Introduction #
The authors assembled the Palm Trees Dataset by capturing aerial images within a palm tree farm located in the Kharj region of Riyadh, Saudi Arabia, utilizing DJI drones. This effort resulted in the compilation of approximately 10,000 instances of palm trees. Moreover, leveraging the geotagged metadata associated with the aerial images, they applied photogrammetry principles and distance corrections to automate the detection of the geographical locations of the identified palm trees. This geolocation method underwent testing using two different drone models, namely the DJI Phantom 4 Pro drone and DJI Mavic Pro drone. The evaluation revealed an average geolocation accuracy of 2.8 meters.
Motivation
Counting trees from aerial images poses a significant challenge, with diverse applications spanning forest inventory, crop estimation, and farm management. However, quantifying the number of palm trees in extensive farms presents a particularly formidable task for agricultural authorities, given the sheer volume of trees and the limitations of manual counting methods. The complexity intensifies when the task extends to identifying the GPS locations of palm trees for regulatory purposes. The inadequacy of conventional approaches results in inconsistent data collection regarding palm tree numbers, as highlighted by agricultural experts. To address this issue, the authors introduce a deep learning framework designed to create an inventory of individual palm trees. This framework automates the process of counting and geolocating palm trees using aerial color images captured by unmanned aerial vehicles (UAVs).
Dataset description
Detecting palm infestation is crucial for accurate crop estimation. However, reliable tree counting methods are equally essential due to the inaccuracies and inefficiencies inherent in traditional counting techniques. To address this challenge, the authors propose an automated tree counting approach from aerial images, which also holds promise for applications such as forest inventory and farm management. To develop this technique, they gathered a dataset comprising 217 UAV images captured in a palm tree farm located in the Kharj region of Saudi Arabia. This dataset encompassed a total of 9,873 instances, including 8,652 palm trees and 1,221 other trees. The authors meticulously annotated this dataset using Labelme. Subsequently, they trained a Faster RCNN model—a state-of-the-art two-stage object detection algorithm—on 80% of the dataset (174 images). On the testing dataset comprising 43 images, the model achieved a precision of 94% and a recall of 84% for detecting palm trees. Additionally, the average precision (AP) at an IoU (Intersection over Union) threshold of 0.5 reached 83%.
Sample image of the palm counting and geolocation application.
Moreover, the authors have devised an algorithm designed to assign each identified tree with its precise GPS location. This algorithm leverages photogrammetry principles applied to the metadata extracted from drone imagery, including factors such as the drone’s altitude and GPS coordinates, image dimensions, calibrated focal length, and yaw angle. Subsequently, a distance correction is implemented based on the ratio between the drone’s altitude and the estimated average height of palm trees. This geolocation method underwent rigorous testing using two different drone models, namely the DJI Mavic Pro and Phantom 4 Pro. The assessment revealed that it yielded an average geolocation accuracy of 2.8 meters, with a maximum deviation of 4.9 meters and a standard deviation of 1.2 meters. The GPS tagging allows to uniquely identify, track and count the number of palm trees from a series of drone images, while correctly dealing with the issue of image overlapping while the drone is flying. This procedure can be generalized to the gelocation of any other objects in UAV images.
Summary #
Palm Trees Dataset is a dataset for object detection and counting tasks. It is used in the forestry industry.
The dataset consists of 349 images with 13067 labeled objects belonging to 1 single class (palm tree).
Images in the Palm Trees dataset have bounding box annotations. All images are labeled (i.e. with annotations). There are 2 splits in the dataset: train (279 images) and test (70 images). Alternatively, the dataset could be split into 2 drone types: DJI Phantom 4 Pro drone (245 images) and DJI Mavic Pro drone (104 images). The dataset was released in 2020 by the Prince Sultan University, Saudi Arabia.
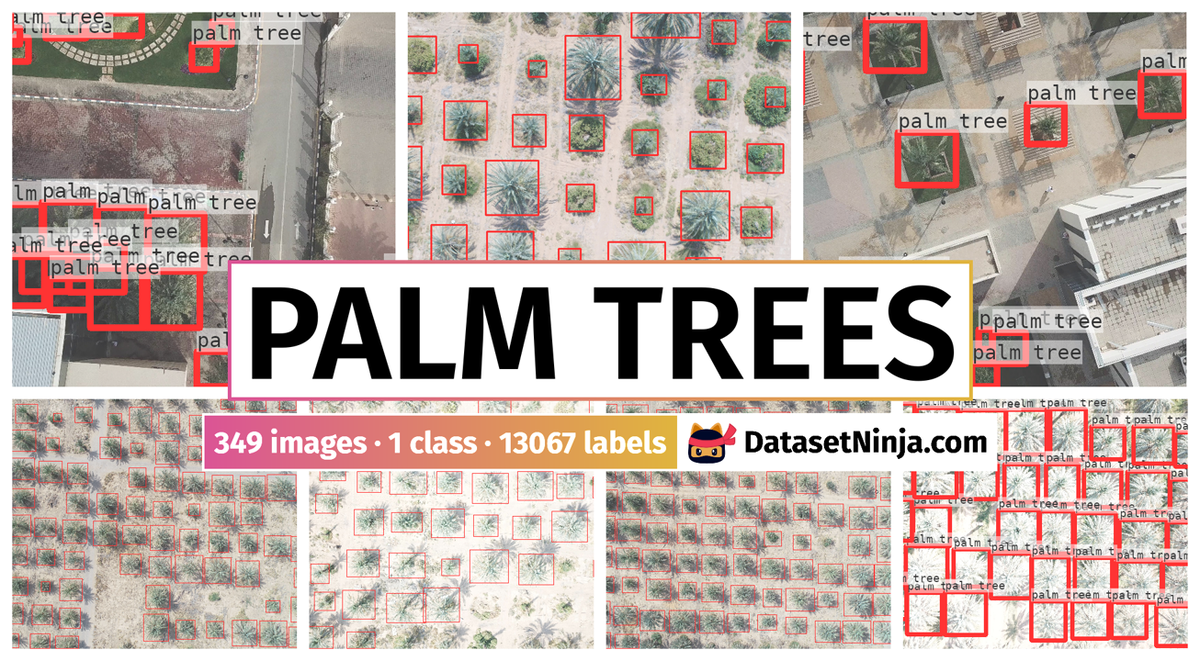
Explore #
Palm Trees dataset has 349 images. Click on one of the examples below or open "Explore" tool anytime you need to view dataset images with annotations. This tool has extended visualization capabilities like zoom, translation, objects table, custom filters and more. Hover the mouse over the images to hide or show annotations.
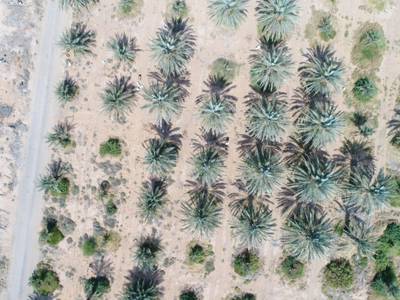
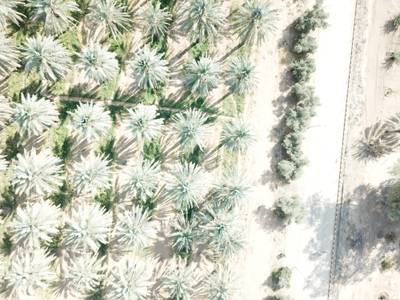
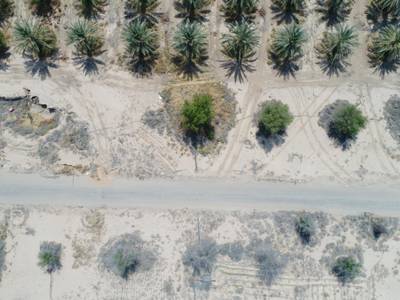
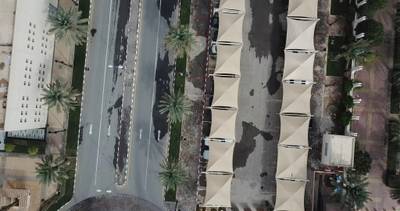
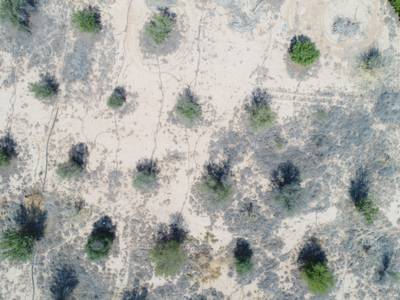

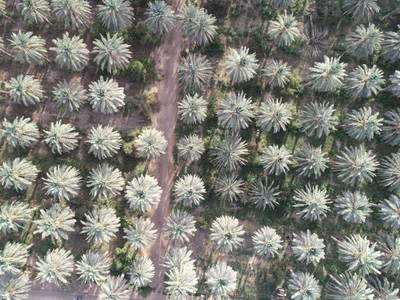


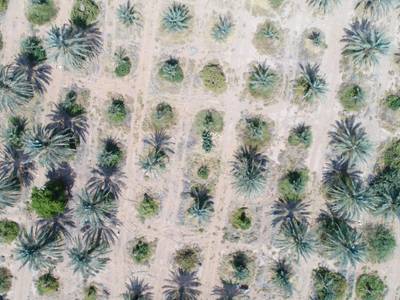
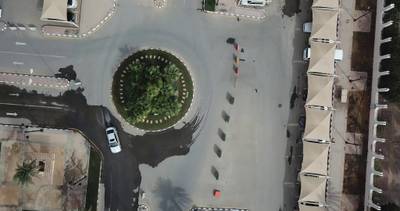

Class balance #
There are 1 annotation classes in the dataset. Find the general statistics and balances for every class in the table below. Click any row to preview images that have labels of the selected class. Sort by column to find the most rare or prevalent classes.
Class ã…¤ | Images ã…¤ | Objects ã…¤ | Count on image average | Area on image average |
---|---|---|---|---|
palm treeâž” rectangle | 349 | 13067 | 37.44 | 37.18% |
Images #
Explore every single image in the dataset with respect to the number of annotations of each class it has. Click a row to preview selected image. Sort by any column to find anomalies and edge cases. Use horizontal scroll if the table has many columns for a large number of classes in the dataset.
Class sizes #
The table below gives various size properties of objects for every class. Click a row to see the image with annotations of the selected class. Sort columns to find classes with the smallest or largest objects or understand the size differences between classes.
Class | Object count | Avg area | Max area | Min area | Min height | Min height | Max height | Max height | Avg height | Avg height | Min width | Min width | Max width | Max width |
---|---|---|---|---|---|---|---|---|---|---|---|---|---|---|
palm tree rectangle | 13067 | 1.01% | 17.38% | 0.01% | 33px | 0.9% | 1535px | 42.08% | 358px | 10.93% | 32px | 0.66% | 2009px | 41.88% |
Spatial Heatmap #
The heatmaps below give the spatial distributions of all objects for every class. These visualizations provide insights into the most probable and rare object locations on the image. It helps analyze objects' placements in a dataset.
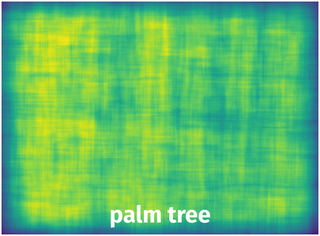
Objects #
Table contains all 13067 objects. Click a row to preview an image with annotations, and use search or pagination to navigate. Sort columns to find outliers in the dataset.
Object ID ã…¤ | Class ã…¤ | Image name click row to open | Image size height x width | Height ã…¤ | Height ã…¤ | Width ã…¤ | Width ã…¤ | Area ã…¤ |
---|---|---|---|---|---|---|---|---|
1âž” | palm tree rectangle | ck2m6m71bz4pl07218bvk4mwb.jpg | 3648 x 4864 | 447px | 12.25% | 428px | 8.8% | 1.08% |
2âž” | palm tree rectangle | ck2m6m71bz4pl07218bvk4mwb.jpg | 3648 x 4864 | 450px | 12.34% | 414px | 8.51% | 1.05% |
3âž” | palm tree rectangle | ck2m6m71bz4pl07218bvk4mwb.jpg | 3648 x 4864 | 479px | 13.13% | 470px | 9.66% | 1.27% |
4âž” | palm tree rectangle | ck2m6m71bz4pl07218bvk4mwb.jpg | 3648 x 4864 | 470px | 12.88% | 476px | 9.79% | 1.26% |
5âž” | palm tree rectangle | ck2m6m71bz4pl07218bvk4mwb.jpg | 3648 x 4864 | 465px | 12.75% | 524px | 10.77% | 1.37% |
6âž” | palm tree rectangle | ck2m6m71bz4pl07218bvk4mwb.jpg | 3648 x 4864 | 581px | 15.93% | 573px | 11.78% | 1.88% |
7âž” | palm tree rectangle | ck2m6m71bz4pl07218bvk4mwb.jpg | 3648 x 4864 | 487px | 13.35% | 487px | 10.01% | 1.34% |
8âž” | palm tree rectangle | ck2m6m71bz4pl07218bvk4mwb.jpg | 3648 x 4864 | 457px | 12.53% | 484px | 9.95% | 1.25% |
9âž” | palm tree rectangle | ck2m6m71bz4pl07218bvk4mwb.jpg | 3648 x 4864 | 484px | 13.27% | 451px | 9.27% | 1.23% |
10âž” | palm tree rectangle | ck2m6m71bz4pl07218bvk4mwb.jpg | 3648 x 4864 | 465px | 12.75% | 442px | 9.09% | 1.16% |
License #
License is unknown for the Palm Trees Dataset dataset.
Citation #
If you make use of the Palm Trees data, please cite the following reference:
@dataset{Palm Trees,
author={Adel Ammar and Anis Koubaa},
title={Palm Trees Dataset},
year={2020},
url={https://www.kaggle.com/datasets/riotulab/aerial-images-of-palm-trees}
}
If you are happy with Dataset Ninja and use provided visualizations and tools in your work, please cite us:
@misc{ visualization-tools-for-palm-trees-dataset,
title = { Visualization Tools for Palm Trees Dataset },
type = { Computer Vision Tools },
author = { Dataset Ninja },
howpublished = { \url{ https://datasetninja.com/palm-trees } },
url = { https://datasetninja.com/palm-trees },
journal = { Dataset Ninja },
publisher = { Dataset Ninja },
year = { 2024 },
month = { oct },
note = { visited on 2024-10-22 },
}
Download #
Please visit dataset homepage to download the data.
Disclaimer #
Our gal from the legal dep told us we need to post this:
Dataset Ninja provides visualizations and statistics for some datasets that can be found online and can be downloaded by general audience. Dataset Ninja is not a dataset hosting platform and can only be used for informational purposes. The platform does not claim any rights for the original content, including images, videos, annotations and descriptions. Joint publishing is prohibited.
You take full responsibility when you use datasets presented at Dataset Ninja, as well as other information, including visualizations and statistics we provide. You are in charge of compliance with any dataset license and all other permissions. You are required to navigate datasets homepage and make sure that you can use it. In case of any questions, get in touch with us at hello@datasetninja.com.