Introduction #
The authors introduce the PACO-LVIS dataset, strategically selecting vocabularies for objects, parts, and attributes by leveraging the strengths of the LVIS dataset. They identified 75 common object categories shared between both datasets, chose 200 parts classes from web-mined data, which expanded to 456 when accounting for object-specific parts. Additionally, a user study helped define a comprehensive set of 29 colors, 10 patterns, 13 materials, and 3 levels of reflectance attributes to enhance the dataset’s object recognition and understanding capabilities.
Image sources
PACO is constructed from LVIS (available on DatasetNinja) in the image domain (current dataset) and Ego4D in the video domain. Authors chose LVIS due to its large object vocabulary and federated dataset construction. Ego4D has temporally aligned narrations, making it easy to source frames corresponding to specific objects.
Object vocabulary selection
Authors first mined all object categories mentioned in the narrations accompanying Ego4D and took the intersection with common and frequent categories in LVIS. Authors then chose categories with at-least 20 instances in Ego4D, resulting in 75 categories commonly found in both LVIS and Ego4D.
Parts vocabulary selection
Excluding specific domains like fashion, there is no exhaustive ontology of parts for common objects. Authors mined part names from web-images obtained through queries like “parts of a car”. These images list part-names along with illustrations and pointers to the parts in the object. Authors manually curate such mined part names for an object category to only retain parts that are visible in majority of the object instances and clearly distinguishable. More details in the appendix. This resulted in a total of 200 part classes shared across all 75 objects. When expanded to object-specific parts this results in 456 object-part classes.
Attribute vocabulary selection
Attributes are particularly useful in distinguishing different instances of the same object type. Motivated by this, authors conducted an in-depth user study (details in appendix) to identify the sufficient set of attributes that can separate all object instances in the dataset. This led to the final vocabulary of 29 colors, 10 patterns and markings, 13 materials and 3 levels of reflectance.

(left) PACO includes objects with object masks, object attributes, part masks, and part attributes. (right) Object instance queries composed of object and part attributes are shown with corresponding positive images in green and negative images in red.

Dataset Statistics. (a) shows the distribution of instances across the 456 object-part categories. (b) shows the size distribution of object and part masks in PACO-LVIS. (c) shows the distribution of the 55 attribute classes across instances in PACO-LVIS.
Please note, bad data detected.
Summary #
PACO-LVIS: Parts and Attributes of Common Objects - Large Vocabulary Instance Segmentation is a dataset for instance segmentation, object detection, and semantic segmentation tasks. It is applicable or relevant across various domains.
The dataset consists of 57643 images with 1644461 labeled objects belonging to 270 different classes including body, rim, handle, and other: base, neck, side, inner_body, leg, bottom, back, strap, top, seat, wheel, inner_side, mirror, lid, bowl, blade, case, shoulder, pipe, screen, foot, button, cover, logo, bench, and 242 more.
Images in the PACO-LVIS dataset have pixel-level instance segmentation annotations. Due to the nature of the instance segmentation task, it can be automatically transformed into a semantic segmentation (only one mask for every class) or object detection (bounding boxes for every object) tasks. All images are labeled (i.e. with annotations). There are 3 splits in the dataset: train2017 (45790 images), test2017 (9443 images), and val2017 (2410 images). Also, the objects contains color, pattern marking, material, transparency and part_of object tags. Explore them in the supervisely labeling tool. The dataset was released in 2023 by the Meta AI and Simon Fraser University, Canada.
Here is a visualized example for randomly selected sample classes:
Explore #
PACO-LVIS dataset has 57643 images. Click on one of the examples below or open "Explore" tool anytime you need to view dataset images with annotations. This tool has extended visualization capabilities like zoom, translation, objects table, custom filters and more. Hover the mouse over the images to hide or show annotations.
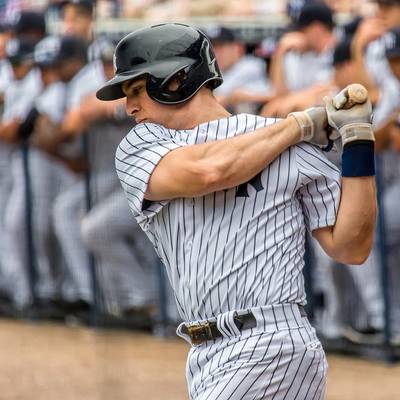
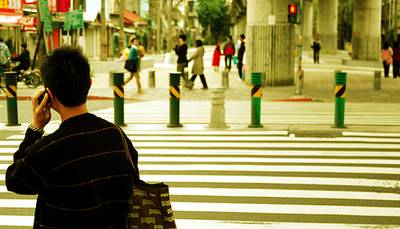
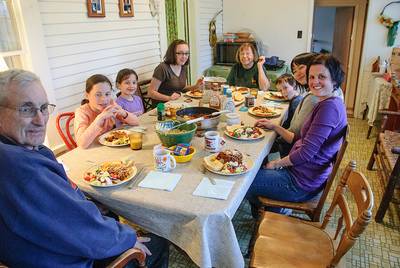
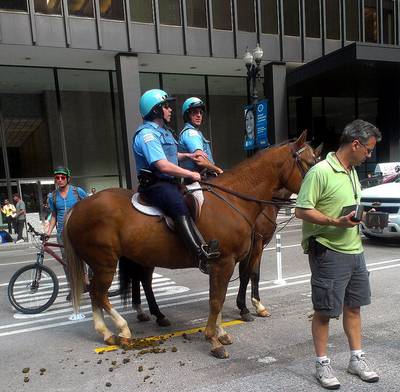
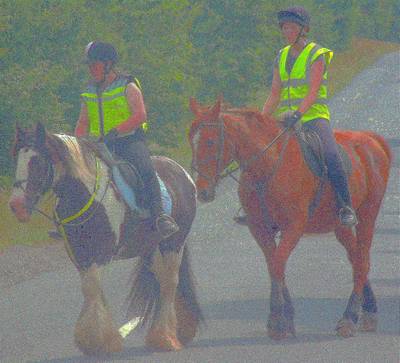
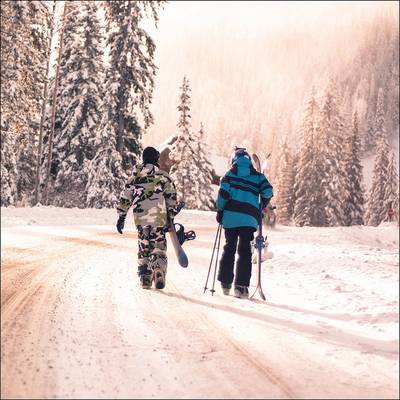
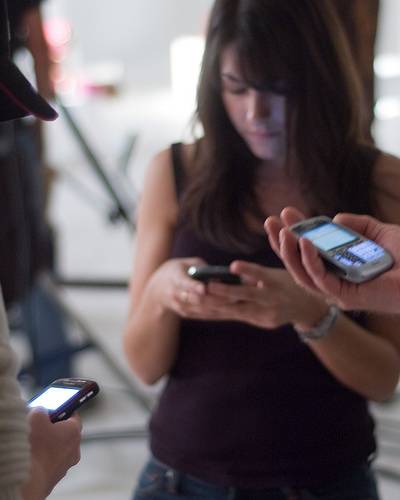
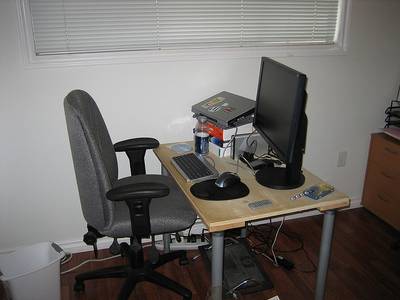
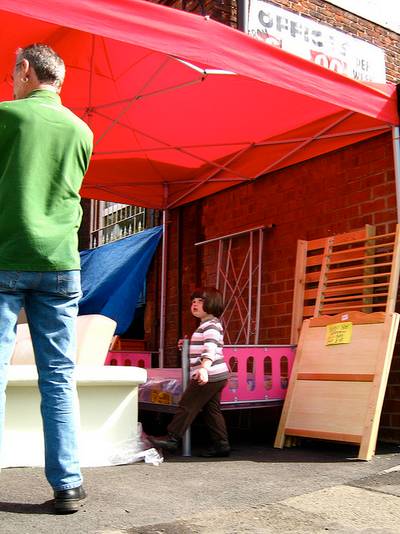
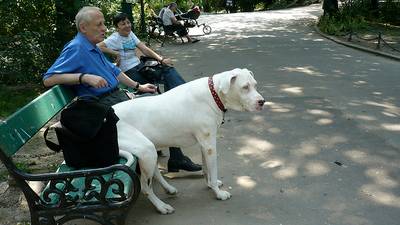
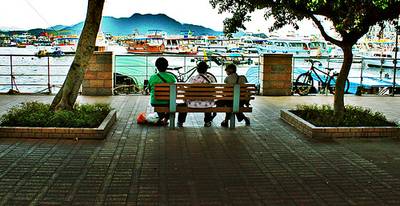
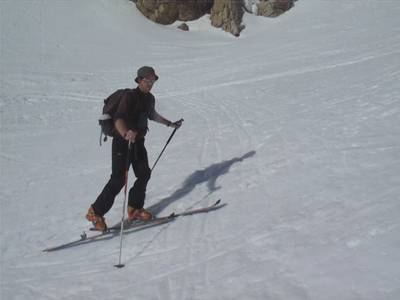
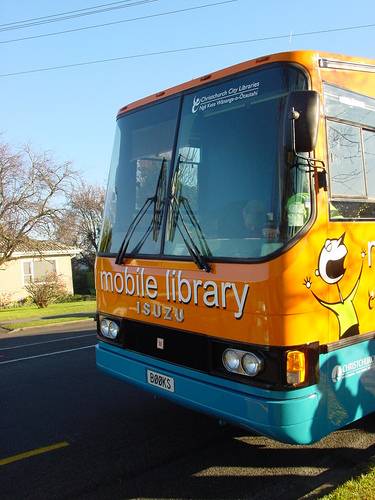
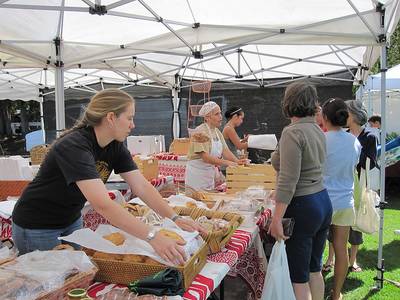
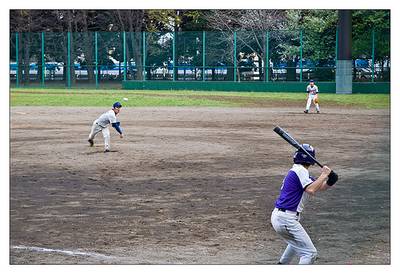
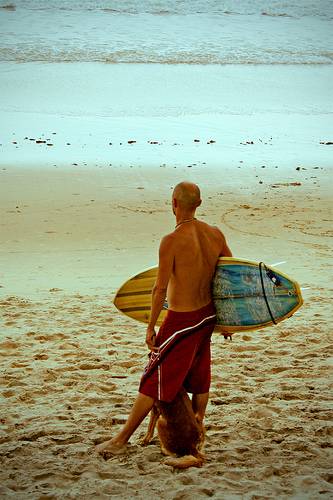

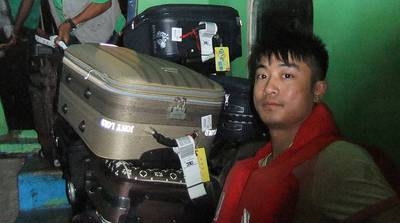
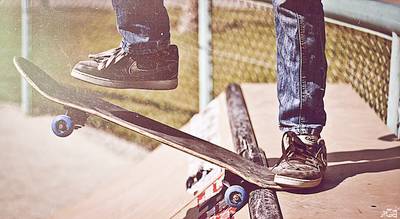
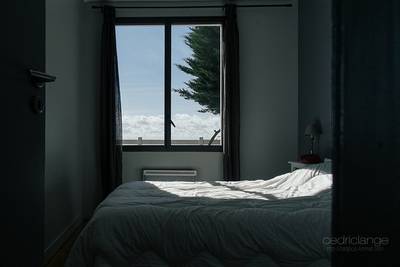

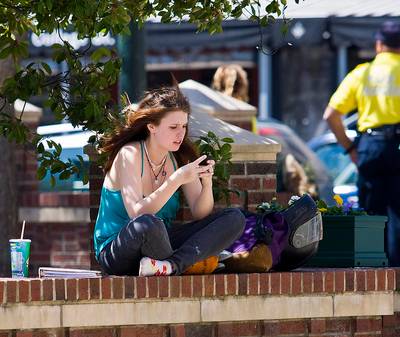
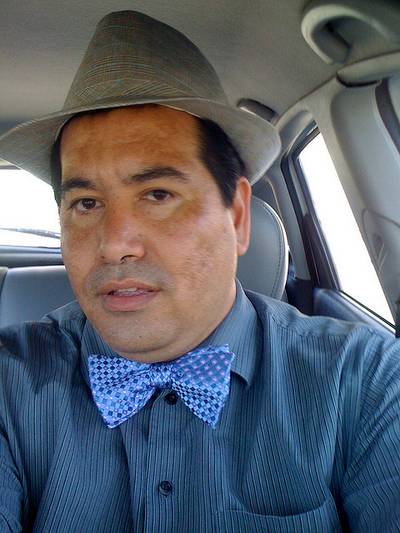

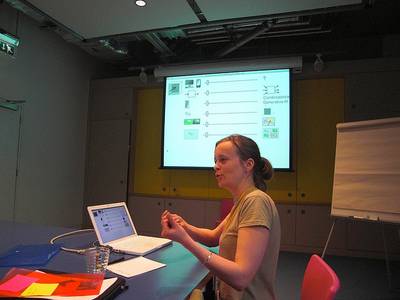

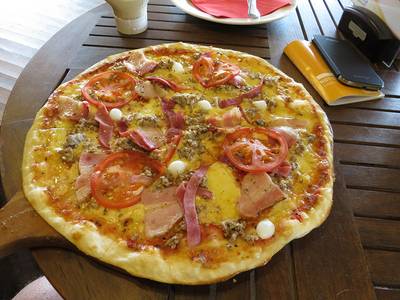
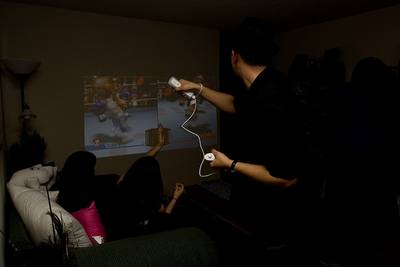

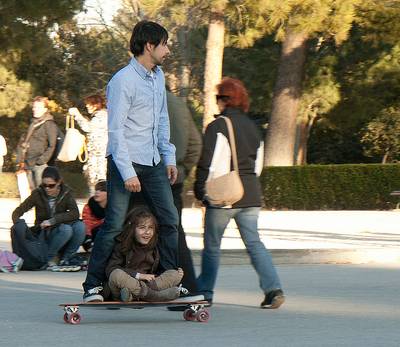
Class balance #
There are 270 annotation classes in the dataset. Find the general statistics and balances for every class in the table below. Click any row to preview images that have labels of the selected class. Sort by column to find the most rare or prevalent classes.
Class ㅤ | Images ㅤ | Objects ㅤ | Count on image average | Area on image average |
---|---|---|---|---|
body➔ any | 21187 | 109510 | 5.17 | 5.68% |
rim➔ any | 13285 | 58842 | 4.43 | 11.77% |
handle➔ any | 9194 | 32594 | 3.55 | 1.83% |
base➔ any | 7516 | 24246 | 3.23 | 1.49% |
neck➔ any | 6266 | 26426 | 4.22 | 1.52% |
side➔ any | 6206 | 35236 | 5.68 | 6.43% |
inner_body➔ any | 6044 | 23512 | 3.89 | 5.87% |
leg➔ any | 5832 | 19888 | 3.41 | 5.61% |
bottom➔ any | 5726 | 16410 | 2.87 | 11.72% |
back➔ any | 4932 | 30844 | 6.25 | 7.81% |
Images #
Explore every single image in the dataset with respect to the number of annotations of each class it has. Click a row to preview selected image. Sort by any column to find anomalies and edge cases. Use horizontal scroll if the table has many columns for a large number of classes in the dataset.
Class sizes #
The table below gives various size properties of objects for every class. Click a row to see the image with annotations of the selected class. Sort columns to find classes with the smallest or largest objects or understand the size differences between classes.
Class | Object count | Avg area | Max area | Min area | Min height | Min height | Max height | Max height | Avg height | Avg height | Min width | Min width | Max width | Max width |
---|---|---|---|---|---|---|---|---|---|---|---|---|---|---|
body any | 109510 | 1.78% | 100% | 0% | 1px | 0.16% | 640px | 100% | 59px | 12.27% | 1px | 0.16% | 640px | 100% |
book any | 82775 | 0.46% | 100% | 0% | 1px | 0.21% | 639px | 100% | 41px | 8.56% | 1px | 0.16% | 640px | 100% |
cover any | 68324 | 0.44% | 94.35% | 0% | 1px | 0.16% | 637px | 100% | 42px | 8.79% | 1px | 0.16% | 640px | 100% |
rim any | 58842 | 3.05% | 100% | 0% | 1px | 0.16% | 640px | 100% | 56px | 11.7% | 1px | 0.16% | 640px | 100% |
chair any | 36538 | 1.37% | 100% | 0% | 1px | 0.16% | 640px | 100% | 50px | 10.53% | 1px | 0.16% | 640px | 100% |
side any | 35236 | 1.68% | 99.84% | 0% | 1px | 0.21% | 640px | 100% | 54px | 11.45% | 1px | 0.16% | 640px | 100% |
handle any | 32594 | 0.69% | 76.88% | 0% | 1px | 0.16% | 640px | 100% | 41px | 8.46% | 1px | 0.16% | 640px | 100% |
car_(automobile) any | 32516 | 1.52% | 100% | 0% | 1px | 0.16% | 640px | 100% | 36px | 7.78% | 1px | 0.16% | 640px | 100% |
back any | 30844 | 1.93% | 100% | 0% | 1px | 0.21% | 640px | 100% | 52px | 11% | 1px | 0.16% | 640px | 100% |
neck any | 26426 | 0.54% | 65.44% | 0% | 1px | 0.16% | 473px | 98.54% | 27px | 5.57% | 1px | 0.16% | 477px | 81.67% |
Spatial Heatmap #
The heatmaps below give the spatial distributions of all objects for every class. These visualizations provide insights into the most probable and rare object locations on the image. It helps analyze objects' placements in a dataset.

Objects #
Table contains all 97027 objects. Click a row to preview an image with annotations, and use search or pagination to navigate. Sort columns to find outliers in the dataset.
Object ID ㅤ | Class ㅤ | Image name click row to open | Image size height x width | Height ㅤ | Height ㅤ | Width ㅤ | Width ㅤ | Area ㅤ |
---|---|---|---|---|---|---|---|---|
1➔ | scissors any | 000000146878.jpg | 567 x 640 | 370px | 65.26% | 437px | 68.28% | 7.67% |
2➔ | scissors any | 000000146878.jpg | 567 x 640 | 370px | 65.26% | 437px | 68.28% | 44.56% |
3➔ | finger_hole any | 000000146878.jpg | 567 x 640 | 214px | 37.74% | 350px | 54.69% | 2.42% |
4➔ | finger_hole any | 000000146878.jpg | 567 x 640 | 215px | 37.92% | 351px | 54.84% | 20.8% |
5➔ | handle any | 000000146878.jpg | 567 x 640 | 121px | 21.34% | 152px | 23.75% | 1.07% |
6➔ | handle any | 000000146878.jpg | 567 x 640 | 122px | 21.52% | 153px | 23.91% | 5.14% |
7➔ | screw any | 000000146878.jpg | 567 x 640 | 19px | 3.35% | 22px | 3.44% | 0.09% |
8➔ | screw any | 000000146878.jpg | 567 x 640 | 20px | 3.53% | 23px | 3.59% | 0.13% |
9➔ | blade any | 000000146878.jpg | 567 x 640 | 181px | 31.92% | 200px | 31.25% | 2.66% |
10➔ | blade any | 000000146878.jpg | 567 x 640 | 182px | 32.1% | 201px | 31.41% | 10.08% |
License #
Citation #
If you make use of the PACO-LVIS data, please cite the following reference:
@misc{ramanathan2023paco,
title={PACO: Parts and Attributes of Common Objects},
author={Vignesh Ramanathan and Anmol Kalia and Vladan Petrovic and Yi Wen and Baixue Zheng and Baishan Guo and Rui Wang and Aaron Marquez and Rama Kovvuri and Abhishek Kadian and Amir Mousavi and Yiwen Song and Abhimanyu Dubey and Dhruv Mahajan},
year={2023},
eprint={2301.01795},
archivePrefix={arXiv},
primaryClass={cs.CV}
}
If you are happy with Dataset Ninja and use provided visualizations and tools in your work, please cite us:
@misc{ visualization-tools-for-paco-lvis-dataset,
title = { Visualization Tools for PACO-LVIS Dataset },
type = { Computer Vision Tools },
author = { Dataset Ninja },
howpublished = { \url{ https://datasetninja.com/paco-lvis } },
url = { https://datasetninja.com/paco-lvis },
journal = { Dataset Ninja },
publisher = { Dataset Ninja },
year = { 2024 },
month = { nov },
note = { visited on 2024-11-21 },
}
Download #
Dataset PACO-LVIS can be downloaded in Supervisely format:
As an alternative, it can be downloaded with dataset-tools package:
pip install --upgrade dataset-tools
… using following python code:
import dataset_tools as dtools
dtools.download(dataset='PACO-LVIS', dst_dir='~/dataset-ninja/')
Make sure not to overlook the python code example available on the Supervisely Developer Portal. It will give you a clear idea of how to effortlessly work with the downloaded dataset.
Disclaimer #
Our gal from the legal dep told us we need to post this:
Dataset Ninja provides visualizations and statistics for some datasets that can be found online and can be downloaded by general audience. Dataset Ninja is not a dataset hosting platform and can only be used for informational purposes. The platform does not claim any rights for the original content, including images, videos, annotations and descriptions. Joint publishing is prohibited.
You take full responsibility when you use datasets presented at Dataset Ninja, as well as other information, including visualizations and statistics we provide. You are in charge of compliance with any dataset license and all other permissions. You are required to navigate datasets homepage and make sure that you can use it. In case of any questions, get in touch with us at hello@datasetninja.com.