Introduction #
The authors of the RGB part of a RELLIS-3D: A Multi-modal Dataset for Off-Road Robotics address the critical need for semantic scene understanding in off-road environments to ensure robust and safe autonomous navigation. Recognizing the scarcity of multimodal off-road data in existing autonomy datasets, they present RELLIS-3D—a multimodal dataset collected in an off-road setting, specifically on the Rellis Campus of Texas A&M University. This dataset includes annotations for 13,556 LiDAR scans and 6,235 images, introducing challenges related to class imbalance and environmental topography for existing algorithms. Furthermore, the authors conduct evaluations of state-of-the-art deep learning semantic segmentation models on RELLIS-3D, revealing the unique challenges it poses compared to datasets focused on urban environments. The dataset aims to provide researchers with the resources necessary to advance algorithms and explore new research directions for enhancing autonomous navigation in off-road scenarios.
Warthog Platform Configuration. Illustration of the dimensions and mounting positions of the sensors with respect to the robot body. (Units: cm)
The sensor setup and calibration involve various instruments:
- 1 × Ouster OS1 LiDAR: 64 Channels, 2048 horizontal resolution, 10 Hz, 45◦ vertical field of view.
- 1 × Velodyne Ultra Puck: 32 Channels, 10hz, 40◦ vertical field of view.
- 1 × Nerian Karmin2 + Nerian SceneScan: 3D Stereo Camera, 10 hz.
- 1 × RGB Camera: Basler acA1920-50gc camera with 16mm/F18 EDMUND Optics lens, image resolution 1920x1200, 10 hz.
- Inertial Navigation System (GPS/IMU): Vectornav VN-300 Dual Antenna GNSS/INS, 300 Hz GPS, 100 Hz IMU.
The platform, Warthog, features two computers—one for robotic control and another for data collection and sensor processing. The synchronization of sensors and computers is achieved using Precision Time Protocol (PTP). Camera calibration is performed using the ROS Camera Calibrator library for intrinsic camera calibration, and the extrinsic calibration between sensors is determined using established methods.
Ground truth annotations examples provided in the RELLIS-3D dataset. Images are densely annotated with pixel-wise labels from 20 different visual classes. LiDAR scans are point-wise labeled with the same ontology.
RELLIS-3D consists of five traversal sequences recorded on non-paved trails of the Ground Research facility, each presenting distinct environmental challenges. The dataset ontology includes object and terrain classes, derived from the RUGD dataset, but expanded to encompass unique classes relevant to off-road scenarios. Annotations were provided by Appen through crowdsourcing, with trained annotators ensuring consistency. Both pixel-wise image annotations and point-wise annotations for 3D point clouds are included in the dataset. The authors highlight the statistics of the dataset, emphasizing the imbalanced class distribution, which is common in datasets used for semantic segmentation, but is more severe in off-road environments compared to urban settings.
The authors present detailed statistics on class distribution for both image and point cloud annotations, shedding light on the challenges posed by the unique characteristics of off-road environments.
Left - Image Label distribution. The sky, grass, tree and bush constitute the major classes. Right - Point Cloud Label distribution. The grass, tree, and bush also dominate the population.
Summary #
RELLIS-3D: A Multi-modal Dataset for Off-Road Robotics (RGB) is a dataset for instance segmentation, semantic segmentation, and object detection tasks. It is used in the robotics industry.
The dataset consists of 5957 images with 200052 labeled objects belonging to 19 different classes including grass, sky, bush, and other: tree, mud, concrete, barrier, pole, vehicle, puddle, building, object, rubble, person, fence, asphalt, log, water, and dirt.
Images in the RELLIS-3D: RGB dataset have pixel-level instance segmentation annotations. Due to the nature of the instance segmentation task, it can be automatically transformed into a semantic segmentation (only one mask for every class) or object detection (bounding boxes for every object) tasks. All images are labeled (i.e. with annotations). There are 3 splits in the dataset: train (3302 images), test (1672 images), and val (983 images). The dataset was released in 2020 by the Texas A&M University and CCDC Army Research Laboratory.
Here are the visualized examples for the classes:
Explore #
RELLIS-3D: RGB dataset has 5957 images. Click on one of the examples below or open "Explore" tool anytime you need to view dataset images with annotations. This tool has extended visualization capabilities like zoom, translation, objects table, custom filters and more. Hover the mouse over the images to hide or show annotations.




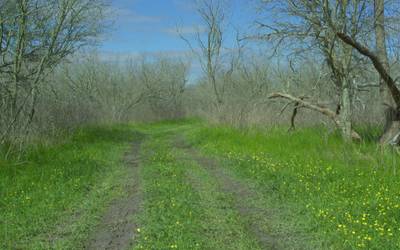
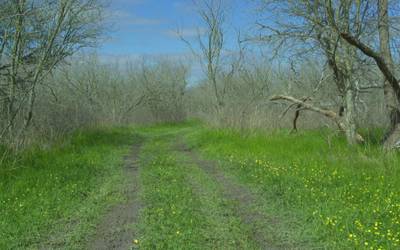



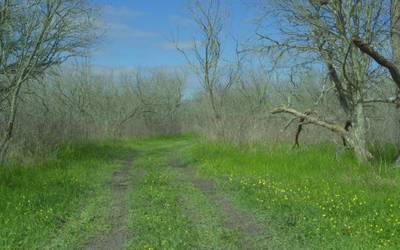






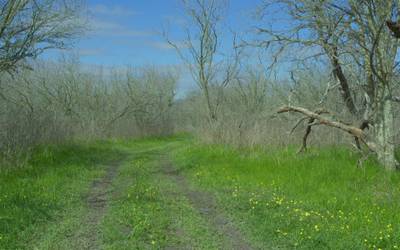




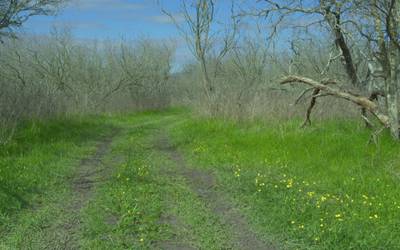








Class balance #
There are 19 annotation classes in the dataset. Find the general statistics and balances for every class in the table below. Click any row to preview images that have labels of the selected class. Sort by column to find the most rare or prevalent classes.
Class ㅤ | Images ㅤ | Objects ㅤ | Count on image average | Area on image average |
---|---|---|---|---|
grass➔ mask | 5955 | 29603 | 4.97 | 34.23% |
sky➔ mask | 5889 | 57595 | 9.78 | 29.43% |
bush➔ mask | 5859 | 20063 | 3.42 | 17.16% |
tree➔ mask | 5733 | 21115 | 3.68 | 14.82% |
mud➔ mask | 3859 | 18847 | 4.88 | 3.53% |
concrete➔ mask | 2605 | 11812 | 4.53 | 3.56% |
barrier➔ mask | 2211 | 9590 | 4.34 | 1% |
pole➔ mask | 1965 | 4521 | 2.3 | 0.06% |
vehicle➔ mask | 1718 | 5241 | 3.05 | 0.13% |
puddle➔ mask | 1630 | 6033 | 3.7 | 2.67% |
Co-occurrence matrix #
Co-occurrence matrix is an extremely valuable tool that shows you the images for every pair of classes: how many images have objects of both classes at the same time. If you click any cell, you will see those images. We added the tooltip with an explanation for every cell for your convenience, just hover the mouse over a cell to preview the description.
Images #
Explore every single image in the dataset with respect to the number of annotations of each class it has. Click a row to preview selected image. Sort by any column to find anomalies and edge cases. Use horizontal scroll if the table has many columns for a large number of classes in the dataset.
Class sizes #
The table below gives various size properties of objects for every class. Click a row to see the image with annotations of the selected class. Sort columns to find classes with the smallest or largest objects or understand the size differences between classes.
Class | Object count | Avg area | Max area | Min area | Min height | Min height | Max height | Max height | Avg height | Avg height | Min width | Min width | Max width | Max width |
---|---|---|---|---|---|---|---|---|---|---|---|---|---|---|
sky mask | 57595 | 3.01% | 78.32% | 0% | 2px | 0.17% | 1083px | 90.25% | 85px | 7.07% | 1px | 0.05% | 1920px | 100% |
grass mask | 29603 | 6.89% | 84.2% | 0% | 1px | 0.08% | 1200px | 100% | 164px | 13.63% | 1px | 0.05% | 1920px | 100% |
tree mask | 21115 | 4.02% | 61.81% | 0% | 1px | 0.08% | 1180px | 98.33% | 170px | 14.13% | 2px | 0.1% | 1920px | 100% |
bush mask | 20063 | 5.01% | 94.07% | 0% | 1px | 0.08% | 1200px | 100% | 218px | 18.2% | 2px | 0.1% | 1920px | 100% |
mud mask | 18847 | 0.72% | 23.28% | 0% | 3px | 0.25% | 745px | 62.08% | 126px | 10.47% | 2px | 0.1% | 1920px | 100% |
concrete mask | 11812 | 0.78% | 23.27% | 0% | 1px | 0.08% | 504px | 42% | 57px | 4.73% | 2px | 0.1% | 1920px | 100% |
barrier mask | 9590 | 0.23% | 23% | 0% | 3px | 0.25% | 615px | 51.25% | 38px | 3.15% | 3px | 0.16% | 1206px | 62.81% |
puddle mask | 6033 | 0.72% | 36.42% | 0% | 5px | 0.42% | 621px | 51.75% | 77px | 6.43% | 7px | 0.36% | 1920px | 100% |
vehicle mask | 5241 | 0.04% | 0.36% | 0% | 3px | 0.25% | 110px | 9.17% | 26px | 2.17% | 3px | 0.16% | 311px | 16.2% |
fence mask | 4583 | 0.05% | 2.08% | 0% | 4px | 0.33% | 559px | 46.58% | 73px | 6.11% | 2px | 0.1% | 797px | 41.51% |
Spatial Heatmap #
The heatmaps below give the spatial distributions of all objects for every class. These visualizations provide insights into the most probable and rare object locations on the image. It helps analyze objects' placements in a dataset.
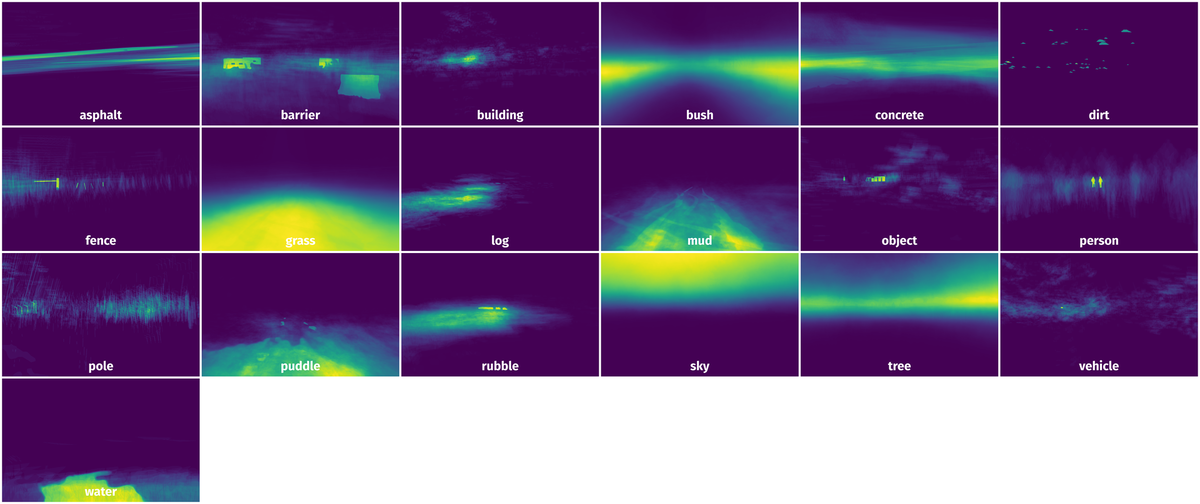
Objects #
Table contains all 101223 objects. Click a row to preview an image with annotations, and use search or pagination to navigate. Sort columns to find outliers in the dataset.
Object ID ㅤ | Class ㅤ | Image name click row to open | Image size height x width | Height ㅤ | Height ㅤ | Width ㅤ | Width ㅤ | Area ㅤ |
---|---|---|---|---|---|---|---|---|
1➔ | grass mask | frame002306-1581624020_950.jpg | 1200 x 1920 | 654px | 54.5% | 1920px | 100% | 29.95% |
2➔ | grass mask | frame002306-1581624020_950.jpg | 1200 x 1920 | 17px | 1.42% | 40px | 2.08% | 0.03% |
3➔ | grass mask | frame002306-1581624020_950.jpg | 1200 x 1920 | 17px | 1.42% | 38px | 1.98% | 0.03% |
4➔ | tree mask | frame002306-1581624020_950.jpg | 1200 x 1920 | 97px | 8.08% | 299px | 15.57% | 0.93% |
5➔ | tree mask | frame002306-1581624020_950.jpg | 1200 x 1920 | 116px | 9.67% | 661px | 34.43% | 1.97% |
6➔ | tree mask | frame002306-1581624020_950.jpg | 1200 x 1920 | 113px | 9.42% | 943px | 49.11% | 3.26% |
7➔ | sky mask | frame002306-1581624020_950.jpg | 1200 x 1920 | 489px | 40.75% | 1920px | 100% | 36.72% |
8➔ | person mask | frame002306-1581624020_950.jpg | 1200 x 1920 | 119px | 9.92% | 48px | 2.5% | 0.17% |
9➔ | person mask | frame002306-1581624020_950.jpg | 1200 x 1920 | 108px | 9% | 44px | 2.29% | 0.11% |
10➔ | person mask | frame002306-1581624020_950.jpg | 1200 x 1920 | 105px | 8.75% | 36px | 1.88% | 0.1% |
License #
RELLIS-3D: A Multi-modal Dataset for Off-Road Robotics is under CC BY-NC-SA 3.0 US license.
Citation #
If you make use of the RELLIS-3D-RGB data, please cite the following reference:
@misc{jiang2020rellis3d,
title={RELLIS-3D Dataset: Data, Benchmarks and Analysis},
author={Peng Jiang and Philip Osteen and Maggie Wigness and Srikanth Saripalli},
year={2020},
eprint={2011.12954},
archivePrefix={arXiv},
primaryClass={cs.CV}
}
If you are happy with Dataset Ninja and use provided visualizations and tools in your work, please cite us:
@misc{ visualization-tools-for-rellis-3d-rgb-dataset,
title = { Visualization Tools for RELLIS-3D: RGB Dataset },
type = { Computer Vision Tools },
author = { Dataset Ninja },
howpublished = { \url{ https://datasetninja.com/rellis-3d-rgb } },
url = { https://datasetninja.com/rellis-3d-rgb },
journal = { Dataset Ninja },
publisher = { Dataset Ninja },
year = { 2025 },
month = { jul },
note = { visited on 2025-07-02 },
}
Download #
Dataset RELLIS-3D: RGB can be downloaded in Supervisely format:
As an alternative, it can be downloaded with dataset-tools package:
pip install --upgrade dataset-tools
… using following python code:
import dataset_tools as dtools
dtools.download(dataset='RELLIS-3D: RGB', dst_dir='~/dataset-ninja/')
Make sure not to overlook the python code example available on the Supervisely Developer Portal. It will give you a clear idea of how to effortlessly work with the downloaded dataset.
The data in original format can be downloaded here.
Disclaimer #
Our gal from the legal dep told us we need to post this:
Dataset Ninja provides visualizations and statistics for some datasets that can be found online and can be downloaded by general audience. Dataset Ninja is not a dataset hosting platform and can only be used for informational purposes. The platform does not claim any rights for the original content, including images, videos, annotations and descriptions. Joint publishing is prohibited.
You take full responsibility when you use datasets presented at Dataset Ninja, as well as other information, including visualizations and statistics we provide. You are in charge of compliance with any dataset license and all other permissions. You are required to navigate datasets homepage and make sure that you can use it. In case of any questions, get in touch with us at hello@datasetninja.com.