Introduction #
The authors have collected and labeled a new large Scut Head Dataset which includes 4405 images with 111251 heads annotated. The dataset consists of two parts. part a includes 2000 images sampled from monitor videos of classrooms in an university with 67321 heads annotated. part b includes 2405 images crawled from internet with 43930 heads annotated. They have labelled every visable heads with xmin, ymin, xmax and ymax coordinates and ensured that annotations cover the entire head including the blocked parts but without extra background. Both part a and part b are divided into training and testing parts.
Motivation
Face detection and pedestrian detection represent pivotal challenges in the field of computer vision, and notable advancements have been made in recent years. However, practical applications reveal certain limitations. For instance, face detection algorithms are restricted to identifying frontal faces, rendering individuals facing away from the camera undetectable. Similarly, in complex indoor environments, where body parts may be obscured, pedestrian detection encounters difficulties. Head detection, on the other hand, presents fewer limitations and proves more adept at locating and enumerating individuals, particularly in indoor settings. Nevertheless, detecting heads indoors poses its own set of challenges, including variations in scale and appearance, as well as the task of identifying smaller heads. Addressing these challenges necessitates effective exploitation of extracted features to accurately localize heads and discern them from the background—a formidable task. Prior approaches have often relied on leveraging multi-scale features derived from different layers of deep convolutional neural networks to tackle these complexities.
Detecting small heads presents a distinct challenge in head detection tasks. To address this, the authors have introduced a cascaded multi-scale architecture tailored specifically for small head detection. Their approach centers on refining local detection outcomes by enhancing the resolution of image segments. The proposed architecture comprises two distinct detectors: a global detector and a local detector. The global detector is tasked with identifying large heads and subsequently relays this information to the local detector, which then focuses on analyzing enlarged segments containing small heads for more precise detection. Additionally, owing to the scarcity of datasets dedicated to head detection, the authors have curated and annotated a large-scale dataset specifically for this purpose, named SCUT-HEAD.
Dataset description
The authors have curated and annotated a comprehensive head detection dataset named SCUT-HEAD, which comprises two distinct parts. part a consists of 2000 images extracted from surveillance videos captured in university classrooms, encompassing a total of 67,321 annotated heads. To ensure dataset diversity and reduce similarity, representative images were thoughtfully selected, considering the inherent similarities across classroom settings.
An example image and annotations of Part A in SCUT-HEAD.
On the other hand, part b consists of 2405 images sourced from the internet, with 43,930 annotated heads. Each visible head in both part a and part b is meticulously labeled with coordinates (xmin, ymin, xmax, ymax), encompassing the entire head region while excluding any extraneous background.
An example image and annotations of Part B in SCUT-HEAD.
Both part a and part b are further divided into training and testing subsets. Specifically, part a includes 1500 images for training and 500 for testing, while part b comprises 1905 training images and 500 testing images.
Summary #
Scut Head Dataset is a dataset for an object detection task. It is used in the surveillance industry.
The dataset consists of 4405 images with 111248 labeled objects belonging to 1 single class (person).
Images in the Scut Head dataset have bounding box annotations. There is 1 unlabeled image (i.e. without annotations). There are 3 splits in the dataset: train (2543 images), test (1000 images), and val (862 images). Additionally, every image marked with part a or part b tag. The dataset was released in 2018 by the South University of Technology, China.
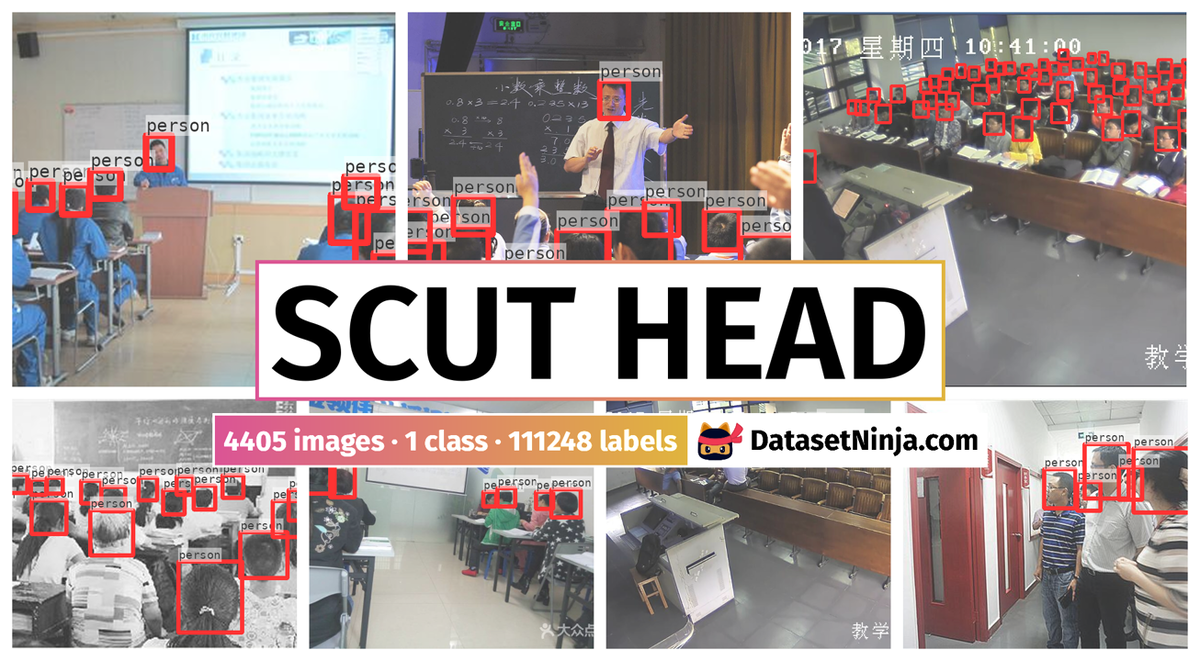
Explore #
Scut Head dataset has 4405 images. Click on one of the examples below or open "Explore" tool anytime you need to view dataset images with annotations. This tool has extended visualization capabilities like zoom, translation, objects table, custom filters and more. Hover the mouse over the images to hide or show annotations.
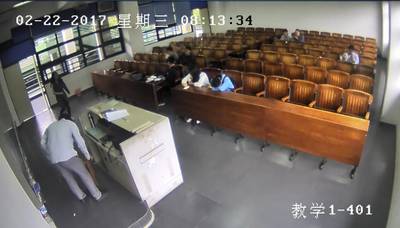
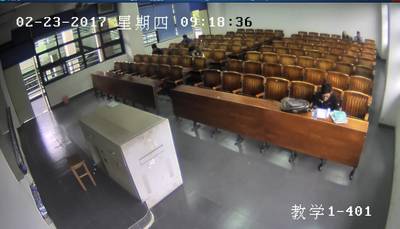

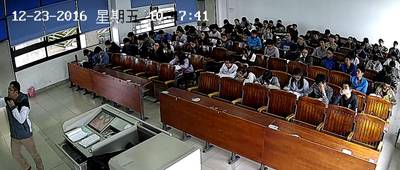
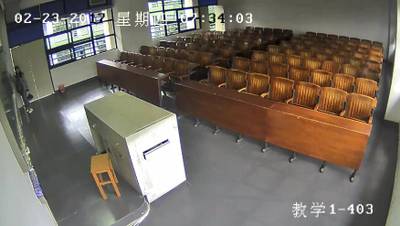
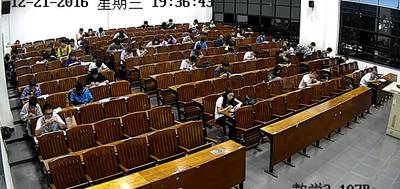
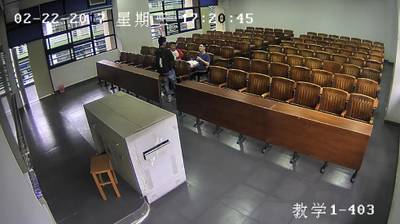
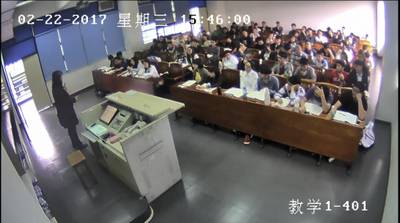
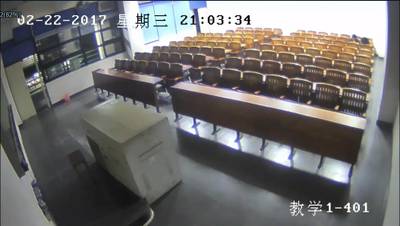
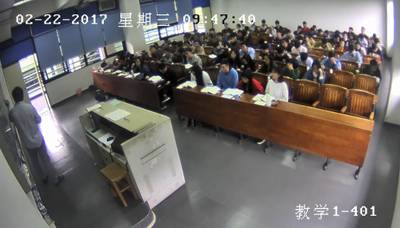
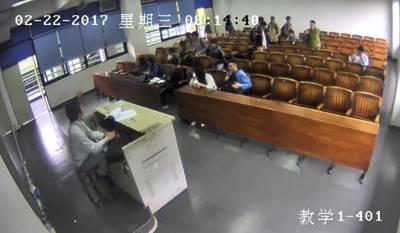
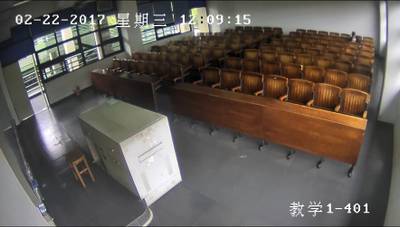
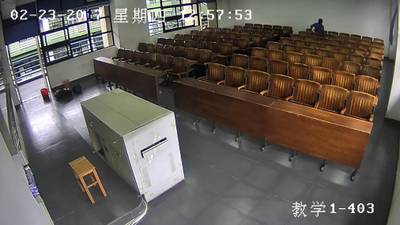

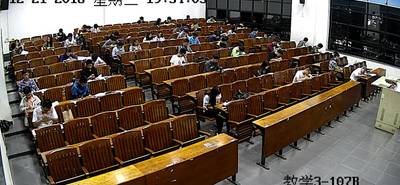
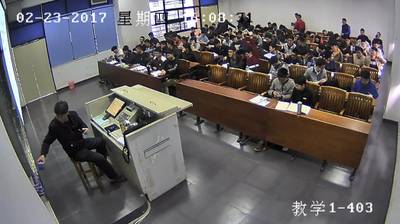
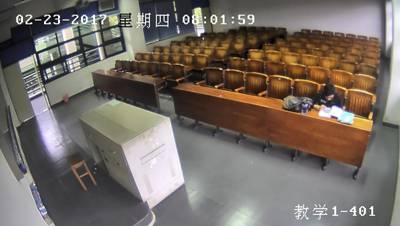
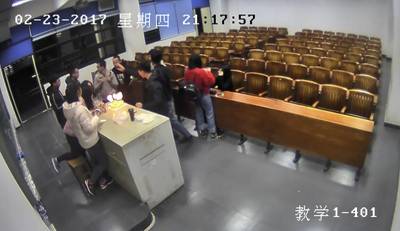
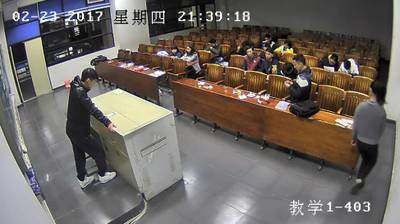
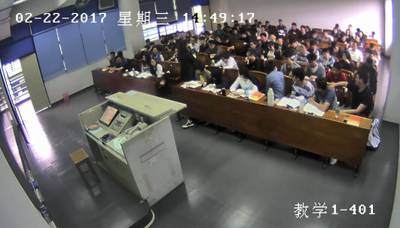
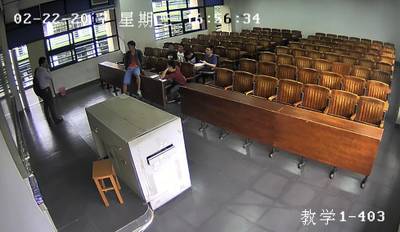
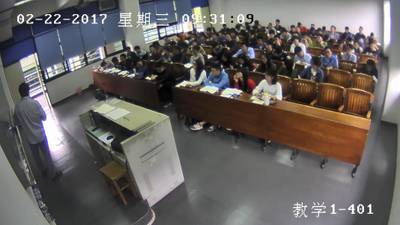
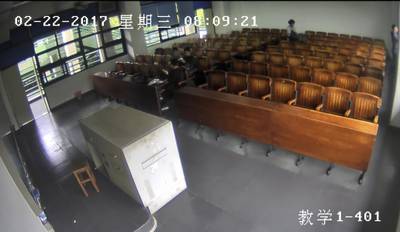
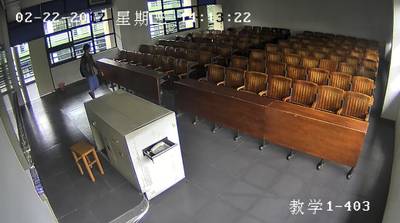

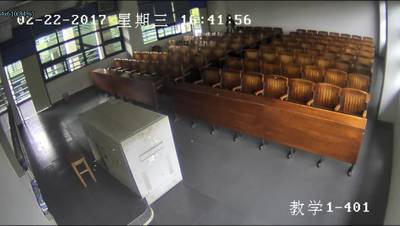

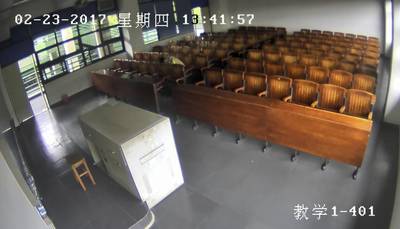
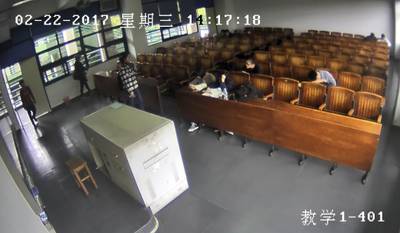

Class balance #
There are 1 annotation classes in the dataset. Find the general statistics and balances for every class in the table below. Click any row to preview images that have labels of the selected class. Sort by column to find the most rare or prevalent classes.
Class ã…¤ | Images ã…¤ | Objects ã…¤ | Count on image average | Area on image average |
---|---|---|---|---|
personâž” rectangle | 4404 | 111248 | 25.26 | 8.46% |
Images #
Explore every single image in the dataset with respect to the number of annotations of each class it has. Click a row to preview selected image. Sort by any column to find anomalies and edge cases. Use horizontal scroll if the table has many columns for a large number of classes in the dataset.
Class sizes #
The table below gives various size properties of objects for every class. Click a row to see the image with annotations of the selected class. Sort columns to find classes with the smallest or largest objects or understand the size differences between classes.
Class | Object count | Avg area | Max area | Min area | Min height | Min height | Max height | Max height | Avg height | Avg height | Min width | Min width | Max width | Max width |
---|---|---|---|---|---|---|---|---|---|---|---|---|---|---|
person rectangle | 111248 | 0.35% | 23.82% | 0.01% | 3px | 0.55% | 1357px | 74.77% | 40px | 6.24% | 4px | 0.44% | 1269px | 38.93% |
Spatial Heatmap #
The heatmaps below give the spatial distributions of all objects for every class. These visualizations provide insights into the most probable and rare object locations on the image. It helps analyze objects' placements in a dataset.
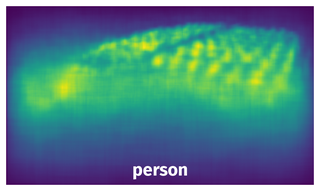
Objects #
Table contains all 99528 objects. Click a row to preview an image with annotations, and use search or pagination to navigate. Sort columns to find outliers in the dataset.
Object ID ã…¤ | Class ã…¤ | Image name click row to open | Image size height x width | Height ã…¤ | Height ã…¤ | Width ã…¤ | Width ã…¤ | Area ã…¤ |
---|---|---|---|---|---|---|---|---|
1âž” | person rectangle | PartB_00514.jpg | 267 x 400 | 54px | 20.22% | 47px | 11.75% | 2.38% |
2âž” | person rectangle | PartB_00514.jpg | 267 x 400 | 33px | 12.36% | 25px | 6.25% | 0.77% |
3âž” | person rectangle | PartB_00514.jpg | 267 x 400 | 42px | 15.73% | 35px | 8.75% | 1.38% |
4âž” | person rectangle | PartB_00514.jpg | 267 x 400 | 34px | 12.73% | 24px | 6% | 0.76% |
5âž” | person rectangle | PartB_00514.jpg | 267 x 400 | 28px | 10.49% | 22px | 5.5% | 0.58% |
6âž” | person rectangle | PartB_00514.jpg | 267 x 400 | 29px | 10.86% | 21px | 5.25% | 0.57% |
7âž” | person rectangle | PartB_00514.jpg | 267 x 400 | 45px | 16.85% | 52px | 13% | 2.19% |
8âž” | person rectangle | PartB_00514.jpg | 267 x 400 | 55px | 20.6% | 56px | 14% | 2.88% |
9âž” | person rectangle | PartA_01076.jpg | 715 x 1259 | 53px | 7.41% | 45px | 3.57% | 0.26% |
10âž” | person rectangle | PartA_01076.jpg | 715 x 1259 | 22px | 3.08% | 23px | 1.83% | 0.06% |
License #
The SCUT-HEAD database is free to the academic community for research purpose usage only.
Citation #
If you make use of the Scut Head data, please cite the following reference:
@article{peng2018detecting,
title={Detecting Heads using Feature Refine Net and Cascaded Multi-scale Architecture},
author={Peng, Dezhi and Sun, Zikai and Chen, Zirong and Cai, Zirui and Xie, Lele and Jin, Lianwen},
journal={arXiv preprint arXiv:1803.09256},
year={2018}
}
If you are happy with Dataset Ninja and use provided visualizations and tools in your work, please cite us:
@misc{ visualization-tools-for-scut-head-dataset,
title = { Visualization Tools for Scut Head Dataset },
type = { Computer Vision Tools },
author = { Dataset Ninja },
howpublished = { \url{ https://datasetninja.com/scut-head } },
url = { https://datasetninja.com/scut-head },
journal = { Dataset Ninja },
publisher = { Dataset Ninja },
year = { 2025 },
month = { jun },
note = { visited on 2025-06-08 },
}
Download #
Dataset Scut Head can be downloaded in Supervisely format:
As an alternative, it can be downloaded with dataset-tools package:
pip install --upgrade dataset-tools
… using following python code:
import dataset_tools as dtools
dtools.download(dataset='Scut Head', dst_dir='~/dataset-ninja/')
Make sure not to overlook the python code example available on the Supervisely Developer Portal. It will give you a clear idea of how to effortlessly work with the downloaded dataset.
The data in original format can be downloaded here:
Disclaimer #
Our gal from the legal dep told us we need to post this:
Dataset Ninja provides visualizations and statistics for some datasets that can be found online and can be downloaded by general audience. Dataset Ninja is not a dataset hosting platform and can only be used for informational purposes. The platform does not claim any rights for the original content, including images, videos, annotations and descriptions. Joint publishing is prohibited.
You take full responsibility when you use datasets presented at Dataset Ninja, as well as other information, including visualizations and statistics we provide. You are in charge of compliance with any dataset license and all other permissions. You are required to navigate datasets homepage and make sure that you can use it. In case of any questions, get in touch with us at hello@datasetninja.com.