Introduction #
The authors of the OpenCows2020 dataset undertook a study focused on Holstein-Friesian cattle, which are known for their distinct black and white coat patterns resembling those generated by Turing’s reaction-diffusion systems. Their research aimed to automate the visual detection and biometric identification of individual Holstein-Friesian cattle using convolutional neural networks and deep metric learning techniques. This approach was conceived as an alternative to existing methods that rely on physical markings, tags, or wearables, all of which have varying maintenance requirements.
In the study, the authors introduced an entirely hands-off method for the automated detection, localization, and identification of individual cattle from overhead imagery, even in open herd settings where new additions to the herd could be identified without the need for retraining the system. Their findings indicated that deep metric learning systems exhibited robust performance, achieving an accuracy of 93.8% when trained on only half of the cattle population.
Traditional methods of traceability involve national tracking databases and unique ear-tag identification, injectable transponders, branding, and more. However, these methods are limited in providing continuous localization of individuals, which is crucial for applications in precision farming and various research areas.
To address this limitation, the authors proposed leveraging the inherent and characteristic coat patterns of Holstein-Friesian cattle for non-intrusive visual identification, laying the foundation for continuous monitoring of herds on an individual level through non-intrusive visual observation.
The study delineated two scenarios: <i>
closed-set identification</i>
, where the system is trained and tested on a fixed set of known cattle, and <i>
open-set identification</i>
, where the system should identify cattle that have never been seen before without retraining. They presented a comprehensive pipeline for detection and open-set recognition, allowing for the flexible identification of individual cattle in real-world scenarios.
The OpenCows2020 dataset includes indoor and outdoor top-down imagery for cattle detection, localization, and open-set identification. The dataset comprised a total of 3,707 non-synthetic and 3336 augmented synthetic images. For open-set identification, they included 46 individuals with an average of 103 instances per class and 4,736 regions overall. The dataset was carefully split into training, validation, and testing sets to support 10-fold 8:1:1 cross-validation. Download original dataset to get detailed info about cross-validation.
Identification Instance Distribution. The distribution of instances per class for the identification component of the OpenCows2020 dataset. Instances were then randomly split to have exactly 10 testing instances per class whilst those remaining were split into training and validation in a ratio of 9 : 1, respectively. Also labelled is the source of each group of categories. ( a ), ( b ) and ( c )
Their work aimed to advance non-intrusive monitoring of cattle, applicable to precision farming, automated productivity assessment, health and welfare monitoring, and veterinary research, including behavioral analysis and disease outbreak tracing.
Summary #
OpenCow2020: Visual Identification of Individual Holstein Friesian Cattle via Deep Metric Learning is a dataset for object detection and identification tasks. It is used in the livestock industry.
The dataset consists of 11779 images with 13026 labeled objects belonging to 1 single class (cow).
Images in the OpenCow2020 dataset have bounding box annotations. There are 4740 (40% of the total) unlabeled images (i.e. without annotations). There are 3 splits in the dataset: detection_and_localisation (7043 images), identification-train (4240 images), and identification-test (496 images). Alternatively, the dataset could be split into 2 detection_and_localisation subsets: non-synthetic (3707 images) and synthetic (3336 images). Additionally, in identification-test and identification-train splits information about cow_id and data source is provided. The dataset was released in 2020 by the University of Bristol, UK.
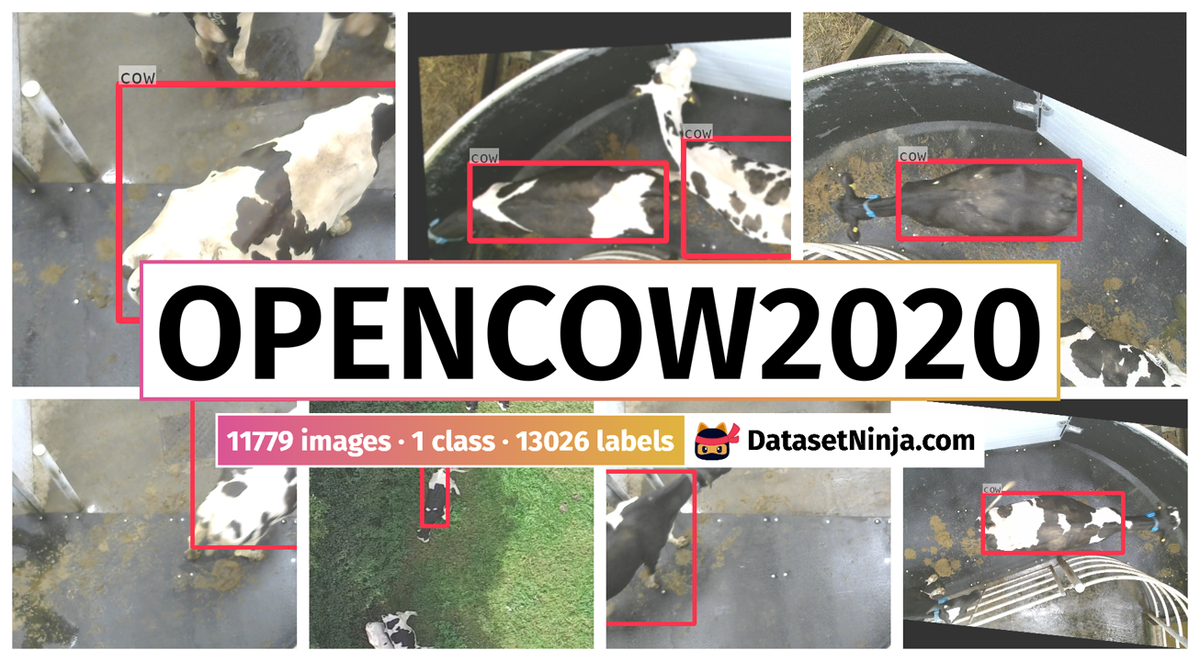
Explore #
OpenCow2020 dataset has 11779 images. Click on one of the examples below or open "Explore" tool anytime you need to view dataset images with annotations. This tool has extended visualization capabilities like zoom, translation, objects table, custom filters and more. Hover the mouse over the images to hide or show annotations.
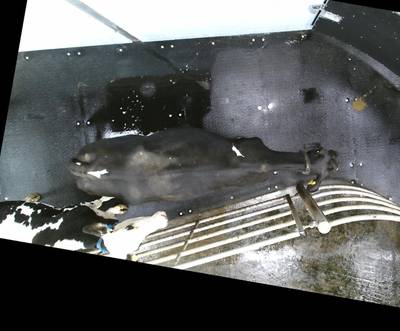
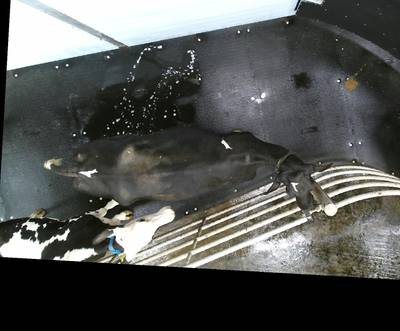
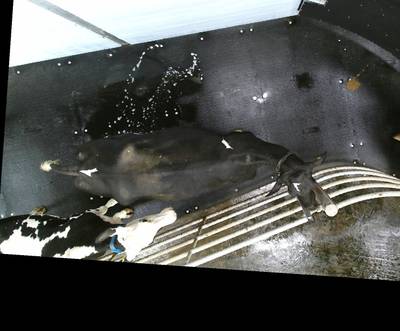

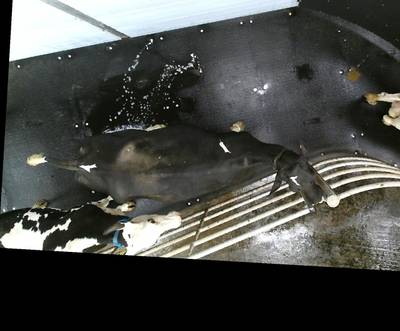
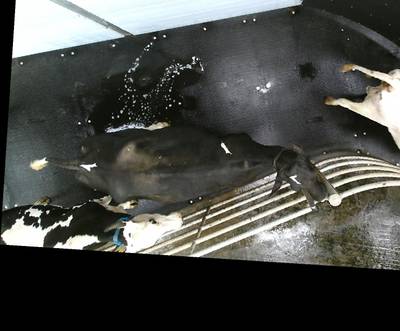
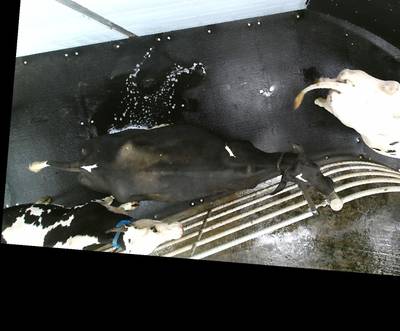
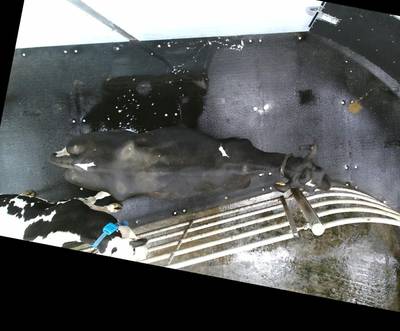
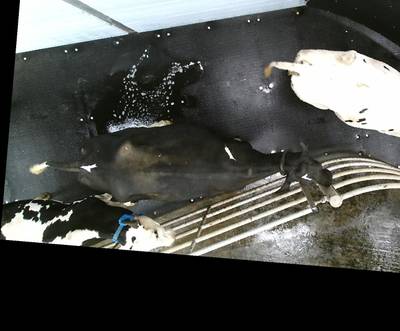

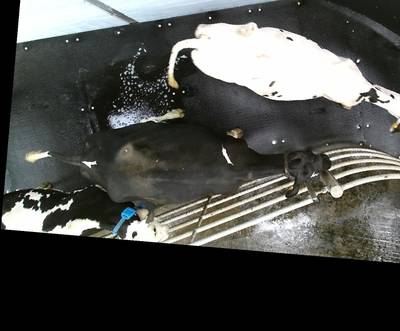
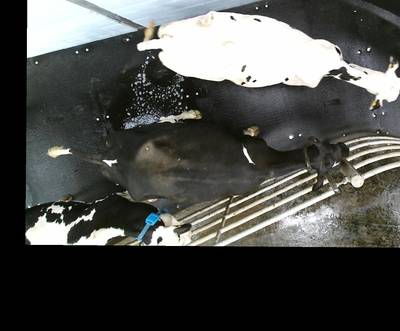
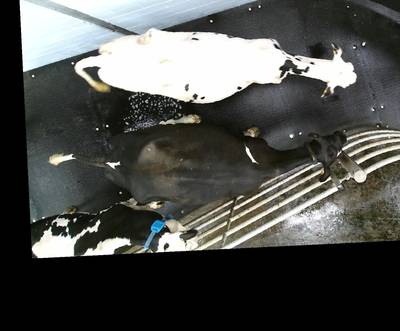
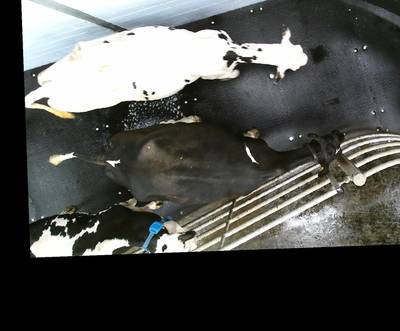
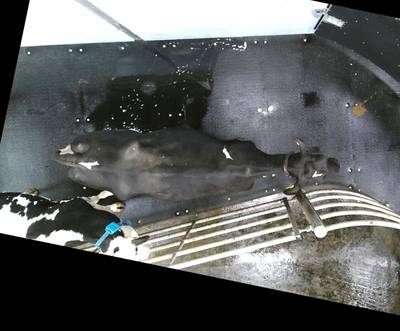
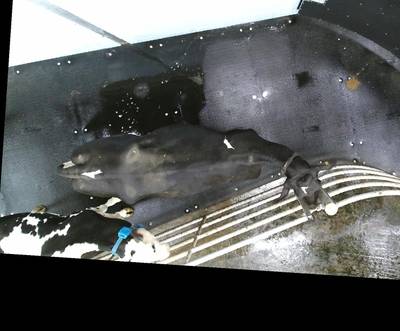

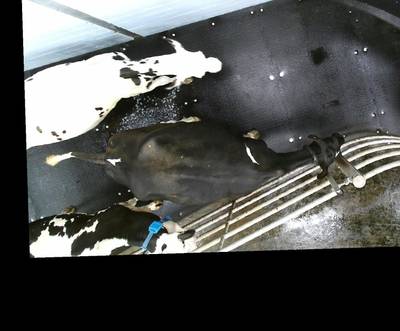
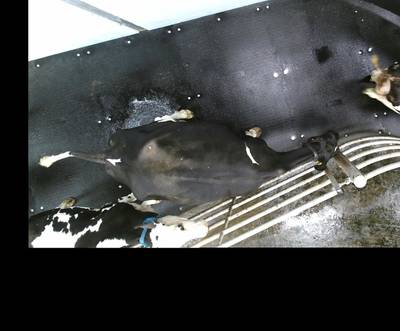

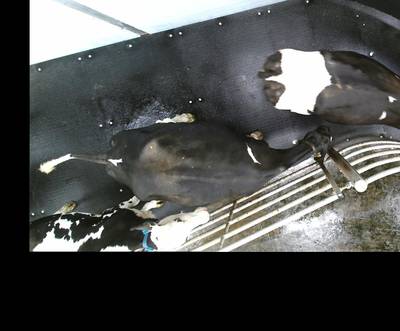
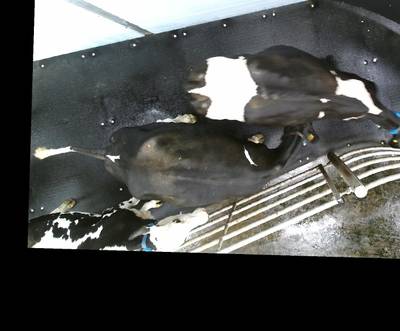
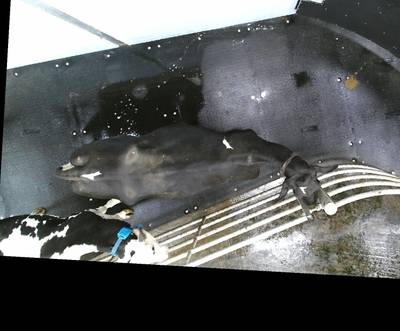
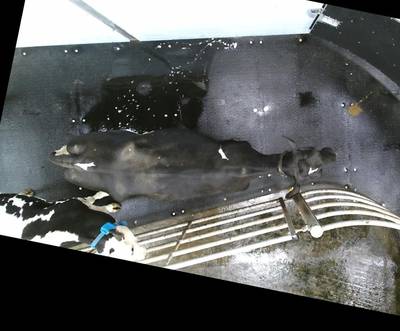
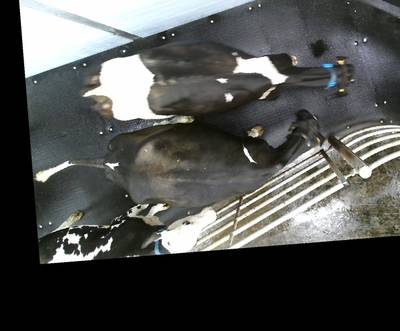
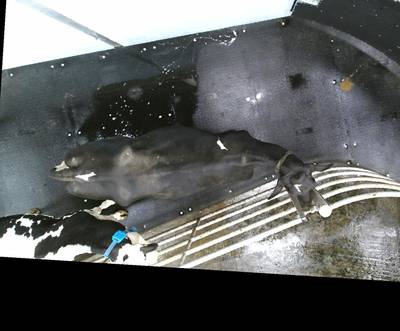
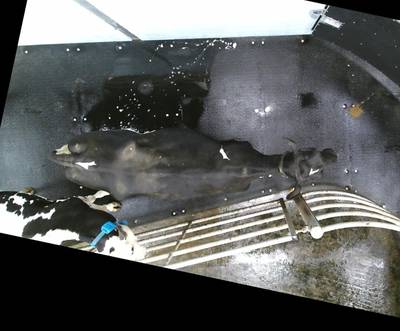
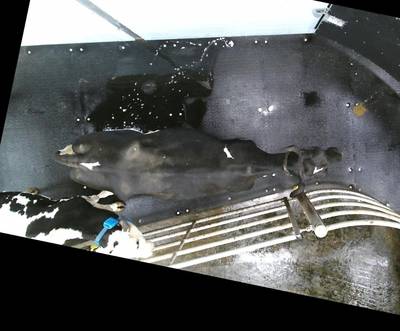

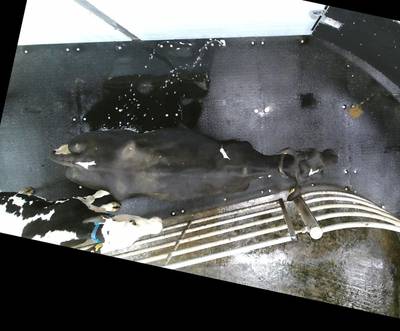
Class balance #
There are 1 annotation classes in the dataset. Find the general statistics and balances for every class in the table below. Click any row to preview images that have labels of the selected class. Sort by column to find the most rare or prevalent classes.
Class ã…¤ | Images ã…¤ | Objects ã…¤ | Count on image average | Area on image average |
---|---|---|---|---|
cowâž” rectangle | 7039 | 13026 | 1.85 | 18.67% |
Images #
Explore every single image in the dataset with respect to the number of annotations of each class it has. Click a row to preview selected image. Sort by any column to find anomalies and edge cases. Use horizontal scroll if the table has many columns for a large number of classes in the dataset.
Object distribution #
Interactive heatmap chart for every class with object distribution shows how many images are in the dataset with a certain number of objects of a specific class. Users can click cell and see the list of all corresponding images.
Class sizes #
The table below gives various size properties of objects for every class. Click a row to see the image with annotations of the selected class. Sort columns to find classes with the smallest or largest objects or understand the size differences between classes.
Class | Object count | Avg area | Max area | Min area | Min height | Min height | Max height | Max height | Avg height | Avg height | Min width | Min width | Max width | Max width |
---|---|---|---|---|---|---|---|---|---|---|---|---|---|---|
cow rectangle | 13026 | 10.56% | 64.25% | 0.02% | 2px | 0.23% | 1023px | 99.86% | 245px | 29.53% | 4px | 0.55% | 1019px | 99.32% |
Spatial Heatmap #
The heatmaps below give the spatial distributions of all objects for every class. These visualizations provide insights into the most probable and rare object locations on the image. It helps analyze objects' placements in a dataset.
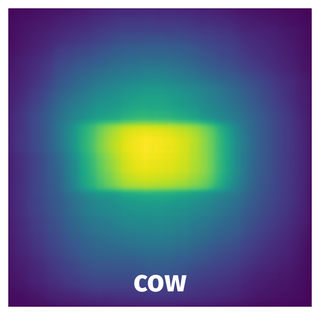
Objects #
Table contains all 13026 objects. Click a row to preview an image with annotations, and use search or pagination to navigate. Sort columns to find outliers in the dataset.
Object ID ã…¤ | Class ã…¤ | Image name click row to open | Image size height x width | Height ã…¤ | Height ã…¤ | Width ã…¤ | Width ã…¤ | Area ã…¤ |
---|---|---|---|---|---|---|---|---|
1âž” | cow rectangle | 005840.jpg | 720 x 1280 | 355px | 49.31% | 504px | 39.38% | 19.41% |
2âž” | cow rectangle | 005840.jpg | 720 x 1280 | 229px | 31.81% | 628px | 49.06% | 15.6% |
3âž” | cow rectangle | 006684.jpg | 720 x 1280 | 536px | 74.44% | 490px | 38.28% | 28.5% |
4âž” | cow rectangle | 000173.jpg | 1230 x 1486 | 259px | 21.06% | 594px | 39.97% | 8.42% |
5âž” | cow rectangle | 000173.jpg | 1230 x 1486 | 264px | 21.46% | 689px | 46.37% | 9.95% |
6âž” | cow rectangle | 006113.jpg | 864 x 1536 | 143px | 16.55% | 214px | 13.93% | 2.31% |
7âž” | cow rectangle | 006113.jpg | 864 x 1536 | 153px | 17.71% | 182px | 11.85% | 2.1% |
8âž” | cow rectangle | 006113.jpg | 864 x 1536 | 154px | 17.82% | 170px | 11.07% | 1.97% |
9âž” | cow rectangle | 006113.jpg | 864 x 1536 | 144px | 16.67% | 190px | 12.37% | 2.06% |
10âž” | cow rectangle | 006113.jpg | 864 x 1536 | 118px | 13.66% | 199px | 12.96% | 1.77% |
License #
Citation #
If you make use of the OpenCow2020 data, please cite the following reference:
William Andrew, Tilo Burghardt, Neill Campbell, Jing Gao (2020): OpenCows2020.
https://doi.org/10.5523/bris.10m32xl88x2b61zlkkgz3fml17
If you are happy with Dataset Ninja and use provided visualizations and tools in your work, please cite us:
@misc{ visualization-tools-for-opencows2020-dataset,
title = { Visualization Tools for OpenCow2020 Dataset },
type = { Computer Vision Tools },
author = { Dataset Ninja },
howpublished = { \url{ https://datasetninja.com/opencows2020 } },
url = { https://datasetninja.com/opencows2020 },
journal = { Dataset Ninja },
publisher = { Dataset Ninja },
year = { 2025 },
month = { feb },
note = { visited on 2025-02-22 },
}
Download #
Dataset OpenCow2020 can be downloaded in Supervisely format:
As an alternative, it can be downloaded with dataset-tools package:
pip install --upgrade dataset-tools
… using following python code:
import dataset_tools as dtools
dtools.download(dataset='OpenCow2020', dst_dir='~/dataset-ninja/')
Make sure not to overlook the python code example available on the Supervisely Developer Portal. It will give you a clear idea of how to effortlessly work with the downloaded dataset.
The data in original format can be downloaded here.
Disclaimer #
Our gal from the legal dep told us we need to post this:
Dataset Ninja provides visualizations and statistics for some datasets that can be found online and can be downloaded by general audience. Dataset Ninja is not a dataset hosting platform and can only be used for informational purposes. The platform does not claim any rights for the original content, including images, videos, annotations and descriptions. Joint publishing is prohibited.
You take full responsibility when you use datasets presented at Dataset Ninja, as well as other information, including visualizations and statistics we provide. You are in charge of compliance with any dataset license and all other permissions. You are required to navigate datasets homepage and make sure that you can use it. In case of any questions, get in touch with us at hello@datasetninja.com.