Introduction #
The Large Dataset of Labeled Optical Coherence Tomography (OCT) and Chest X-Ray Images encountered challenges related to reliability and interpretability in implementing clinical-decision support algorithms for medical imaging. They embarked on developing a diagnostic tool utilizing a deep-learning framework specifically designed for screening patients with common treatable blinding retinal diseases. The final OCT dataset contains 109,309 images.
The full dataset consists of the following parts:
- ZhangLabData: OCT (current)
- ZhangLabData: Chest X-Ray (available on DatasetNinja)
Spectral-Domain OCT Imaging
The primary application was in the diagnosis of retinal OCT images. Spectral-domain OCT captures high-resolution optical cross sections of the retina, assembling them into three-dimensional-volume images. It has become a widely performed medical imaging procedure, particularly in diagnosing age-related macular degeneration (DRUSEN, choroidal neovascularization CNV) and diabetic macular edema (DME).
OCT imaging has become a standard of care in guiding the diagnosis and treatment of leading causes of blindness, including AMD and diabetic macular edema. The prevalence of these diseases is significant, with millions affected, and the utilization of anti-vascular endothelial growth factor (anti-VEGF) medications has revolutionized treatment.
Dataset Details and Training Outcome
The authors obtained 207,130 OCT images initially, with 108,312 images passing quality review for training the AI system. Testing involved 1,000 images from 633 patients. After 100 epochs, training was halted due to the absence of further improvement in both accuracy and cross-entropy loss.
An independent test set of 1,000 images was used to compare the AI networkās referral decisions with those of human experts. The AI systemās performance was comparable to human experts in distinguishing patients needing urgent referral.
The authors conducted an occlusion test on 491 images to identify areas contributing most to the neural networkās assignment of the predicted diagnosis. The testing successfully identified regions of interest, demonstrating high accuracy in recognizing clinically significant areas of pathology.
Summary #
Large Dataset of Labeled Optical Coherence Tomography (OCT) and Chest X-Ray Images is a dataset for a classification task. It is used in the medical industry.
The dataset consists of 109309 images with 0 labeled objects. There are 2 splits in the dataset: train (108309 images) and test (1000 images). Alternatively, the dataset could be split into 4 classification splits: NORMAL (51390 images), CNV (37455 images), DME (11598 images), and DRUSEN (8866 images). The dataset was released in 2018 by the Zhang Lab, Univercity of San Diego, USA.
Here are the visualized examples for the classes:
Explore #
ZhangLabData: OCT dataset has 109309 images. Click on one of the examples below or open "Explore" tool anytime you need to view dataset images with annotations. This tool has extended visualization capabilities like zoom, translation, objects table, custom filters and more. Hover the mouse over the images to hide or show annotations.
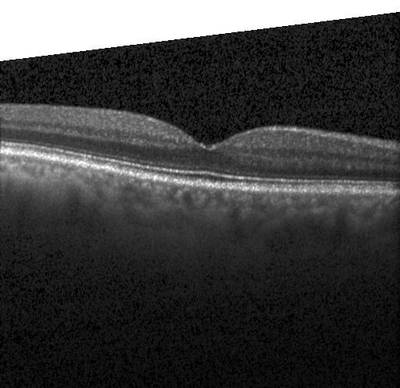
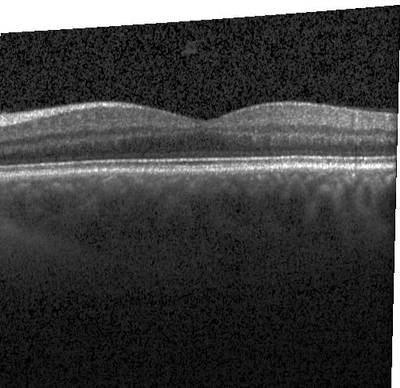





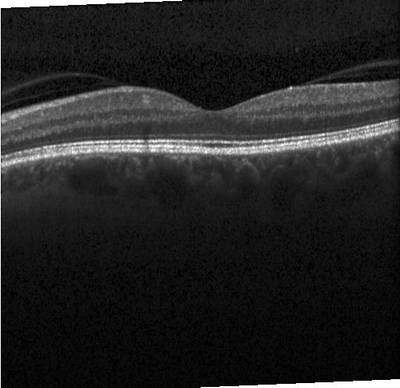

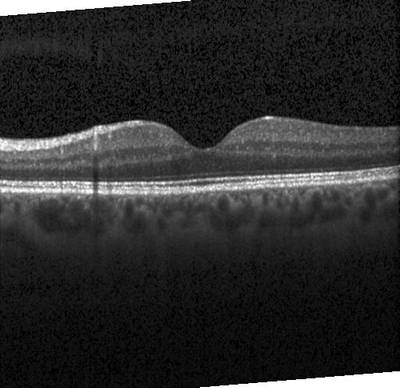


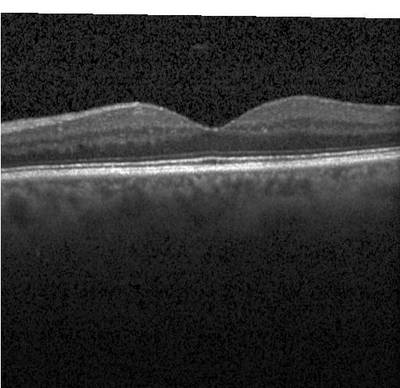

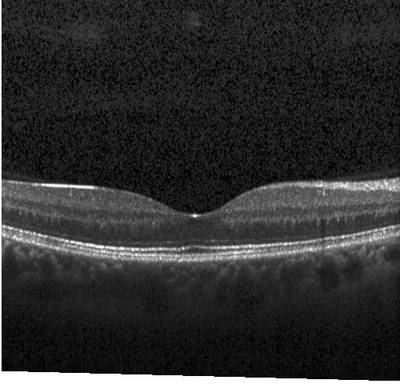

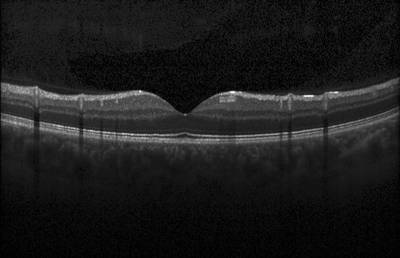


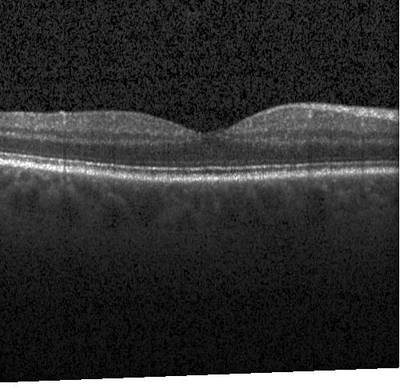

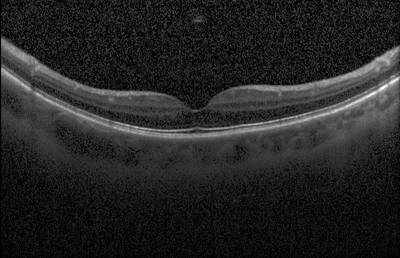
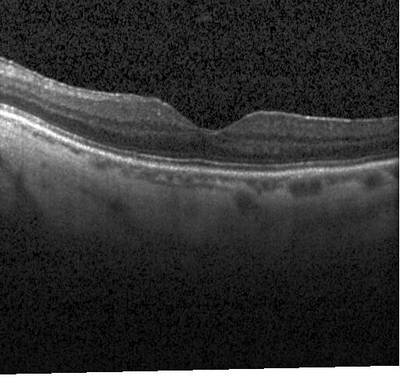





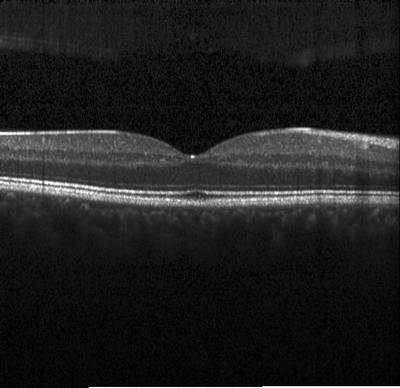
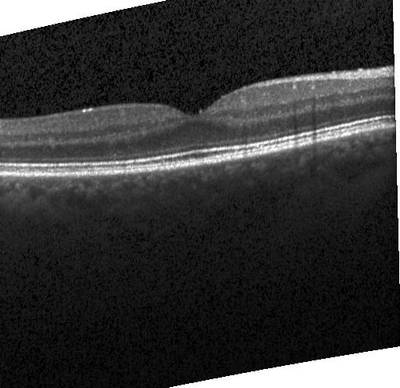
License #
Citation #
If you make use of the ZhangLabData: OCT data, please cite the following reference:
Kermany, Daniel; Zhang, Kang; Goldbaum, Michael (2018),
āLarge Dataset of Labeled Optical Coherence Tomography (OCT) and Chest X-Ray Imagesā,
Mendeley Data, V3, doi: 10.17632/rscbjbr9sj.3
If you are happy with Dataset Ninja and use provided visualizations and tools in your work, please cite us:
@misc{ visualization-tools-for-zhang-lab-data-oct-dataset,
title = { Visualization Tools for ZhangLabData: OCT Dataset },
type = { Computer Vision Tools },
author = { Dataset Ninja },
howpublished = { \url{ https://datasetninja.com/zhang-lab-data-oct } },
url = { https://datasetninja.com/zhang-lab-data-oct },
journal = { Dataset Ninja },
publisher = { Dataset Ninja },
year = { 2025 },
month = { jun },
note = { visited on 2025-06-20 },
}
Download #
Dataset ZhangLabData: OCT can be downloaded in Supervisely format:
As an alternative, it can be downloaded with dataset-tools package:
pip install --upgrade dataset-tools
⦠using following python code:
import dataset_tools as dtools
dtools.download(dataset='ZhangLabData: OCT', dst_dir='~/dataset-ninja/')
Make sure not to overlook the python code example available on the Supervisely Developer Portal. It will give you a clear idea of how to effortlessly work with the downloaded dataset.
The data in original format can be downloaded here.
Disclaimer #
Our gal from the legal dep told us we need to post this:
Dataset Ninja provides visualizations and statistics for some datasets that can be found online and can be downloaded by general audience. Dataset Ninja is not a dataset hosting platform and can only be used for informational purposes. The platform does not claim any rights for the original content, including images, videos, annotations and descriptions. Joint publishing is prohibited.
You take full responsibility when you use datasets presented at Dataset Ninja, as well as other information, including visualizations and statistics we provide. You are in charge of compliance with any dataset license and all other permissions. You are required to navigate datasets homepage and make sure that you can use it. In case of any questions, get in touch with us at hello@datasetninja.com.