Introduction #
To address the challenges of training neural networks for automated diagnosis of pigmented skin lesions, the authors introduced the HAM10000 (“Human Against Machine with 10000 training images”) dataset. This dataset aimed to overcome the limitations of small-sized and homogeneous dermatoscopic image datasets by providing a diverse and extensive collection. To achieve this, they collected dermatoscopic images from various populations using different modalities, which necessitated employing distinct acquisition and cleaning methods. The authors also designed semi-automatic workflows that incorporated specialized neural networks to enhance the dataset’s quality. The resulting HAM10000 dataset comprised 10,015 dermatoscopic images, which were made available for academic machine learning applications through the ISIC archive. This dataset served as a benchmark for machine learning experiments and comparisons with human experts.
Dermatoscopy, a diagnostic technique for pigmented skin lesions, offers improved diagnostic accuracy compared to unaided visual examination. The authors noted that dermatoscopic images could be employed to train artificial neural networks for automated diagnosis. While previous attempts had shown promise, their effectiveness was limited by small sample sizes and a lack of diversity in dermatoscopic images. Recent advancements in machine learning and graphics card capabilities indicated the potential for comprehensive automated diagnostic systems, capable of diagnosing various pigmented skin lesions without human intervention.
Historically, available datasets were primarily focused on melanocytic lesions and disregarded non-melanocytic pigmented lesions, despite their prevalence in clinical practice. This discrepancy between training data and real-world scenarios contributed to moderate performance in clinical settings despite favorable results in controlled experiments. The authors recognized the need for a dataset that encompassed a broader range of lesions, leading to the release of the HAM10000 dataset. While initially developed for the ISIC 2018 classification challenge, this dataset was also made accessible to other research groups for further exploration.
The HAM10000 dataset contained 10,015 dermatoscopic images collected over two decades from diverse sources, including the Department of Dermatology at the Medical University of Vienna, Austria, and a skin cancer practice in Queensland, Australia. The authors highlighted their use of various formats to store images and metadata, given the evolution of image storage technologies over time. For instance, images from the Vienna site were initially stored as diapositives and later digitized.
To ensure dataset quality, the authors introduced an automated method to categorize images based on image type. This approach utilized a trained InceptionV3 neural network to classify images as overviews, close-ups, or dermatoscopy images. This method significantly expedited the process of selecting dermatoscopic images for the dataset. The authors also unified histopathologic diagnoses to form seven generic classes that covered the majority of pigmented lesions encountered in clinical practice.
Finally, a manual quality review was conducted to eliminate cases that didn’t meet predefined criteria. This step involved filtering out close-up and overview images, removing images with identifiable content, and ensuring image quality and content alignment with the dataset’s purpose.
Note, that presented dataset is a modification of the original HAM10000 dataset.
Summary #
Skin Cancer: HAM10000 is a dataset for a semantic segmentation task. It is used in the medical research. The dataset presented here is not the original one. Learn more on the dataset’s homepage.
The dataset consists of 10015 images with 10013 labeled objects belonging to 7 different classes including melanocytic nevi, melanoma, benign keratosis-like lesions, and other: basal cell carcinoma, actinic keratoses, vascular lesions, and dermatofibroma.
Images in the Skin Cancer: HAM10000 dataset have pixel-level semantic segmentation annotations. There are 2 (0% of the total) unlabeled images (i.e. without annotations). There are no pre-defined train/val/test splits in the dataset. The dataset was released in 2021 by the ViDIR Group in Medical University of Vienna, Austria and University of Queensland, Austria.
Here are the visualized examples for the classes:
Explore #
Skin Cancer: HAM10000 dataset has 10015 images. Click on one of the examples below or open "Explore" tool anytime you need to view dataset images with annotations. This tool has extended visualization capabilities like zoom, translation, objects table, custom filters and more. Hover the mouse over the images to hide or show annotations.
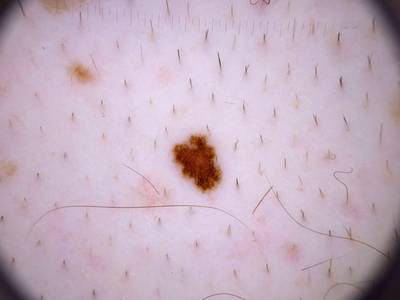

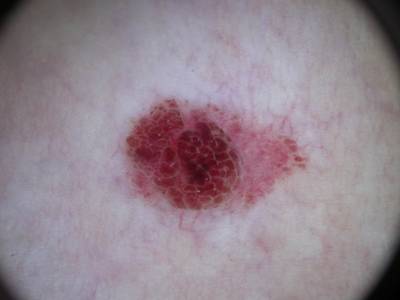


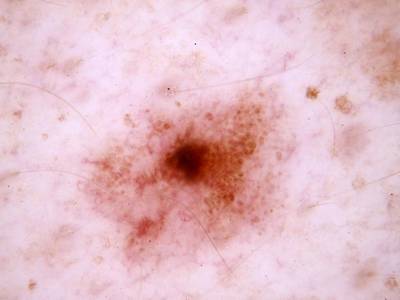
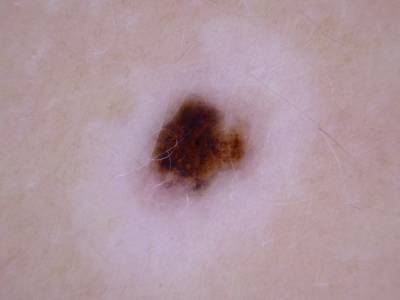

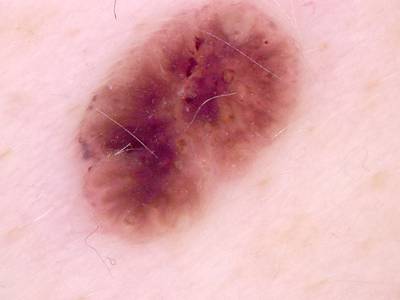

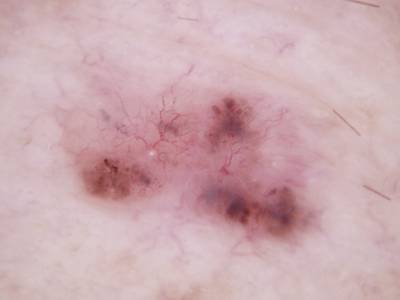
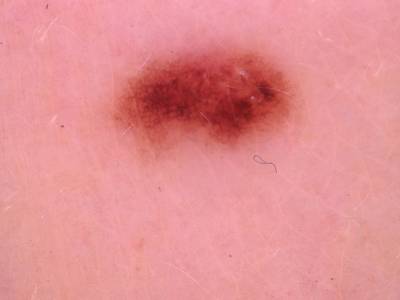

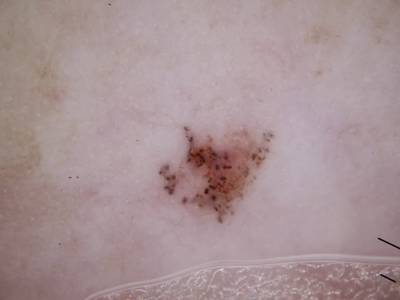
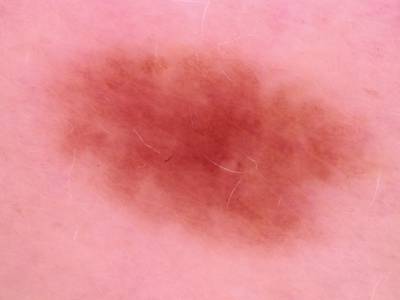
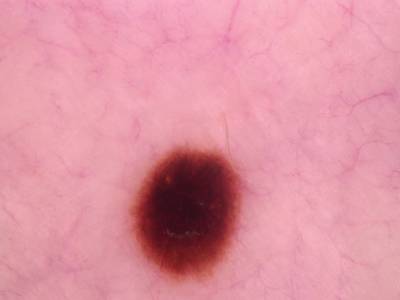
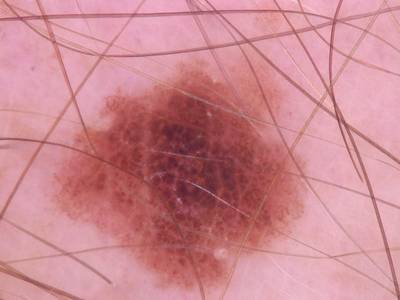
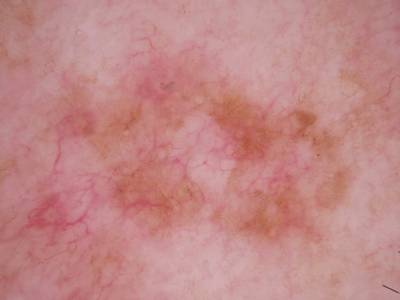
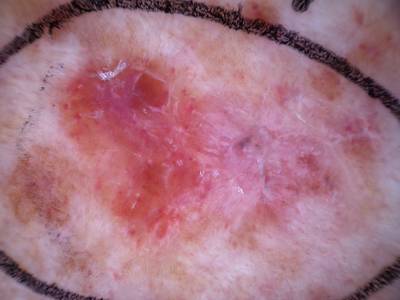
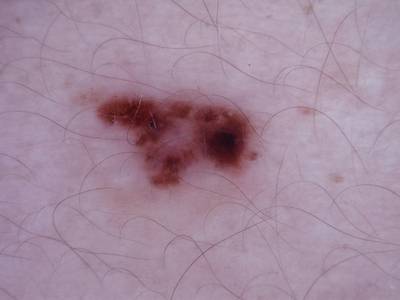
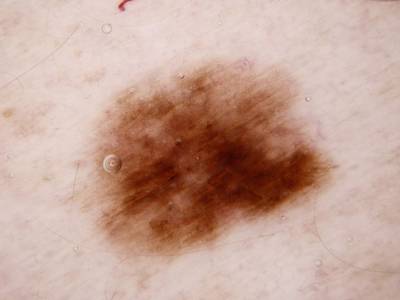
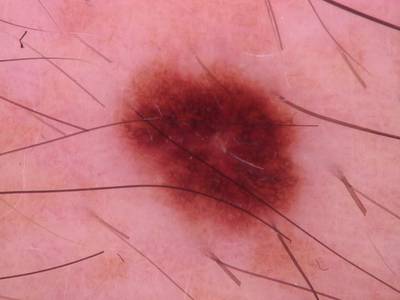
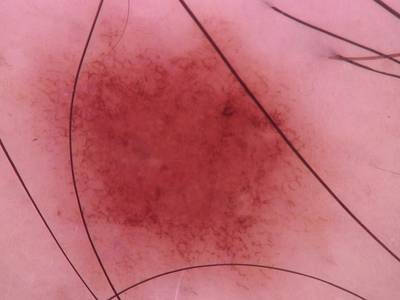
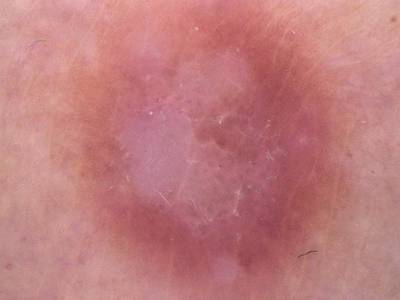
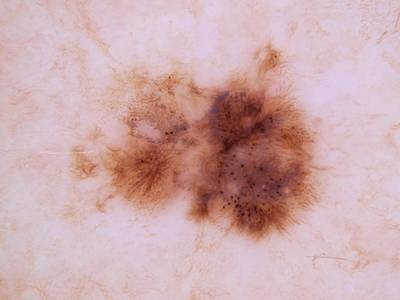
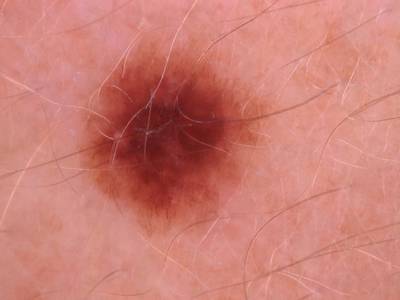
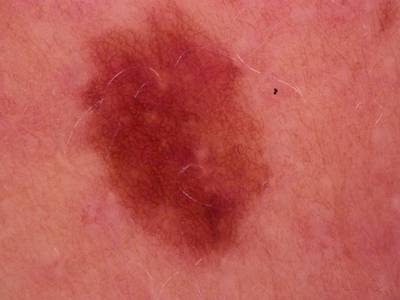
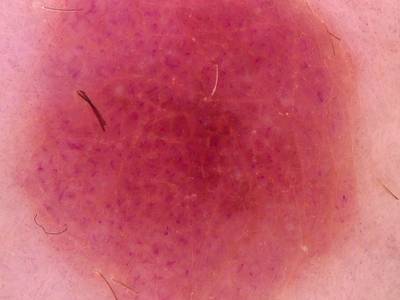
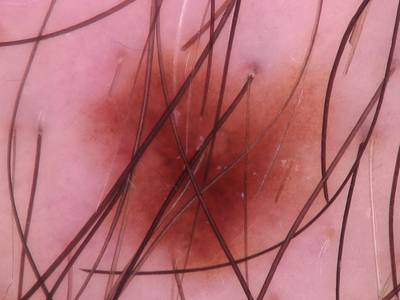
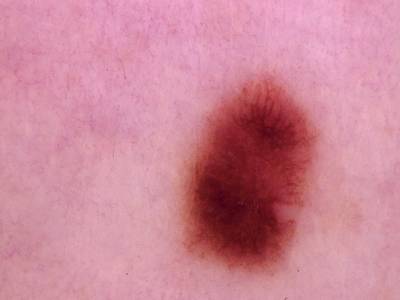
Class balance #
There are 7 annotation classes in the dataset. Find the general statistics and balances for every class in the table below. Click any row to preview images that have labels of the selected class. Sort by column to find the most rare or prevalent classes.
Class ㅤ | Images ㅤ | Objects ㅤ | Count on image average | Area on image average |
---|---|---|---|---|
melanocytic nevi➔ mask | 6705 | 6705 | 1 | 24.34% |
melanoma➔ mask | 1112 | 1112 | 1 | 32.38% |
benign keratosis-like lesions➔ mask | 1098 | 1098 | 1 | 31.9% |
basal cell carcinoma➔ mask | 514 | 514 | 1 | 26.01% |
actinic keratoses➔ mask | 327 | 327 | 1 | 40.3% |
vascular lesions➔ mask | 142 | 142 | 1 | 15.2% |
dermatofibroma➔ mask | 115 | 115 | 1 | 31.19% |
Co-occurrence matrix #
Co-occurrence matrix is an extremely valuable tool that shows you the images for every pair of classes: how many images have objects of both classes at the same time. If you click any cell, you will see those images. We added the tooltip with an explanation for every cell for your convenience, just hover the mouse over a cell to preview the description.
Images #
Explore every single image in the dataset with respect to the number of annotations of each class it has. Click a row to preview selected image. Sort by any column to find anomalies and edge cases. Use horizontal scroll if the table has many columns for a large number of classes in the dataset.
Object distribution #
Interactive heatmap chart for every class with object distribution shows how many images are in the dataset with a certain number of objects of a specific class. Users can click cell and see the list of all corresponding images.
Class sizes #
The table below gives various size properties of objects for every class. Click a row to see the image with annotations of the selected class. Sort columns to find classes with the smallest or largest objects or understand the size differences between classes.
Class | Object count | Avg area | Max area | Min area | Min height | Min height | Max height | Max height | Avg height | Avg height | Min width | Min width | Max width | Max width |
---|---|---|---|---|---|---|---|---|---|---|---|---|---|---|
melanocytic nevi mask | 6705 | 24.34% | 99.15% | 0.39% | 35px | 7.78% | 450px | 100% | 268px | 59.58% | 37px | 6.17% | 600px | 100% |
melanoma mask | 1112 | 32.38% | 98.86% | 0.56% | 48px | 10.67% | 450px | 100% | 307px | 68.17% | 40px | 6.67% | 600px | 100% |
benign keratosis-like lesions mask | 1098 | 31.9% | 93.99% | 0.85% | 57px | 12.67% | 450px | 100% | 307px | 68.16% | 50px | 8.33% | 600px | 100% |
basal cell carcinoma mask | 514 | 26.01% | 93.61% | 0.58% | 39px | 8.67% | 450px | 100% | 271px | 60.29% | 51px | 8.5% | 600px | 100% |
actinic keratoses mask | 327 | 40.3% | 97.04% | 2.18% | 88px | 19.56% | 450px | 100% | 344px | 76.35% | 83px | 13.83% | 600px | 100% |
vascular lesions mask | 142 | 15.2% | 90.2% | 0.96% | 54px | 12% | 450px | 100% | 202px | 45% | 62px | 10.33% | 584px | 97.33% |
dermatofibroma mask | 115 | 31.19% | 89.98% | 2.67% | 89px | 19.78% | 450px | 100% | 299px | 66.37% | 77px | 12.83% | 600px | 100% |
Spatial Heatmap #
The heatmaps below give the spatial distributions of all objects for every class. These visualizations provide insights into the most probable and rare object locations on the image. It helps analyze objects' placements in a dataset.
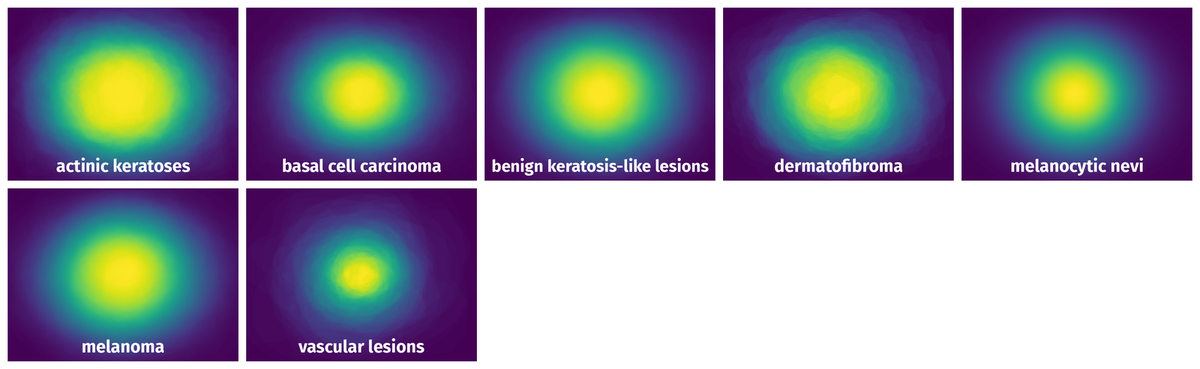
Objects #
Table contains all 10013 objects. Click a row to preview an image with annotations, and use search or pagination to navigate. Sort columns to find outliers in the dataset.
Object ID ㅤ | Class ㅤ | Image name click row to open | Image size height x width | Height ㅤ | Height ㅤ | Width ㅤ | Width ㅤ | Area ㅤ |
---|---|---|---|---|---|---|---|---|
1➔ | melanocytic nevi mask | ISIC_0027917.jpg | 450 x 600 | 266px | 59.11% | 398px | 66.33% | 29.17% |
2➔ | melanocytic nevi mask | ISIC_0025340.jpg | 450 x 600 | 229px | 50.89% | 198px | 33% | 11.45% |
3➔ | melanoma mask | ISIC_0033310.jpg | 450 x 600 | 435px | 96.67% | 474px | 79% | 61.52% |
4➔ | melanocytic nevi mask | ISIC_0028266.jpg | 450 x 600 | 219px | 48.67% | 158px | 26.33% | 9.86% |
5➔ | melanocytic nevi mask | ISIC_0029047.jpg | 450 x 600 | 387px | 86% | 288px | 48% | 30.25% |
6➔ | melanocytic nevi mask | ISIC_0024954.jpg | 450 x 600 | 408px | 90.67% | 571px | 95.17% | 59.09% |
7➔ | melanocytic nevi mask | ISIC_0027942.jpg | 450 x 600 | 400px | 88.89% | 288px | 48% | 31.18% |
8➔ | melanocytic nevi mask | ISIC_0026195.jpg | 450 x 600 | 408px | 90.67% | 406px | 67.67% | 46.05% |
9➔ | melanocytic nevi mask | ISIC_0030164.jpg | 450 x 600 | 248px | 55.11% | 251px | 41.83% | 16.45% |
10➔ | melanocytic nevi mask | ISIC_0025288.jpg | 450 x 600 | 376px | 83.56% | 443px | 73.83% | 43.34% |
License #
Skin Cancer: HAM10000 is under CC BY-NC 4.0 license.
Citation #
If you make use of the Skin Cancer (HAM10000) data, please cite the following reference:
@article{Tschandl2018_HAM10000,
author = {Philipp Tschandl and
Cliff Rosendahl and
Harald Kittler},
title = {The {HAM10000} dataset, a large collection of multi-source dermatoscopic
images of common pigmented skin lesions},
journal = {Sci. Data},
volume = {5},
year = {2018},
pages = {180161},
doi = {10.1038/sdata.2018.161}
}
@article{Tschandl2020_NatureMedicine,
author = {Philipp Tschandl and Christoph Rinner and Zoe Apalla and Giuseppe Argenziano and Noel Codella and Allan Halpern and Monika Janda and Aimilios Lallas and Caterina Longo and Josep Malvehy and John Paoli and Susana Puig and Cliff Rosendahl and H. Peter Soyer and Iris Zalaudek and Harald Kittler},
title = {Human{\textendash}computer collaboration for skin cancer recognition},
journal = {Nature Medicine},
volume = {26},
number = {8},
year = {2020},
pages = {1229--1234},
doi = {10.1038/s41591-020-0942-0},
url = {https://doi.org/10.1038/s41591-020-0942-0}
}
If you are happy with Dataset Ninja and use provided visualizations and tools in your work, please cite us:
@misc{ visualization-tools-for-skin-cancer-ham10000-dataset,
title = { Visualization Tools for Skin Cancer: HAM10000 Dataset },
type = { Computer Vision Tools },
author = { Dataset Ninja },
howpublished = { \url{ https://datasetninja.com/skin-cancer-ham10000 } },
url = { https://datasetninja.com/skin-cancer-ham10000 },
journal = { Dataset Ninja },
publisher = { Dataset Ninja },
year = { 2024 },
month = { nov },
note = { visited on 2024-11-21 },
}
Download #
Dataset Skin Cancer: HAM10000 can be downloaded in Supervisely format:
As an alternative, it can be downloaded with dataset-tools package:
pip install --upgrade dataset-tools
… using following python code:
import dataset_tools as dtools
dtools.download(dataset='Skin Cancer: HAM10000', dst_dir='~/dataset-ninja/')
Make sure not to overlook the python code example available on the Supervisely Developer Portal. It will give you a clear idea of how to effortlessly work with the downloaded dataset.
The data in original format can be downloaded here.
Disclaimer #
Our gal from the legal dep told us we need to post this:
Dataset Ninja provides visualizations and statistics for some datasets that can be found online and can be downloaded by general audience. Dataset Ninja is not a dataset hosting platform and can only be used for informational purposes. The platform does not claim any rights for the original content, including images, videos, annotations and descriptions. Joint publishing is prohibited.
You take full responsibility when you use datasets presented at Dataset Ninja, as well as other information, including visualizations and statistics we provide. You are in charge of compliance with any dataset license and all other permissions. You are required to navigate datasets homepage and make sure that you can use it. In case of any questions, get in touch with us at hello@datasetninja.com.