Introduction #
In their pursuit to address the significant challenges posed by pests and diseases in agriculture, the authors of the Rust and Leaf Miner in Coffee Crop dataset have developed an algorithm designed for the detection of rust (Hemileia vastatrix) and leaf miner (Leucoptera coffeella) within coffee leaves (Coffea arabica). Their solution extends to quantifying disease severity, facilitated through a mobile application functioning as a high-level interface for model inferences.
Within the realm of coffee cultivation, numerous phytosanitary issues abound, including bacteria, fungi, viruses, nematodes, and pests. Among these, rust has emerged as a particularly impactful concern since its inception in East Africa in 1869. Rust inflicts severe harm, leading to the premature defoliation of coffee trees and substantial production declines in subsequent years. Reports indicate production reductions of up to 34%. Similarly, leaf miner infestations, which began in Africa around the mid-1850s, have now become the most prevalent pest globally. Leaf miners damage the leaf epidermis, causing malnourishment and desiccation, resulting in production losses of up to 40%.
To combat these phytopathologies, the authors have turned to artificial intelligence, a field that has witnessed remarkable advancements in recent years. These advancements, particularly in image recognition, owe their success not only to more robust hardware and abundant data but also to the development of new and improved algorithms, including convolutional neural networks (CNNs). CNNs are particularly well-suited for tasks involving image analysis as they can learn and generalize features from images effectively.
Data Acquisition
The dataset for this study was meticulously assembled through the random collection of coffee leaves afflicted with rust and leaf miners from a farm located in Brazil. These leaves were then photographed in a laboratory against a white background using a smartphone camera operating in the RGB color system, which is the standard color space adopted by digital cameras. The RGB color system has demonstrated satisfactory performance in machine-learning applications when compared to other color spaces.
The resulting dataset comprises 285 samples of rust-infested leaves and 257 samples of leaves affected by the leaf miner. Initially, the images were captured at a resolution of 4000 x 2250 pixels. However, to align with the standards set by Liu et al. for Single Shot MultiBox Detector (SSD) and to optimize training time and computational resources, the images were resized to dimensions of 300 x 300 pixels and 512 x 512 pixels.
Creating a labeled dataset poses one of the central challenges in object detection techniques. It necessitates the manual annotation of object locations within images, often involving the delineation of bounding boxes. This process is not only resource-intensive but also time-consuming and costly, particularly when numerous objects per image need to be labeled, specialized knowledge is required, or the dataset is extensive.
Data Labeling
The authors describe that the initially collected photos underwent manual labeling using LabelImage software. However, to streamline this labor-intensive process and expedite future dataset expansion, an algorithm was devised for automatic image labeling, adopting a semi-supervised approach. Consequently, for each new unlabeled image, a pre-trained model, fueled by a limited number of strongly-labeled images, inferred the objects of interest.
The automatically generated labels underwent a meticulous review process due to inherent inaccuracies in detecting objects within certain images. Unaddressed inaccuracies could potentially diminish model accuracy. Nevertheless, this revised labeling process, albeit more rapid and cost-effective, continues to demand less human effort compared to conventional approaches.
Summary #
Rust (Hemileia Vastatrix) and Leaf Miner (Leucoptera Coffeella) in Coffee Crop (Coffea Arabica) is a dataset for an object detection task. It is used in the agricultural industry.
The dataset consists of 542 images with 3487 labeled objects belonging to 2 different classes including rust and leaf miner.
Images in the Rust and Leaf Miner in Coffee Crop dataset have bounding box annotations. All images are labeled (i.e. with annotations). There are no pre-defined train/val/test splits in the dataset. The dataset was released in 2020 by the São Paulo State Faculty of Technology, Brazil.
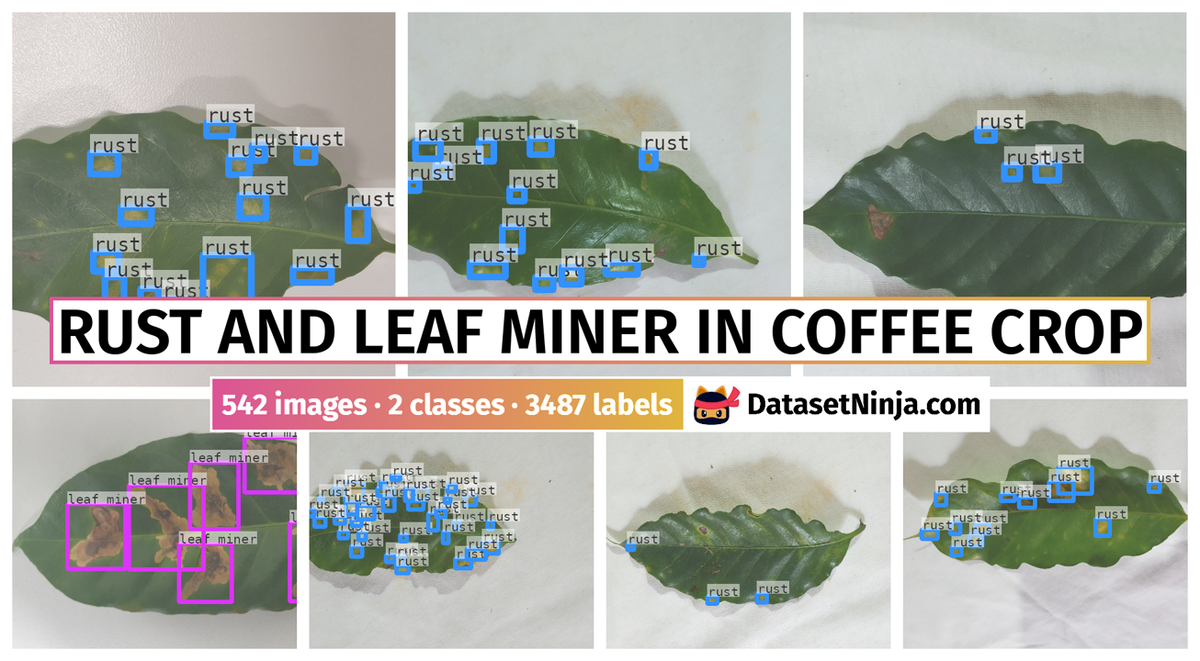
Explore #
Rust and Leaf Miner in Coffee Crop dataset has 542 images. Click on one of the examples below or open "Explore" tool anytime you need to view dataset images with annotations. This tool has extended visualization capabilities like zoom, translation, objects table, custom filters and more. Hover the mouse over the images to hide or show annotations.
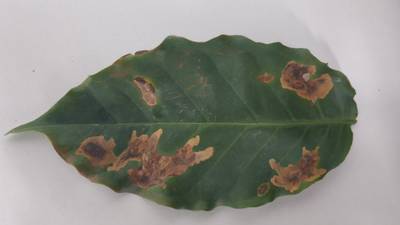

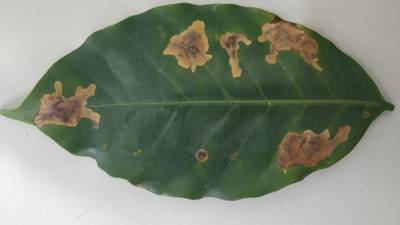
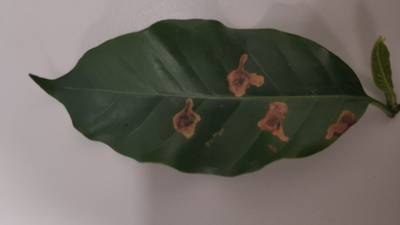
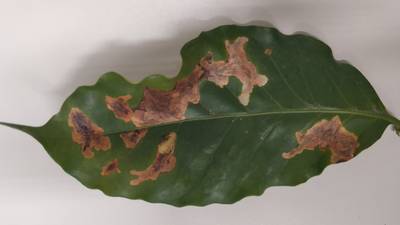
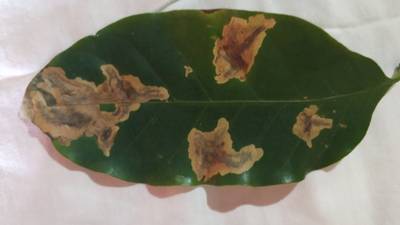
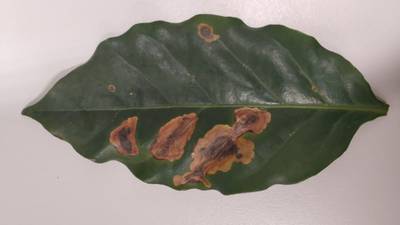
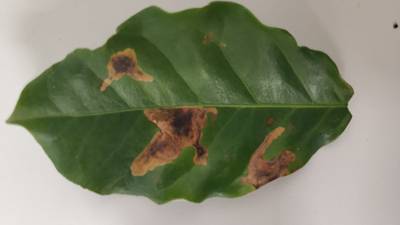
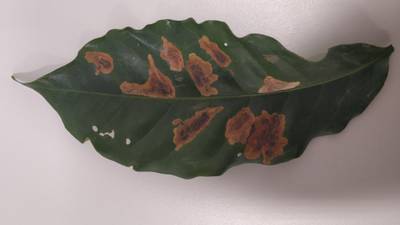
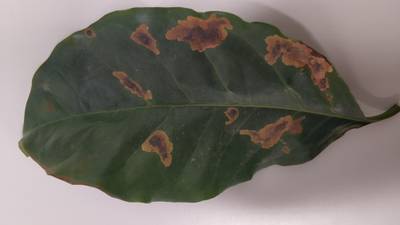
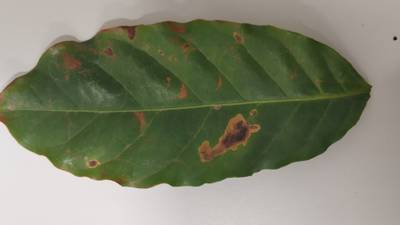
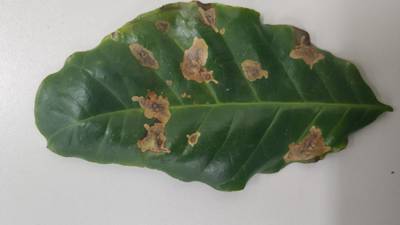
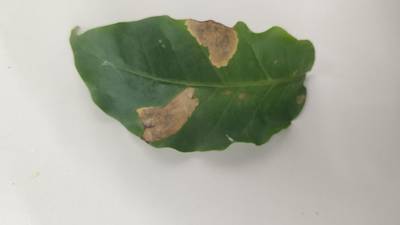
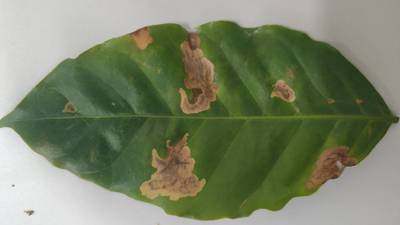
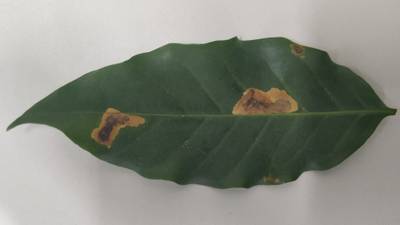
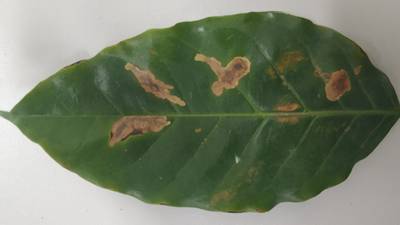

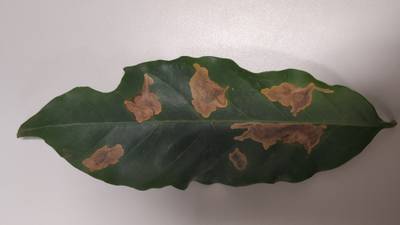
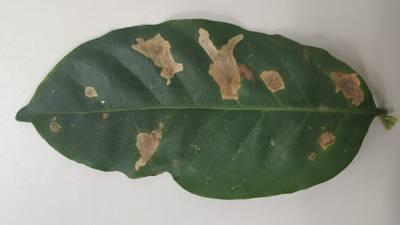
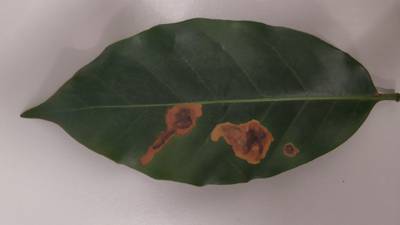
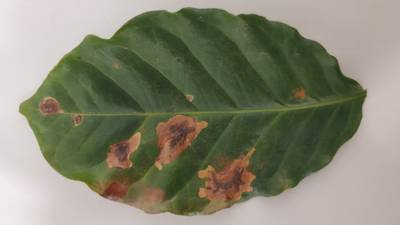


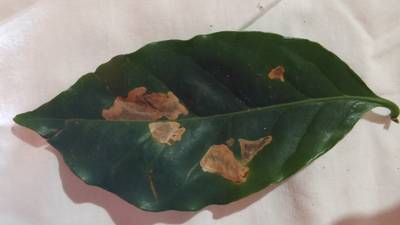
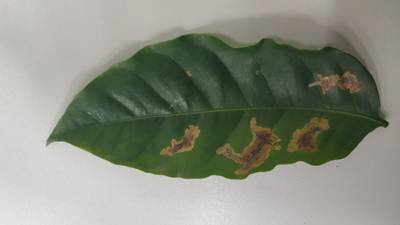
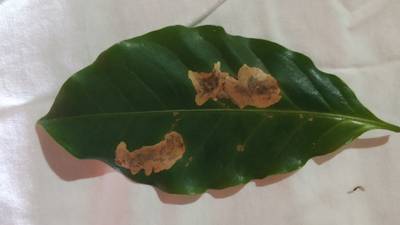
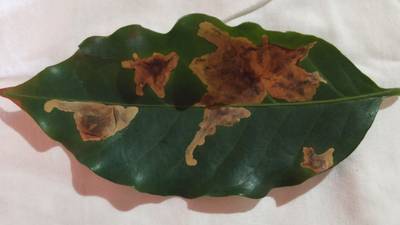
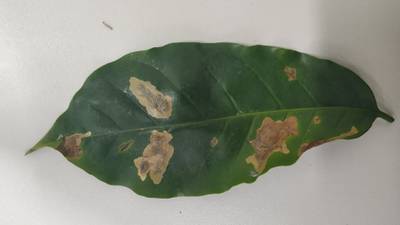

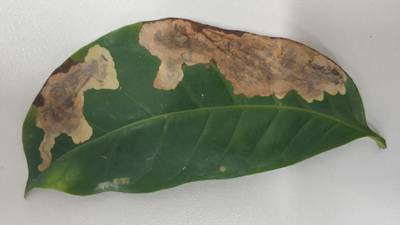
Class balance #
There are 2 annotation classes in the dataset. Find the general statistics and balances for every class in the table below. Click any row to preview images that have labels of the selected class. Sort by column to find the most rare or prevalent classes.
Class ㅤ | Images ㅤ | Objects ㅤ | Count on image average | Area on image average |
---|---|---|---|---|
rust➔ rectangle | 285 | 2242 | 7.87 | 2.42% |
leaf miner➔ rectangle | 257 | 1245 | 4.84 | 14.47% |
Co-occurrence matrix #
Co-occurrence matrix is an extremely valuable tool that shows you the images for every pair of classes: how many images have objects of both classes at the same time. If you click any cell, you will see those images. We added the tooltip with an explanation for every cell for your convenience, just hover the mouse over a cell to preview the description.
Images #
Explore every single image in the dataset with respect to the number of annotations of each class it has. Click a row to preview selected image. Sort by any column to find anomalies and edge cases. Use horizontal scroll if the table has many columns for a large number of classes in the dataset.
Object distribution #
Interactive heatmap chart for every class with object distribution shows how many images are in the dataset with a certain number of objects of a specific class. Users can click cell and see the list of all corresponding images.
Class sizes #
The table below gives various size properties of objects for every class. Click a row to see the image with annotations of the selected class. Sort columns to find classes with the smallest or largest objects or understand the size differences between classes.
Class | Object count | Avg area | Max area | Min area | Min height | Min height | Max height | Max height | Avg height | Avg height | Min width | Min width | Max width | Max width |
---|---|---|---|---|---|---|---|---|---|---|---|---|---|---|
rust rectangle | 2242 | 0.31% | 8.62% | 0.02% | 37px | 1.06% | 1317px | 32.92% | 144px | 6.31% | 36px | 0.9% | 976px | 26.18% |
leaf miner rectangle | 1245 | 3.04% | 23.53% | 0.08% | 74px | 3.29% | 1410px | 62.67% | 436px | 19.36% | 80px | 2% | 2273px | 56.83% |
Spatial Heatmap #
The heatmaps below give the spatial distributions of all objects for every class. These visualizations provide insights into the most probable and rare object locations on the image. It helps analyze objects' placements in a dataset.
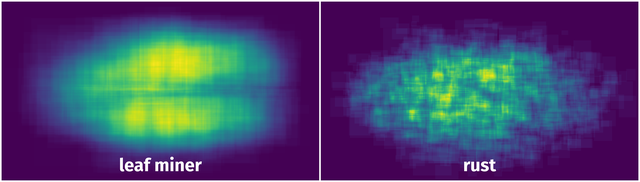
Objects #
Table contains all 3487 objects. Click a row to preview an image with annotations, and use search or pagination to navigate. Sort columns to find outliers in the dataset.
Object ID ㅤ | Class ㅤ | Image name click row to open | Image size height x width | Height ㅤ | Height ㅤ | Width ㅤ | Width ㅤ | Area ㅤ |
---|---|---|---|---|---|---|---|---|
1➔ | rust rectangle | name319.jpg | 2250 x 4000 | 107px | 4.76% | 242px | 6.05% | 0.29% |
2➔ | rust rectangle | name319.jpg | 2250 x 4000 | 293px | 13.02% | 157px | 3.92% | 0.51% |
3➔ | rust rectangle | name319.jpg | 2250 x 4000 | 159px | 7.07% | 126px | 3.15% | 0.22% |
4➔ | rust rectangle | name319.jpg | 2250 x 4000 | 112px | 4.98% | 131px | 3.27% | 0.16% |
5➔ | rust rectangle | name319.jpg | 2250 x 4000 | 104px | 4.62% | 101px | 2.52% | 0.12% |
6➔ | rust rectangle | name319.jpg | 2250 x 4000 | 156px | 6.93% | 159px | 3.98% | 0.28% |
7➔ | rust rectangle | name319.jpg | 2250 x 4000 | 123px | 5.47% | 112px | 2.8% | 0.15% |
8➔ | rust rectangle | name319.jpg | 2250 x 4000 | 74px | 3.29% | 107px | 2.67% | 0.09% |
9➔ | rust rectangle | name319.jpg | 2250 x 4000 | 131px | 5.82% | 163px | 4.08% | 0.24% |
10➔ | rust rectangle | name319.jpg | 2250 x 4000 | 102px | 4.53% | 148px | 3.7% | 0.17% |
License #
Citation #
If you make use of the Rust and Leaf Miner in Coffee Crop data, please cite the following reference:
Brito Silva, Lucas; Cavalcante Carneiro, Álvaro Leandro; Silveira Almeida Renaud Faulin, Marisa (2020),
“rust (Hemileia vastatrix) and leaf miner (Leucoptera coffeella) in coffee crop (Coffea arabica).”,
Mendeley Data, V5,
doi: 10.17632/vfxf4trtcg.5
If you are happy with Dataset Ninja and use provided visualizations and tools in your work, please cite us:
@misc{ visualization-tools-for-rust-and-leaf-miner-in-coffee-crop-dataset,
title = { Visualization Tools for Rust and Leaf Miner in Coffee Crop Dataset },
type = { Computer Vision Tools },
author = { Dataset Ninja },
howpublished = { \url{ https://datasetninja.com/rust-and-leaf-miner-in-coffee-crop } },
url = { https://datasetninja.com/rust-and-leaf-miner-in-coffee-crop },
journal = { Dataset Ninja },
publisher = { Dataset Ninja },
year = { 2025 },
month = { jan },
note = { visited on 2025-01-15 },
}
Download #
Dataset Rust and Leaf Miner in Coffee Crop can be downloaded in Supervisely format:
As an alternative, it can be downloaded with dataset-tools package:
pip install --upgrade dataset-tools
… using following python code:
import dataset_tools as dtools
dtools.download(dataset='Rust and Leaf Miner in Coffee Crop', dst_dir='~/dataset-ninja/')
Make sure not to overlook the python code example available on the Supervisely Developer Portal. It will give you a clear idea of how to effortlessly work with the downloaded dataset.
The data in original format can be downloaded here.
Disclaimer #
Our gal from the legal dep told us we need to post this:
Dataset Ninja provides visualizations and statistics for some datasets that can be found online and can be downloaded by general audience. Dataset Ninja is not a dataset hosting platform and can only be used for informational purposes. The platform does not claim any rights for the original content, including images, videos, annotations and descriptions. Joint publishing is prohibited.
You take full responsibility when you use datasets presented at Dataset Ninja, as well as other information, including visualizations and statistics we provide. You are in charge of compliance with any dataset license and all other permissions. You are required to navigate datasets homepage and make sure that you can use it. In case of any questions, get in touch with us at hello@datasetninja.com.