Introduction #
TICaM Real Images: A Time-of-Flight In-Car Cabin Monitoring Dataset is a time-of-flight dataset of car in-cabin images providing means to test extensive car cabin monitoring systems based on deep learning methods. The authors provide depth, RGB, and infrared images of front car cabin that have been recorded using a driving simulator capturing various dynamic scenarios that usually occur while driving. For dataset they provide ground truth annotations for 2D and 3D object detection, as well as for instance segmentation.
Note, similar TICaM Real Images: A Time-of-Flight In-Car Cabin Monitoring Dataset dataset is also available on the DatasetNinja.com:
Dataset description
With the advent of autonomous and driver-less vehicles, it is imperative to monitor the entire in-car cabin scene in order to realize active and passive safety functions, as well as comfort functions and advanced human-vehicle interfaces. Such car cabin monitoring systems typically involve a camera fitted in the overhead module of a car and a suite of algorithms to monitor the environment within a vehicle. To aid these monitoring systems, several in-car datasets exist to train deep leaning methods for solving problems like driver distraction monitoring, occupant detection or activity recognition. The authors present TICaM, an in-car cabin dataset of 6.7K real time-of-flight depth images with ground truth annotations for 2D and 3D object detection, and semantic and instance segmentation. Their intention is to provide a comprehensive in-car cabin depth image dataset that addresses the deficiencies of currently available such datasets in terms of the ambit of labeled classes, recorded scenarios and provided annotations; all at the same time.
Real images. IR-image, depth image with 2D bounding box annotation, segmentation mask.
A noticeable constraint in existing car cabin datasets is the omission of certain frequently encountered driving scenarios. Notably absent are situations involving passengers, commonplace objects, and the presence of children and infants in forward and rearward facing child seats. The authors have conscientiously addressed this limitation by capturing a diverse array of everyday driving scenarios. This meticulous approach ensures that our dataset proves valuable for pivotal automotive safety applications, such as optimizing airbag adjustments. Using the same airbag configuration for both children and adults can pose a fatal risk to the child. Therefore, it is imperative to identify the occupant class (person, child, infant, object, or empty) for each car seat and determine the child seat configuration (forward-facing FF or rearward-facing RF). The dataset also includes annotations for both driver and passenger activity. Recognizing activity is not only vital for innovative contactless human-machine interfaces but can also be integrated with other modalities, such as driver gaze monitoring. This integration enables a robust estimation of the driver’s state, activity, awareness, and distraction critical factors for hand-over maneuvers in conditional or highly automated driving scenarios. A notable omission in widely used in-car cabin datasets is their lack of multi-modality.
In addressing this gap, the authors present a comprehensive set of depth, RGB, and infrared images, emphasizing the inclusion of 3D data annotations. These images were systematically captured within a driving simulator, employing a Kinect Azure device securely positioned near the rear-view mirror. This setup offers a more practical viewpoint compared to other datasets and a mounting position that can be faithfully replicated within actual cars. The utilization of Time-of-Flight depth modality is chosen for its distinct advantages. Depth images offer enhanced privacy as subjects remain unidentifiable, exhibit increased resilience to variations in illumination and color, and facilitate natural background removal. The authors furnish 2D and 3D bounding boxes, along with class and instance masks, for depth images. These annotations can be directly applied for training on infrared images and, with some pre-processing, on RGB images as well. This is possible since the relative rotation and translation between depth and RGB cameras are known and provided. Furthermore, the authors enhance the dataset’s comprehensiveness by including annotations for activity recognition tasks, ensuring a thorough coverage of input modalities and ground truth annotations.
Data Acquisition
For data recording, the authors used an in-car cabin test platform. It consists of a realistic in-car cabin mock-up, equipped with a wide angle projection system for a realistic driving experience. A Kinect Azure camera with a wide field of view is mounted at the rear-view mirror position for 2D and 3D data recording. The camera is set to record at 30fps with 2×2 binning. The captured data consists of RGB, depth and IR amplitude images. To ensure a wide range of variability in the dataset, we adjust the seat positioning of the driver and passenger seats in the driving simulator.
The data capturing setup equipped with a wideangle projection system, car front seats and a Kinect Azure camera in the front.
The authors initiate their dataset creation process by outlining specific use cases or scenarios they aim to include. Subsequently, they document these scenarios with the participation of 13 individuals, comprising 4 females and 9 males. The scenarios encompass various configurations:
- Sole presence of the driver,
- Presence of both driver and passenger,
- Presence of the driver and an object,
- Presence of the driver with an empty Forward Facing Child Seat (FF),
- Presence of the driver with an empty Rearward Facing Infant Seat (RF),
- Presence of the driver with an occupied Forward Facing Child Seat (FF),
- Presence of the driver with an occupied Rearward Facing Infant Seat (RF),
- Sole presence of an object.
To ensure a well-orchestrated dataset, the authors choreograph specific actions for both the driver and passenger. These instructions are shared with participants prior to recording driving sequences. For instance, drivers engage in actions such as sitting, normal driving, looking left while turning the wheel, turning right, and more. On the other hand, passengers perform actions such as conversing with the driver, retrieving items from the dashboard, and the like. In total, the authors delineate 20 distinct actions for both drivers and passengers.
For each participant or pair of participants (in cases where both driver and passenger are involved), multiple sequences are recorded with varying positions of car seats. This deliberate variation ensures that any occupant classification and activity recognition model trained on the dataset remains robust across different seat configurations.
Depth and IR images of different driving scenarios provided in TICaM.
In addition to diversifying the scenarios, the authors introduce variations in the appearance of individuals by incorporating different clothing accessories such as jackets and hats. To address practical considerations, the authors opt for human dolls as substitutes for children and infants in scenarios where a single person is driving with a Forward Facing (FF) or Rearward Facing (RF) seat securely positioned on the passenger seat. In conjunction with the dolls, three Forward Facing (FF) and three Rearward Facing (RF) seats are employed, each set in different orientations such as sunshade up/down or handle up/down.
Example human dolls and child seats used for recording scenarios with children and infants on the passenger seat.
Data Format
-
Depth Z-image. The depth image is undistorted with a pixel resolution of 512 × 512 pixels and captures a 105◦ × 105◦ FOV. The depth values are normalized to [1mm] resolution and clipped to a range of [0, 2550mm]. Invalid depth values are coded as ‘0’. Images are stored in 16bit PNG-format.
-
IR Amplitude Image. Undistorted IR images from the depth sensor are provided in the same format as the depth image above.
-
RGB Image. Undistorted color images are saved in PNG-format in 24bit depth. The recorded RGB images have resolution of 1280×720 pixels, but a lower field of view of 90◦×59◦ FOV.
-
2D bounding boxes. For each depth image, the 2D boxes are defined by the top-left and bottom-right corners of the box, its class label and a flag low remission which is set to 1 for objects which are either blac kor very reflective or both, and therefore are barely visible in the depth image.
-
Pixel segmentation masks. For each depth image two corresponding masks are generated: instance mask and class mask. The pixel intensities in these
masks correspond to the class ID in the class mask and the instance ID for a certain class in the instance mask. -
Activity annotations. For all sequences with people in the driver or passenger seat, we provide a .csv file describing the activities performed throughout the sequence. Each .csv contains the activity ID, activity name, person ID, a label either driver or passenger to specify if the action is performed by the driver or he passenger, the starting frame number of the action, the ending frame and the duration of that action in frames.
Summary #
TICaM Real Images: A Time-of-Flight In-Car Cabin Monitoring Dataset is a dataset for instance segmentation, semantic segmentation, object detection, and monocular depth estimation tasks. It is used in the safety and automotive industries.
The dataset consists of 19368 images with 40682 labeled objects belonging to 17 different classes including person, ff, rf, and other: winter jacket, child, backpack, infant, box, water bottle, mobile phone, accessory, book, handbag, blanket, laptop, laptop bag, and background.
Images in the TiCaM: Real Images dataset have pixel-level instance segmentation annotations. There are 10036 (52% of the total) unlabeled images (i.e. without annotations). There are 2 splits in the dataset: train (13998 images) and test (5370 images). Additionally, images are grouped by im id. Also every image contains information about its sequence, activity, duration, person id, status. Images labels have low remission tag. Explore it in supervisely labeling tool. The dataset was released in 2021 by the Germany Research Center for Artificial Intelligence.
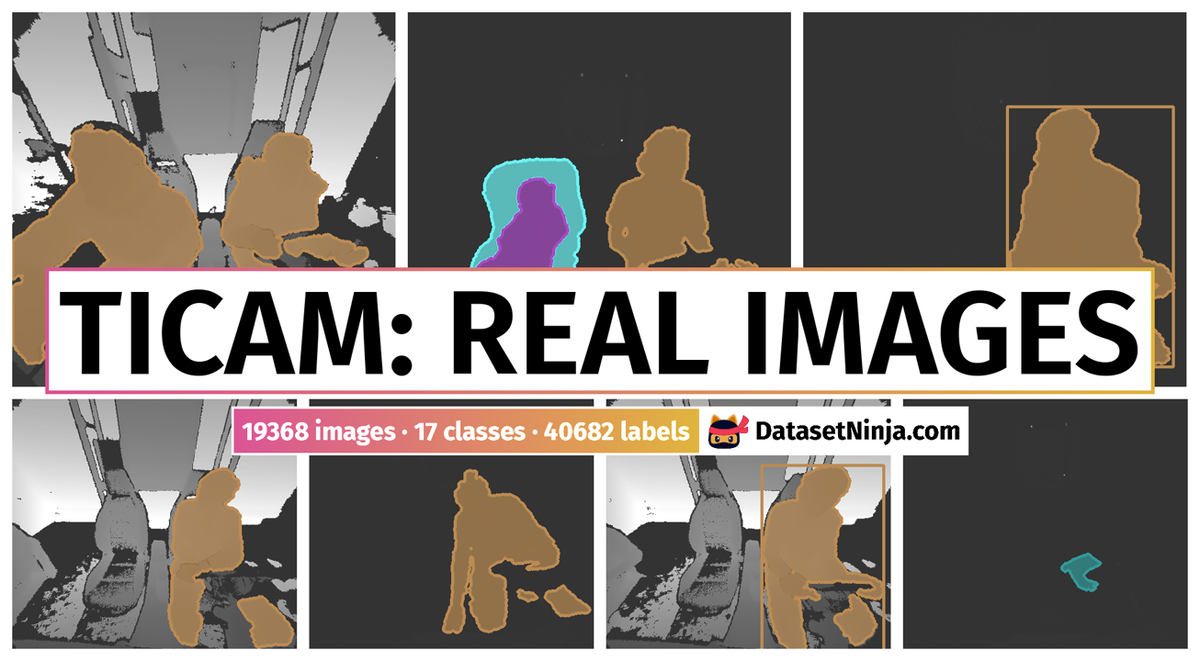
Explore #
TiCaM: Real Images dataset has 19368 images. Click on one of the examples below or open "Explore" tool anytime you need to view dataset images with annotations. This tool has extended visualization capabilities like zoom, translation, objects table, custom filters and more. Hover the mouse over the images to hide or show annotations.
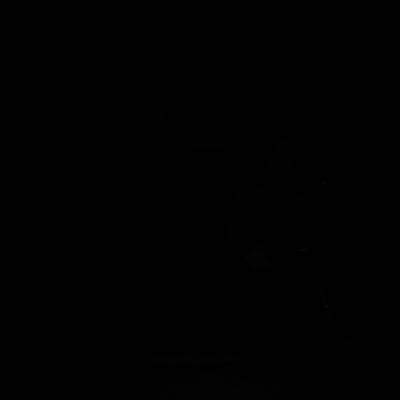
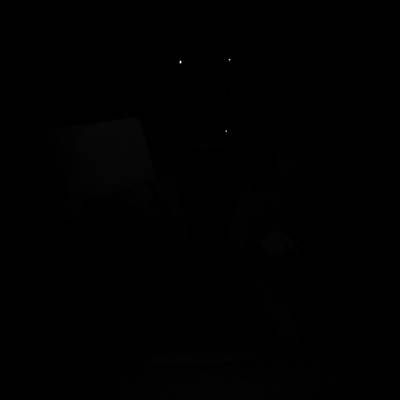
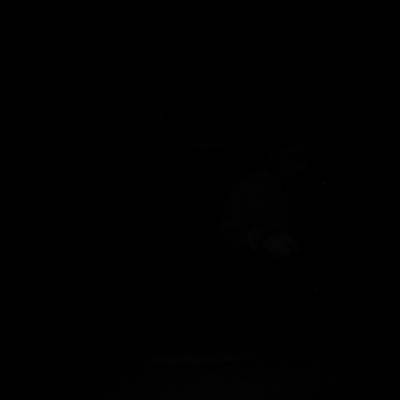
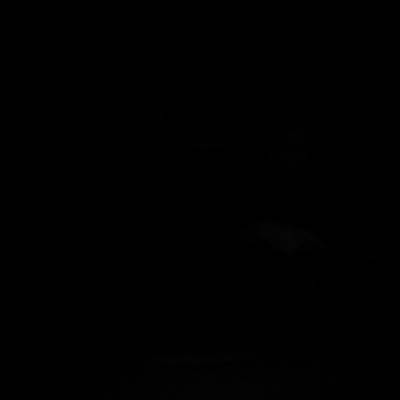
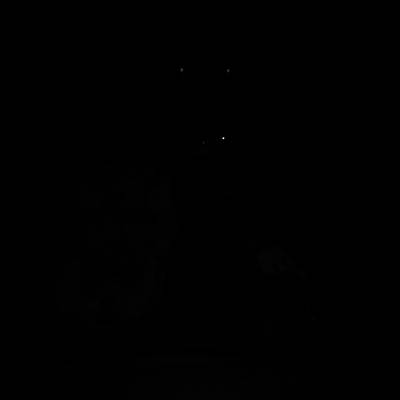
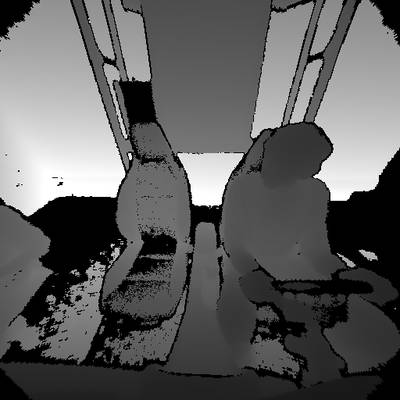

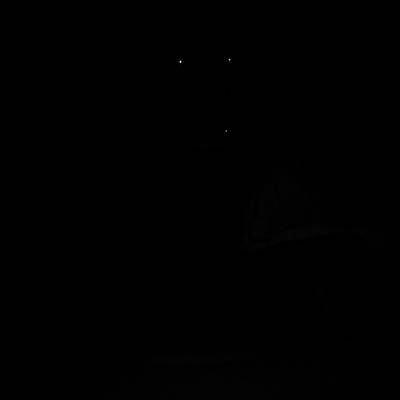
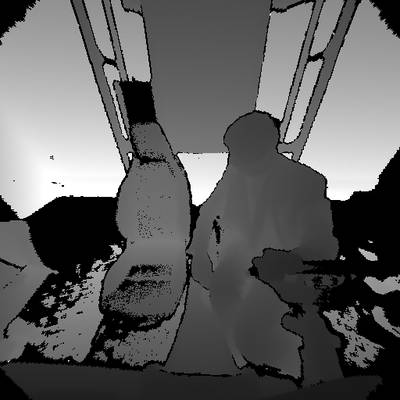
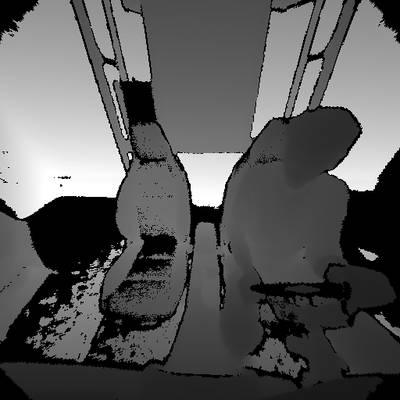
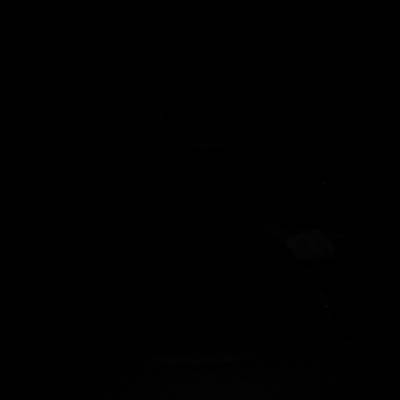

Class balance #
There are 17 annotation classes in the dataset. Find the general statistics and balances for every class in the table below. Click any row to preview images that have labels of the selected class. Sort by column to find the most rare or prevalent classes.
Class ã…¤ | Images ã…¤ | Objects ã…¤ | Count on image average | Area on image average |
---|---|---|---|---|
personâž” any | 8836 | 33914 | 3.84 | 15.55% |
ffâž” any | 1550 | 2064 | 1.33 | 9.09% |
rfâž” any | 668 | 1440 | 2.16 | 8.6% |
winter jacketâž” any | 624 | 650 | 1.04 | 3.47% |
childâž” any | 606 | 750 | 1.24 | 4.28% |
backpackâž” any | 330 | 384 | 1.16 | 2.49% |
infantâž” any | 328 | 356 | 1.09 | 0.54% |
boxâž” any | 306 | 314 | 1.03 | 1.12% |
water bottleâž” any | 182 | 202 | 1.11 | 0.33% |
mobile phoneâž” any | 168 | 176 | 1.05 | 0.03% |
Co-occurrence matrix #
Co-occurrence matrix is an extremely valuable tool that shows you the images for every pair of classes: how many images have objects of both classes at the same time. If you click any cell, you will see those images. We added the tooltip with an explanation for every cell for your convenience, just hover the mouse over a cell to preview the description.
Images #
Explore every single image in the dataset with respect to the number of annotations of each class it has. Click a row to preview selected image. Sort by any column to find anomalies and edge cases. Use horizontal scroll if the table has many columns for a large number of classes in the dataset.
Object distribution #
Interactive heatmap chart for every class with object distribution shows how many images are in the dataset with a certain number of objects of a specific class. Users can click cell and see the list of all corresponding images.
Class sizes #
The table below gives various size properties of objects for every class. Click a row to see the image with annotations of the selected class. Sort columns to find classes with the smallest or largest objects or understand the size differences between classes.
Class | Object count | Avg area | Max area | Min area | Min height | Min height | Max height | Max height | Avg height | Avg height | Min width | Min width | Max width | Max width |
---|---|---|---|---|---|---|---|---|---|---|---|---|---|---|
person any | 33914 | 4.67% | 44.51% | 0.01% | 6px | 1.17% | 379px | 74.02% | 126px | 24.52% | 5px | 0.98% | 510px | 99.61% |
ff any | 2064 | 8.52% | 16.12% | 0.01% | 7px | 1.37% | 253px | 49.41% | 203px | 39.72% | 7px | 1.37% | 177px | 34.57% |
rf any | 1440 | 4.37% | 14.82% | 0.01% | 7px | 1.37% | 212px | 41.41% | 100px | 19.48% | 7px | 1.37% | 232px | 45.31% |
child any | 750 | 3.62% | 9.79% | 0.04% | 18px | 3.52% | 177px | 34.57% | 142px | 27.74% | 9px | 1.76% | 148px | 28.91% |
winter jacket any | 650 | 3.33% | 9.3% | 0.01% | 7px | 1.37% | 199px | 38.87% | 118px | 23.05% | 7px | 1.37% | 246px | 48.05% |
backpack any | 384 | 2.14% | 5.06% | 0.01% | 7px | 1.37% | 167px | 32.62% | 95px | 18.52% | 7px | 1.37% | 151px | 29.49% |
infant any | 356 | 0.54% | 1.42% | 0.08% | 20px | 3.91% | 51px | 9.96% | 40px | 7.73% | 17px | 3.32% | 73px | 14.26% |
box any | 314 | 1.09% | 1.47% | 0.08% | 15px | 2.93% | 97px | 18.95% | 63px | 12.29% | 20px | 3.91% | 82px | 16.02% |
water bottle any | 202 | 0.3% | 0.36% | 0.02% | 8px | 1.56% | 52px | 10.16% | 45px | 8.76% | 8px | 1.56% | 46px | 8.98% |
mobile phone any | 176 | 0.03% | 0.09% | 0.01% | 7px | 1.37% | 17px | 3.32% | 9px | 1.72% | 7px | 1.37% | 22px | 4.3% |
Spatial Heatmap #
The heatmaps below give the spatial distributions of all objects for every class. These visualizations provide insights into the most probable and rare object locations on the image. It helps analyze objects' placements in a dataset.
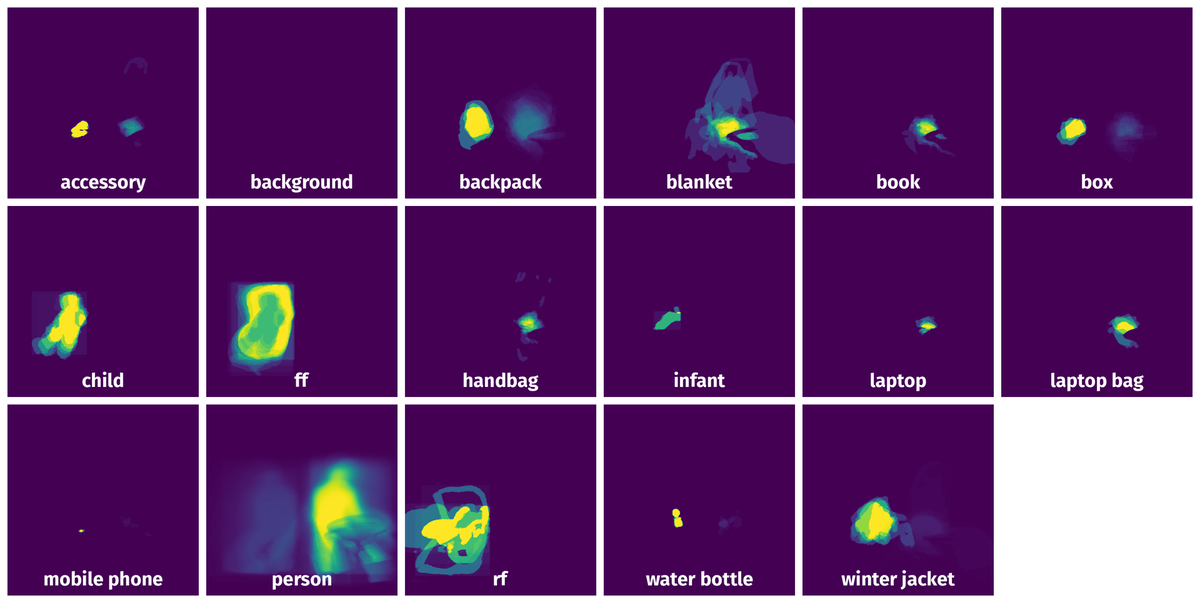
Objects #
Table contains all 40682 objects. Click a row to preview an image with annotations, and use search or pagination to navigate. Sort columns to find outliers in the dataset.
Object ID ã…¤ | Class ã…¤ | Image name click row to open | Image size height x width | Height ã…¤ | Height ã…¤ | Width ã…¤ | Width ã…¤ | Area ã…¤ |
---|---|---|---|---|---|---|---|---|
1âž” | person any | 191107134100_0001_cs00_p01_I7e_a00_c00_q000_o00_MMM_xxx_xxx_q000_DEPTH.png | 512 x 512 | 337px | 65.82% | 223px | 43.55% | 11.63% |
2âž” | person any | 191107134100_0001_cs00_p01_I7e_a00_c00_q000_o00_MMM_xxx_xxx_q000_DEPTH.png | 512 x 512 | 78px | 15.23% | 71px | 13.87% | 1.24% |
3âž” | person any | 200121104739_1261_cs01_p00_E6e_a00_c00_q000_p03_E5c_a00_c01_q000_DEPTH.png | 512 x 512 | 314px | 61.33% | 246px | 48.05% | 9.68% |
4âž” | person any | 200121104739_1261_cs01_p00_E6e_a00_c00_q000_p03_E5c_a00_c01_q000_DEPTH.png | 512 x 512 | 280px | 54.69% | 181px | 35.35% | 9.72% |
5âž” | person any | 200121104739_1261_cs01_p00_E6e_a00_c00_q000_p03_E5c_a00_c01_q000_DEPTH.png | 512 x 512 | 7px | 1.37% | 7px | 1.37% | 0.01% |
6âž” | person any | 200121104739_1261_cs01_p00_E6e_a00_c00_q000_p03_E5c_a00_c01_q000_DEPTH.png | 512 x 512 | 32px | 6.25% | 70px | 13.67% | 0.56% |
7âž” | person any | 200121104739_1261_cs01_p00_E6e_a00_c00_q000_p03_E5c_a00_c01_q000_DEPTH.png | 512 x 512 | 24px | 4.69% | 43px | 8.4% | 0.24% |
8âž” | person any | 200121104739_1261_cs01_p00_E6e_a00_c00_q000_p03_E5c_a00_c01_q000_DEPTH.png | 512 x 512 | 29px | 5.66% | 24px | 4.69% | 0.13% |
9âž” | person any | 200121104739_1261_cs01_p00_E6e_a00_c00_q000_p03_E5c_a00_c01_q000_DEPTH.png | 512 x 512 | 16px | 3.12% | 16px | 3.12% | 0.06% |
10âž” | person any | 200121104739_1261_cs01_p00_E6e_a00_c00_q000_p03_E5c_a00_c01_q000_DEPTH.png | 512 x 512 | 280px | 54.69% | 181px | 35.35% | 19.33% |
License #
License is unknown for the TICaM: A Time-of-flight In-car Cabin Monitoring Dataset dataset.
Citation #
If you make use of the TiCaM data, please cite the following reference:
@inproceedings{katrolia2021ticam,
author = {Jigyasa Singh Katrolia and
Ahmed El{-}Sherif and
Hartmut Feld and
Bruno Mirbach and
Jason R. Rambach and
Didier Stricker},
title = {TICaM: {A} Time-of-flight In-car Cabin Monitoring Dataset},
booktitle = {32nd British Machine Vision Conference 2021, {BMVC} 2021, Online,
November 22-25, 2021},
pages = {277},
publisher = {{BMVA} Press},
year = {2021},
url = {https://www.bmvc2021-virtualconference.com/assets/papers/0701.pdf},
timestamp = {Wed, 22 Jun 2022 16:52:45 +0200},
biburl = {https://dblp.org/rec/conf/bmvc/KatroliaEFMRS21.bib},
bibsource = {dblp computer science bibliography, https://dblp.org}
}
If you are happy with Dataset Ninja and use provided visualizations and tools in your work, please cite us:
@misc{ visualization-tools-for-ticam-real-images-dataset,
title = { Visualization Tools for TiCaM: Real Images Dataset },
type = { Computer Vision Tools },
author = { Dataset Ninja },
howpublished = { \url{ https://datasetninja.com/ticam-real-images } },
url = { https://datasetninja.com/ticam-real-images },
journal = { Dataset Ninja },
publisher = { Dataset Ninja },
year = { 2025 },
month = { apr },
note = { visited on 2025-04-03 },
}
Download #
Please visit dataset homepage to download the data.
Disclaimer #
Our gal from the legal dep told us we need to post this:
Dataset Ninja provides visualizations and statistics for some datasets that can be found online and can be downloaded by general audience. Dataset Ninja is not a dataset hosting platform and can only be used for informational purposes. The platform does not claim any rights for the original content, including images, videos, annotations and descriptions. Joint publishing is prohibited.
You take full responsibility when you use datasets presented at Dataset Ninja, as well as other information, including visualizations and statistics we provide. You are in charge of compliance with any dataset license and all other permissions. You are required to navigate datasets homepage and make sure that you can use it. In case of any questions, get in touch with us at hello@datasetninja.com.