Introduction #
The authors of the WIDER FACE dataset delve into the extensively researched domain of face detection in the field of computer vision. They selected a set of 32,203 images and labeled a total of 393,703 faces. The dataset is structured according to 61 distinct event classes. Within each event class, we adopted a random split of 40% for train, 10% for val, and 50% for test. The authors mention that a discrepancy exists between the current performance of face detection systems and the actual demands of real-world scenarios. To bridge this gap and to propel future face detection research, the authors introduce the WIDER FACE dataset, a substantial expansion over existing datasets with a tenfold increase in scale. This dataset is rich in annotations, incorporating attributes such as occlusions, poses, event categories, and bounding boxes for faces.
The dataset’s content includes faces that pose extreme challenges due to considerable variations in scale, pose, and occlusion. Furthermore, the authors demonstrate the efficacy of the WIDER FACE dataset as a robust training source for advancing face detection research. They evaluate several representative face detection systems, thereby offering an overview of the current state-of-the-art performance. Additionally, the authors propose a solution to address the challenge of accommodating large-scale variations. Lastly, they explore common failure cases that warrant further investigation.
The process of face detection is pivotal for various facial analysis algorithms, encompassing tasks such as face alignment, face recognition, face verification, and face parsing. The core objective of face detection is to discern the presence of faces within an image, and if detected, to localize the position and extent of each face. This seemingly straightforward task for humans proves to be immensely challenging for computational systems. The complexity associated with face detection stems from variations in factors such as pose, scale, facial expression, occlusion, blur, and illumination.
The WIDER FACE dataset, currently the most extensive face detection dataset, is chosen from the publicly accessible WIDER dataset. This dataset selection encompasses 32,203 images and encompasses 393,703 labeled faces. Organized into 60 event classes, the dataset’s images are divided into train, val, and test subsets for each event class. Two specific training/testing scenarios are outlined: Scenario-Ext, where an external face detector is trained and tested on the WIDER FACE test partition, and Scenario-Int, where a face detector is trained using the WIDER FACE training/validation partitions and subsequently tested on the WIDER FACE test partition. The evaluation metric follows that of the PASCAL VOC dataset.
The collection methodology for the WIDER FACE dataset is a subset of the larger WIDER dataset. Images were acquired through a multi-step process, involving the definition of event categories based on the Large Scale Ontology for Multimedia (LSCOM), retrieval of images using search engines, and manual cleaning of the dataset. The data annotation process involves bounding box labeling for all recognizable faces within the dataset. The bounding boxes are required to encompass the forehead, chin, and cheeks. Even when a face is occluded, it is labeled with a bounding box, along with an estimate of the occlusion scale. Faces deemed challenging for recognition due to low resolution and small scale receive an invalid flag. After annotating bounding boxes, attributes such as pose and occlusion level are further annotated. These annotations are conducted by a single annotator and cross-validated by two different individuals.
The distinctive properties of the WIDER FACE dataset present notable challenges due to the extensive variations in scale, occlusion, pose, and background complexity. These characteristics mirror the real-world requirements, providing a benchmark that’s more reflective of practical scenarios. The authors adopt generic object proposal techniques to assess the dataset’s difficulty and potential detection performance. This assessment reveals a lower detection rate for WIDER FACE compared to other face detection datasets, indicating its higher level of challenge. The dataset is classified into three difficulty levels: “Easy”, “Medium”, and “Hard”, based on the detection rate.
Summary #
WIDER FACE: A Face Detection Benchmark is a dataset for an object detection task. Possible applications of the dataset could be in the surveillance industry.
The dataset consists of 32203 images with 199130 labeled objects belonging to 1 single class (face).
Images in the WIDER FACE dataset have bounding box annotations. There are 16097 (50% of the total) unlabeled images (i.e. without annotations). There are 3 splits in the dataset: test (16097 images), train (12880 images), and val (3226 images). Alternatively, images could be split into 60 event categories, while every object contains information about its pose, facial expression, occlusion, blur, and illumination. Objects with low resolution and small scale receive an invalid flag. The dataset was released in 2016 by the Chinese University of Hong Kong and Shenzhen Institutes of Advanced Technology, China.
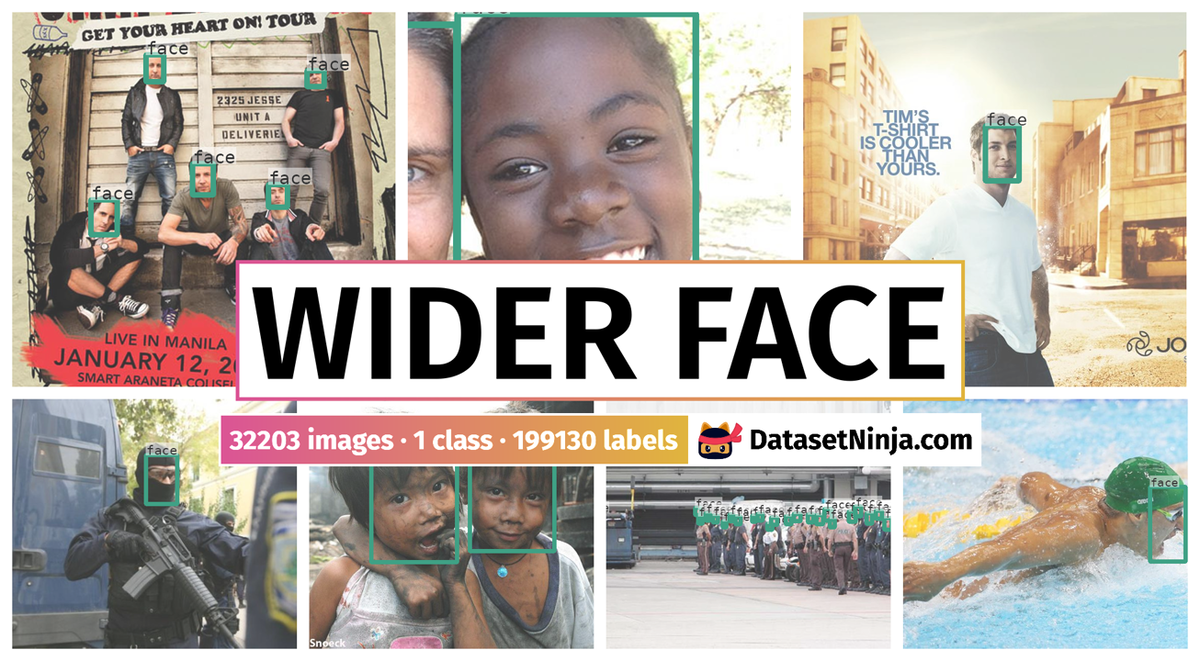
Explore #
WIDER FACE dataset has 32203 images. Click on one of the examples below or open "Explore" tool anytime you need to view dataset images with annotations. This tool has extended visualization capabilities like zoom, translation, objects table, custom filters and more. Hover the mouse over the images to hide or show annotations.
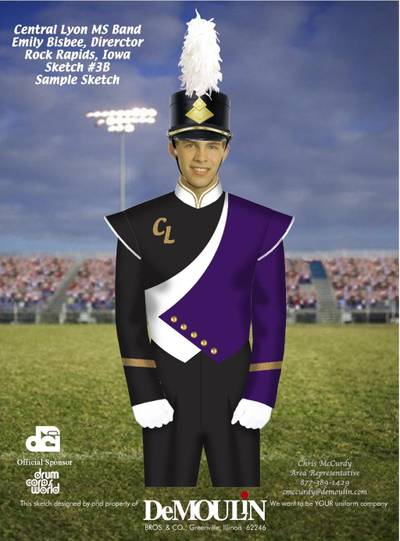
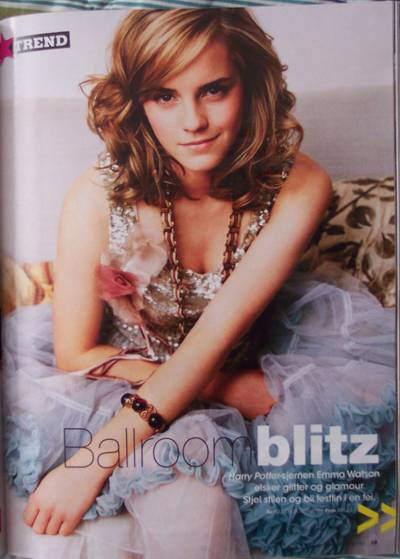
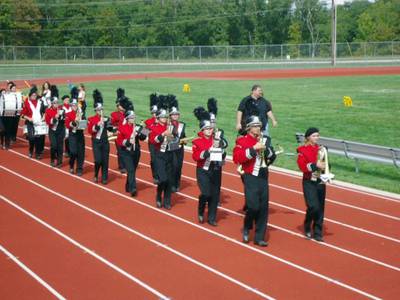
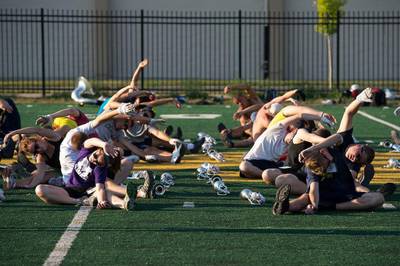

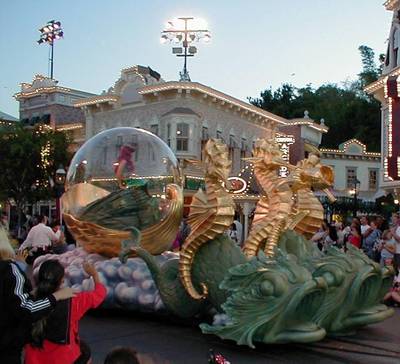
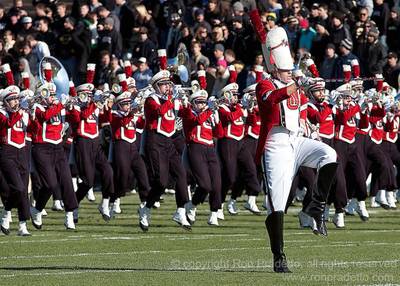
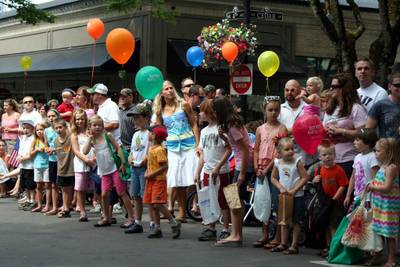
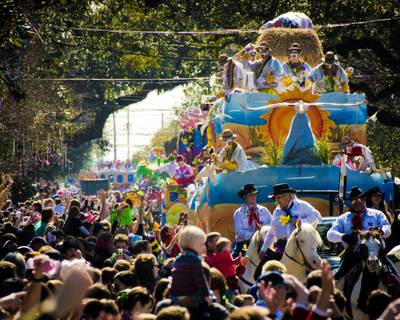
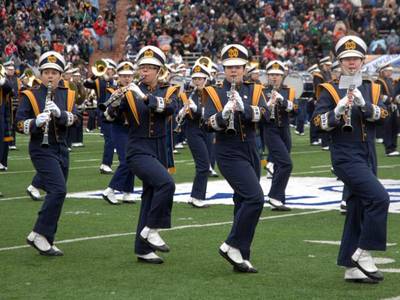
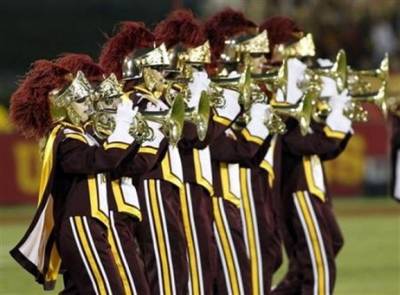

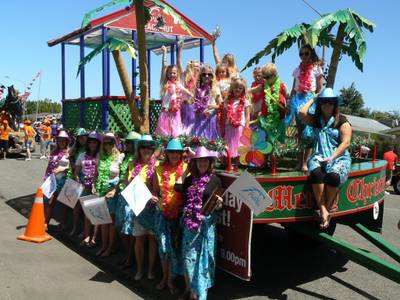
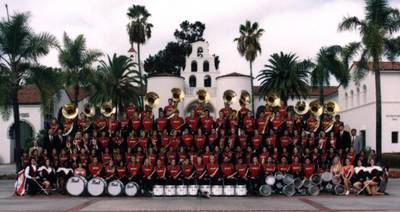
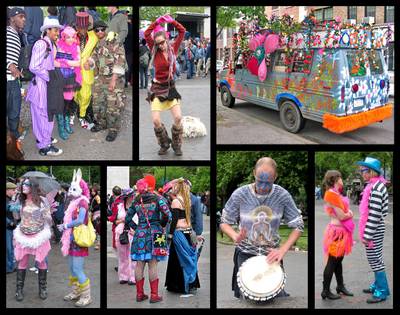
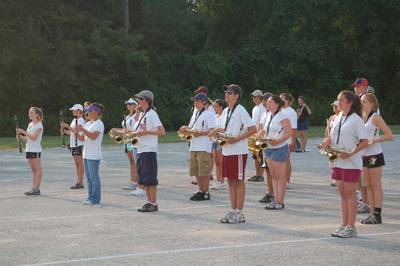
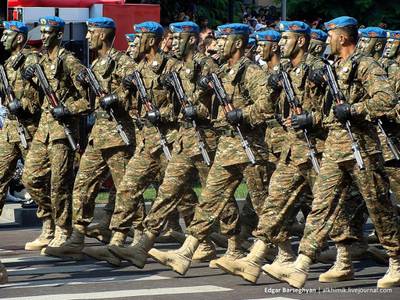
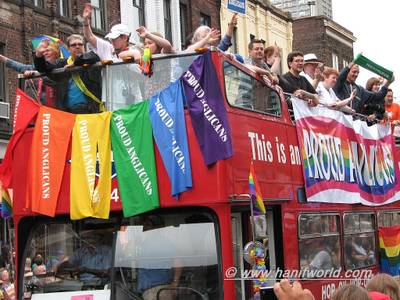
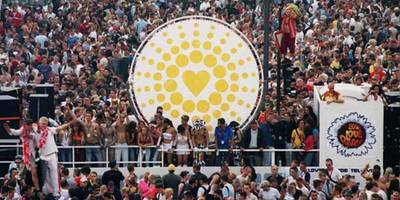
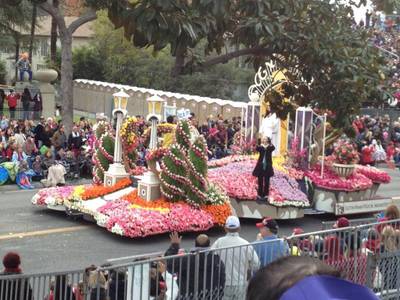
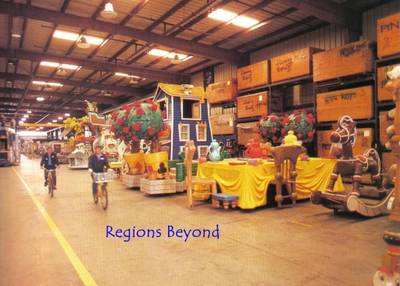
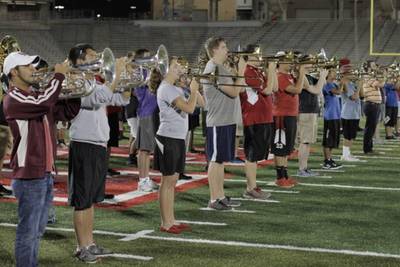
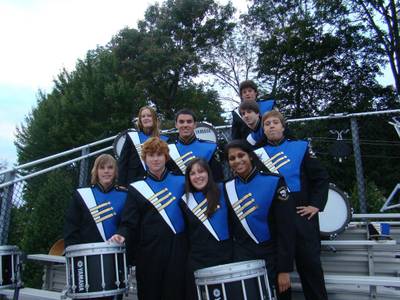
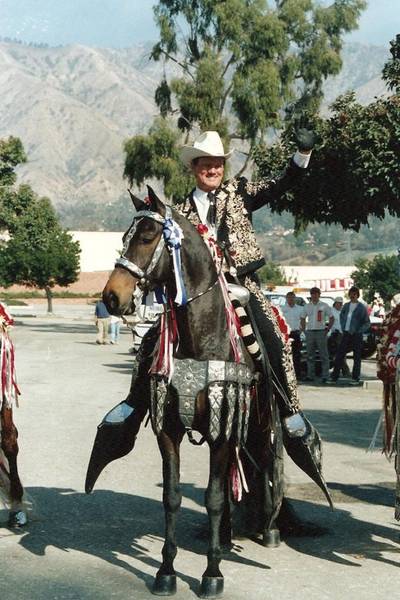
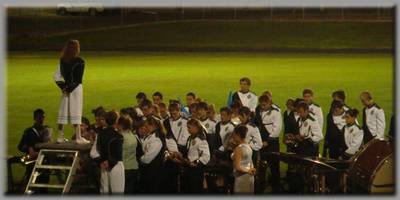
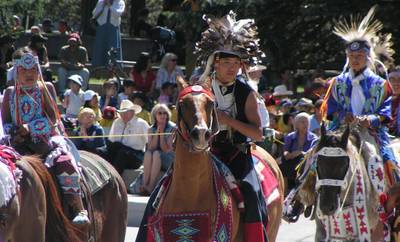
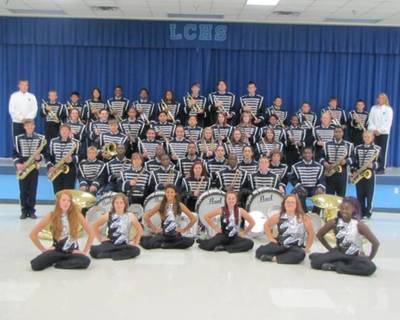
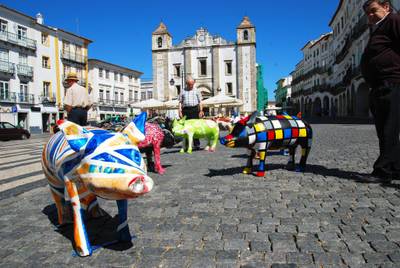
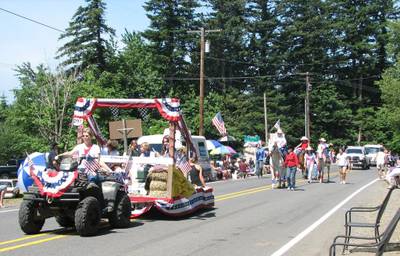
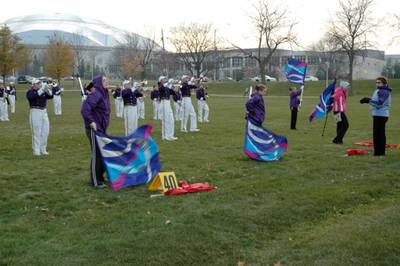
Class balance #
There are 1 annotation classes in the dataset. Find the general statistics and balances for every class in the table below. Click any row to preview images that have labels of the selected class. Sort by column to find the most rare or prevalent classes.
Class ㅤ | Images ㅤ | Objects ㅤ | Count on image average | Area on image average |
---|---|---|---|---|
face➔ rectangle | 16106 | 199130 | 12.36 | 5.05% |
Images #
Explore every single image in the dataset with respect to the number of annotations of each class it has. Click a row to preview selected image. Sort by any column to find anomalies and edge cases. Use horizontal scroll if the table has many columns for a large number of classes in the dataset.
Class sizes #
The table below gives various size properties of objects for every class. Click a row to see the image with annotations of the selected class. Sort columns to find classes with the smallest or largest objects or understand the size differences between classes.
Class | Object count | Avg area | Max area | Min area | Min height | Min height | Max height | Max height | Avg height | Avg height | Min width | Min width | Max width | Max width |
---|---|---|---|---|---|---|---|---|---|---|---|---|---|---|
face rectangle | 199130 | 0.41% | 86.03% | 0% | 1px | 0.07% | 1289px | 100% | 38px | 4.94% | 1px | 0.1% | 1009px | 98.54% |
Spatial Heatmap #
The heatmaps below give the spatial distributions of all objects for every class. These visualizations provide insights into the most probable and rare object locations on the image. It helps analyze objects' placements in a dataset.
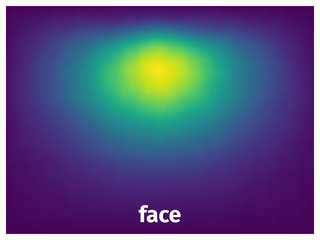
Objects #
Table contains all 102041 objects. Click a row to preview an image with annotations, and use search or pagination to navigate. Sort columns to find outliers in the dataset.
Object ID ㅤ | Class ㅤ | Image name click row to open | Image size height x width | Height ㅤ | Height ㅤ | Width ㅤ | Width ㅤ | Area ㅤ |
---|---|---|---|---|---|---|---|---|
1➔ | face rectangle | 0_Parade_Parade_0_522.jpg | 1539 x 1024 | 238px | 15.46% | 175px | 17.09% | 2.64% |
2➔ | face rectangle | 0_Parade_Parade_0_522.jpg | 1539 x 1024 | 157px | 10.2% | 109px | 10.64% | 1.09% |
3➔ | face rectangle | 10_People_Marching_People_Marching_2_701.jpg | 768 x 1024 | 79px | 10.29% | 53px | 5.18% | 0.53% |
4➔ | face rectangle | 10_People_Marching_People_Marching_2_701.jpg | 768 x 1024 | 75px | 9.77% | 63px | 6.15% | 0.6% |
5➔ | face rectangle | 10_People_Marching_People_Marching_2_701.jpg | 768 x 1024 | 59px | 7.68% | 55px | 5.37% | 0.41% |
6➔ | face rectangle | 10_People_Marching_People_Marching_2_701.jpg | 768 x 1024 | 75px | 9.77% | 53px | 5.18% | 0.51% |
7➔ | face rectangle | 10_People_Marching_People_Marching_2_701.jpg | 768 x 1024 | 83px | 10.81% | 57px | 5.57% | 0.6% |
8➔ | face rectangle | 10_People_Marching_People_Marching_2_701.jpg | 768 x 1024 | 91px | 11.85% | 57px | 5.57% | 0.66% |
9➔ | face rectangle | 58_Hockey_icehockey_puck_58_408.jpg | 1228 x 1024 | 116px | 9.45% | 80px | 7.81% | 0.74% |
10➔ | face rectangle | 58_Hockey_icehockey_puck_58_408.jpg | 1228 x 1024 | 102px | 8.31% | 85px | 8.3% | 0.69% |
License #
WIDER FACE: A Face Detection Benchmark is under CC BY-NC-ND 2.0 license.
Citation #
If you make use of the WIDER FACE data, please cite the following reference:
@inproceedings{yang2016wider,
Author = {Yang, Shuo and Luo, Ping and Loy, Chen Change and Tang, Xiaoou},
Booktitle = {IEEE Conference on Computer Vision and Pattern Recognition (CVPR)},
Title = {WIDER FACE: A Face Detection Benchmark},
Year = {2016}
}
If you are happy with Dataset Ninja and use provided visualizations and tools in your work, please cite us:
@misc{ visualization-tools-for-wider-face-dataset,
title = { Visualization Tools for WIDER FACE Dataset },
type = { Computer Vision Tools },
author = { Dataset Ninja },
howpublished = { \url{ https://datasetninja.com/wider-face } },
url = { https://datasetninja.com/wider-face },
journal = { Dataset Ninja },
publisher = { Dataset Ninja },
year = { 2025 },
month = { apr },
note = { visited on 2025-04-24 },
}
Download #
Dataset WIDER FACE can be downloaded in Supervisely format:
As an alternative, it can be downloaded with dataset-tools package:
pip install --upgrade dataset-tools
… using following python code:
import dataset_tools as dtools
dtools.download(dataset='WIDER FACE', dst_dir='~/dataset-ninja/')
Make sure not to overlook the python code example available on the Supervisely Developer Portal. It will give you a clear idea of how to effortlessly work with the downloaded dataset.
The data in original format can be downloaded here:
Disclaimer #
Our gal from the legal dep told us we need to post this:
Dataset Ninja provides visualizations and statistics for some datasets that can be found online and can be downloaded by general audience. Dataset Ninja is not a dataset hosting platform and can only be used for informational purposes. The platform does not claim any rights for the original content, including images, videos, annotations and descriptions. Joint publishing is prohibited.
You take full responsibility when you use datasets presented at Dataset Ninja, as well as other information, including visualizations and statistics we provide. You are in charge of compliance with any dataset license and all other permissions. You are required to navigate datasets homepage and make sure that you can use it. In case of any questions, get in touch with us at hello@datasetninja.com.