Introduction #
In the pursuit of accurate road detection and centerline extraction from very high-resolution (VHR) remote sensing imagery, the authors of the Road Detection and Centerline Extraction Dataset address the challenges posed by complex backgrounds and occlusions of trees and cars. Traditional road detection methods often introduce heterogeneous segments, while existing centerline extraction approaches struggle to produce a smooth, complete, and single-pixel width road network. To overcome these complexities, the authors propose a novel deep model, the Cascaded End-to-End Convolutional Neural Network (CasNet), designed to simultaneously handle road detection and centerline extraction tasks.
CasNet comprises two interconnected networks. The first network focuses on road detection, leveraging strong representation abilities to navigate complex backgrounds and occlusions. The second network is cascaded to the first, utilizing previously generated feature maps to achieve effective centerline extraction. To obtain a smooth, complete, and single-pixel width road centerline network, a thinning algorithm is introduced as a final step in the process.
In the realm of road extraction, two fundamental subtasks exist: road detection and road centerline extraction. The former involves extracting all road pixels, while the latter focuses solely on labeling the centerline pixels to provide directional information for applications such as vehicle navigation. Many road detection approaches are pixel-level, leading to heterogeneous results in VHR remote sensing images due to noise and occlusions. Object-level-based approaches offer some improvement but struggle to provide consistent results around road boundaries.
Dataset Information
There is a scarcity of publicly available very high-resolution (VHR) urban road datasets. In response, the authors collected 224 VHR images from Google Earth and manually labeled corresponding road segmentation reference maps and centerline reference maps. This dataset is touted as the largest road dataset with accurate segmentation and centerline maps.*
Road Detection Annotations:
- Original images have a spatial resolution of 1.2 m per pixel.
- Each image contains at least 600x600 pixels, and the road width ranges from 12 to 15 pixels.
- The dataset includes images with complex backgrounds and occlusions, presenting a challenging road detection task.
Road Centerline Annotations:
- Corresponds to the road centerline maps of the original images.
- The road centerline is single-pixel width, accurately positioned at the center of the road.
- Provides a visual overlay between road segmentation reference maps and road centerline reference maps for better understanding.
- Close-ups highlight the single-pixel width and central positioning of the road centerline in the dataset.
Summary #
Road Detection and Centerline Extraction Dataset is a dataset for a semantic segmentation task. It is used in the geospatial domain.
The dataset consists of 224 images with 448 labeled objects belonging to 2 different classes including centerline and road.
Images in the Road Detection and Centerline Extraction dataset have pixel-level semantic segmentation annotations. All images are labeled (i.e. with annotations). There are 3 splits in the dataset: train (160 images), test (44 images), and validation (20 images). The dataset was released in 2017 by the Chinese Academy of Sciences, China.
Here is the visualized example grid with annotations:
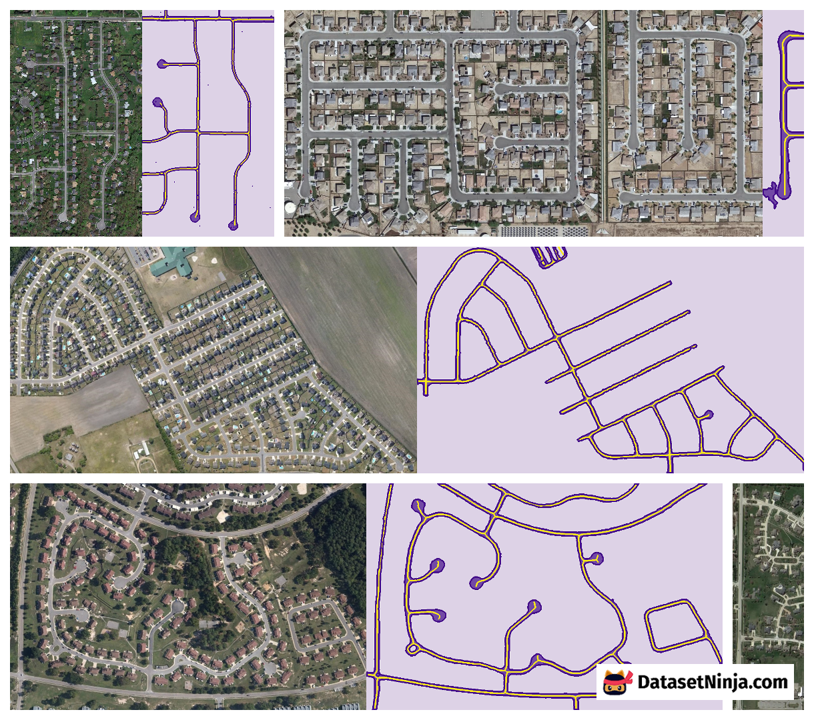
Explore #
Road Detection and Centerline Extraction dataset has 224 images. Click on one of the examples below or open "Explore" tool anytime you need to view dataset images with annotations. This tool has extended visualization capabilities like zoom, translation, objects table, custom filters and more. Hover the mouse over the images to hide or show annotations.

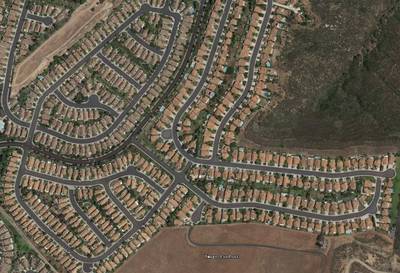

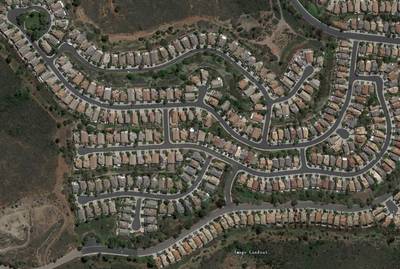
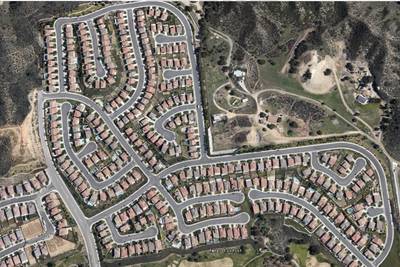
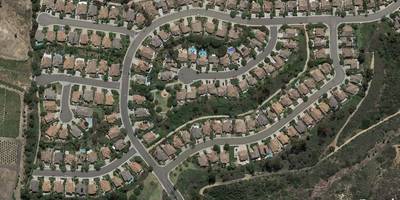

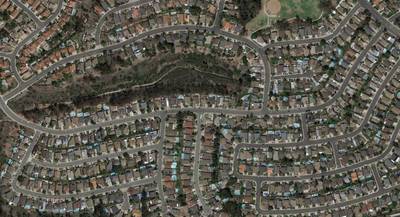
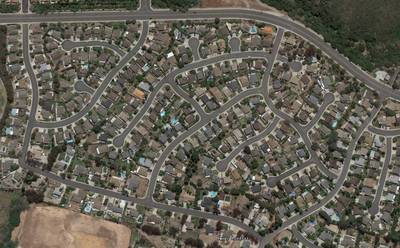


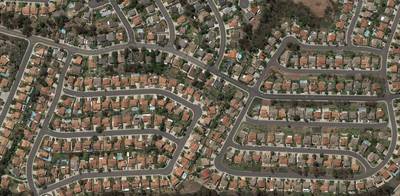
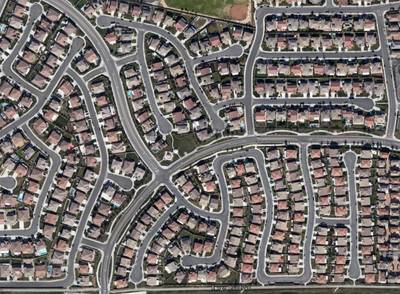

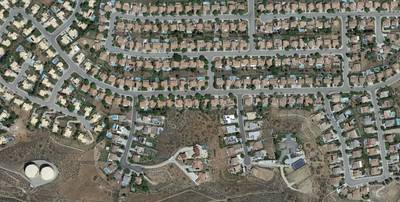

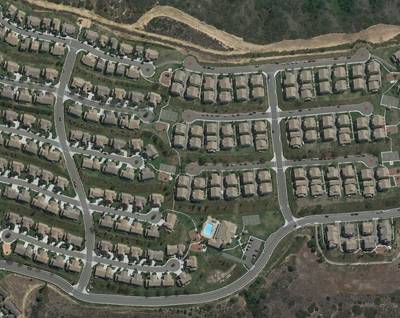
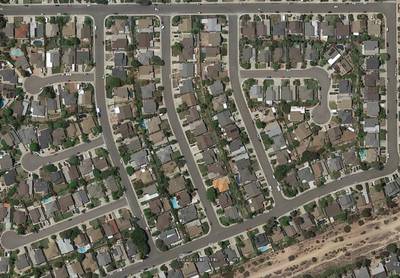
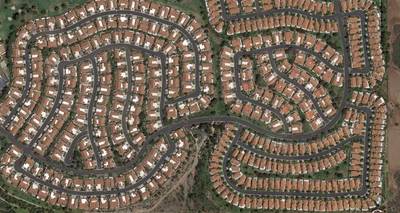
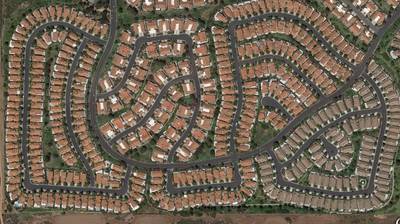
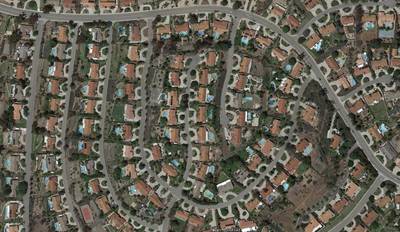
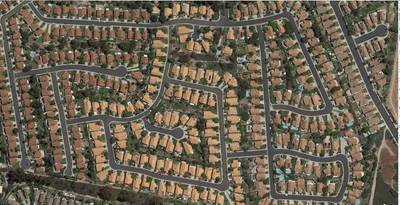
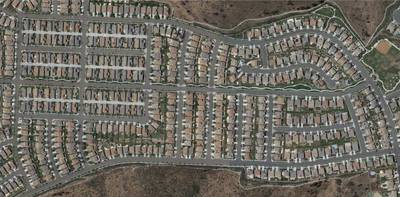
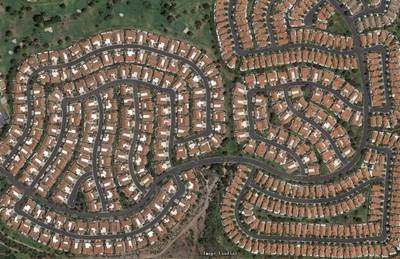

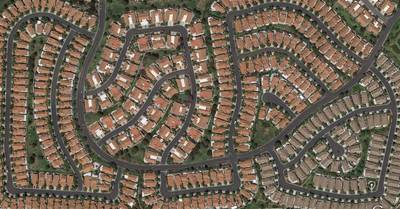



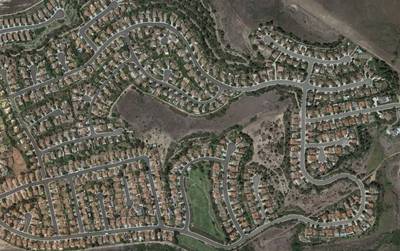
Class balance #
There are 2 annotation classes in the dataset. Find the general statistics and balances for every class in the table below. Click any row to preview images that have labels of the selected class. Sort by column to find the most rare or prevalent classes.
Class ã…¤ | Images ã…¤ | Objects ã…¤ | Count on image average | Area on image average |
---|---|---|---|---|
roadâž” mask | 224 | 224 | 1 | 11.57% |
centerlineâž” mask | 224 | 224 | 1 | 0.79% |
Co-occurrence matrix #
Co-occurrence matrix is an extremely valuable tool that shows you the images for every pair of classes: how many images have objects of both classes at the same time. If you click any cell, you will see those images. We added the tooltip with an explanation for every cell for your convenience, just hover the mouse over a cell to preview the description.
Images #
Explore every single image in the dataset with respect to the number of annotations of each class it has. Click a row to preview selected image. Sort by any column to find anomalies and edge cases. Use horizontal scroll if the table has many columns for a large number of classes in the dataset.
Object distribution #
Interactive heatmap chart for every class with object distribution shows how many images are in the dataset with a certain number of objects of a specific class. Users can click cell and see the list of all corresponding images.
Class sizes #
The table below gives various size properties of objects for every class. Click a row to see the image with annotations of the selected class. Sort columns to find classes with the smallest or largest objects or understand the size differences between classes.
Class | Object count | Avg area | Max area | Min area | Min height | Min height | Max height | Max height | Avg height | Avg height | Min width | Min width | Max width | Max width |
---|---|---|---|---|---|---|---|---|---|---|---|---|---|---|
road mask | 224 | 11.57% | 26.72% | 3.88% | 318px | 72.74% | 860px | 100% | 663px | 97.19% | 409px | 69.52% | 1426px | 100% |
centerline mask | 224 | 0.79% | 1.87% | 0.34% | 314px | 70.14% | 854px | 100% | 657px | 96.31% | 411px | 67.65% | 1425px | 100% |
Spatial Heatmap #
The heatmaps below give the spatial distributions of all objects for every class. These visualizations provide insights into the most probable and rare object locations on the image. It helps analyze objects' placements in a dataset.
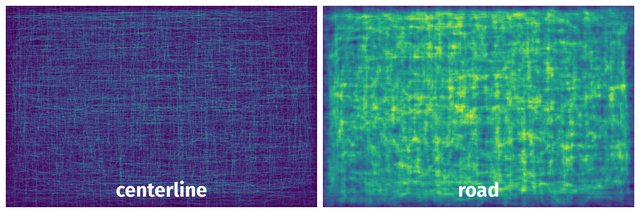
Objects #
Table contains all 448 objects. Click a row to preview an image with annotations, and use search or pagination to navigate. Sort columns to find outliers in the dataset.
Object ID ã…¤ | Class ã…¤ | Image name click row to open | Image size height x width | Height ã…¤ | Height ã…¤ | Width ã…¤ | Width ã…¤ | Area ã…¤ |
---|---|---|---|---|---|---|---|---|
1âž” | centerline mask | image205.bmp | 700 x 1369 | 682px | 97.43% | 1351px | 98.69% | 0.68% |
2âž” | road mask | image205.bmp | 700 x 1369 | 696px | 99.43% | 1364px | 99.63% | 16.38% |
3âž” | centerline mask | image222.bmp | 768 x 908 | 768px | 100% | 906px | 99.78% | 1.09% |
4âž” | road mask | image222.bmp | 768 x 908 | 766px | 99.74% | 906px | 99.78% | 21.97% |
5âž” | centerline mask | image186.bmp | 700 x 987 | 700px | 100% | 808px | 81.86% | 0.63% |
6âž” | road mask | image186.bmp | 700 x 987 | 698px | 99.71% | 829px | 83.99% | 12.25% |
7âž” | centerline mask | image216.bmp | 753 x 1184 | 727px | 96.55% | 1183px | 99.92% | 0.89% |
8âž” | road mask | image216.bmp | 753 x 1184 | 735px | 97.61% | 1182px | 99.83% | 17.96% |
9âž” | centerline mask | image193.bmp | 704 x 1350 | 666px | 94.6% | 1324px | 98.07% | 0.83% |
10âž” | road mask | image193.bmp | 704 x 1350 | 676px | 96.02% | 1336px | 98.96% | 16.5% |
License #
The Road Detection and Centerline Extraction Dataset is publicly available.
Citation #
If you make use of the Road Detection and Centerline Extraction data, please cite the following reference:
G. Cheng, Y. Wang, S. Xu, H. Wang, S. Xiang and C. Pan,
"Automatic Road Detection and Centerline Extraction via Cascaded End-to-End Convolutional Neural Network,"
in IEEE Transactions on Geoscience and Remote Sensing,
vol. 55, no. 6, pp. 3322-3337,
June 2017,
doi: 10.1109/TGRS.2017.2669341.
If you are happy with Dataset Ninja and use provided visualizations and tools in your work, please cite us:
@misc{ visualization-tools-for-road-detection-dataset,
title = { Visualization Tools for Road Detection and Centerline Extraction Dataset },
type = { Computer Vision Tools },
author = { Dataset Ninja },
howpublished = { \url{ https://datasetninja.com/road-detection } },
url = { https://datasetninja.com/road-detection },
journal = { Dataset Ninja },
publisher = { Dataset Ninja },
year = { 2025 },
month = { may },
note = { visited on 2025-05-15 },
}
Download #
Dataset Road Detection and Centerline Extraction can be downloaded in Supervisely format:
As an alternative, it can be downloaded with dataset-tools package:
pip install --upgrade dataset-tools
… using following python code:
import dataset_tools as dtools
dtools.download(dataset='Road Detection and Centerline Extraction', dst_dir='~/dataset-ninja/')
Make sure not to overlook the python code example available on the Supervisely Developer Portal. It will give you a clear idea of how to effortlessly work with the downloaded dataset.
The data in original format can be downloaded here.
Disclaimer #
Our gal from the legal dep told us we need to post this:
Dataset Ninja provides visualizations and statistics for some datasets that can be found online and can be downloaded by general audience. Dataset Ninja is not a dataset hosting platform and can only be used for informational purposes. The platform does not claim any rights for the original content, including images, videos, annotations and descriptions. Joint publishing is prohibited.
You take full responsibility when you use datasets presented at Dataset Ninja, as well as other information, including visualizations and statistics we provide. You are in charge of compliance with any dataset license and all other permissions. You are required to navigate datasets homepage and make sure that you can use it. In case of any questions, get in touch with us at hello@datasetninja.com.