Introduction #
The authors proposed a new aerial UAV123 Dataset for low altitude UAV target tracking, as well as, a photorealistic UAV simulator that can be coupled with tracking methods. Their benchmark provides the first evaluation of many state-of-the-art and popular trackers on 123 new and fully annotated HD video sequences captured from a low-altitude aerial perspective.
Motivation
Despite decades of advancements, visual tracking remains a persistently challenging problem. Evaluating tracking algorithms typically involves testing them on established video benchmarks. The effectiveness of a tracker is gauged against these benchmarks, making it crucial to ensure they encompass a comprehensive range of real-world scenarios and tracking challenges, such as fast motion, changes in illumination, scale variations, occlusions, and more. These benchmarks play a vital role in shaping future research directions and in the development of robust algorithms. However, a notable gap in these benchmarks is the absence of comprehensive annotated aerial datasets, which are essential for addressing challenges posed by unmanned aerial flight.
The integration of automated computer vision capabilities into unmanned aerial vehicles (UAVs), including tracking and object/activity recognition, has emerged as a significant research focus. This trend is fueled by the growing accessibility of low-cost commercial UAVs. Aerial tracking, beyond its traditional surveillance applications, has opened up new avenues in computer vision, ranging from search and rescue operations to wildlife monitoring, crowd management, navigation, obstacle avoidance, and extreme sports videography. Aerial tracking extends to a diverse array of objects, including humans, animals, cars, boats, and more, many of which are difficult or impossible to track persistently from the ground. Real-world aerial tracking scenarios present unique challenges, necessitating innovative approaches to tackle the tracking problem effectively.
Dataset description
The authors’ work entails evaluating trackers using over 100 newly annotated HD videos captured by a professional-grade UAV. This benchmark serves to complement existing benchmarks by addressing the aerial aspect of tracking comprehensively and offering a more diverse range of tracking challenges commonly encountered in low-altitude UAV footage. It stands as the first benchmark to systematically analyze the performance of state-of-the-art trackers on a comprehensive set of annotated aerial sequences featuring specific tracking challenges. The authors anticipate that this dataset, along with the tracker evaluation, will establish a foundational reference point for future advancements in UAV technology and improvements in target trackers. Visual tracking on UAVs holds significant promise, as the camera can dynamically adjust its orientation and position to optimize tracking performance based on visual feedback. This dynamic capability sets it apart from static tracking systems, which passively analyze dynamic scenes. Current benchmarks, which consist of pre-recorded scenes, fall short in quantifying how slower trackers may impact the UAV’s ability to effectively track targets in real-time.
Video captured from low-altitude UAVs is inherently different from video in popular tracking datasets. Therefore, the authors propose a new dataset UAV123 with sequences from an aerial viewpoint, a subset of which is meant for long-term aerial tracking (uav20l). The results highlight the effect of camera viewpoint change arising from UAV motion. The variation in bounding box size and aspect ratio with respect to the initial frame is significantly larger in UAV123. Furthermore, being mounted on the UAV, the camera is able to move with the target resulting in longer tracking sequences on average.
Column 1 and 2: Proportional change of the target’s aspect ratio and bounding box size (area in pixels) with respect to the first frame and across three datasets: OTB100, TC128, and UAV123. Results are compiled over all sequences in each dataset as a histogram with log scale on the x-axis. Column 3: Histogram of sequence duration (in seconds) across the three datasets.
The new UAV123 dataset contains a total of 123 video sequences and more than 110K frames making it the second largest object tracking dataset after ALOV300++. The statistics of the authors dataset are compared to existing datasets.
Dataset | UAV123 | UAV20L | VIVID | OTB50 | OTB100 | TC128 | VOT14 | VOT15 | ALOV300 |
---|---|---|---|---|---|---|---|---|---|
Sequences | 123 | 20 | 9 | 51 | 100 | 129 | 25 | 60 | 314 |
Min frames | 109 | 1717 | 1301 | 71 | 71 | 71 | 171 | 48 | 19 |
Mean frames | 915 | 2934 | 1808 | 578 | 590 | 429 | 416 | 365 | 483 |
Max frames | 3085 | 5527 | 2571 | 3872 | 3872 | 3872 | 1217 | 1507 | 5975 |
Total frames | 112578 | 58670 | 16274 | 29491 | 59040 | 55346 | 10389 | 21871 | 151657 |
Comparison of tracking datasets in the literature.
The UAV123 dataset comprises three distinct subsets:
- Set1 encompasses 103 sequences captured using a commercial-grade UAV (DJI S1000). These sequences feature various objects tracked at altitudes ranging from 5 to 25 meters. Video recordings were made at frame rates spanning from 30 to 96 FPS and resolutions from 720p to 4K, utilizing a Panasonic GH4 camera equipped with an Olympus M.Zuiko 12mm f2.0 lens mounted on a fully stabilized gimbal system (DJI Zenmuse Z15). All sequences are standardized at 720p and 30 FPS, with annotations provided in the form of upright bounding boxes at 30 FPS. The annotations were manually conducted at 10 FPS and subsequently interpolated linearly to 30 FPS.
- Set2 comprises 12 sequences captured using a boardcam (lacking image stabilization) affixed to an inexpensive UAV tracking other UAVs. These sequences exhibit lower quality and resolution, often containing noticeable noise due to limitations in video transmission bandwidth. Annotation protocols mirror those of Set1.
- Set3 features 8 synthetic sequences generated by the authors’ proposed UAV simulator. In these sequences, targets traverse predetermined trajectories within various virtual environments rendered using the Unreal4 Game Engine, simulating the perspective of a flying UAV. Annotations are automatically generated at 30 FPS, with full object mask/segmentation also available.
Note: the authors did not provide the opportunity to divide the dataset according to the above criteria.
First frame of selected sequences from UAV123 dataset. The red bounding box indicates the ground truth annotation.
The UAV123 dataset encompasses a diverse array of scenes, ranging from urban landscapes to roads, building, fields, beaches, and harbor/marina settings. It features a wide spectrum of targets, including car, truck, boat, individuals, group, and aerial vehicles (uav) engaged in various activities such as walking, cycling, wakeboarding, driving, swimming, and flying. As expected, these sequences present typical visual tracking challenges, such as long-term full and partial occlusion, scale variations, changes in illumination, shifts in viewpoint, background clutter, camera motion, and more.
Attr | Description |
---|---|
ARC | aspect ratio change: the fraction of ground truth aspect ratio in the first frame and at least one subsequent frame is outside the range [0.5, 2]. |
BC | background clutter: the background near the target has similar appearance as the target. |
CM | camera motion: abrupt motion of the camera. |
FM | fast motion: motion of the ground truth bounding box is larger than 20 pixels between two consecutive frames. |
FOC | full occlusion: the target is fully occluded. |
IV | illumination variation: the illumination of the target changes significantly. |
LR | low resolution: at least one ground truth bounding box has less than 400 pixels. |
OV | out of view: some portion of the target leaves the view. |
POC | partial occlusion: the target is partially occluded. |
SOB | similar object: there are objects of similar shape or same type near the target. |
SV | scale variation: the ratio of initial and at least one subsequent bounding box is outside the range [0.5, 2]. |
VC | viewpoint change: viewpoint affects target appearance significantly. |
Attributes used to characterize each sequence from a tracking perspective.
In aerial surveillance scenarios, object tracking often demands long-term continuity, as the camera can dynamically pursue the target, unlike in static surveillance setups. In designing the dataset, fully annotated lengthy sequences captured in a single continuous shot were intentionally subdivided into subsequences to maintain a manageable level of difficulty. To accommodate long-term tracking, these subsequences were subsequently merged, and the 20 longest sequences were selected for inclusion in the dataset (uav20l).
Summary #
UAV123 Dataset is a dataset for an object detection task. It is used in the drone inspection domain.
The dataset consists of 113476 images with 109866 labeled objects belonging to 10 different classes including person, car, group, and other: wakeboard, boat, uav, bike, building, truck, and bird.
Images in the UAV123 dataset have bounding box annotations. There are 3610 (3% of the total) unlabeled images (i.e. without annotations). There are no pre-defined train/val/test splits in the dataset. Alternatively, the dataset could be split into 12 tracking perspectives: scale variation (100919 images), camera motion (75025 images), partial occlusion (73677 images), aspect ratio change (70737 images), viewpoint change (60143 images), similar object (43669 images), low resolution (39016 images), out of view (33421 images), illumination variation (32803 images), full occlusion (30736 images), fast motion (29387 images), and background clutter (17942 images). Additionally, images marked with its sequence and uav20l tag. The dataset was released in 2016 by the King Abdullah University of Science and Technology, Saudi Arabia.
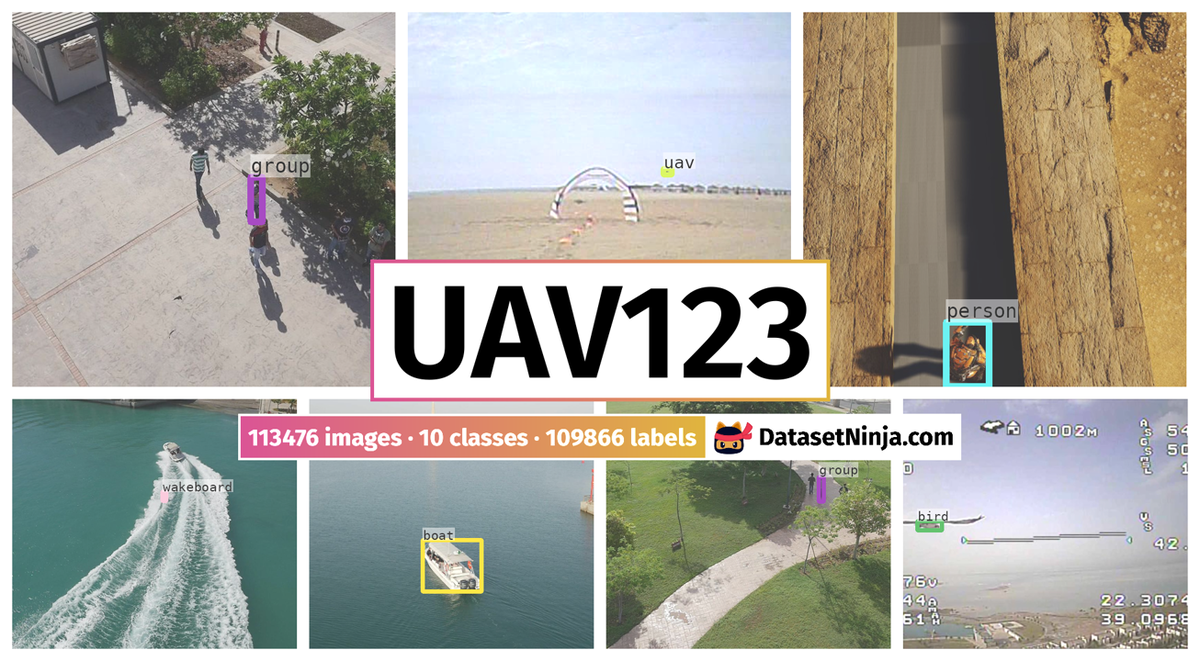
Explore #
UAV123 dataset has 113476 images. Click on one of the examples below or open "Explore" tool anytime you need to view dataset images with annotations. This tool has extended visualization capabilities like zoom, translation, objects table, custom filters and more. Hover the mouse over the images to hide or show annotations.
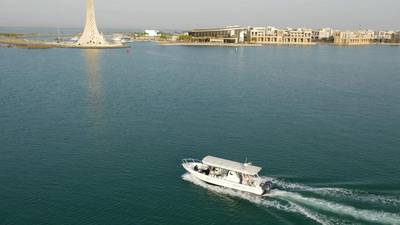
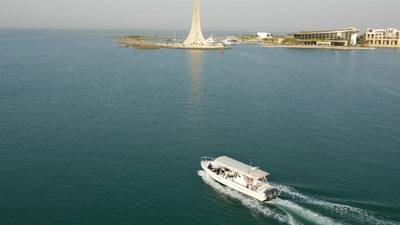
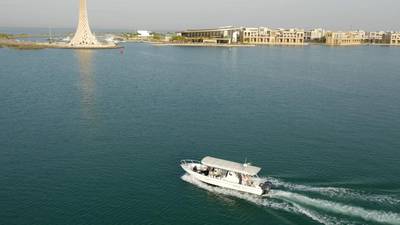
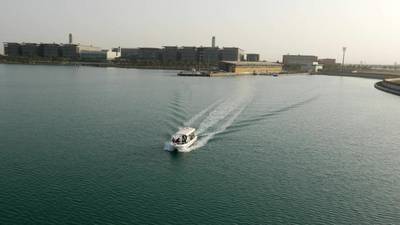
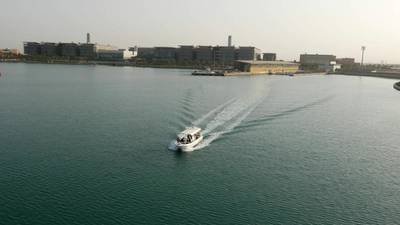
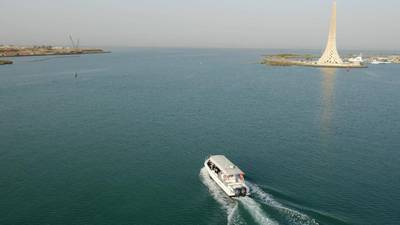
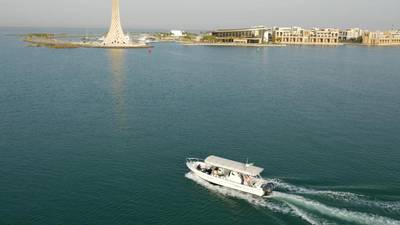
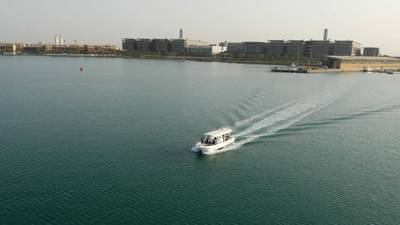
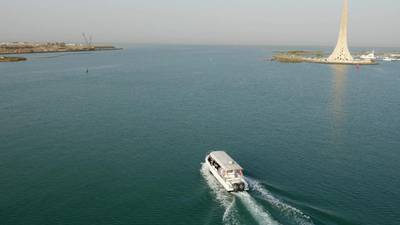
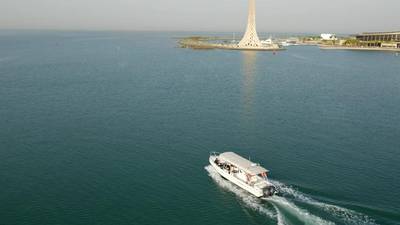
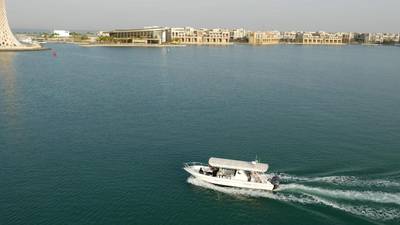
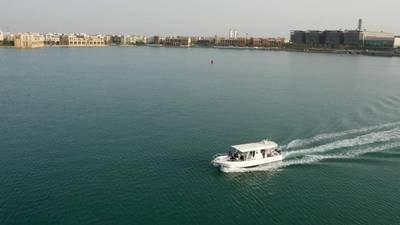
Class balance #
There are 10 annotation classes in the dataset. Find the general statistics and balances for every class in the table below. Click any row to preview images that have labels of the selected class. Sort by column to find the most rare or prevalent classes.
Class ã…¤ | Images ã…¤ | Objects ã…¤ | Count on image average | Area on image average |
---|---|---|---|---|
personâž” rectangle | 36051 | 36051 | 1 | 0.95% |
carâž” rectangle | 30233 | 30233 | 1 | 1.23% |
groupâž” rectangle | 12670 | 12670 | 1 | 0.2% |
wakeboardâž” rectangle | 8080 | 8080 | 1 | 0.21% |
boatâž” rectangle | 7083 | 7083 | 1 | 1.36% |
uavâž” rectangle | 4674 | 4674 | 1 | 0.28% |
bikeâž” rectangle | 4036 | 4036 | 1 | 1.02% |
buildingâž” rectangle | 3143 | 3143 | 1 | 0.25% |
truckâž” rectangle | 2644 | 2644 | 1 | 1.18% |
birdâž” rectangle | 1252 | 1252 | 1 | 0.19% |
Co-occurrence matrix #
Co-occurrence matrix is an extremely valuable tool that shows you the images for every pair of classes: how many images have objects of both classes at the same time. If you click any cell, you will see those images. We added the tooltip with an explanation for every cell for your convenience, just hover the mouse over a cell to preview the description.
Images #
Explore every single image in the dataset with respect to the number of annotations of each class it has. Click a row to preview selected image. Sort by any column to find anomalies and edge cases. Use horizontal scroll if the table has many columns for a large number of classes in the dataset.
Object distribution #
Interactive heatmap chart for every class with object distribution shows how many images are in the dataset with a certain number of objects of a specific class. Users can click cell and see the list of all corresponding images.
Class sizes #
The table below gives various size properties of objects for every class. Click a row to see the image with annotations of the selected class. Sort columns to find classes with the smallest or largest objects or understand the size differences between classes.
Class | Object count | Avg area | Max area | Min area | Min height | Min height | Max height | Max height | Avg height | Avg height | Min width | Min width | Max width | Max width |
---|---|---|---|---|---|---|---|---|---|---|---|---|---|---|
person rectangle | 36051 | 0.95% | 10.66% | 0.01% | 14px | 1.94% | 560px | 77.78% | 124px | 17.22% | 4px | 0.31% | 197px | 15.39% |
car rectangle | 30233 | 1.23% | 26.23% | 0.01% | 4px | 0.56% | 463px | 64.31% | 82px | 11.39% | 9px | 0.7% | 658px | 51.41% |
group rectangle | 12670 | 0.2% | 0.57% | 0.02% | 20px | 2.78% | 117px | 16.25% | 75px | 10.48% | 5px | 0.39% | 47px | 3.67% |
wakeboard rectangle | 8080 | 0.21% | 2.5% | 0% | 5px | 0.69% | 202px | 28.06% | 47px | 6.49% | 2px | 0.16% | 120px | 9.38% |
boat rectangle | 7083 | 1.36% | 12.65% | 0.01% | 9px | 1.25% | 320px | 44.44% | 89px | 12.32% | 15px | 1.17% | 435px | 33.98% |
uav rectangle | 4674 | 0.28% | 3.43% | 0.02% | 7px | 1.46% | 83px | 17.29% | 18px | 3.8% | 8px | 1.11% | 143px | 19.86% |
bike rectangle | 4036 | 1.02% | 8.06% | 0% | 7px | 0.97% | 307px | 42.64% | 103px | 14.28% | 5px | 0.39% | 244px | 19.06% |
building rectangle | 3143 | 0.25% | 0.76% | 0.05% | 27px | 3.75% | 111px | 15.42% | 51px | 7.15% | 17px | 1.33% | 100px | 7.81% |
truck rectangle | 2644 | 1.18% | 34.14% | 0.01% | 8px | 1.11% | 368px | 51.11% | 39px | 5.48% | 10px | 0.78% | 855px | 66.8% |
bird rectangle | 1252 | 0.19% | 0.53% | 0.01% | 3px | 0.42% | 97px | 13.47% | 36px | 5.05% | 10px | 0.78% | 97px | 7.58% |
Spatial Heatmap #
The heatmaps below give the spatial distributions of all objects for every class. These visualizations provide insights into the most probable and rare object locations on the image. It helps analyze objects' placements in a dataset.
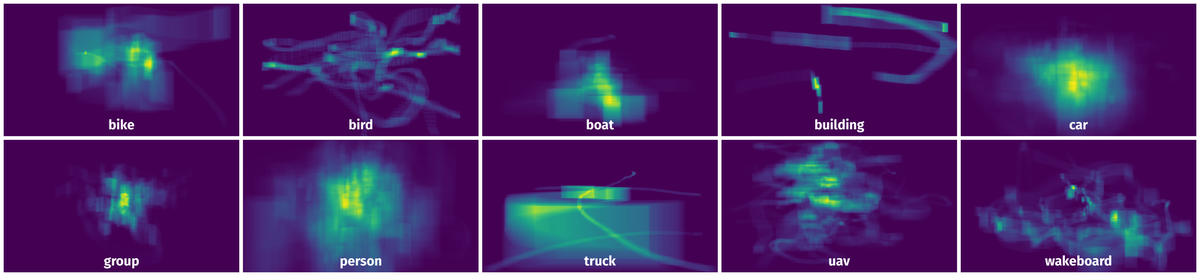
Objects #
Table contains all 100088 objects. Click a row to preview an image with annotations, and use search or pagination to navigate. Sort columns to find outliers in the dataset.
Object ID ã…¤ | Class ã…¤ | Image name click row to open | Image size height x width | Height ã…¤ | Height ã…¤ | Width ã…¤ | Width ã…¤ | Area ã…¤ |
---|---|---|---|---|---|---|---|---|
1âž” | boat rectangle | boat1_000202.jpg | 720 x 1280 | 222px | 30.83% | 123px | 9.61% | 2.96% |
2âž” | group rectangle | group1_003746.jpg | 720 x 1280 | 103px | 14.31% | 27px | 2.11% | 0.3% |
3âž” | boat rectangle | boat1_000670.jpg | 720 x 1280 | 138px | 19.17% | 88px | 6.88% | 1.32% |
4âž” | person rectangle | person20_000521.jpg | 720 x 1280 | 266px | 36.94% | 113px | 8.83% | 3.26% |
5âž” | boat rectangle | boat7_000012.jpg | 720 x 1280 | 72px | 10% | 166px | 12.97% | 1.3% |
6âž” | car rectangle | car1_000600.jpg | 720 x 1280 | 35px | 4.86% | 32px | 2.5% | 0.12% |
7âž” | group rectangle | group1_000200.jpg | 720 x 1280 | 105px | 14.58% | 38px | 2.97% | 0.43% |
8âž” | person rectangle | person13_000313.jpg | 720 x 1280 | 97px | 13.47% | 40px | 3.12% | 0.42% |
9âž” | car rectangle | car5_000144.jpg | 720 x 1280 | 166px | 23.06% | 157px | 12.27% | 2.83% |
10âž” | uav rectangle | uav1_002267.jpg | 480 x 720 | 21px | 4.38% | 42px | 5.83% | 0.26% |
License #
License is unknown for the UAV123 Dataset dataset.
Citation #
If you make use of the UAV123 data, please cite the following reference:
@dataset{UAV123,
author={Matthias Mueller and Neil Smith and Bernard Ghanem},
title={UAV123 Dataset},
year={2016},
url={https://cemse.kaust.edu.sa/ivul/uav123}
}
If you are happy with Dataset Ninja and use provided visualizations and tools in your work, please cite us:
@misc{ visualization-tools-for-uav123-dataset,
title = { Visualization Tools for UAV123 Dataset },
type = { Computer Vision Tools },
author = { Dataset Ninja },
howpublished = { \url{ https://datasetninja.com/uav123 } },
url = { https://datasetninja.com/uav123 },
journal = { Dataset Ninja },
publisher = { Dataset Ninja },
year = { 2025 },
month = { apr },
note = { visited on 2025-04-24 },
}
Download #
Please visit dataset homepage to download the data.
Disclaimer #
Our gal from the legal dep told us we need to post this:
Dataset Ninja provides visualizations and statistics for some datasets that can be found online and can be downloaded by general audience. Dataset Ninja is not a dataset hosting platform and can only be used for informational purposes. The platform does not claim any rights for the original content, including images, videos, annotations and descriptions. Joint publishing is prohibited.
You take full responsibility when you use datasets presented at Dataset Ninja, as well as other information, including visualizations and statistics we provide. You are in charge of compliance with any dataset license and all other permissions. You are required to navigate datasets homepage and make sure that you can use it. In case of any questions, get in touch with us at hello@datasetninja.com.