Introduction #
The authors present Sea Turtle ID 2022 Dataset, the inaugural public dataset comprising sea turtle photographs taken in their natural habitat. This extensive dataset consists of 8,729 images capturing 438 distinct individuals over a remarkable span of 13 years, marking it as the most extensive animal re-identification dataset to date. Each photograph comes with comprehensive annotations, including identity markers, encounter timestamps, and segmentation masks outlining different body parts.
Motivation
Recognizing individual animals through image-based re-identification is crucial for various wildlife studies, including population monitoring, behavioral analysis, and wildlife management. With the expansion of photo databases spanning multiple years, there’s a growing need for automated methods to streamline the labor-intensive process of individual animal identification. In response, numerous automatic re-identification methods have emerged in recent years. These methods are assessed using benchmark databases that cover various animal groups, such as mammals, reptiles, and smaller organisms. Typically, these databases are divided into a reference set, containing images with known individual identities, and a query set, containing images where identities need to be matched with the reference set. In deep learning, these sets are commonly referred to as training and test sets. The quality of these datasets significantly influences the evaluation of re-identification methods. Hence, it’s crucial for the dataset and its division to mimic real-world scenarios. This entails ensuring that images in the query and reference sets originate from different encounters, such as burst mode in camera traps or consecutive video frames. Additionally, incorporating images with unknown identities, different locations, varied capture conditions, and images depicting changes in animal appearances over time are essential factors to consider.
The long-span difference in visual appearance of one individual sea turtle. The shapes of the facial scales remain the same, but other features, e.g., coloration, pigmentation, shape, and scratches, change over time.
During a single encounter, images typically share common characteristics as the encounter occurs within a short timeframe. To differentiate between various encounters and factors within a dataset effectively, including capture date and time in the metadata, known as timestamps, is crucial. Without timestamp information, datasets are often divided into reference and query sets purely at random. However, this random split can lead to issues, as images in the training and test sets may originate from the same encounter or observation, resulting in unintended data leakage between training and testing phases. This can potentially cause overfitting to the specific factors of a particular encounter rather than learning a generalized representation of each individual. Implicitly, a random split assumes encountering the same factors in the future, which is highly unrealistic. In contrast, utilizing timestamps allows for time-aware splits, where images from a specific time period are grouped together in either the reference or the query set. This approach reflects a more realistic scenario, where new factors may be encountered in future observations.
Dataset description
The authors introduce a novel dataset with photographs of loggerhead sea turtles (Caretta caretta) – the SeaTurtleID2022. The dataset was collected over 13 years and consists of 8729 high-resolution photographs of 438 unique individuals. Each photograph includes various annotations, e.g., identities, encounter timestamps, and body parts segmentation masks. The SeaTurtleID2022 is the longest-spanned public wild animal image dataset and the only public dataset of sea turtles with photographs captured in the wild. In contrast to existing datasets, the SeaTurtleID2022 allows for two realistic and ecologically motivated splits instead of a ”random” split:
- time-aware closed-set: with reference images belonging to different encounters than query ones,
- time-aware open-set: with new unknown individuals (i.e., newly introduced to population) in test and validation sets (common in ecology).
While the primary purpose of the SeaTurtleID2022 dataset is animal re-identification, it also serves as a valuable resource for evaluating various fundamental computer vision tasks, including:
- Object detection
- Instance segmentation
- Fully and weakly supervised semantic segmentation
- 3D reconstruction
- Concept drift analysis
It’s worth noting that SeaTurtleID2022 mitigates common limitations found in other human re-identification datasets. For instance, datasets focusing on human faces often suffer from low-resolution images, restricted pose variations, limited time spans, and privacy concerns arising from either artificial generation or web crawling for data collection.
Location and species
All photographs in the dataset were captured in Laganas Bay, located on Zakynthos Island, Greece, at coordinates 37°43′N, 20°52′E, spanning from 2010 to 2022, during the months of May to October. Laganas Bay serves as a primary breeding ground for Mediterranean loggerhead sea turtles. Annually, approximately 300 female turtles undertake migratory journeys to the island for breeding purposes, returning every 2 to 3 years. However, some individuals establish residency on the island and can be observed in consecutive breeding seasons. Loggerhead sea turtles exhibit long lifespans, with some individuals retaining reproductive capability for over three decades, resulting in extensive image recordings spanning multiple years for certain individuals. Sea turtles are well-suited for photo-identification due to their distinctive scale patterns. Specifically, the polygonal scales present on the lateral (side) and dorsal (top) surfaces of their heads are unique to each individual and remain consistent throughout their lifetimes. It is noteworthy that the scale patterns on the left and right sides of a turtle’s head differ, further enhancing their identification potential.
Photographic procedure
All underwater photographs were captured during snorkeling surveys, taken from varying distances ranging from 7 meters to just a few centimeters. Three different cameras were employed throughout the survey periods:
- From 2010 to 2013, a Canon IXUS 105 digital compact camera was utilized, housed within a Canon underwater housing.
- In the years 2014 to 2017, a Canon 6D full-frame DSLR camera was employed, paired with a Sigma 15mm fisheye lens and encased in an Ikelite underwater housing.
- From 2018 to 2022, the same Canon 6D camera setup was used, with the addition of an INON Z330 external flash. The resolutions of the photographs varied, ranging from 4000×3000 pixels for the Canon IXUS camera to 5472×3648 pixels for the Canon 6D, with an average resolution of 5269×3564 pixels. The water depth during photography sessions fluctuated between 1 to 8 meters, with the majority of photographs taken at depths less than 5 meters.
Images captured from 2014 to 2022 generally exhibit higher quality due to the utilization of a more advanced camera setup and shorter camera-to-subject distances. However, the use of fisheye lenses may introduce barrel-shaped distortion, particularly noticeable in close-up shots. Additionally, the incorporation of an external flash resulted in more natural color reproduction in the images.
Selected individual turtle (t023) from the SeaTurtleID2022 database, photographed with three different mcamera set-ups. Photographs taken with the DSLR camera are of higher quality, and the additional use of flash recovers the natural colouration of the animal. All the photographs were cropped for illustration purposes.
Containing 8729 photographs capturing 438 individual sea turtles, this dataset stands as the most comprehensive publicly available resource for sea turtle identification in their natural habitat. These images are presented in their original resolution and showcase a variety of backgrounds. Around 90% of the photographs boast dimensions of 5472×3648 pixels, with an average size of 5269×3564 pixels. On average, the dimensions dedicated to the turtle’s head occupy approximately 635×554 pixels within each image.
Number of photographs for each of the 438 turtles. The orange line corresponds to 10 photographs.
The dataset contains photographs continuously captured over 13 years from 2010 to 2022. In contrast to most existing animal datasets that are usually collected in controlled environments and/or over a short time span.
Time-related statistics within the SeaTurtleID2022 dataset: number of encounters per year (left), distribution of all individuals to the total number of observation years, i.e., recurrence of individuals (middle), and number of newly observed identities in each year (right).
Segmentation masks and bounding boxes
The dataset predominantly comprises photographs featuring visible turtle head and/or flipper. To facilitate detailed analysis and research, the authors have meticulously annotated these images with segmentation masks and bounding boxes for various body parts. In addition to the masks, they have included orientation details (such as left, right, top, top-right, top-left, front, or bottom) for each head mask, along with orientation (top or bottom) and location (front left/right or rear left/right) for flipper masks. These comprehensive annotations not only enable the enhancement and evaluation of turtle identification techniques but also pave the way for the development of innovative methods in object detection and semantic segmentation. The dataset includes multiple images from different angles and, therefore, provides a ground for the challenging task of 3D animal reconstruction.
Examples of body parts (head, carapace, flippers) segmentation masks.
Dataset splits and subsets
Traditionally, re-identification datasets are divided randomly into reference (training) and query (test) sets, a practice that can lead to unintended data leakage and artificially inflated performance metrics. Essentially, this means that images from the same observation may appear in both sets. To demonstrate this challenge, the authors present four images of a single turtle: two taken on the same day in 2011 and two on the same day in 2021.
Unwanted background similarities in photographs from same/similar locations or time of observations.
Matching images from the same day is relatively straightforward due to consistent background and coloration. However, images from different days or years lack this similarity, making them considerably more difficult to match. To address this issue, the authors propose two ecologically motivated splits that leverage timestamps to prevent information leakage from the test set to the training set. These splits, referred to as time-aware splits, offer a more realistic representation of real-world scenarios. Additionally, the authors provide predefined training/validation/test splits for easier comparison in future research, although validation may not always be necessary.
The time-aware closed-set split closely resembles a standard closed-set re-identification scenario, wherein all identities present in the validation/test sets are also available for training. This scenario is particularly applicable to environments with well-controlled populations, such as zoos or wildlife reservations. In constructing this split, the authors organize the data based on the acquisition date and partition it in a time-aware manner. Approximately 80% of the days’ worth of data are allocated to the development set (comprising both training and validation subsets), while the remaining days’ data constitute the test set. For instances where an individual turtle was observed only once, it was retained for training purposes. Specifically, the authors provide 438 identities for training and 270 for testing. The development set was further subdivided into training and validation subsets using the same approach.
The time-aware open-set split is established based on predefined cutoff time points, which are specific years delineating different periods. In this setup, each subset (training/validation/test) encompasses all images captured within consecutive time intervals. Essentially, this split formulates an open-set problem, mirroring the inherent dynamics and growth of the natural population. During the construction process, the authors utilized data from the 2010–2018 period for training, the entirety of 2019 for validation, and the 2020–2022 period for the test set. The training set comprises 357 identities, while the test set consists of 151 identities, with 51 newly observed identities among them. A similar new-to-known identity ratio is naturally reflected in the validation set, where 38 out of 83 identities are newly encountered.
Subset | Closed-set | Open-set | |
---|---|---|---|
Training | # of images | 4679 | 5303 |
# of identities | 438 | 357 | |
Validation | # of images | 1418 | 1118 |
# of identities | 91 | 83 | |
Test | # of images | 2632 | 2308 |
# of identities | 270 | 151 |
Provided time-aware datasets split and their statistics.
Note: The open-set split is much closer to the real-world re-identification settings than the closed-set problem. Therefore, the open-set split should be preferred for automated method evaluation over all datasets. In case closed-set evaluation is desired, then the time-aware split must be the preferred option over the random split.
Additionally, the authors offer three subsets that encompass different body parts, including full-body, flipper, and head, by extracting crops from the original resolution. The quantity of data points varies for each body part due to the visibility of certain features. Utilizing the time-aware closed-set approach, they formulated part-based sets with the following number of training/test samples: 6139 / 2650 full turtle bodies, 14849 / 6237 flipper, and 5956 / 2583 head.
Summary #
Sea Turtle ID 2022 Dataset is a dataset for instance segmentation, semantic segmentation, object detection, and identification tasks. It is used in the environmental research.
The dataset consists of 8729 images with 77937 labeled objects belonging to 3 different classes including turtle, flipper, and head.
Images in the Sea Turtle 2022 dataset have pixel-level instance segmentation and bounding box annotations. Due to the nature of the instance segmentation task, it can be automatically transformed into a semantic segmentation task (only one mask for every class). There are 3 (0% of the total) unlabeled images (i.e. without annotations). There are 3 splits in the dataset: train (5303 images), test (2308 images), and val (1118 images). Alternatively, the dataset could be split into 3 closed splits: split closed train (4679 images), split closed test (2632 images), and split closed val (1418 images), or into 3 random closed splits: split closed random train (4679 images), split closed random test (2632 images), and split closed random val (1418 images). Additionally, every image marked with its identity, date tags. Labels marked with its orientation and occluded. Explore it in Supervisely labelling tool. The dataset was released in 2022 by the Czech Technical University and Queen Mary University of London, UK.
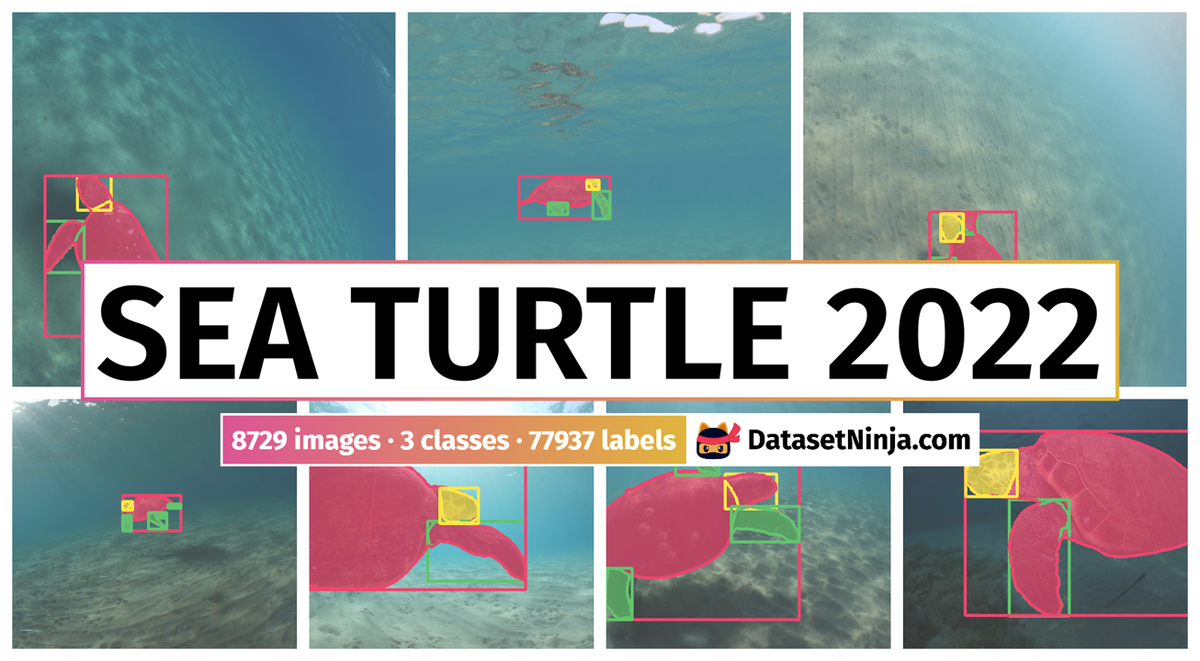
Explore #
Sea Turtle 2022 dataset has 8729 images. Click on one of the examples below or open "Explore" tool anytime you need to view dataset images with annotations. This tool has extended visualization capabilities like zoom, translation, objects table, custom filters and more. Hover the mouse over the images to hide or show annotations.
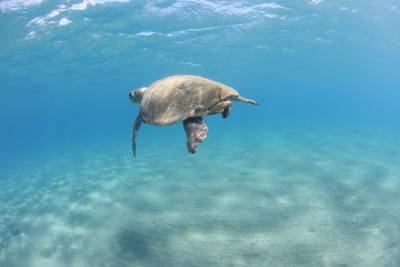


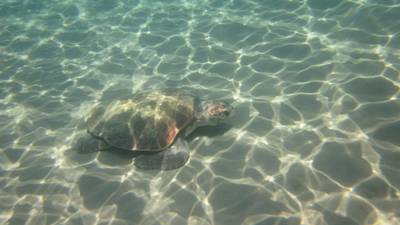
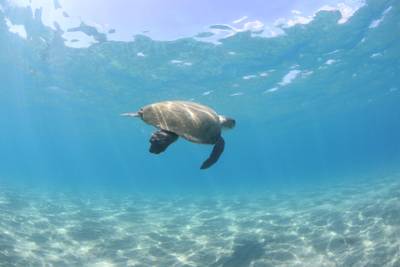
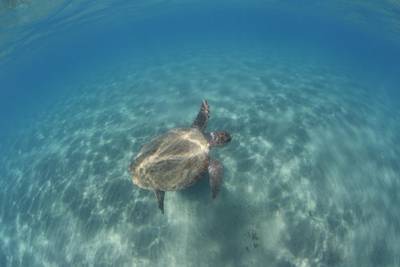
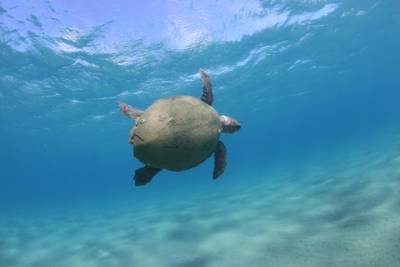





Class balance #
There are 3 annotation classes in the dataset. Find the general statistics and balances for every class in the table below. Click any row to preview images that have labels of the selected class. Sort by column to find the most rare or prevalent classes.
Class ㅤ | Images ㅤ | Objects ㅤ | Count on image average | Area on image average |
---|---|---|---|---|
turtle➔ any | 8709 | 17945 | 2.06 | 27.13% |
flipper➔ any | 8628 | 42891 | 4.97 | 6.25% |
head➔ any | 8526 | 17101 | 2.01 | 2.66% |
Co-occurrence matrix #
Co-occurrence matrix is an extremely valuable tool that shows you the images for every pair of classes: how many images have objects of both classes at the same time. If you click any cell, you will see those images. We added the tooltip with an explanation for every cell for your convenience, just hover the mouse over a cell to preview the description.
Images #
Explore every single image in the dataset with respect to the number of annotations of each class it has. Click a row to preview selected image. Sort by any column to find anomalies and edge cases. Use horizontal scroll if the table has many columns for a large number of classes in the dataset.
Object distribution #
Interactive heatmap chart for every class with object distribution shows how many images are in the dataset with a certain number of objects of a specific class. Users can click cell and see the list of all corresponding images.
Class sizes #
The table below gives various size properties of objects for every class. Click a row to see the image with annotations of the selected class. Sort columns to find classes with the smallest or largest objects or understand the size differences between classes.
Class | Object count | Avg area | Max area | Min area | Min height | Min height | Max height | Max height | Avg height | Avg height | Min width | Min width | Max width | Max width |
---|---|---|---|---|---|---|---|---|---|---|---|---|---|---|
flipper any | 42891 | 1.91% | 54.47% | 0% | 2px | 0.1% | 1213px | 91% | 218px | 16.2% | 2px | 0.1% | 1752px | 87.6% |
turtle any | 17945 | 19.92% | 99.77% | 0% | 2px | 0.14% | 1796px | 99.92% | 657px | 48.71% | 2px | 0.1% | 2000px | 100% |
head any | 17101 | 2.31% | 57.64% | 0% | 2px | 0.15% | 1041px | 72.99% | 207px | 15.37% | 2px | 0.1% | 1661px | 83.05% |
Spatial Heatmap #
The heatmaps below give the spatial distributions of all objects for every class. These visualizations provide insights into the most probable and rare object locations on the image. It helps analyze objects' placements in a dataset.
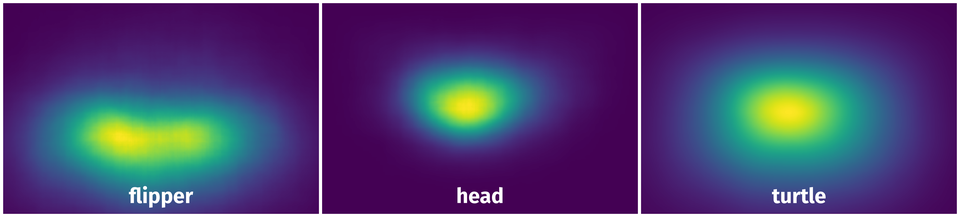
Objects #
Table contains all 77937 objects. Click a row to preview an image with annotations, and use search or pagination to navigate. Sort columns to find outliers in the dataset.
Object ID ㅤ | Class ㅤ | Image name click row to open | Image size height x width | Height ㅤ | Height ㅤ | Width ㅤ | Width ㅤ | Area ㅤ |
---|---|---|---|---|---|---|---|---|
1➔ | turtle any | hLkDzxWNZX.JPG | 1333 x 2000 | 687px | 51.54% | 1240px | 62% | 17.1% |
2➔ | turtle any | hLkDzxWNZX.JPG | 1333 x 2000 | 688px | 51.61% | 1241px | 62.05% | 32.03% |
3➔ | flipper any | hLkDzxWNZX.JPG | 1333 x 2000 | 143px | 10.73% | 94px | 4.7% | 0.25% |
4➔ | flipper any | hLkDzxWNZX.JPG | 1333 x 2000 | 144px | 10.8% | 95px | 4.75% | 0.51% |
5➔ | head any | hLkDzxWNZX.JPG | 1333 x 2000 | 205px | 15.38% | 258px | 12.9% | 1.39% |
6➔ | head any | hLkDzxWNZX.JPG | 1333 x 2000 | 206px | 15.45% | 259px | 12.95% | 2% |
7➔ | flipper any | hLkDzxWNZX.JPG | 1333 x 2000 | 79px | 5.93% | 99px | 4.95% | 0.2% |
8➔ | flipper any | hLkDzxWNZX.JPG | 1333 x 2000 | 80px | 6% | 100px | 5% | 0.3% |
9➔ | flipper any | hLkDzxWNZX.JPG | 1333 x 2000 | 358px | 26.86% | 329px | 16.45% | 3.42% |
10➔ | flipper any | hLkDzxWNZX.JPG | 1333 x 2000 | 359px | 26.93% | 330px | 16.5% | 4.44% |
License #
ADD CUSTOM LICENSE MANUALLY
Citation #
If you make use of the Sea Turtle 2022 data, please cite the following reference:
@InProceedings{Adam_2024_WACV,
author = {Adam, Luk\'a\v{s} and \v{C}erm\'ak, Vojt\v{e}ch and Papafitsoros, Kostas and Picek, Lukas},
title = {SeaTurtleID2022: A Long-Span Dataset for Reliable Sea Turtle Re-Identification},
booktitle = {Proceedings of the IEEE/CVF Winter Conference on Applications of Computer Vision (WACV)},
month = {January},
year = {2024},
pages = {7146-7156}
}
If you are happy with Dataset Ninja and use provided visualizations and tools in your work, please cite us:
@misc{ visualization-tools-for-sea-turtle-dataset,
title = { Visualization Tools for Sea Turtle 2022 Dataset },
type = { Computer Vision Tools },
author = { Dataset Ninja },
howpublished = { \url{ https://datasetninja.com/sea-turtle-2022 } },
url = { https://datasetninja.com/sea-turtle-2022 },
journal = { Dataset Ninja },
publisher = { Dataset Ninja },
year = { 2024 },
month = { sep },
note = { visited on 2024-09-08 },
}
Download #
Please visit dataset homepage to download the data.
Disclaimer #
Our gal from the legal dep told us we need to post this:
Dataset Ninja provides visualizations and statistics for some datasets that can be found online and can be downloaded by general audience. Dataset Ninja is not a dataset hosting platform and can only be used for informational purposes. The platform does not claim any rights for the original content, including images, videos, annotations and descriptions. Joint publishing is prohibited.
You take full responsibility when you use datasets presented at Dataset Ninja, as well as other information, including visualizations and statistics we provide. You are in charge of compliance with any dataset license and all other permissions. You are required to navigate datasets homepage and make sure that you can use it. In case of any questions, get in touch with us at hello@datasetninja.com.