Introduction #
The complete Road Potholes Images dataset comprises two distinct sets, one categorized as relatively simple and the other as more intricate. Some files are common between these datasets, and there are instances where two distinct images share the same name. Consequently, if one intends to merge these datasets into a larger one, it’s crucial to implement appropriate precautions. Each of the training datasets is further divided into positive and negative images, along with a set of positive test images.
The authors of the dataset address the issue of potholes, particularly in developing regions, which can lead to vehicle damage and risks to drivers. They propose a novel approach employing vehicle-based computer vision to detect potholes using a camera mounted on the window. Unlike previous methods that often use theoretical models or footage from specific vantage points at low speeds, the authors emphasize real-time detection from within a moving vehicle.
A notable feature of their work is the creation of an extensive image library featuring actual and representative potholes under diverse conditions. This approach allows for more accurate and practical results. The authors aim to provide drivers with real-time warnings to avoid potholes, as well as log and share their locations for the benefit of other drivers and road maintenance agencies.
Potholes are primarily caused by water-related processes and can lead to road degradation over time. The authors illustrate this phenomenon and emphasize the role of regular road maintenance in preventing their formation. Neglected cracks in the road surface can lead to significant damage if not addressed promptly.
Besides affecting roads, potholes pose risks to drivers and their vehicles. The authors highlight the absence of a viable solution for drivers to avoid potholes and identify a potential market for such a system.
Their proposed solution involves the development of a device attached to vehicles that continuously scans the road surface for potholes. When a pothole is detected, the device alerts the driver and records the pothole’s GPS coordinates. These coordinates can be uploaded to a network system that incorporates mapping software, accessible by the public, municipalities, and road maintenance agencies. This increased awareness of pothole locations can lead to safer driving habits and reduced road usage in affected areas.
Summary #
Road Pothole Images for Pothole Detection is a dataset for an object detection task. It is used in the automotive industry, and in the engineering research.
The dataset consists of 11497 images with 7588 labeled objects belonging to 1 single class (pothole).
Images in the Road Pothole Images dataset have bounding box annotations. There are 8552 (74% of the total) unlabeled images (i.e. without annotations). There are 4 splits in the dataset: ds2_complex-train (7490 images), ds1_simplex-train (3126 images), ds2_complex-test (604 images), and ds1_simplex-test (277 images). Alternatively, the dataset could be split into 2 subsets reflecting bbox availability: negative data (8608 images) and positive data (2008 images). Additionaly, the pothole numbers data is available. The dataset was released in 2018 by the Stellenbosch University, South Africa.
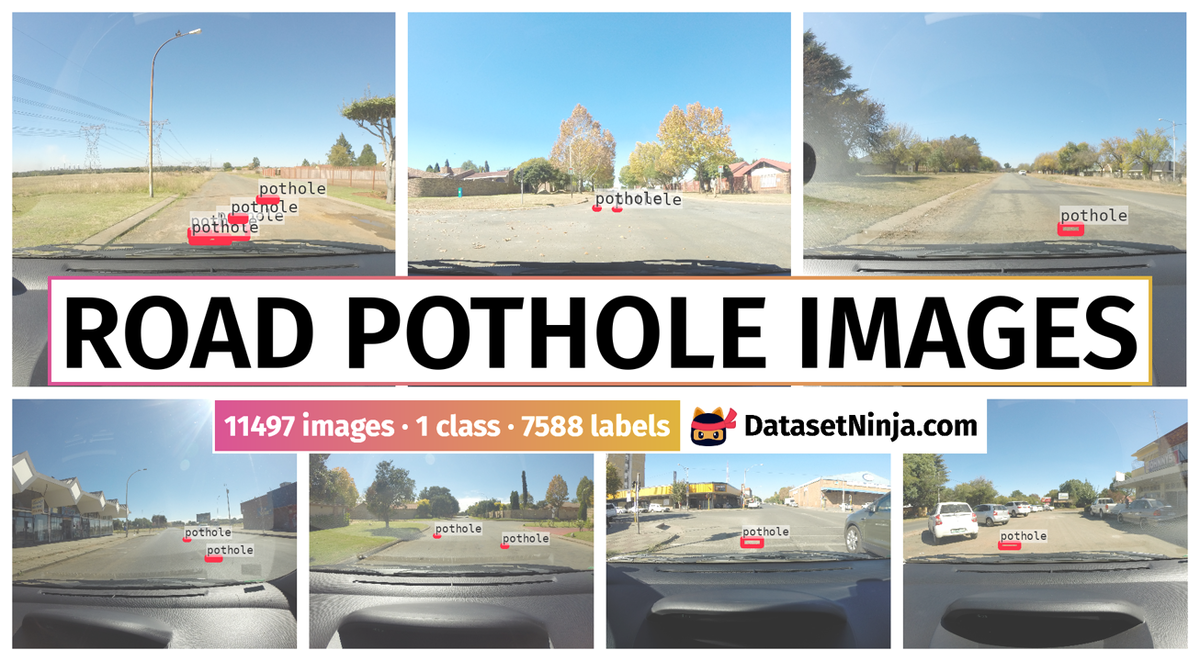
Explore #
Road Pothole Images dataset has 11497 images. Click on one of the examples below or open "Explore" tool anytime you need to view dataset images with annotations. This tool has extended visualization capabilities like zoom, translation, objects table, custom filters and more. Hover the mouse over the images to hide or show annotations.
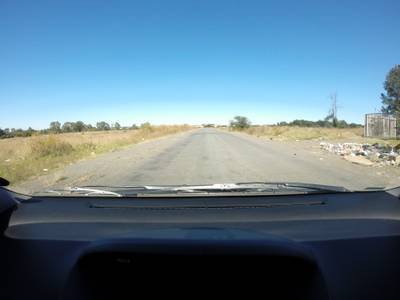
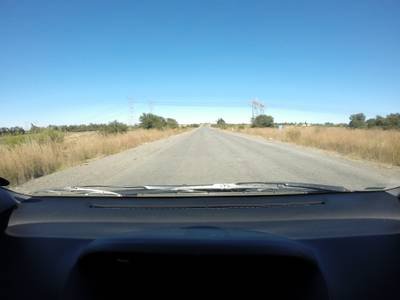
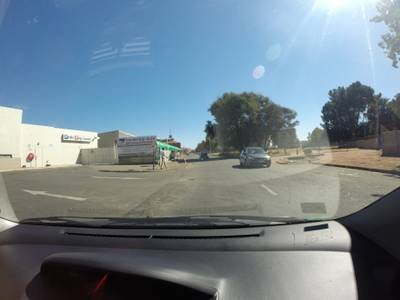
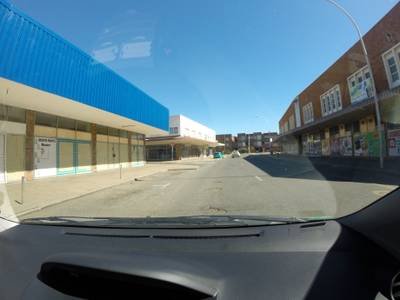
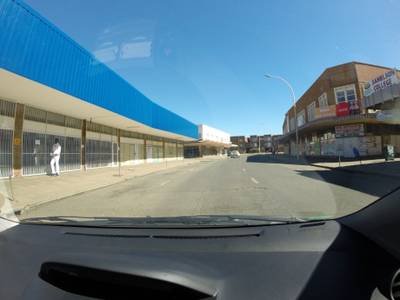
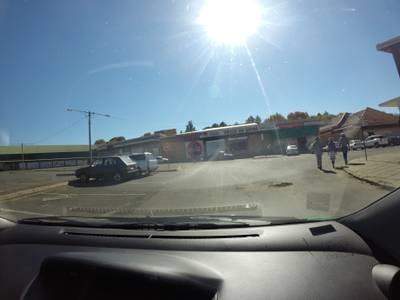
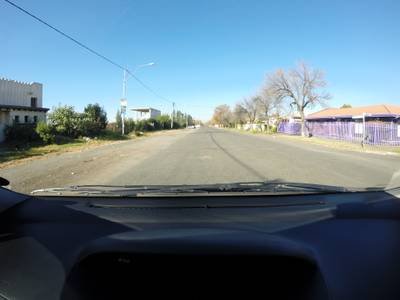
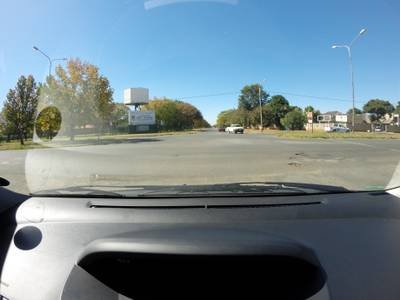
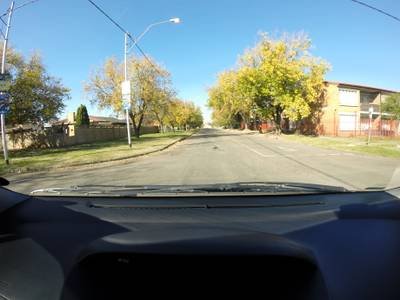
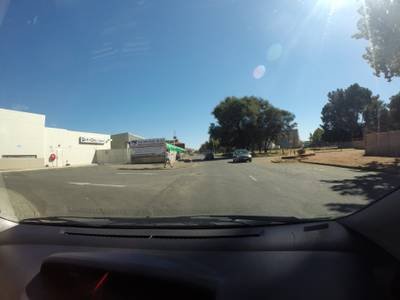
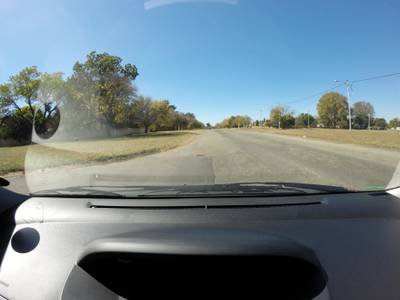
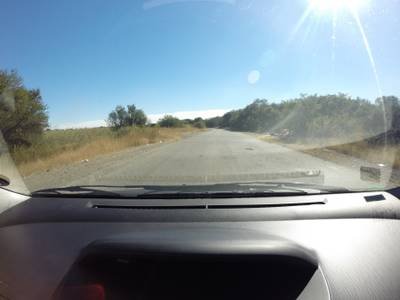
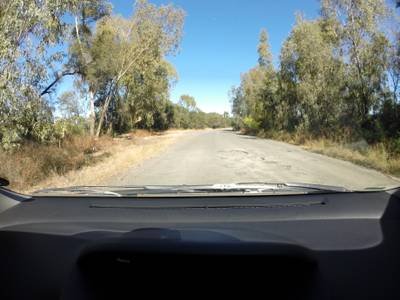
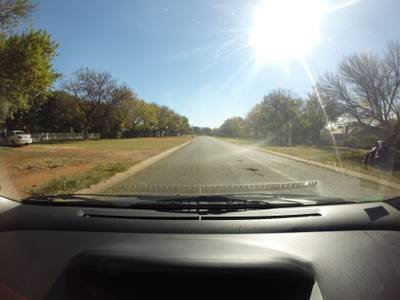
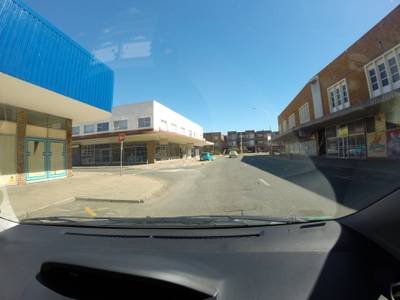
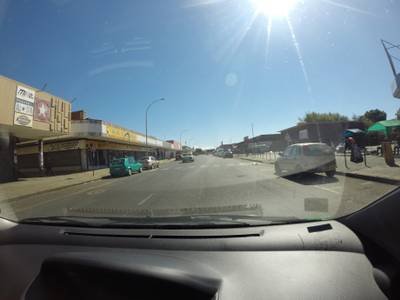
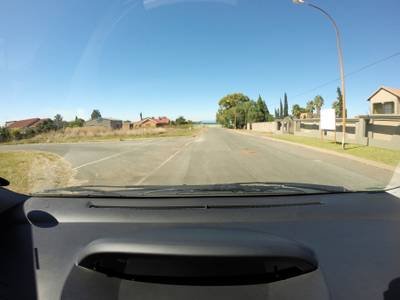
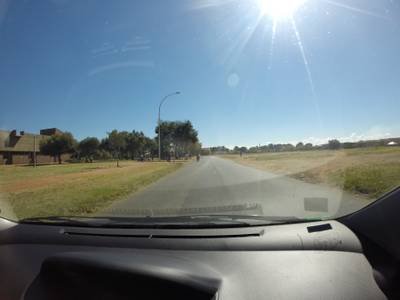
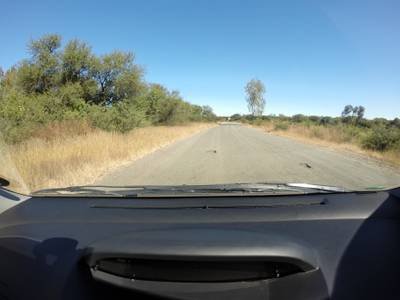
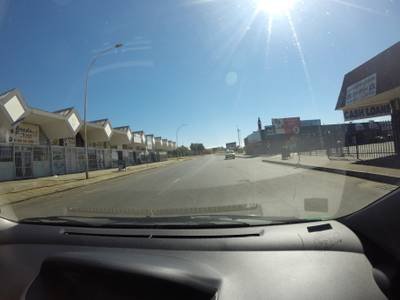
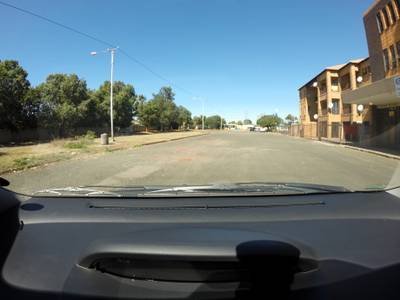
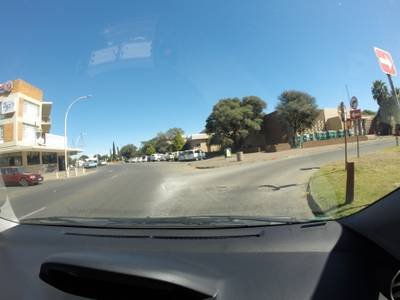
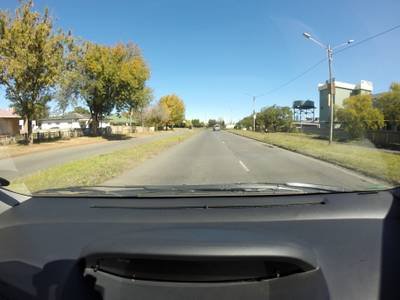
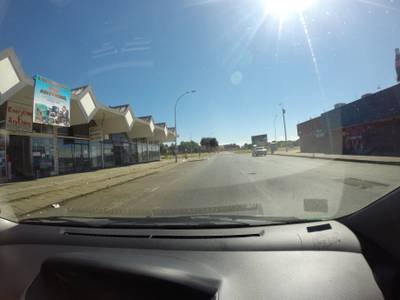
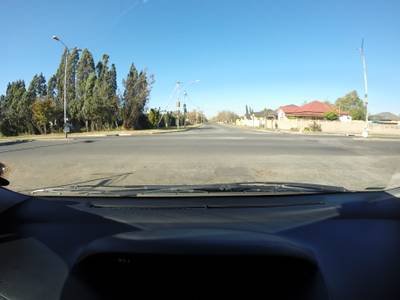
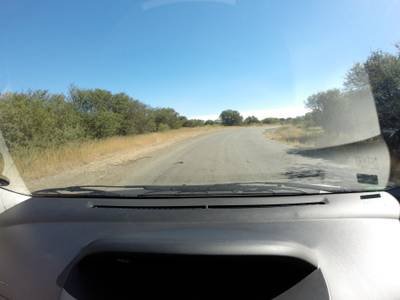
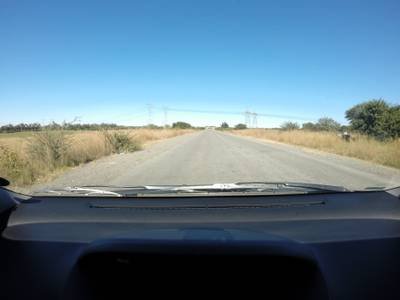
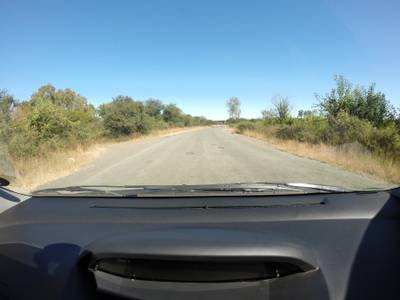
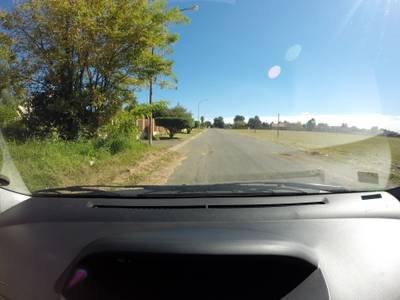
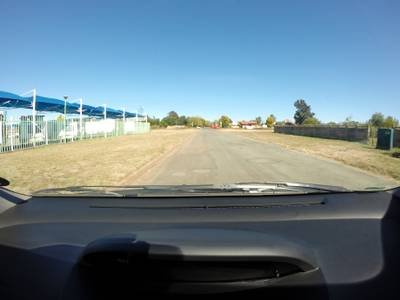
Class balance #
There are 1 annotation classes in the dataset. Find the general statistics and balances for every class in the table below. Click any row to preview images that have labels of the selected class. Sort by column to find the most rare or prevalent classes.
Class ㅤ | Images ㅤ | Objects ㅤ | Count on image average | Area on image average |
---|---|---|---|---|
pothole➔ rectangle | 2945 | 7588 | 2.58 | 0.14% |
Images #
Explore every single image in the dataset with respect to the number of annotations of each class it has. Click a row to preview selected image. Sort by any column to find anomalies and edge cases. Use horizontal scroll if the table has many columns for a large number of classes in the dataset.
Object distribution #
Interactive heatmap chart for every class with object distribution shows how many images are in the dataset with a certain number of objects of a specific class. Users can click cell and see the list of all corresponding images.
Class sizes #
The table below gives various size properties of objects for every class. Click a row to see the image with annotations of the selected class. Sort columns to find classes with the smallest or largest objects or understand the size differences between classes.
Class | Object count | Avg area | Max area | Min area | Min height | Min height | Max height | Max height | Avg height | Avg height | Min width | Min width | Max width | Max width |
---|---|---|---|---|---|---|---|---|---|---|---|---|---|---|
pothole rectangle | 7588 | 0.06% | 1.93% | 0% | 3px | 0.11% | 215px | 7.79% | 36px | 1.3% | 15px | 0.41% | 911px | 24.76% |
Spatial Heatmap #
The heatmaps below give the spatial distributions of all objects for every class. These visualizations provide insights into the most probable and rare object locations on the image. It helps analyze objects' placements in a dataset.
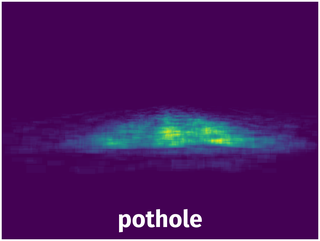
Objects #
Table contains all 7588 objects. Click a row to preview an image with annotations, and use search or pagination to navigate. Sort columns to find outliers in the dataset.
Object ID ㅤ | Class ㅤ | Image name click row to open | Image size height x width | Height ㅤ | Height ㅤ | Width ㅤ | Width ㅤ | Area ㅤ |
---|---|---|---|---|---|---|---|---|
1➔ | pothole rectangle | positive_G0105238.JPG | 2760 x 3680 | 37px | 1.34% | 143px | 3.89% | 0.05% |
2➔ | pothole rectangle | positive_G0105238.JPG | 2760 x 3680 | 53px | 1.92% | 307px | 8.34% | 0.16% |
3➔ | pothole rectangle | positive_G0105238.JPG | 2760 x 3680 | 43px | 1.56% | 185px | 5.03% | 0.08% |
4➔ | pothole rectangle | positive_G0105238.JPG | 2760 x 3680 | 31px | 1.12% | 115px | 3.12% | 0.04% |
5➔ | pothole rectangle | positive_G0105238.JPG | 2760 x 3680 | 19px | 0.69% | 89px | 2.42% | 0.02% |
6➔ | pothole rectangle | positive_G0089830.JPG | 2760 x 3680 | 99px | 3.59% | 241px | 6.55% | 0.23% |
7➔ | pothole rectangle | positive_G0089830.JPG | 2760 x 3680 | 29px | 1.05% | 81px | 2.2% | 0.02% |
8➔ | pothole rectangle | positive_G0089830.JPG | 2760 x 3680 | 31px | 1.12% | 261px | 7.09% | 0.08% |
9➔ | pothole rectangle | positive_G0089830.JPG | 2760 x 3680 | 15px | 0.54% | 77px | 2.09% | 0.01% |
10➔ | pothole rectangle | positive_G0067039.JPG | 2760 x 3680 | 19px | 0.69% | 79px | 2.15% | 0.01% |
License #
Citation #
If you make use of the Road Pothole Images data, please cite the following reference:
S. Nienaber, M.J. Booysen, R.S. Kroon, “Detecting potholes using simple image processing techniques and real-world footage”, SATC, July 2015, Pretoria, South Africa.
S. Nienaber, R.S. Kroon, M.J. Booysen , “A Comparison of Low-Cost Monocular Vision Techniques for Pothole Distance Estimation”, IEEE CIVTS, December 2015, Cape Town, South Africa.
If you are happy with Dataset Ninja and use provided visualizations and tools in your work, please cite us:
@misc{ visualization-tools-for-road-pothole-images-dataset,
title = { Visualization Tools for Road Pothole Images Dataset },
type = { Computer Vision Tools },
author = { Dataset Ninja },
howpublished = { \url{ https://datasetninja.com/road-pothole-images } },
url = { https://datasetninja.com/road-pothole-images },
journal = { Dataset Ninja },
publisher = { Dataset Ninja },
year = { 2024 },
month = { may },
note = { visited on 2024-05-10 },
}
Download #
Dataset Road Pothole Images can be downloaded in Supervisely format:
As an alternative, it can be downloaded with dataset-tools package:
pip install --upgrade dataset-tools
… using following python code:
import dataset_tools as dtools
dtools.download(dataset='Road Pothole Images', dst_dir='~/dataset-ninja/')
Make sure not to overlook the python code example available on the Supervisely Developer Portal. It will give you a clear idea of how to effortlessly work with the downloaded dataset.
The data in original format can be downloaded here.
Disclaimer #
Our gal from the legal dep told us we need to post this:
Dataset Ninja provides visualizations and statistics for some datasets that can be found online and can be downloaded by general audience. Dataset Ninja is not a dataset hosting platform and can only be used for informational purposes. The platform does not claim any rights for the original content, including images, videos, annotations and descriptions. Joint publishing is prohibited.
You take full responsibility when you use datasets presented at Dataset Ninja, as well as other information, including visualizations and statistics we provide. You are in charge of compliance with any dataset license and all other permissions. You are required to navigate datasets homepage and make sure that you can use it. In case of any questions, get in touch with us at hello@datasetninja.com.