Introduction #
The authors unveiled the NDD20: The Northumberland Dolphin Dataset 2020, an intricate image dataset meticulously annotated for both coarse and fine-grained instance segmentation and categorization. This dataset was conceived to address the burgeoning integration of computer vision into conservation research and the development of field-deployable systems tailored for extreme environmental conditions β an area notably lacking in open-source datasets. NDD20 comprises an extensive array of above and below water images capturing two distinct dolphin species, meticulously annotated for both coarse and fine-grained segmentation. All data within NDD20 was painstakingly collected manually in the North Sea along the Northumberland coastline, UK.
Motivation
Conservation presents a promising avenue for the application of computer vision. Cetacean conservation, in particular, stands to gain significant benefits from the integration of computer vision tools. In the realm of studying cetacean population dynamics and health, researchers often rely on manual methods like photo ID, which involves identifying individuals based on unique characteristics. However, this manual identification process is time-consuming, often spanning several months. Introducing computer vision systems capable of fine-grained cetacean classification could provide researchers with more field time and reduce the time spent processing collected data.
Despite the potential, the availability of open-source datasets tailored for conservation or ecological purposes is notably scarce. Existing datasets mostly focus on simple animal detection in scenes, often limited to specific subsets like pets or birds. While some large-scale datasets showcase animals in natural settings, they typically provide species-level labels, insufficient for precise population estimation requiring individual identification.
Though challenging, cetacean researchers have manually identified individuals for over four decades by noting prominent markings on fins as they breach the waterline. This longstanding practice suggests the feasibility of an automated photo-ID process using computer vision, with ongoing efforts in this direction already underway.
Data collection
Data collection for NDD20 involved two distinct fieldwork endeavors. below water data gathering comprised 36 opportunistic surveys of the Farne Deeps, a glacial trench known for its rich biodiversity, located approximately 14-20 nautical miles offshore from Seahouses, UK. These surveys spanned from 2011 to 2018. On the other hand, above water data collection involved 27 surveys along a designated stretch of the Northumberland coast known as the Coquet to St. Maryβs Marine Conservation Zone (MCZ). above water photographs were captured using a DSLR camera from a small rigid inflatable boat during days characterized by fair weather and favorable sea conditions (typically less than four on the Beaufort scale). below water images in the dataset were extracted from high-definition video footage filmed with GoPro Hero 3 and GoPro Hero 4 cameras, operated by a diver under optimal sea conditions. Initially, above water surveys adhered to predetermined transect lines but later transitioned to more opportunistic approaches, leveraging shore-based volunteer observations shared on dedicated social media platforms.
Dataset description
NDD20 encompasses a diverse range of image data categorized into two primary sections: above and below water. above water images, captured from the deck of a research vessel, adhere to the conventional data format widely used in cetacean research. In this format, individuals are typically identified based on the structure of their dorsal fins. Conversely, below water images, although less common, offer additional identifying features such as overall coloration, distinctive body markings, scars, and patterns resulting from injury or skin conditions.
To safeguard ongoing cetacean research endeavors, a pseudo-anonymization process has been undertaken. However, this does not diminish the dataβs significance for computer vision researchers. Notably, images with sequential filenames were not captured sequentially, and individual IDs have been randomly assigned numerical values instead of the codes used by Northumberland cetacean researchers. Furthermore, all EXIF data contained within the images has been removed.
Above water images
NDD20 comprises a total of 2201 above water images, each accompanied by a JSON file. This JSON file provides detailed annotations for each image, including sets of (x,y) coordinates indicating regions of interest within the image. These coordinates are supplemented with attribute labels indicating various levels of difficulty in the recognition task. The first attribute level, labeled dolphin, delineates the area of the image containing any portion of a dolphin visible above the waterline at the time of capture. This attribute presents a standard, albeit challenging, instance segmentation task. Approximately 2900 masks are present in the above-water data, as some images feature multiple masks. The second attribute denotes the dolphinβs species, either BND (bottle nose dolphin) or WBD (white beaked dolphin), corresponding to tursiops truncatus and lagenorhynchus albirostris, respectively. This aspect poses a fine-grained categorization challenge due to subtle inter-species differences. Although all above-water masks bear this label, thereβs an imbalance in class distribution, with 73% labeled as BND. The final attribute label signifies individual dolphin identifications, meticulously determined by cetacean researchers specializing in the Northumberland coastal area. Around 14% of masks contain an id attribute, with 44 distinct classes present. Once again, this distribution imbalance presents both a fine-grained and few-shot learning challenge.
The number of above water images per ID class.
Identification based on individual id class labels poses the most daunting fine-grained challenge within NDD20, primarily because individual recognition beyond human facial recognition remains largely unexplored in research. To discern individuals within the dataset, nuances such as scarring or pigmentation on the individualβs surface, alongside the distinctive shape of the dorsal fin, must be meticulously considered. Moreover, the dataset contains only a limited number of examples for each individual, thereby presenting both a fine-grained and few-shot learning task. Several hurdles exist within the above-water data that necessitate overcoming. These challenges include images featuring small regions of interest, extensive blur or water splashes, partially obscured dolphin fins, photographs taken from oblique angles, and markings crucial for identification being present solely on one side of the fin.
Example above water images. Both images contain one mask with the following attributes: Left - object dolphin, species WBD, id:11. Right - object:dolphin, species BND, id:8.
Below water images
The 2201 below water images presented constitute a subset of a much broader image collection dating back to 2011. The labeling methodology for these images mirrors that of the above water images. The authors supply a JSON file containing coordinates for manually drawn mask annotations and multiple attribute labels. Similarly to the above water images, the first attribute level pertains to dolphin, representing areas within the image where any part of the object is visible. However, in contrast to the above-water images, all below water images feature at least one mask with an id attribute, encompassing 82 classes. Variations in the frequency of appearance among id class labels arise due to the data collection process involving free-roaming individuals, thereby presenting a genuine few-shot learning challenge reflective of real-world scenarios. Unlike the above water images, no species label is provided for the below water images, as all images depict lagenorhynchus albirostris. Additionally, below water images are tagged with an out of focus flag, indicating whether the individual is deemed to be out of focus.
The number of below water images per ID class.
The primary obstacles concerning the below-water images revolve around water clarity, influenced by factors like algae blooms and sunlight refraction. These elements can obscure areas of the individual crucial for identification or introduce artificial markings that impede the process. Many challenges akin to those encountered with above-water images are also pertinent here, particularly the probability that unique features are exclusive to one side of the body.
Example below water images. Both images contain one mask with the following attributes: Left - object dolphin, id:9, out of focus false. Right - object dolphin, id:30, out of focus false.
Summary #
NDD20: The Northumberland Dolphin Dataset 2020 is a dataset for semantic segmentation, object detection, and identification tasks. It is used in the environmental research.
The dataset consists of 4402 images with 6103 labeled objects belonging to 1 single class (dolphin).
Images in the NDD20 dataset have pixel-level semantic segmentation annotations. All images are labeled (i.e. with annotations). There are no pre-defined train/val/test splits in the dataset. Alternatively, the dataset could be split into 2 positions: above (2201 images) and below (2201 images), or into 2 dolphins species: tursiops truncatus (2668 objects) and lagenorhynchus albirostris (271 objects). Additionally, some labels marked with dolphin id and out of focus tags, explore them in supervisely labeling tool. The dataset was released in 2020 by the Newcastle University, UK.
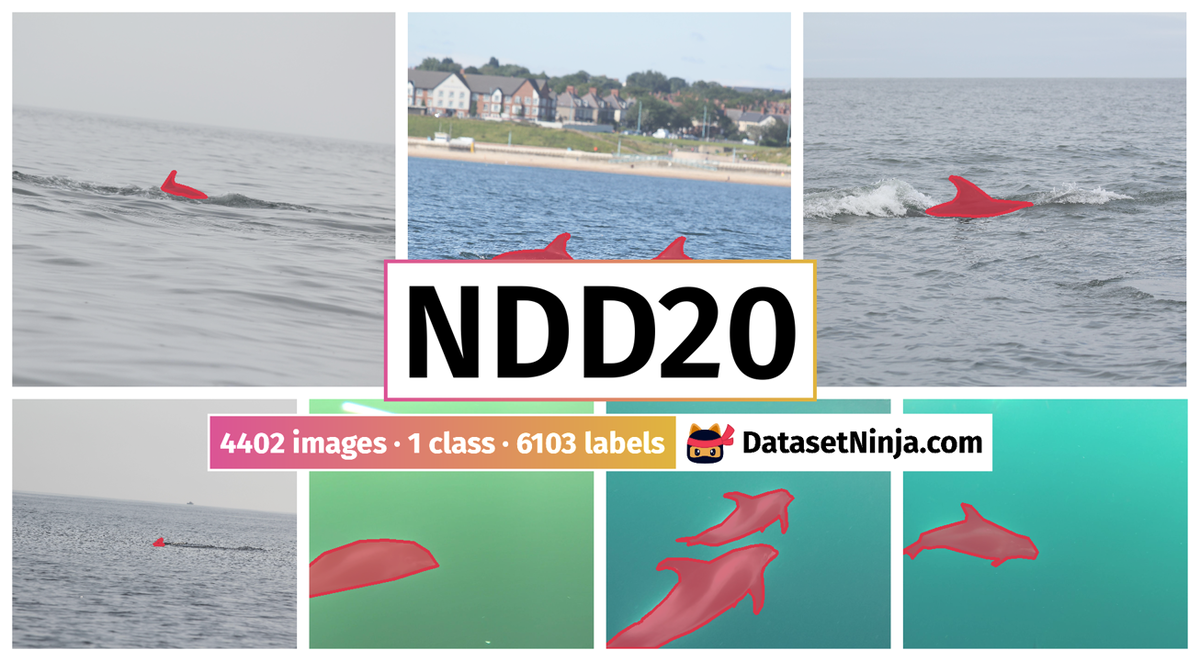
Explore #
NDD20 dataset has 4402 images. Click on one of the examples below or open "Explore" tool anytime you need to view dataset images with annotations. This tool has extended visualization capabilities like zoom, translation, objects table, custom filters and more. Hover the mouse over the images to hide or show annotations.
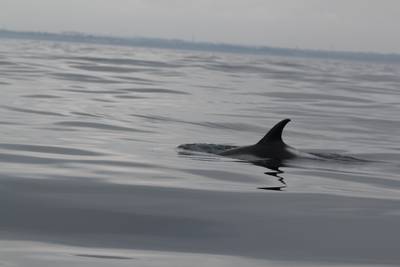
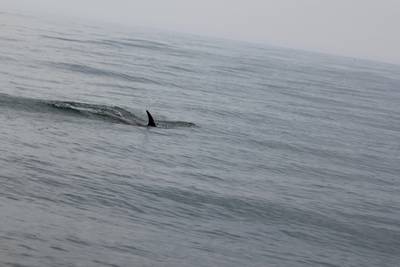
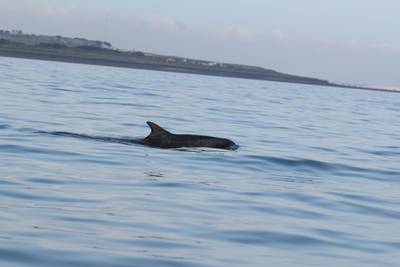
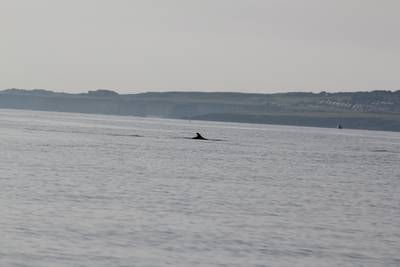
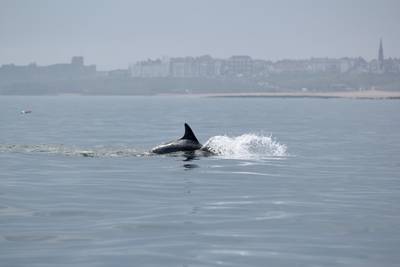
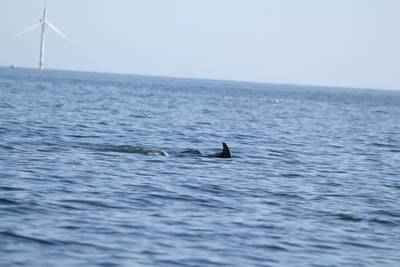
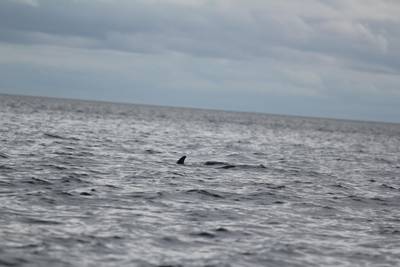


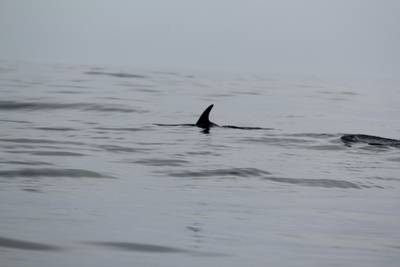
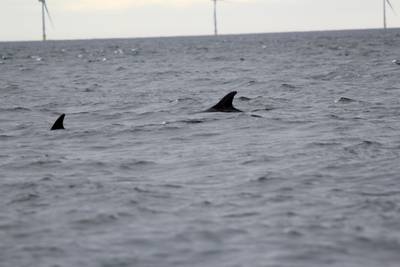
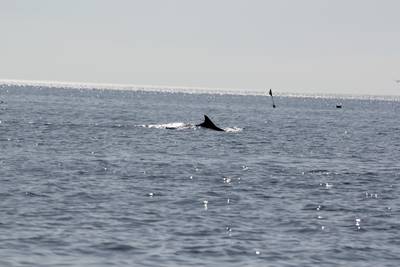

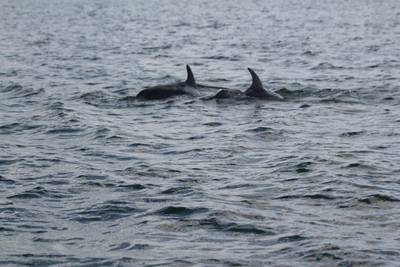
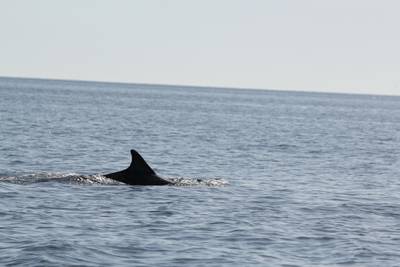
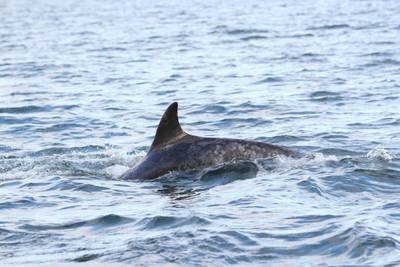
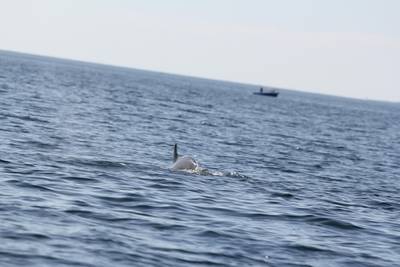
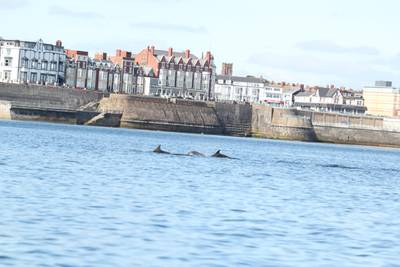
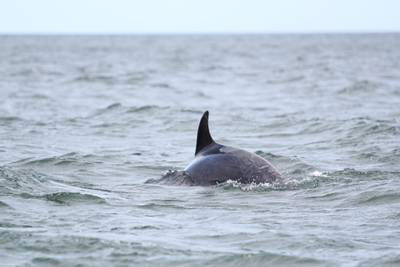
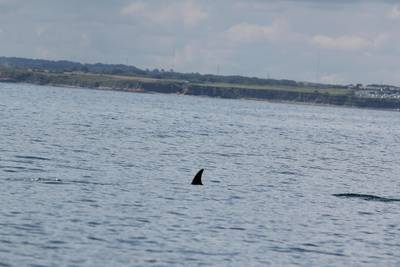
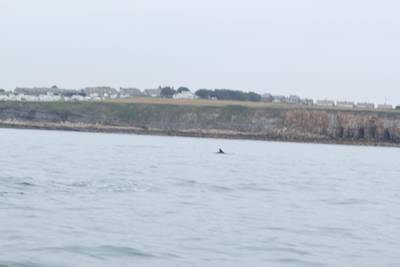
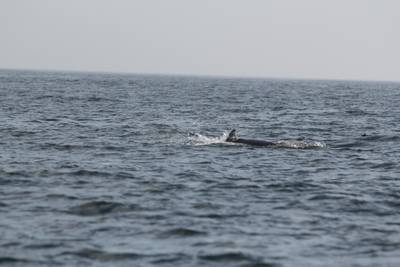
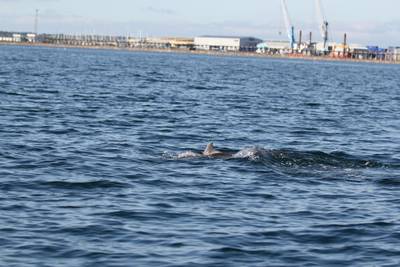
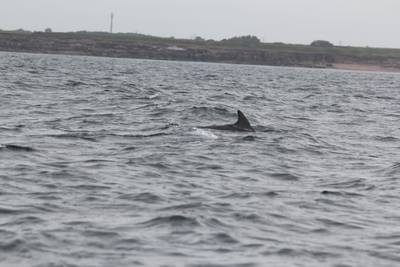
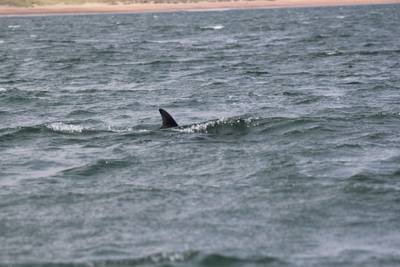
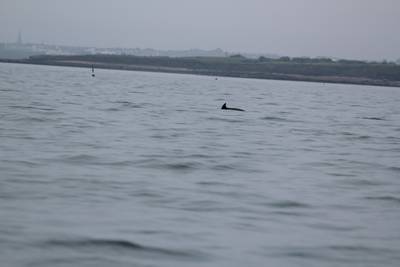
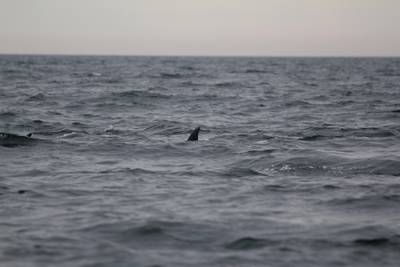
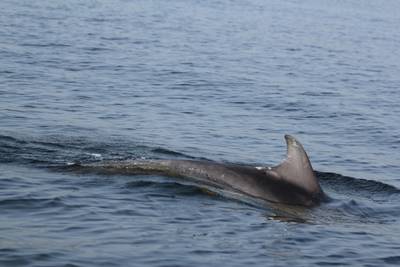
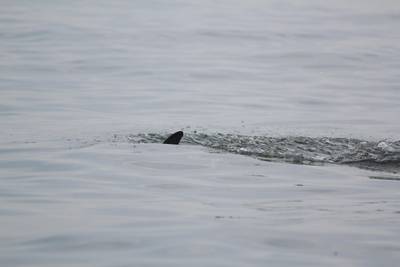
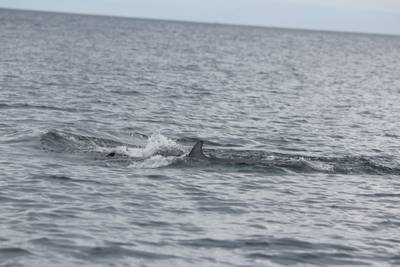
Class balance #
There are 1 annotation classes in the dataset. Find the general statistics and balances for every class in the table below. Click any row to preview images that have labels of the selected class. Sort by column to find the most rare or prevalent classes.
Class γ
€ | Images γ
€ | Objects γ
€ | Count on image average | Area on image average |
---|---|---|---|---|
dolphinβ polygon | 4402 | 6103 | 1.39 | 4.93% |
Images #
Explore every single image in the dataset with respect to the number of annotations of each class it has. Click a row to preview selected image. Sort by any column to find anomalies and edge cases. Use horizontal scroll if the table has many columns for a large number of classes in the dataset.
Object distribution #
Interactive heatmap chart for every class with object distribution shows how many images are in the dataset with a certain number of objects of a specific class. Users can click cell and see the list of all corresponding images.
Class sizes #
The table below gives various size properties of objects for every class. Click a row to see the image with annotations of the selected class. Sort columns to find classes with the smallest or largest objects or understand the size differences between classes.
Class | Object count | Avg area | Max area | Min area | Min height | Min height | Max height | Max height | Avg height | Avg height | Min width | Min width | Max width | Max width |
---|---|---|---|---|---|---|---|---|---|---|---|---|---|---|
dolphin polygon | 6103 | 3.53% | 49.7% | 0% | 26px | 0.75% | 3445px | 99.91% | 410px | 25.28% | 31px | 0.6% | 5176px | 99.85% |
Spatial Heatmap #
The heatmaps below give the spatial distributions of all objects for every class. These visualizations provide insights into the most probable and rare object locations on the image. It helps analyze objects' placements in a dataset.
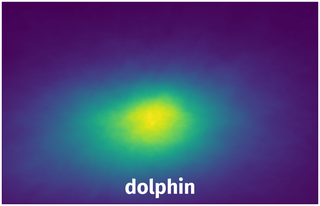
Objects #
Table contains all 6103 objects. Click a row to preview an image with annotations, and use search or pagination to navigate. Sort columns to find outliers in the dataset.
Object ID γ
€ | Class γ
€ | Image name click row to open | Image size height x width | Height γ
€ | Height γ
€ | Width γ
€ | Width γ
€ | Area γ
€ |
---|---|---|---|---|---|---|---|---|
1β | dolphin polygon | below_1584.jpg | 1080 x 1920 | 331px | 30.65% | 153px | 7.97% | 1.02% |
2β | dolphin polygon | above_2175.jpg | 3456 x 5184 | 222px | 6.42% | 348px | 6.71% | 0.14% |
3β | dolphin polygon | above_1026.jpg | 3456 x 5184 | 356px | 10.3% | 824px | 15.9% | 0.59% |
4β | dolphin polygon | above_1026.jpg | 3456 x 5184 | 213px | 6.16% | 392px | 7.56% | 0.16% |
5β | dolphin polygon | above_1026.jpg | 3456 x 5184 | 218px | 6.31% | 263px | 5.07% | 0.12% |
6β | dolphin polygon | below_1599.jpg | 1080 x 1920 | 123px | 11.39% | 306px | 15.94% | 0.69% |
7β | dolphin polygon | below_1599.jpg | 1080 x 1920 | 385px | 35.65% | 607px | 31.61% | 3.28% |
8β | dolphin polygon | below_1599.jpg | 1080 x 1920 | 352px | 32.59% | 483px | 25.16% | 2.79% |
9β | dolphin polygon | above_842.jpg | 3456 x 5184 | 796px | 23.03% | 1725px | 33.28% | 2.97% |
10β | dolphin polygon | above_1831.jpg | 3648 x 5472 | 523px | 14.34% | 535px | 9.78% | 0.74% |
License #
NDD20: The Northumberland Dolphin Dataset 2020 is under CC BY-NC-SA 4.0 license.
Citation #
If you make use of the Northumberland Dolphin data, please cite the following reference:
@inproceedings{Trotter2020NDD20AL,
title={NDD20: A large-scale few-shot dolphin dataset for coarse and fine-grained categorisation},
author={Cameron Trotter and Georgia Atkinson and Matt Sharpe and Kirsten Richardson and A. Stephen McGough and Nick Wright and Ben Burville and Per Berggren},
year={2020}
}
If you are happy with Dataset Ninja and use provided visualizations and tools in your work, please cite us:
@misc{ visualization-tools-for-northumberland-dolphin-dataset,
title = { Visualization Tools for NDD20 Dataset },
type = { Computer Vision Tools },
author = { Dataset Ninja },
howpublished = { \url{ https://datasetninja.com/northumberland-dolphin } },
url = { https://datasetninja.com/northumberland-dolphin },
journal = { Dataset Ninja },
publisher = { Dataset Ninja },
year = { 2025 },
month = { jul },
note = { visited on 2025-07-04 },
}
Download #
Dataset NDD20 can be downloaded in Supervisely format:
As an alternative, it can be downloaded with dataset-tools package:
pip install --upgrade dataset-tools
β¦ using following python code:
import dataset_tools as dtools
dtools.download(dataset='NDD20', dst_dir='~/dataset-ninja/')
Make sure not to overlook the python code example available on the Supervisely Developer Portal. It will give you a clear idea of how to effortlessly work with the downloaded dataset.
The data in original format can be downloaded here.
Disclaimer #
Our gal from the legal dep told us we need to post this:
Dataset Ninja provides visualizations and statistics for some datasets that can be found online and can be downloaded by general audience. Dataset Ninja is not a dataset hosting platform and can only be used for informational purposes. The platform does not claim any rights for the original content, including images, videos, annotations and descriptions. Joint publishing is prohibited.
You take full responsibility when you use datasets presented at Dataset Ninja, as well as other information, including visualizations and statistics we provide. You are in charge of compliance with any dataset license and all other permissions. You are required to navigate datasets homepage and make sure that you can use it. In case of any questions, get in touch with us at hello@datasetninja.com.