Introduction #
StrawDI: The Strawberry Digital Images Data Set contains 8000 images of strawberries, taken from 20 plantations, within an approximate area of 150 hectares, in the province of Huelva, Spain. Later, it was rescaled to the StrawDI_Db1 database. The plantations were not changed in any way for the experiment and the images were taken from real production conditions during a full picking campaign (from mid-December 2018 to early May 2019).
The capture device used was a Samsung Galaxy S7 Edge smartphone attached to an extendable arm. In order to build a data set close to the target application, the images were taken under different conditions of brightness, at a distance of approximately 20 cm from the ridge, at about 35 ±10 cm height, and at an approximate angle of 25 ±10º. The images have a 4032x3024 resolution and are stored in a JPEG format.
From the total set of images that make up the StrawDI data set, 3100 images have been selected at random. For each of these images, a ground truth labeled image has been manually generated containing the individual segmentation of all the strawberries appearing in the image.
To reduce the computational demands on the models, the images have been rescaled to 1008 x 756 and stored in PNG format. This set of images constitutes the StrawDI_Db1 database. This database is divided into train (2800 images), validation (100 images), and test (200 images) subsets.
The image labeling process has been validated by three blind reviews of the data with four reviewers, thus guaranteeing the quality of the segmentation. The criterion followed in the labeling process consists of creating as accurate a mask as possible for each of the strawberries appearing in the image, including extreme cases such as strawberries that are almost imperceptible due to distance, occlusions, being at the edges of the image or still unripe.
Summary #
StrawDI_Db1: The Strawberry Digital Images Data Set is a dataset for instance segmentation, semantic segmentation, and object detection tasks. It is used in the agricultural industry.
The dataset consists of 3100 images with 17938 labeled objects belonging to 1 single class (strawberry).
Images in the StrawDI_Db1 dataset have pixel-level instance segmentation annotations. Due to the nature of the instance segmentation task, it can be automatically transformed into a semantic segmentation (only one mask for every class) or object detection (bounding boxes for every object) tasks. All images are labeled (i.e. with annotations). There are 3 splits in the dataset: train (2800 images), test (200 images), and val (100 images). The dataset was released in 2020 by the University of Huelva, Spain.
Here is the visualized example grid with animated annotations:
Explore #
StrawDI_Db1 dataset has 3100 images. Click on one of the examples below or open "Explore" tool anytime you need to view dataset images with annotations. This tool has extended visualization capabilities like zoom, translation, objects table, custom filters and more. Hover the mouse over the images to hide or show annotations.
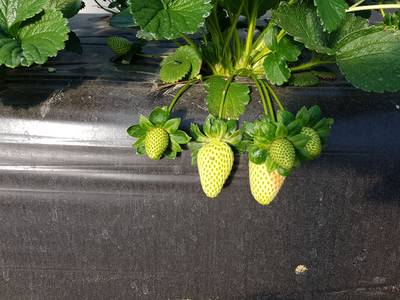
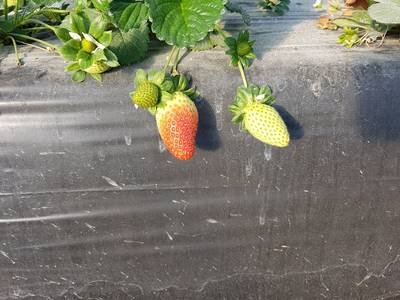
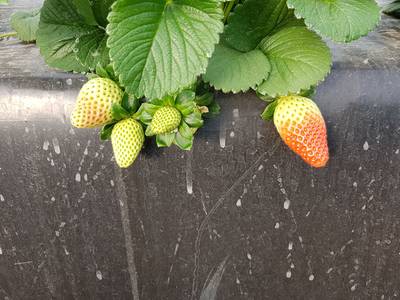

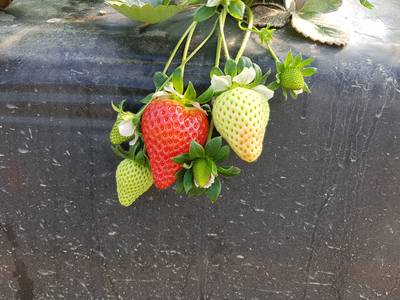
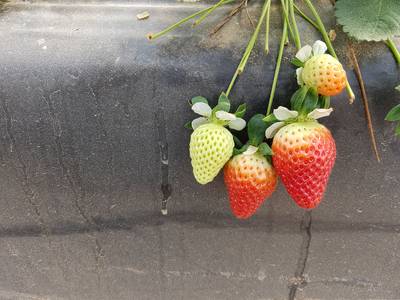
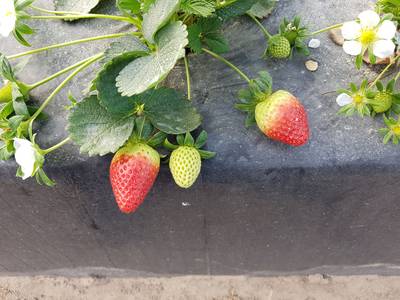
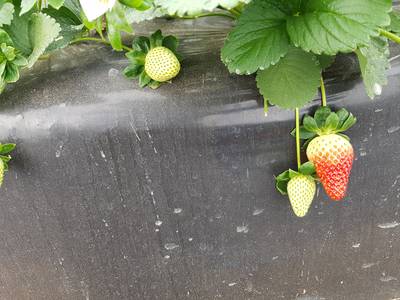
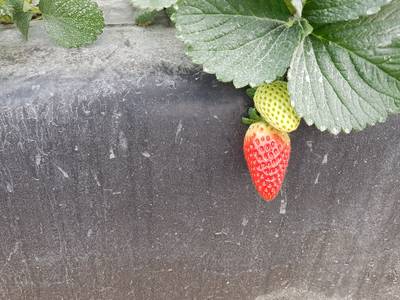
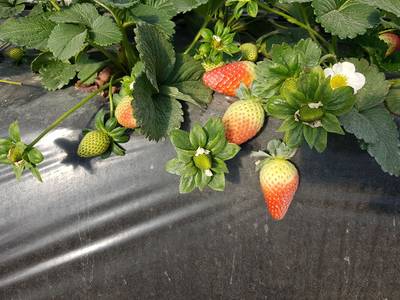
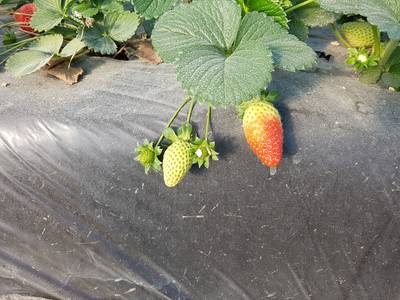
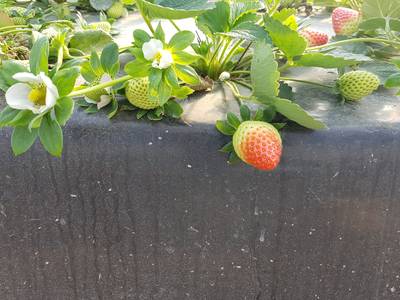
Class balance #
There are 1 annotation classes in the dataset. Find the general statistics and balances for every class in the table below. Click any row to preview images that have labels of the selected class. Sort by column to find the most rare or prevalent classes.
Class ㅤ | Images ㅤ | Objects ㅤ | Count on image average | Area on image average |
---|---|---|---|---|
strawberry➔ mask | 3100 | 17938 | 5.79 | 5.72% |
Images #
Explore every single image in the dataset with respect to the number of annotations of each class it has. Click a row to preview selected image. Sort by any column to find anomalies and edge cases. Use horizontal scroll if the table has many columns for a large number of classes in the dataset.
Object distribution #
Interactive heatmap chart for every class with object distribution shows how many images are in the dataset with a certain number of objects of a specific class. Users can click cell and see the list of all corresponding images.
Class sizes #
The table below gives various size properties of objects for every class. Click a row to see the image with annotations of the selected class. Sort columns to find classes with the smallest or largest objects or understand the size differences between classes.
Class | Object count | Avg area | Max area | Min area | Min height | Min height | Max height | Max height | Avg height | Avg height | Min width | Min width | Max width | Max width |
---|---|---|---|---|---|---|---|---|---|---|---|---|---|---|
strawberry mask | 17938 | 0.99% | 9.61% | 0% | 4px | 0.53% | 379px | 50.13% | 100px | 13.2% | 5px | 0.5% | 310px | 30.75% |
Spatial Heatmap #
The heatmaps below give the spatial distributions of all objects for every class. These visualizations provide insights into the most probable and rare object locations on the image. It helps analyze objects' placements in a dataset.
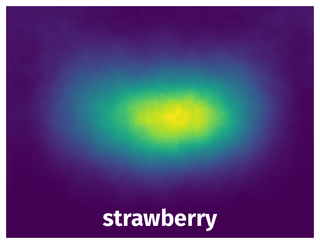
Objects #
Table contains all 17938 objects. Click a row to preview an image with annotations, and use search or pagination to navigate. Sort columns to find outliers in the dataset.
Object ID ㅤ | Class ㅤ | Image name click row to open | Image size height x width | Height ㅤ | Height ㅤ | Width ㅤ | Width ㅤ | Area ㅤ |
---|---|---|---|---|---|---|---|---|
1➔ | strawberry mask | 515.png | 756 x 1008 | 67px | 8.86% | 55px | 5.46% | 0.24% |
2➔ | strawberry mask | 515.png | 756 x 1008 | 75px | 9.92% | 111px | 11.01% | 0.23% |
3➔ | strawberry mask | 515.png | 756 x 1008 | 87px | 11.51% | 132px | 13.1% | 0.99% |
4➔ | strawberry mask | 515.png | 756 x 1008 | 87px | 11.51% | 85px | 8.43% | 0.28% |
5➔ | strawberry mask | 515.png | 756 x 1008 | 34px | 4.5% | 36px | 3.57% | 0.08% |
6➔ | strawberry mask | 515.png | 756 x 1008 | 100px | 13.23% | 120px | 11.9% | 1.22% |
7➔ | strawberry mask | 515.png | 756 x 1008 | 35px | 4.63% | 20px | 1.98% | 0.03% |
8➔ | strawberry mask | 515.png | 756 x 1008 | 34px | 4.5% | 32px | 3.17% | 0.08% |
9➔ | strawberry mask | 1822.png | 756 x 1008 | 52px | 6.88% | 21px | 2.08% | 0.12% |
10➔ | strawberry mask | 1822.png | 756 x 1008 | 112px | 14.81% | 76px | 7.54% | 0.78% |
License #
This dataset is made freely available to academic and non-academic entities for non-commercial purposes such as academic research, teaching, scientific publications, or personal experimentation. Permission is granted to use the data given that you agree:
- That the dataset comes “AS IS”, without express or implied warranty. Although every effort has been made to ensure accuracy, we (Isaac Perez-Borrero, Diego Marin, Manuel E. Gegundez-Arias and Estefanía Cortés-Ancos) do not accept any responsibility for errors or omissions.
- That you include a reference to the StrawDI_Db1 Dataset in any work that makes use of the dataset. For research papers, cite our preferred publication as listed on Using the database Section.
- That you do not distribute this dataset or modified versions. It is permissible to distribute derivative works in as far as they are abstract representations of this dataset (such as models trained on it or additional annotations that do not directly include any of our data) and do not allow to recover the dataset or something similar in character.
- That you may not use the dataset or any derivative work for commercial purposes as, for example, licensing or selling the data, or using the data with a purpose to procure a commercial gain.
- That all rights not expressly granted to you are reserved by us (Isaac Perez-Borrero, Diego Marin, Manuel E. Gegundez-Arias and Estefanía Cortés-Ancos).
Citation #
If you make use of the VOC2012 data, please cite the following reference (to be prepared after the challenge workshop) in any publications:
Kindly provided by the StrawDI Team (see https://strawdi.github.io/).
@article{PEREZBORRERO2020105736,
title = "A fast and accurate deep learning method for strawberry instance segmentation",
journal = "Computers and Electronics in Agriculture",
volume = "178",
pages = "105736",
year = "2020",
issn = "0168-1699",
doi = "https://doi.org/10.1016/j.compag.2020.105736",
url = "http://www.sciencedirect.com/science/article/pii/S0168169920300624",
author = "Isaac Pérez-Borrero and Diego Marín-Santos and Manuel E. Gegúndez-Arias and Estefanía Cortés-Ancos"
}
If you are happy with Dataset Ninja and use provided visualizations and tools in your work, please cite us:
@misc{ visualization-tools-for-strawdi-dataset,
title = { Visualization Tools for StrawDI_Db1 Dataset },
type = { Computer Vision Tools },
author = { Dataset Ninja },
howpublished = { \url{ https://datasetninja.com/strawdi } },
url = { https://datasetninja.com/strawdi },
journal = { Dataset Ninja },
publisher = { Dataset Ninja },
year = { 2025 },
month = { jul },
note = { visited on 2025-07-12 },
}
Download #
Please visit dataset homepage to download the data.
Disclaimer #
Our gal from the legal dep told us we need to post this:
Dataset Ninja provides visualizations and statistics for some datasets that can be found online and can be downloaded by general audience. Dataset Ninja is not a dataset hosting platform and can only be used for informational purposes. The platform does not claim any rights for the original content, including images, videos, annotations and descriptions. Joint publishing is prohibited.
You take full responsibility when you use datasets presented at Dataset Ninja, as well as other information, including visualizations and statistics we provide. You are in charge of compliance with any dataset license and all other permissions. You are required to navigate datasets homepage and make sure that you can use it. In case of any questions, get in touch with us at hello@datasetninja.com.