Introduction #
The dataset, known as Vehicle Wheel Detection | Axle Detection, comprises an exceptionally challenging collection featuring over 6,000 images dedicated to the detection of vehicle wheels. These images are sourced from a diverse range of more than 2,000 locations, with each image undergoing meticulous manual inspection and validation by computer vision experts at Datacluster Labs. This extensive dataset encompasses a broad spectrum of vehicles, including trucks, cars, bicycles, and more.
Parameter | Details |
---|---|
Dataset size | 6000+ images |
Captured by | Over 2000+ crowdsource contributors |
Resolution | HD and above |
Location | Captured with 2000+ locations |
Diversity | Various lighting conditions like day, night, varied distances, view points etc. |
Device used | Captured using mobile phones in 2020-2022 |
Usage | Wheel Detection, Wheel Counting, Object detection |
Summary #
Vehicle Wheel Detection | Axle Detection is a dataset for an object detection task. It is used in the automotive industry.
The dataset consists of 675 images with 2993 labeled objects belonging to 8 different classes including wheel, 2_wheeler, 3_wheeler, and other: 4_wheeler, 6_wheeler, 8_wheeler, auto, and 10_wheeler.
Images in the Vehicle Wheel Detection dataset have bounding box annotations. There are 6 (1% of the total) unlabeled images (i.e. without annotations). There are no pre-defined train/val/test splits in the dataset. The dataset was released in 2023 by the DataCluster Labs, India.
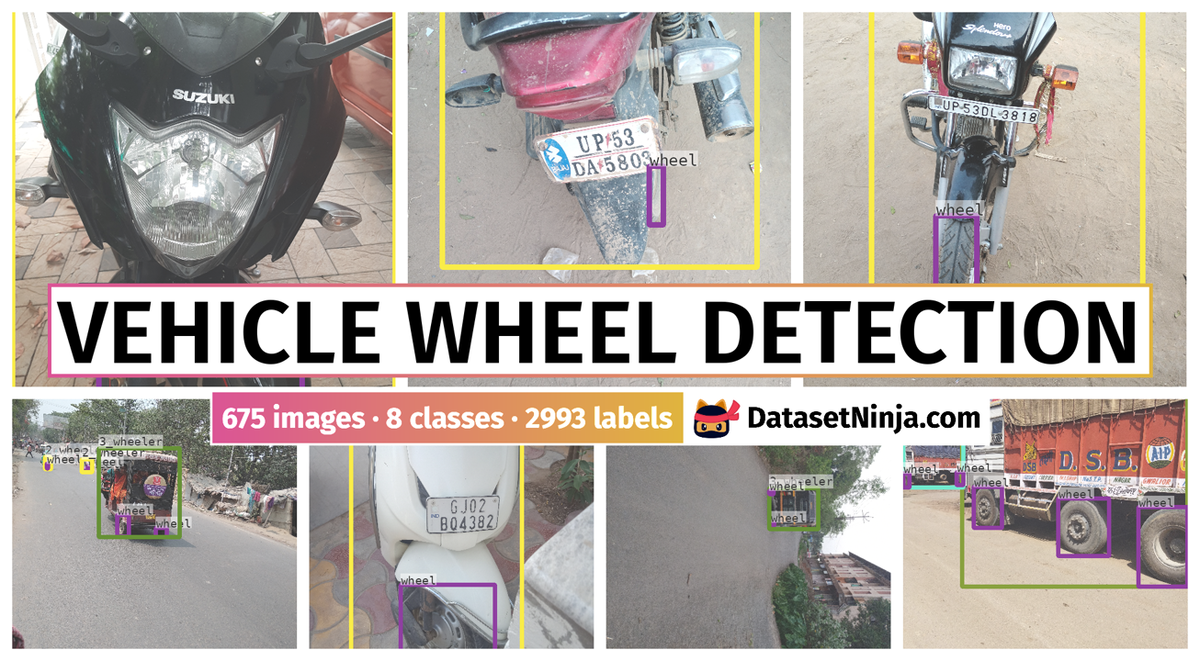
Explore #
Vehicle Wheel Detection dataset has 675 images. Click on one of the examples below or open "Explore" tool anytime you need to view dataset images with annotations. This tool has extended visualization capabilities like zoom, translation, objects table, custom filters and more. Hover the mouse over the images to hide or show annotations.
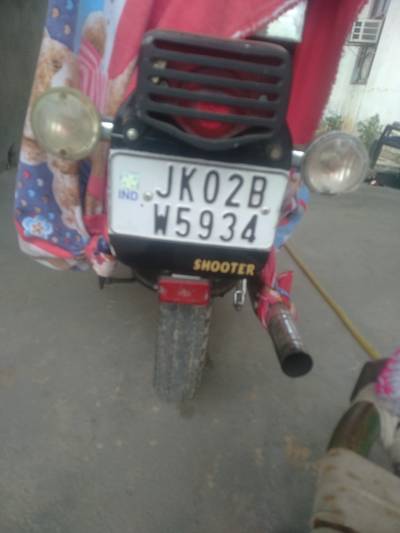
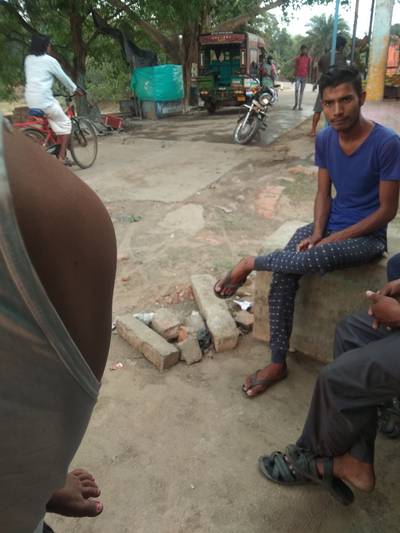

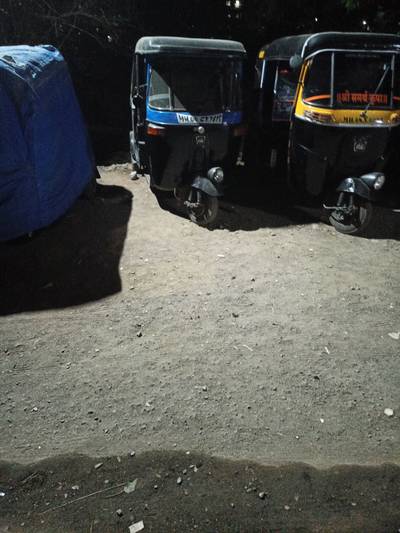
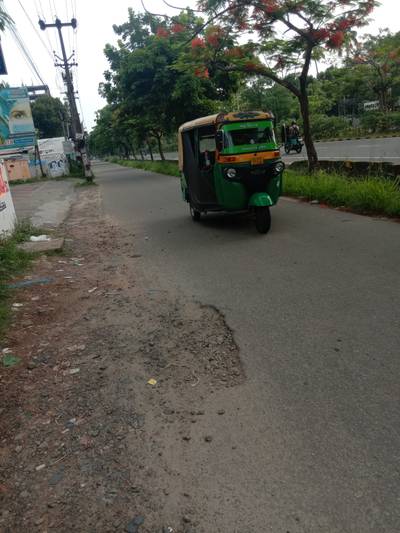
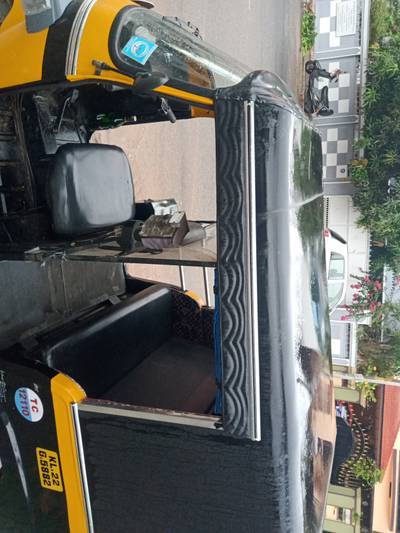
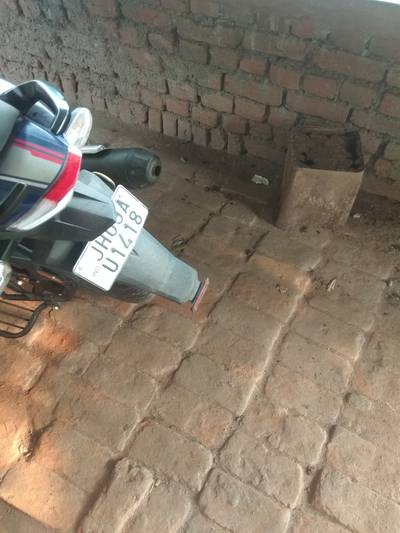
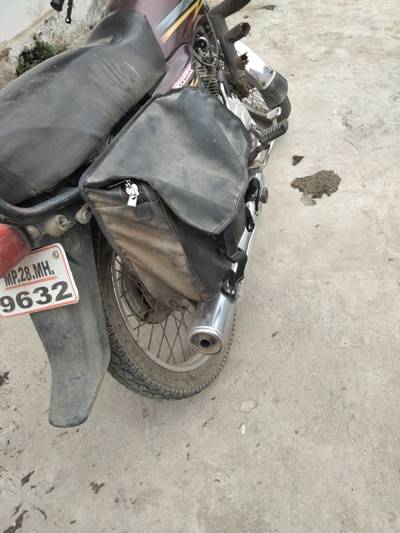
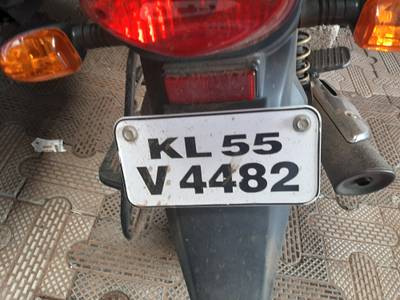
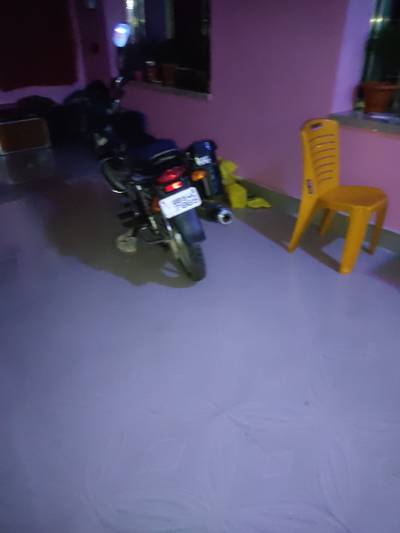
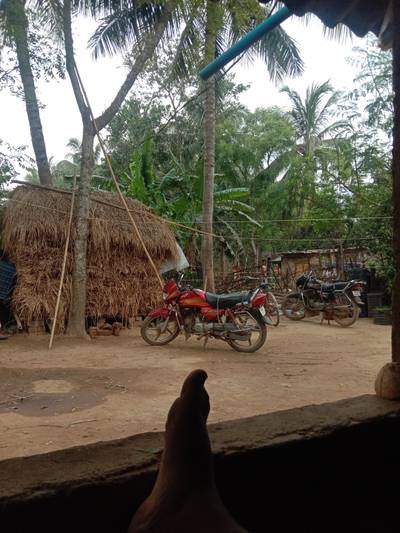
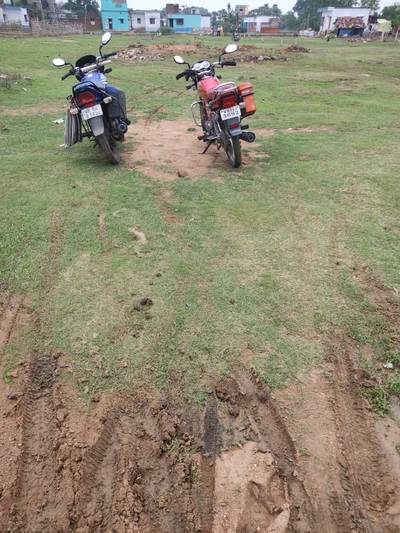
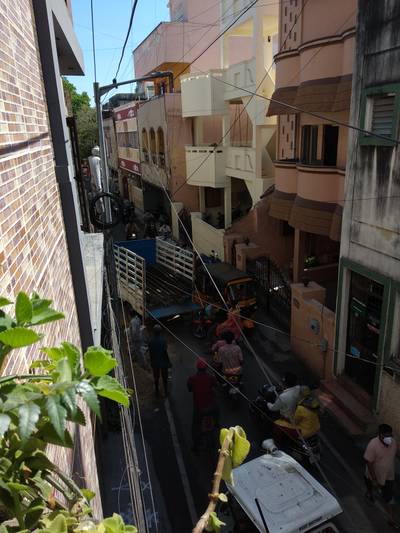
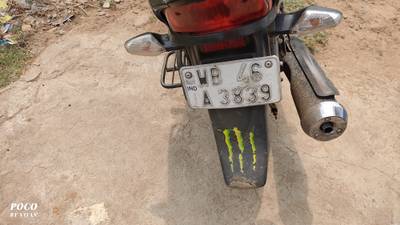
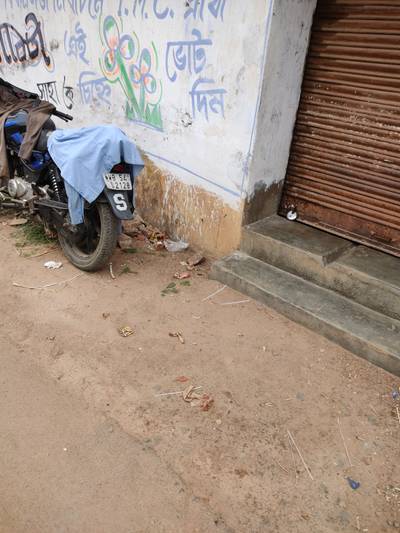
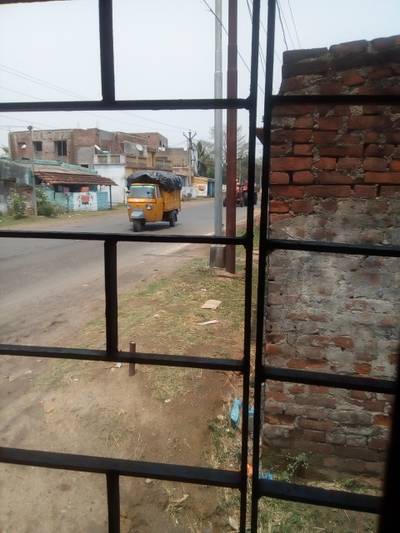

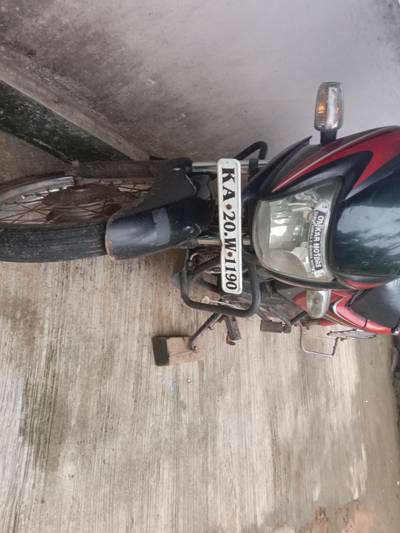
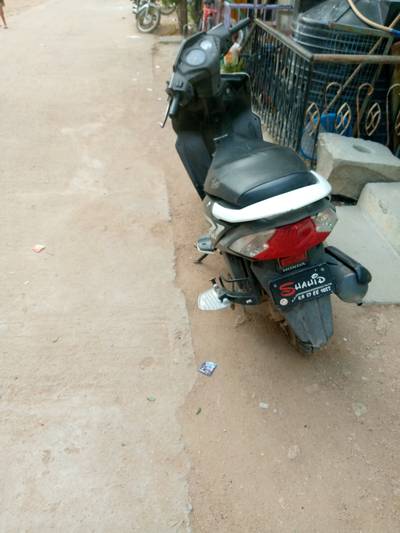
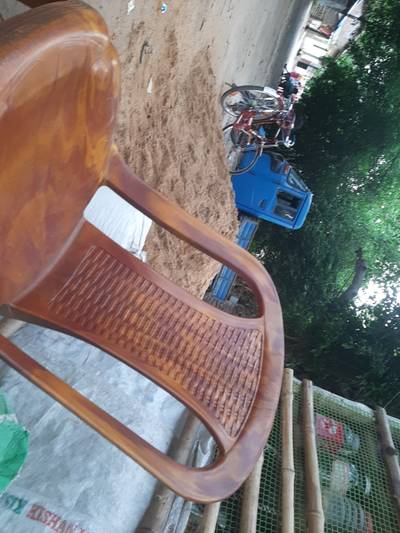

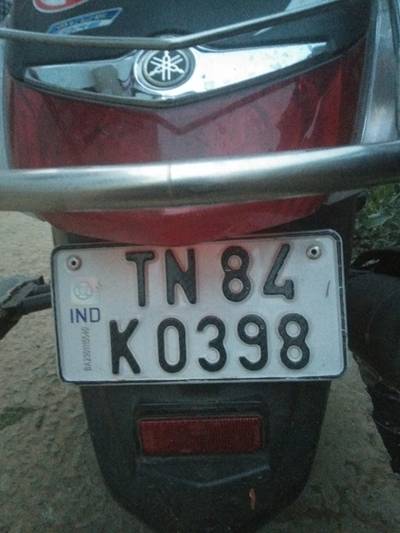
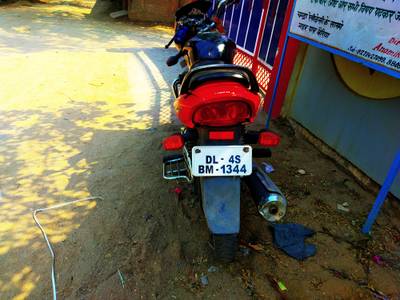
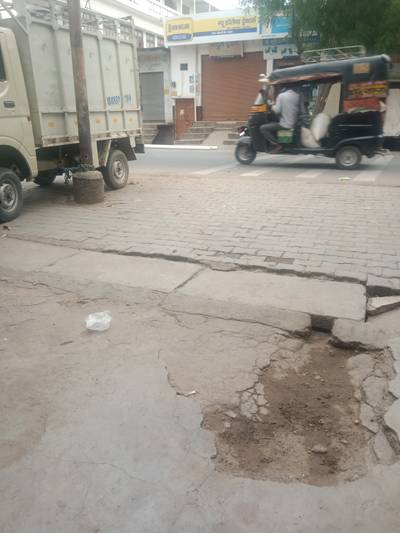

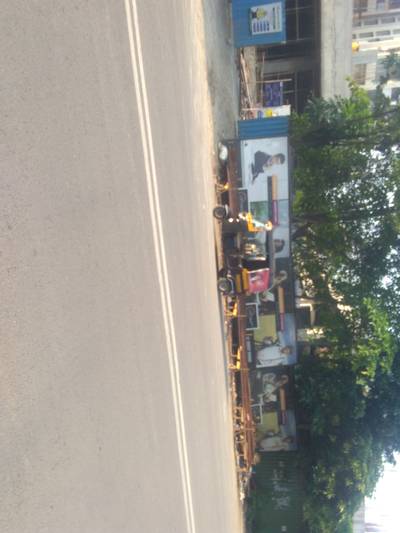


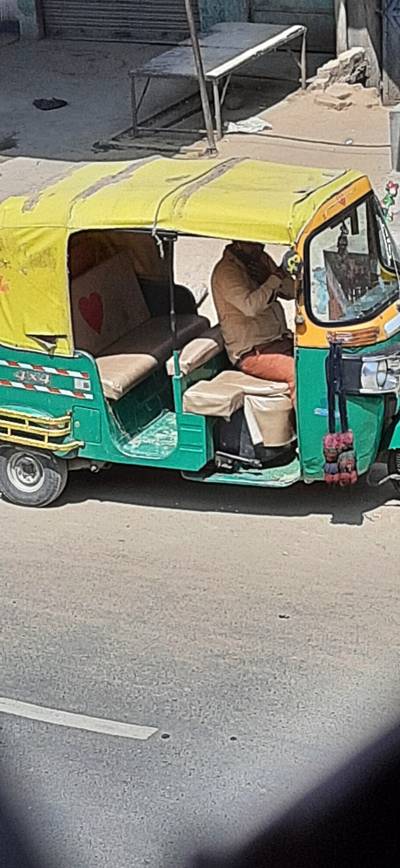
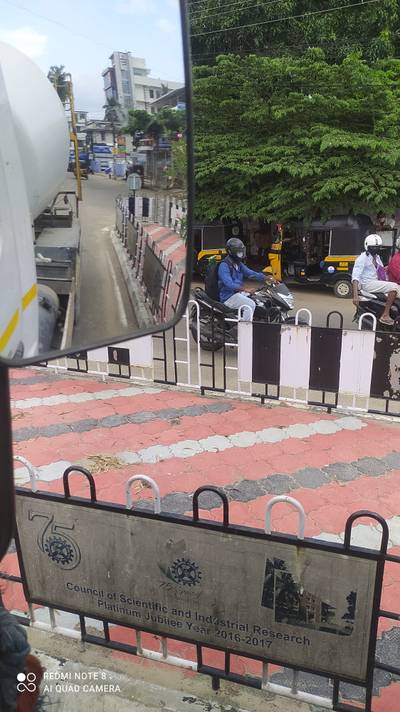
Class balance #
There are 8 annotation classes in the dataset. Find the general statistics and balances for every class in the table below. Click any row to preview images that have labels of the selected class. Sort by column to find the most rare or prevalent classes.
Class ã…¤ | Images ã…¤ | Objects ã…¤ | Count on image average | Area on image average |
---|---|---|---|---|
wheelâž” rectangle | 564 | 1692 | 3 | 3.12% |
2_wheelerâž” rectangle | 425 | 629 | 1.48 | 28.74% |
3_wheelerâž” rectangle | 345 | 432 | 1.25 | 14.88% |
4_wheelerâž” rectangle | 115 | 183 | 1.59 | 6.36% |
6_wheelerâž” rectangle | 34 | 44 | 1.29 | 35.94% |
8_wheelerâž” rectangle | 8 | 9 | 1.12 | 11.68% |
autoâž” rectangle | 3 | 3 | 1 | 40.12% |
10_wheelerâž” rectangle | 1 | 1 | 1 | 14.62% |
Co-occurrence matrix #
Co-occurrence matrix is an extremely valuable tool that shows you the images for every pair of classes: how many images have objects of both classes at the same time. If you click any cell, you will see those images. We added the tooltip with an explanation for every cell for your convenience, just hover the mouse over a cell to preview the description.
Images #
Explore every single image in the dataset with respect to the number of annotations of each class it has. Click a row to preview selected image. Sort by any column to find anomalies and edge cases. Use horizontal scroll if the table has many columns for a large number of classes in the dataset.
Object distribution #
Interactive heatmap chart for every class with object distribution shows how many images are in the dataset with a certain number of objects of a specific class. Users can click cell and see the list of all corresponding images.
Class sizes #
The table below gives various size properties of objects for every class. Click a row to see the image with annotations of the selected class. Sort columns to find classes with the smallest or largest objects or understand the size differences between classes.
Class | Object count | Avg area | Max area | Min area | Min height | Min height | Max height | Max height | Avg height | Avg height | Min width | Min width | Max width | Max width |
---|---|---|---|---|---|---|---|---|---|---|---|---|---|---|
wheel rectangle | 1692 | 1.04% | 32.57% | 0% | 8px | 0.25% | 2258px | 59.43% | 240px | 6.73% | 8px | 0.26% | 2291px | 61.89% |
2_wheeler rectangle | 629 | 19.65% | 100% | 0.02% | 49px | 1.5% | 5760px | 100% | 1165px | 32.74% | 35px | 1.38% | 4320px | 100% |
3_wheeler rectangle | 432 | 11.99% | 99.02% | 0.06% | 86px | 2.4% | 4000px | 100% | 919px | 25.77% | 79px | 2.53% | 3120px | 100% |
4_wheeler rectangle | 183 | 4.14% | 47.2% | 0.06% | 55px | 1.69% | 3211px | 80.28% | 525px | 14.59% | 50px | 2.57% | 2047px | 67.69% |
6_wheeler rectangle | 44 | 27.84% | 100% | 0.25% | 102px | 3.98% | 4160px | 100% | 1414px | 37.84% | 108px | 4.41% | 3008px | 100% |
8_wheeler rectangle | 9 | 10.39% | 32.87% | 0.2% | 127px | 3.89% | 1300px | 37.5% | 693px | 20.27% | 128px | 5.23% | 2146px | 87.66% |
auto rectangle | 3 | 40.12% | 75.9% | 6.29% | 417px | 16.29% | 3522px | 76.43% | 2031px | 52.89% | 741px | 38.59% | 3432px | 99.31% |
10_wheeler rectangle | 1 | 14.62% | 14.62% | 14.62% | 957px | 29.32% | 957px | 29.32% | 957px | 29.32% | 1221px | 49.88% | 1221px | 49.88% |
Spatial Heatmap #
The heatmaps below give the spatial distributions of all objects for every class. These visualizations provide insights into the most probable and rare object locations on the image. It helps analyze objects' placements in a dataset.
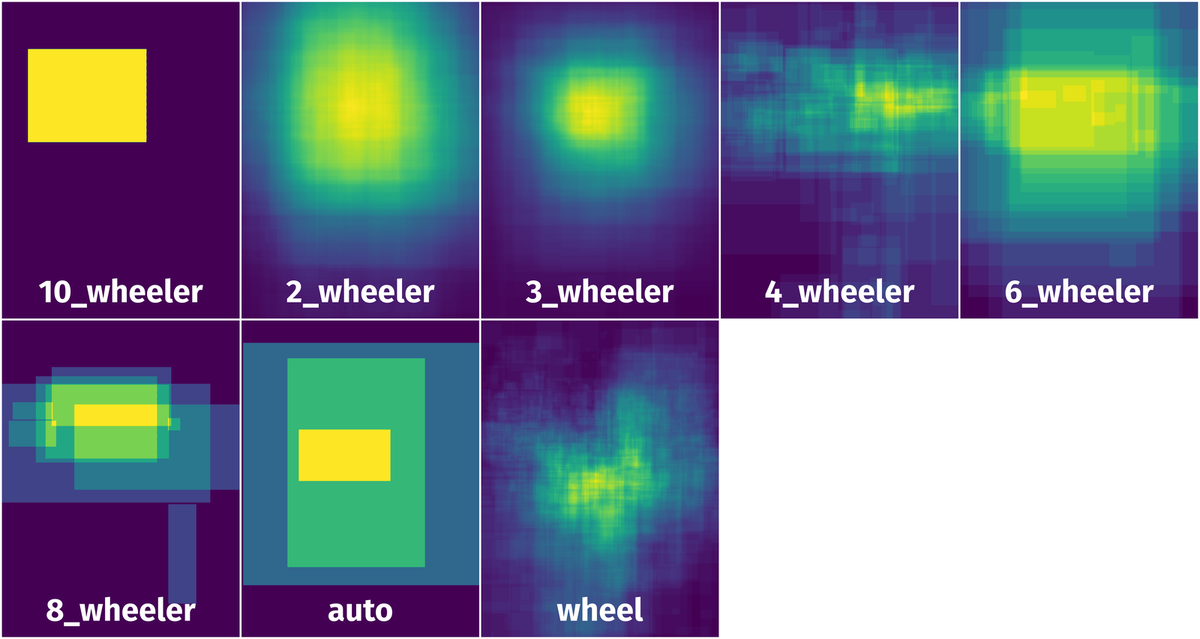
Objects #
Table contains all 2993 objects. Click a row to preview an image with annotations, and use search or pagination to navigate. Sort columns to find outliers in the dataset.
Object ID ã…¤ | Class ã…¤ | Image name click row to open | Image size height x width | Height ã…¤ | Height ã…¤ | Width ã…¤ | Width ã…¤ | Area ã…¤ |
---|---|---|---|---|---|---|---|---|
1âž” | wheel rectangle | 20210430_07_54_03_000_3lfMUU1rI2fHQ2oTEhRa9UMEY8v2_T_2250_4000 (1).jpg | 4000 x 2250 | 769px | 19.23% | 359px | 15.96% | 3.07% |
2âž” | 2_wheeler rectangle | 20210430_07_54_03_000_3lfMUU1rI2fHQ2oTEhRa9UMEY8v2_T_2250_4000 (1).jpg | 4000 x 2250 | 2329px | 58.23% | 1385px | 61.56% | 35.84% |
3âž” | wheel rectangle | 20210503_17_19_21_000_x1Al88rRcbgbIm5Y4D4pjFVpufq2_F_4000_3000.jpg | 4000 x 3000 | 127px | 3.17% | 107px | 3.57% | 0.11% |
4âž” | wheel rectangle | 20210503_17_19_21_000_x1Al88rRcbgbIm5Y4D4pjFVpufq2_F_4000_3000.jpg | 4000 x 3000 | 461px | 11.53% | 322px | 10.73% | 1.24% |
5âž” | 2_wheeler rectangle | 20210503_17_19_21_000_x1Al88rRcbgbIm5Y4D4pjFVpufq2_F_4000_3000.jpg | 4000 x 3000 | 1176px | 29.4% | 673px | 22.43% | 6.6% |
6âž” | wheel rectangle | 20210503_17_19_21_000_x1Al88rRcbgbIm5Y4D4pjFVpufq2_F_4000_3000.jpg | 4000 x 3000 | 121px | 3.02% | 105px | 3.5% | 0.11% |
7âž” | wheel rectangle | 20210503_17_19_21_000_x1Al88rRcbgbIm5Y4D4pjFVpufq2_F_4000_3000.jpg | 4000 x 3000 | 68px | 1.7% | 97px | 3.23% | 0.05% |
8âž” | 4_wheeler rectangle | 20210503_17_19_21_000_x1Al88rRcbgbIm5Y4D4pjFVpufq2_F_4000_3000.jpg | 4000 x 3000 | 2668px | 66.7% | 672px | 22.4% | 14.94% |
9âž” | 3_wheeler rectangle | 20210503_17_19_21_000_x1Al88rRcbgbIm5Y4D4pjFVpufq2_F_4000_3000.jpg | 4000 x 3000 | 624px | 15.6% | 824px | 27.47% | 4.28% |
10âž” | 4_wheeler rectangle | 20210503_17_19_21_000_x1Al88rRcbgbIm5Y4D4pjFVpufq2_F_4000_3000.jpg | 4000 x 3000 | 441px | 11.03% | 746px | 24.87% | 2.74% |
License #
Citation #
If you make use of the Vehicle Wheel Detection data, please cite the following reference:
@dataset{Vehicle Wheel Detection,
author={DataCluster Labs},
title={Vehicle Wheel Detection | Axle Detection},
year={2023},
url={https://www.kaggle.com/datasets/dataclusterlabs/vehicle-wheel-detection}
}
If you are happy with Dataset Ninja and use provided visualizations and tools in your work, please cite us:
@misc{ visualization-tools-for-vehicle-wheel-detection-dataset,
title = { Visualization Tools for Vehicle Wheel Detection Dataset },
type = { Computer Vision Tools },
author = { Dataset Ninja },
howpublished = { \url{ https://datasetninja.com/vehicle-wheel-detection } },
url = { https://datasetninja.com/vehicle-wheel-detection },
journal = { Dataset Ninja },
publisher = { Dataset Ninja },
year = { 2025 },
month = { jul },
note = { visited on 2025-07-26 },
}
Download #
Dataset Vehicle Wheel Detection can be downloaded in Supervisely format:
As an alternative, it can be downloaded with dataset-tools package:
pip install --upgrade dataset-tools
… using following python code:
import dataset_tools as dtools
dtools.download(dataset='Vehicle Wheel Detection', dst_dir='~/dataset-ninja/')
Make sure not to overlook the python code example available on the Supervisely Developer Portal. It will give you a clear idea of how to effortlessly work with the downloaded dataset.
The data in original format can be downloaded here.
Disclaimer #
Our gal from the legal dep told us we need to post this:
Dataset Ninja provides visualizations and statistics for some datasets that can be found online and can be downloaded by general audience. Dataset Ninja is not a dataset hosting platform and can only be used for informational purposes. The platform does not claim any rights for the original content, including images, videos, annotations and descriptions. Joint publishing is prohibited.
You take full responsibility when you use datasets presented at Dataset Ninja, as well as other information, including visualizations and statistics we provide. You are in charge of compliance with any dataset license and all other permissions. You are required to navigate datasets homepage and make sure that you can use it. In case of any questions, get in touch with us at hello@datasetninja.com.