âž” | swapped_labels mask | juice_bottle_logical_anomalies_063.png | 1600 x 800 | 304px | 19% | 330px | 41.25% | 7.6% |
10âž” | 2_very_short_screws mask | screw_bag_logical_anomalies_090.png | 1100 x 1600 | 197px | 17.91% | 195px | 12.19% | 0.78% |
License #
MVTec LOCO AD: MVTec Logical Constraints Anomaly Detection is under CC BY-NC-SA 4.0 license.
Citation #
If you make use of the MVTec LOCO AD data, please cite the following reference:
Paul Bergmann, Kilian Batzner, Michael Fauser, David Sattlegger, and Carsten Steger,
"Beyond Dents and Scratches: Logical Constraints in Unsupervised Anomaly Detection and Localization",
International Journal of Computer Vision (IJCV), 2022
If you are happy with Dataset Ninja and use provided visualizations and tools in your work, please cite us:
@misc{ visualization-tools-for-mvtec-loco-ad-dataset,
title = { Visualization Tools for MVTec LOCO AD Dataset },
type = { Computer Vision Tools },
author = { Dataset Ninja },
howpublished = { \url{ https://datasetninja.com/mvtec-loco-ad } },
url = { https://datasetninja.com/mvtec-loco-ad },
journal = { Dataset Ninja },
publisher = { Dataset Ninja },
year = { 2024 },
month = { may },
note = { visited on 2024-05-17 },
}
Download #
Dataset MVTec LOCO AD can be downloaded in Supervisely format:
As an alternative, it can be downloaded with dataset-tools package:
pip install --upgrade dataset-tools
… using following python code:
import dataset_tools as dtools
dtools.download(dataset='MVTec LOCO AD', dst_dir='~/dataset-ninja/')
Make sure not to overlook the python code example available on the Supervisely Developer Portal. It will give you a clear idea of how to effortlessly work with the downloaded dataset.
The data in original format can be downloaded here.
Disclaimer #
Our gal from the legal dep told us we need to post this:
Dataset Ninja provides visualizations and statistics for some datasets that can be found online and can be downloaded by general audience. Dataset Ninja is not a dataset hosting platform and can only be used for informational purposes. The platform does not claim any rights for the original content, including images, videos, annotations and descriptions. Joint publishing is prohibited.
You take full responsibility when you use datasets presented at Dataset Ninja, as well as other information, including visualizations and statistics we provide. You are in charge of compliance with any dataset license and all other permissions. You are required to navigate datasets homepage and make sure that you can use it. In case of any questions, get in touch with us at hello@datasetninja.com.