Introduction #
The authors presented a new large scale multi-object tracking dataset in diverse sports scenes, coined as SportsMOT Dataset, where all players on the court are supposed to be tracked. It consists of 240 video sequences, over 150K frames and over 1.6M bounding boxes collected from 3 sports categories, including basketball, volleyball and football.
Motivation
Multi-object tracking (MOT) stands as a cornerstone in computer vision tasks over recent decades, dedicated to pinpointing and associating objects within video sequences. Considerable attention from researchers has been directed towards diverse practical scenarios, including crowded street scenes, static dancing scenes, and driving scenarios, leading to significant advancements in MOT methodologies. However, the realm of sports scenes has often been neglected in this domain. In sports contexts, the focus typically centers on tracking players on the field for subsequent analysis, such as quantifying running distances, determining average speeds, and enabling automatic tactical analyses. Contemporary state-of-the-art trackers typically comprise various components to fulfill the tracking objective, encompassing object localization modules, motion-based object association modules, and appearance-based association modules. Nevertheless, these components encounter challenges when applied to sports scenes due to biases in the data distributions of prevalent human tracking benchmarks, such as MOT17, MOT20, and DanceTrack. These challenges stem from the fact that existing benchmarks, driven primarily by surveillance or self-driving applications, provide tracks for almost all individuals within scenes. However, in sports contexts like basketball or football games, the focus is primarily on tracking players on the court. Thus, specialized training platforms are necessary to adapt detectors to sports scenes adequately. Moreover, prevailing trackers highlighted in MOT17 and MOT20 emphasize Kalman Filter-based Intersection over Union (IoU) matching for object association, tailored to the slow and regular motion patterns observed in pedestrians. In contrast, DanceTrack emphasizes diverse motion patterns over fast movements, reflecting the frequent directional changes and relative position shifts observed in dancers. However, sports scenes present unique challenges characterized by fast and variable-speed movements of objects across adjacent frames. Players in professional sports events typically exhibit high speeds and frequently adjust their running speeds, presenting significant hurdles for existing motion-based association methodologies.
IoU on adjacent frames | Kalman-Filter-based IoU on adjacent frames. |
---|---|
Consequently, there is a pressing need for motion-based association methods that are better suited to sports scenes. Furthermore, in contrast to the MOT17 and MOT20 datasets, which primarily feature street scenes, objects in sports scenes often exhibit less distinguishable appearances. This is due to several factors, including players wearing similar attire and frequent blurring caused by rapid camera or target motion. Unlike DanceTrack, where dancers typically wear similar clothing, players in sports scenes usually don jerseys with distinct numbers and adopt varying postures. Consequently, they contend that while objects in sports scenes share similar appearances, they possess enough distinguishable features to warrant the development of appearance models capable of generating more discriminative and comprehensive representations.
Dataset description
Based on the analysis presented earlier, the authors propose the creation of a multi-object tracking dataset tailored specifically for sports scenes, termed as the SportsMOT. This dataset is characterized by its large-scale, high-quality nature and comprehensive annotations, covering every player on the court across various sports scenarios. SportsMOT comprises 240 videos, totaling over 150,000 frames and containing more than 1.6 million bounding boxes. These annotations span three categories of sports: basketball, volleyball, and football. To facilitate the adaptation of trackers to sports scenes, the authors have divided the dataset into training, validation, and test subsets, encompassing 45, 45, and 150 video sequences, respectively. SportsMOT is distinguished by two fundamental properties:
- It features fast and variable-speed motion, necessitating the development of motion modeling associations capable of accommodating such dynamics.
- Despite similarities in appearance among players, there are enough distinguishing features to warrant the creation of appearance models capable of generating more discriminative and extensive representations.
Video collection
The authors have curated videos from three globally renowned sports football, basketball, and volleyball selecting high-quality footage from professional games such as those from the NCAA, Premier League, and Olympics. These videos were sourced from MultiSports, a comprehensive dataset focusing on spatio-temporal action localization within the realm of sports. Each sport category encompasses typical player formations and motion patterns, effectively capturing the diversity of sports scenarios. Only overhead shots from the sports game scenes were utilized, ensuring the exclusion of potentially extreme viewpoints. The resultant dataset comprises a total of 240 video sequences, each boasting 720p resolution and a frame rate of 25 FPS (frames per second). Adhering to the principles of multi-object tracking, every video clip underwent manual scrutiny to ensure the absence of abrupt viewpoint changes throughout the footage.
Annotation pipeline
The authors annotate the collected video saccording to the following guidelines:
- The entire athlete’s limbs and torso, excluding any other objects like balls touching the athlete’s body, are required to be annotated.
- The annotators are asked to predict the bounding box of the athlete in the case of occlusion, as long as the athletes have a visible part of body. However, if half of the athletes’ torso is outside the view, annotators should just skip them.
- The authors ask the annotators to confirm that each player has a unique ID throughout the whole clip.
They developed a bespoke labeling tool specifically designed for SportsMOT, accompanied by a comprehensive manual for annotators. Upon initiating the annotation process for a new object, the labeling tool automatically assigns a unique identifier (ID) to the object and propagates the bounding box from the previous state to the current state, leveraging the capabilities of the single object tracker KCF. Subsequently, annotators refine the generated bounding boxes to enhance the overall annotation quality. Following a meticulous review of each annotation result, the authors meticulously refine any bounding boxes and person id that fail to meet the prescribed standards, thereby ensuring the creation of a high-quality dataset. Finally, bounding boxes deemed excessively small, where either the width (w) or height (h) falls below 5 pixels, are removed from consideration.
Category | Frames | Tracks | Track Gap len. | Track len. per frame | Track Bboxes |
---|---|---|---|---|---|
Basketball | 845.4 | 10 | 68.7 | 767.9 | 9.1 |
Volleyball | 360.4 | 12 | 38.2 | 335.9 | 11.2 |
Football | 673.9 | 20.5 | 116.1 | 422.1 | 12.8 |
Total | 626.6 | 14.2 | 96.6 | 479.1 | 10.8 |
Detailed statistics of the three categories in SportsMOT.
Summary #
SportsMOT Dataset is a dataset for object detection and identification tasks. It is used in the sports industry.
The dataset consists of 150379 images with 608152 labeled objects belonging to 1 single class (person).
Images in the SportsMOT dataset have bounding box annotations. There are 94835 (63% of the total) unlabeled images (i.e. without annotations). There are 3 splits in the dataset: test (94835 images), train (28574 images), and val (26970 images). Alternatively, the dataset could be split into 3 kinds of sports: basketball (67635 images), football (53913 images), and volleyball (28831 images). Additionally, every image marked with its sequence tag. Every label contains information about its person id. Explore it in Supervisely labeling tool. The dataset was released in 2023 by the Nanjing University, China.
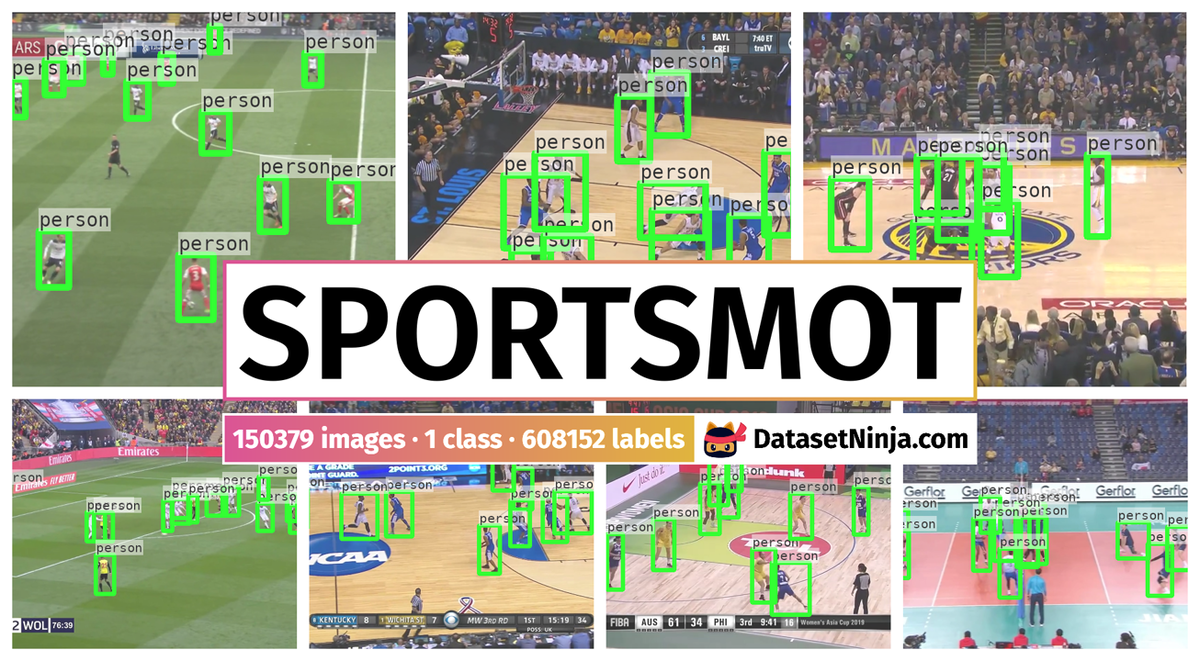
Explore #
SportsMOT dataset has 150379 images. Click on one of the examples below or open "Explore" tool anytime you need to view dataset images with annotations. This tool has extended visualization capabilities like zoom, translation, objects table, custom filters and more. Hover the mouse over the images to hide or show annotations.
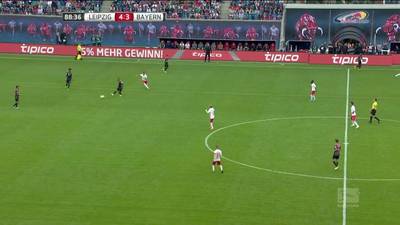
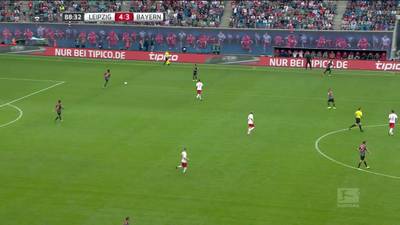
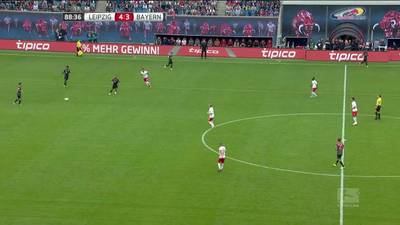
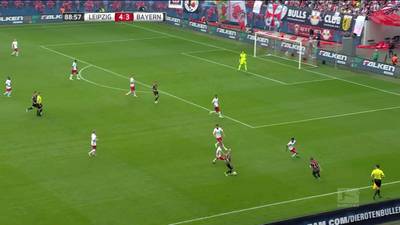


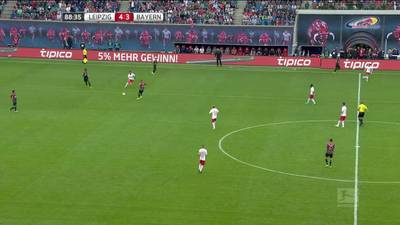
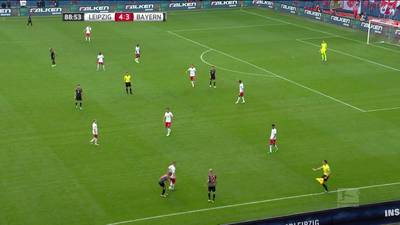
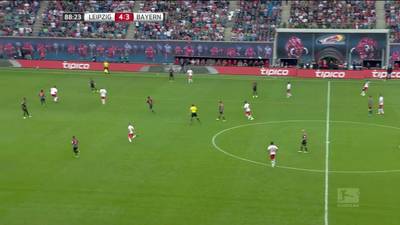
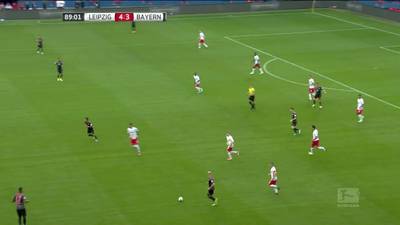

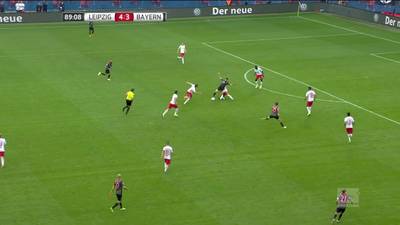
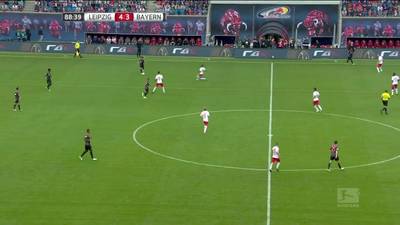
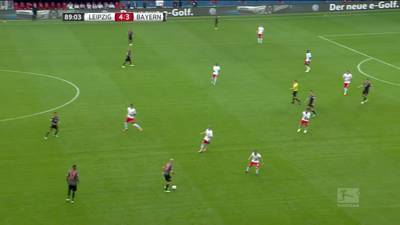
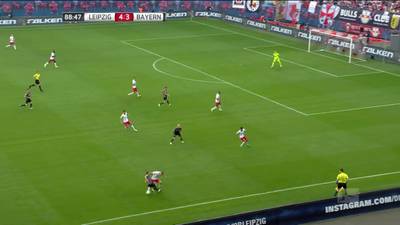
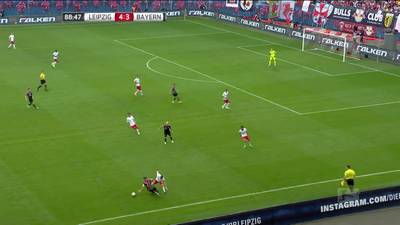
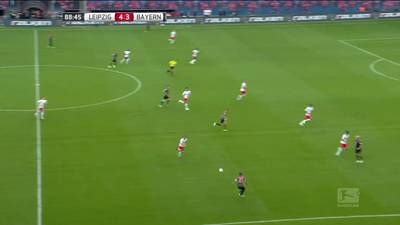
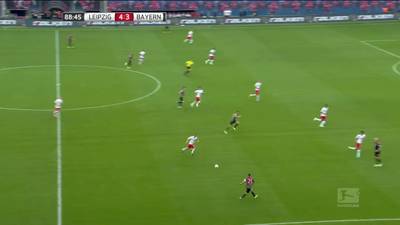
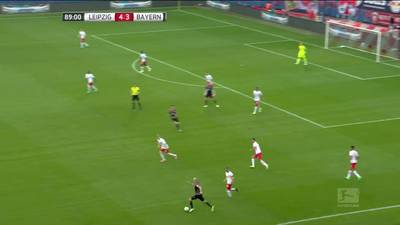

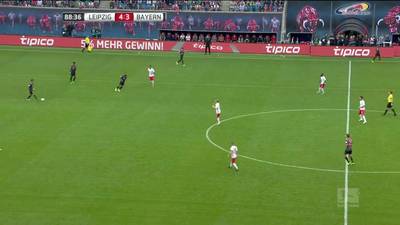
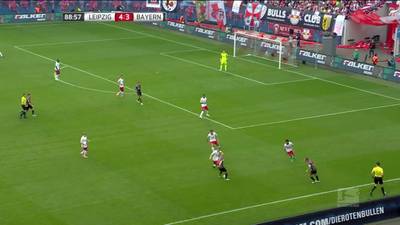
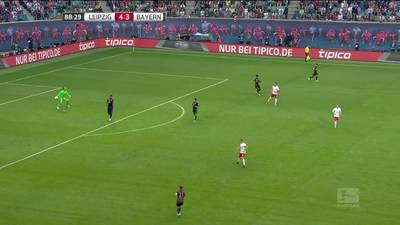
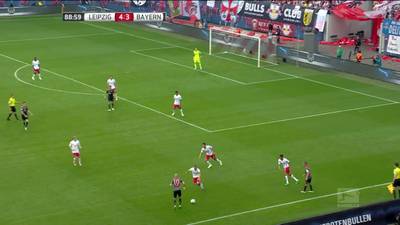
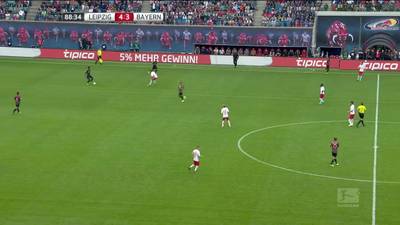
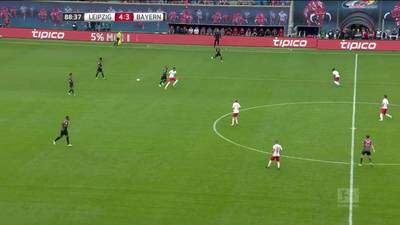
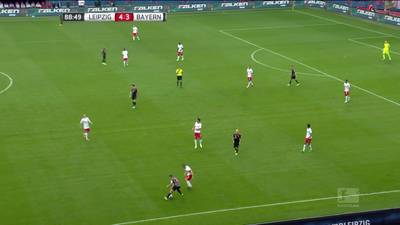

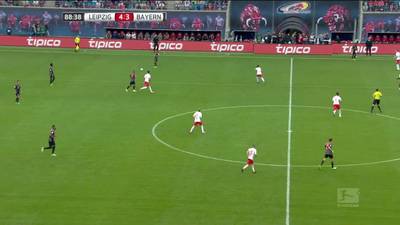
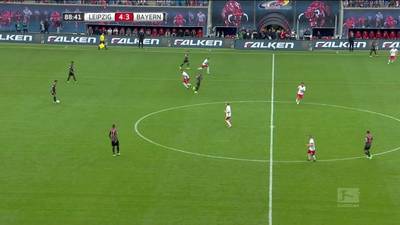
Class balance #
There are 1 annotation classes in the dataset. Find the general statistics and balances for every class in the table below. Click any row to preview images that have labels of the selected class. Sort by column to find the most rare or prevalent classes.
Class ã…¤ | Images ã…¤ | Objects ã…¤ | Count on image average | Area on image average |
---|---|---|---|---|
personâž” rectangle | 55544 | 608152 | 10.95 | 8.37% |
Images #
Explore every single image in the dataset with respect to the number of annotations of each class it has. Click a row to preview selected image. Sort by any column to find anomalies and edge cases. Use horizontal scroll if the table has many columns for a large number of classes in the dataset.
Object distribution #
Interactive heatmap chart for every class with object distribution shows how many images are in the dataset with a certain number of objects of a specific class. Users can click cell and see the list of all corresponding images.
Class sizes #
The table below gives various size properties of objects for every class. Click a row to see the image with annotations of the selected class. Sort columns to find classes with the smallest or largest objects or understand the size differences between classes.
Class | Object count | Avg area | Max area | Min area | Min height | Min height | Max height | Max height | Avg height | Avg height | Min width | Min width | Max width | Max width |
---|---|---|---|---|---|---|---|---|---|---|---|---|---|---|
person rectangle | 608152 | 0.89% | 7.91% | 0.02% | 5px | 0.69% | 399px | 55.42% | 120px | 16.71% | 5px | 0.39% | 281px | 21.95% |
Spatial Heatmap #
The heatmaps below give the spatial distributions of all objects for every class. These visualizations provide insights into the most probable and rare object locations on the image. It helps analyze objects' placements in a dataset.
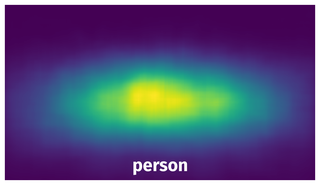
Objects #
Table contains all 99138 objects. Click a row to preview an image with annotations, and use search or pagination to navigate. Sort columns to find outliers in the dataset.
Object ID ã…¤ | Class ã…¤ | Image name click row to open | Image size height x width | Height ã…¤ | Height ã…¤ | Width ã…¤ | Width ã…¤ | Area ã…¤ |
---|---|---|---|---|---|---|---|---|
1âž” | person rectangle | v_2j7kLB-vEEk_c009_000323.jpg | 720 x 1280 | 152px | 21.11% | 95px | 7.42% | 1.57% |
2âž” | person rectangle | v_2j7kLB-vEEk_c009_000323.jpg | 720 x 1280 | 169px | 23.47% | 109px | 8.52% | 2% |
3âž” | person rectangle | v_2j7kLB-vEEk_c009_000323.jpg | 720 x 1280 | 198px | 27.5% | 167px | 13.05% | 3.59% |
4âž” | person rectangle | v_2j7kLB-vEEk_c009_000323.jpg | 720 x 1280 | 194px | 26.94% | 77px | 6.02% | 1.62% |
5âž” | person rectangle | v_2j7kLB-vEEk_c009_000323.jpg | 720 x 1280 | 138px | 19.17% | 72px | 5.62% | 1.08% |
6âž” | person rectangle | v_2j7kLB-vEEk_c009_000323.jpg | 720 x 1280 | 192px | 26.67% | 78px | 6.09% | 1.62% |
7âž” | person rectangle | v_2j7kLB-vEEk_c009_000323.jpg | 720 x 1280 | 215px | 29.86% | 117px | 9.14% | 2.73% |
8âž” | person rectangle | v_2j7kLB-vEEk_c009_000323.jpg | 720 x 1280 | 187px | 25.97% | 67px | 5.23% | 1.36% |
9âž” | person rectangle | v_2j7kLB-vEEk_c009_000323.jpg | 720 x 1280 | 197px | 27.36% | 88px | 6.88% | 1.88% |
10âž” | person rectangle | v_2j7kLB-vEEk_c009_000323.jpg | 720 x 1280 | 173px | 24.03% | 68px | 5.31% | 1.28% |
License #
SportsMOT Dataset is under CC BY-NC 4.0 license.
Citation #
If you make use of the SportsMOT data, please cite the following reference:
@article{cui2023sportsmot,
title={SportsMOT: A Large Multi-Object Tracking Dataset in Multiple Sports Scenes},
author={Cui, Yutao and Zeng, Chenkai and Zhao, Xiaoyu and Yang, Yichun and Wu, Gangshan and Wang, Limin},
journal={arXiv preprint arXiv:2304.05170},
year={2023}
}
If you are happy with Dataset Ninja and use provided visualizations and tools in your work, please cite us:
@misc{ visualization-tools-for-sports-mot-dataset,
title = { Visualization Tools for SportsMOT Dataset },
type = { Computer Vision Tools },
author = { Dataset Ninja },
howpublished = { \url{ https://datasetninja.com/sports-mot } },
url = { https://datasetninja.com/sports-mot },
journal = { Dataset Ninja },
publisher = { Dataset Ninja },
year = { 2025 },
month = { mar },
note = { visited on 2025-03-26 },
}
Download #
Dataset SportsMOT can be downloaded in Supervisely format:
As an alternative, it can be downloaded with dataset-tools package:
pip install --upgrade dataset-tools
… using following python code:
import dataset_tools as dtools
dtools.download(dataset='SportsMOT', dst_dir='~/dataset-ninja/')
Make sure not to overlook the python code example available on the Supervisely Developer Portal. It will give you a clear idea of how to effortlessly work with the downloaded dataset.
The data in original format can be downloaded here.
Disclaimer #
Our gal from the legal dep told us we need to post this:
Dataset Ninja provides visualizations and statistics for some datasets that can be found online and can be downloaded by general audience. Dataset Ninja is not a dataset hosting platform and can only be used for informational purposes. The platform does not claim any rights for the original content, including images, videos, annotations and descriptions. Joint publishing is prohibited.
You take full responsibility when you use datasets presented at Dataset Ninja, as well as other information, including visualizations and statistics we provide. You are in charge of compliance with any dataset license and all other permissions. You are required to navigate datasets homepage and make sure that you can use it. In case of any questions, get in touch with us at hello@datasetninja.com.