Introduction #
The authors introduce the FGVD: Fine-Grained Vehicle Detection Dataset, captured from a moving camera mounted on a car. It contains 5502 scene images with 210 unique fine-grained labels of multiple vehicle types organized in a three-level hierarchy. While previous classification datasets also include makes for different kinds of cars, the FGVD dataset introduces new class labels for categorizing two-wheelers, autorickshaws, and trucks. The FGVD dataset is challenging as it has vehicles in complex traffic scenarios with intra-class and inter-class variations.
Note, similar FGVD: Fine-Grained Vehicle Detection Dataset datasets are also available on the DatasetNinja.com:
Motivation
Intelligent traffic monitoring systems are of utmost need in big cities for public security, planning, and surveillance. For the tasks like vehicle re-identification and robust detection (e.g., when a vehicle occludes another vehicle that is similar in appearance), the detectors used in the surveillance systems should finely classify the vehicle type, manufacturer, and model of the on-road vehicles. Conventionally, detection models are trained to classify vehicles based on coarse categories of on-road datasets. A coarse class can contain multiple sub-classes with minute variations, referred to as the fine-grained classes. The localization of such sub-class vehicles based on their granularity in the design is known as Fine-Grained Vehicle Detection. The FGVD models and datasets can enable robust vehicle re-identification and detection in highly dense and occluded traffic scenarios.
Dataset description
The authors propose a novel FGVD dataset with multiple hierarchy levels for the fine-grained labels. In addition to enabling the detection task, the FGVD dataset includes complex intra-class and inter-class variations in types, scales, and orientations compared to the previous fine-grained classification datasets.
Fine-grained datasets samples. Top: Previous datasets focus only on the classification of cars on vehicle centric images. Middle: The proposed FGVD dataset enables fine-grained (multi) vehicle detection on unconstrained road scenes captured from vehicle-mounted cameras. Bottom left GradCAM++ visualizations on predicted crops show that the model focuses on the backlight and blinker at the motorcycle’s top and scooter’s bottom. For Tavera, the design on the left and right of license plates, and for Ciaz, the radiator and headlight regions are highlighted classification features.
The dataset also contains challenging occlusion scenarios and lighting conditions. FGVD comprises three levels of hierarchy, i.e., vehicle type, manufacturer, and model.
Sample images of different categories in FGVD exhibiting inter-class similarities (bottom two rows), multiple vehicle orientations (all rows), and frequent occlusions.
The authors introduce the complementary hierarchical labels for two-wheelers, autorickshaws, trucks, and buses. The granularity of the FGVD dataset increases as they move from parent to child level. For the hierarchical FGVD dataset, every level has its uniqueness. Firstly, different vehicles may look similar at the first level of granularity, e.g., motorcycles and scooters, both being the two-wheelers. However, the overall design of the scooter is different from the motorcycle, e.g., scooters have a backlight at the bottom as compared to the top backlight of the motorcycle. The overall appearance of the two vehicles with the same parent may look even more similar. However, the minute subtle and local differences are present in the same subcategory. Also, some categories are not present in the earlier vehicle datasets, for example, scooter, autorickshaw, truck, and bus; hence, they must be added to a fine-grained dataset. Therefore, the authors introduce classes unique to the FGVD dataset to facilitate detailed research in fine-grained on-road scenarios.
Dataset acquisition
The authors utilize images paired with coarse labels and bounding boxes sourced from the IDD detection dataset. However, annotating vehicles situated far from the camera proves impractical. Consequently, they filter out bounding boxes with height-to-width ratios below specified thresholds, adjusted to accommodate the varying dimensions of vehicle types. To streamline annotation efforts, they set higher threshold values for trucks, followed by cars and bikes. This thresholding approach simplifies the annotators’ task, rendering it more manageable and efficient. The FGVD dataset comprises 5,502 scene images encompassing approximately 24,450 bounding boxes, annotated with 217 fine-grained labels at the third level, including 210 unique labels and 7 repetitions from higher levels.
From the IDD Detection dataset, the authors curate 5,502 high-quality images out of 16,311 based on factors like occlusion, vehicle box size, and traffic density. An annotation team comprising four skilled annotators and two expert reviewers oversees the process, ensuring quality control. Initially, annotators receive training on fine-grained labeling using select FGVD samples. They are then provided with guidelines, templates, and a list of objects for annotation. Following comprehensive training, annotators can identify popular vehicles within scenes. In cases where recognition proves challenging, annotators resort to tools like Google Lens or online image searches. For instance, if a vehicle’s model name is obscured but the brand logo is visible, annotators may search for similar vehicles on the manufacturer’s website to aid identification. Notably, the IDD-Detection dataset incorporates images captured from continuous video frames, establishing a temporal connection. This temporal context aids in labeling vehicles, even when their design is not fully discernible in isolated frames. Annotators leverage information from different frames to propagate labels, enhancing accuracy, particularly in instances of occlusion or truncation.
Annotation Strategy: (b) is the only confidently identifiable image frame where the label cue (logo at bottom-left of the car) is visible. As the frames are from common video sequence, the label is propagated to the vehicle instances in (a), (c) & (d).
The authors implement a two-step approach in the data creation process, consisting of the pilot phase and the takeoff phase. During the pilot phase, each annotator works on a small subset of images characterized by lower traffic density. Additionally, they provide training to annotators on labeling vehicles that are confidently identifiable but lack bounding boxes. For cars, motorcycles, and scooters, a special attribute labeled “new” is introduced to identify new variants encountered in the dataset. Annotators mark this attribute whenever they encounter such variants.
Subsequently, all labels generated during the takeoff stage undergo review. Images with labels marked with high confidence by reviewers are retained for inclusion in the dataset. Any vehicle bounding boxes remaining ambiguous at fine-grained levels are categorized as “others.” Instances where vehicles are partially obscured by materials like covers or cloth are labeled as “covered.”
Classes hierarchy
Each vehicle type in the proposed Fine-Grained Vehicle Detection (FGVD) dataset has different hierarchical levels. The FGVD contains three different levels of hierarchy:
- Vehicle-type: The highest coarse level labels of the vehicle come under the vehicle-type category. The authors consider it as level 1 of the hierarchy. car, motorcycle, scooter, truck, autorickshaw, bus and mini-bus are the six categories present in vehicle type.
- Manufacturer: The manufacturer level contains the primary producer of the vehicles. The manufacturer category has finer details than the vehicle type level. A producer may manufacture multiple kinds of vehicles. For example, Bajaj manufactures motorcycles as well as autorickshaw.
- Model: The model level is at the last group of the hierarchy. This level comprises highly fine-grained features that are unique for the variant. For example, a car’s design must be unique for each manufacturer.
Sample Hierarchy Tree of the FGVD dataset.
Vehicle Type | Levels of Hierarchy | L-2 labels | L-3 labels |
---|---|---|---|
Car | 3 | 22 | 112 |
Motorcycle | 3 | 11 | 67 |
Scooter | 3 | 9 | 23 |
Truck | 2 | 7 | 7 |
Autorickshaw | 2 | 6 | 6 |
Bus | 2 | 2 | 2 |
Total | 3 | 57 | 217 |
Levels of Hierarchy for different Vehicles in FGVD.
Summary #
FGVD: Fine-Grained Vehicle Detection Dataset is a dataset for object detection and identification tasks. It is used in the automotive industry.
The dataset consists of 5502 images with 24450 labeled objects belonging to 7 different classes including car, motorcycle, scooter, and other: autorickshaw, truck, bus, and mini-bus.
Images in the FGVD dataset have bounding box annotations. All images are labeled (i.e. with annotations). There are 3 splits in the dataset: train (3535 images), test (1083 images), and val (884 images). Additionally, labels contain information about manufacturer and model of vehicle. Explore it in supervisely labeling tool. The dataset was released in 2022 by the Indian Institute Of Technology, India and The International Institute of Information Technology, India.
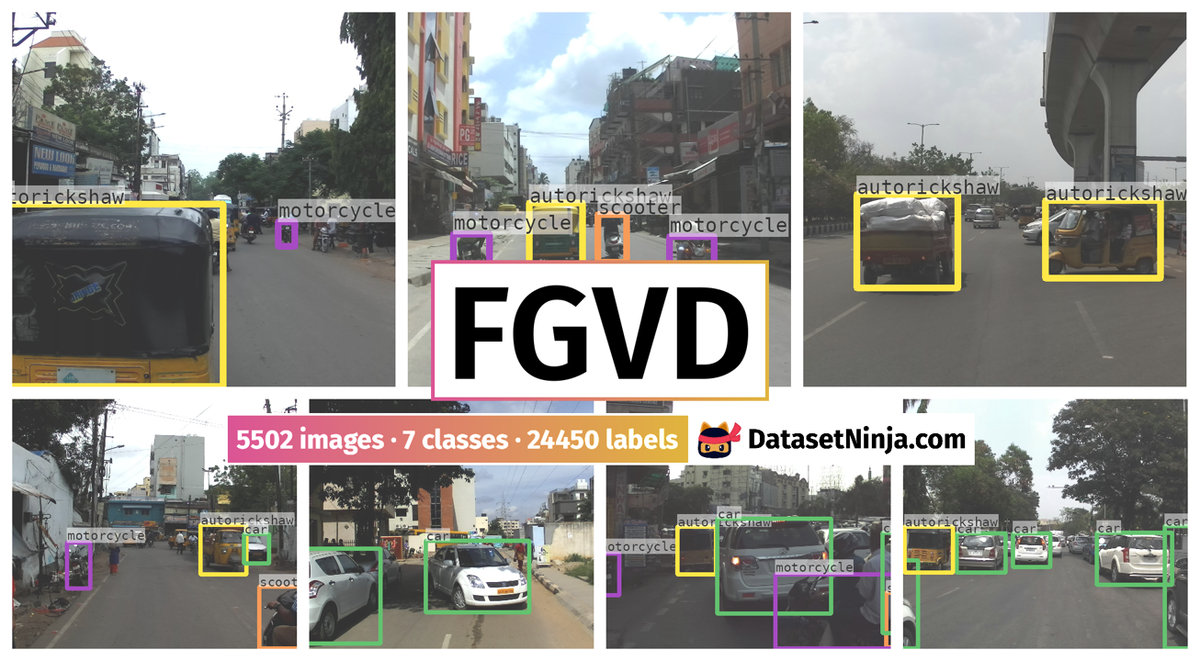
Explore #
FGVD dataset has 5502 images. Click on one of the examples below or open "Explore" tool anytime you need to view dataset images with annotations. This tool has extended visualization capabilities like zoom, translation, objects table, custom filters and more. Hover the mouse over the images to hide or show annotations.
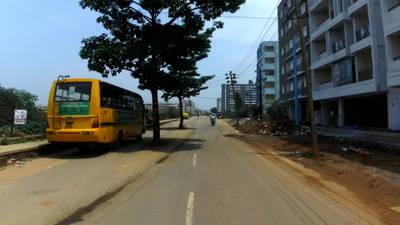
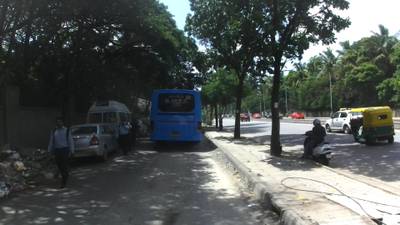
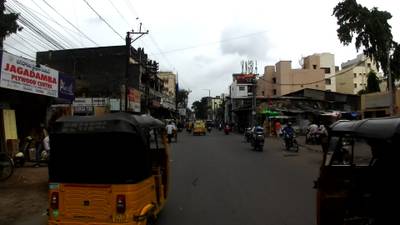
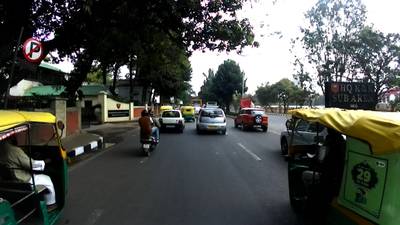
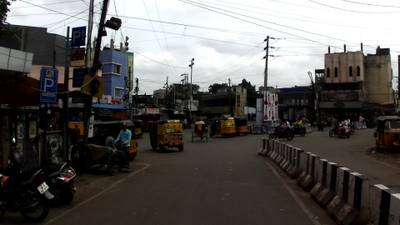

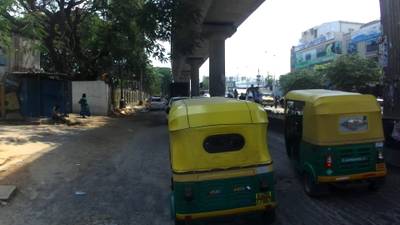
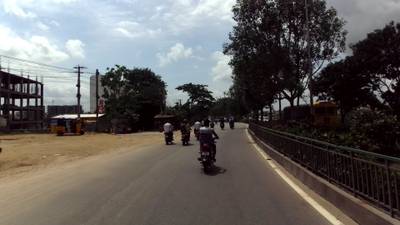
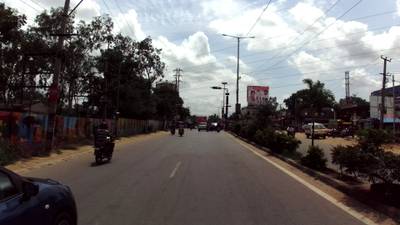
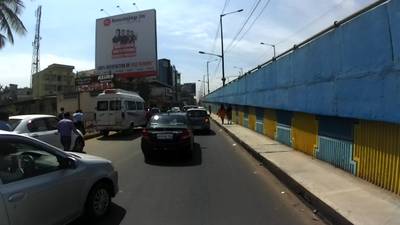
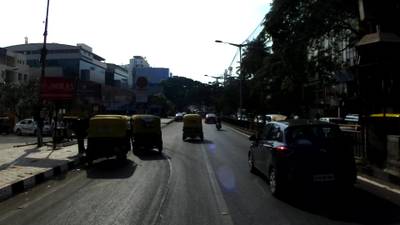

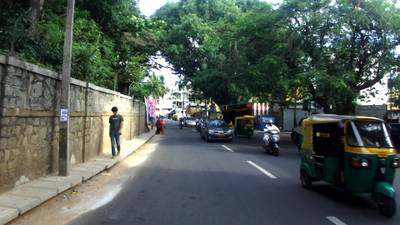

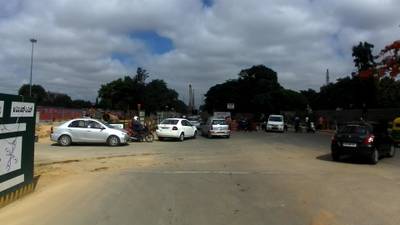
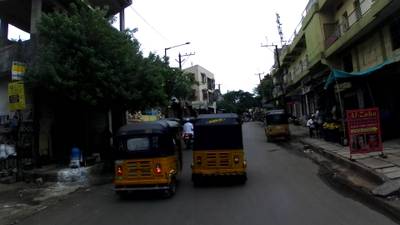
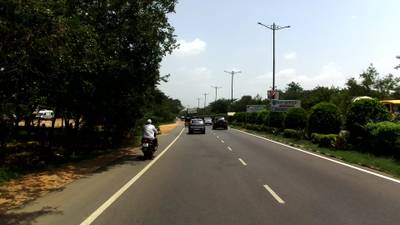
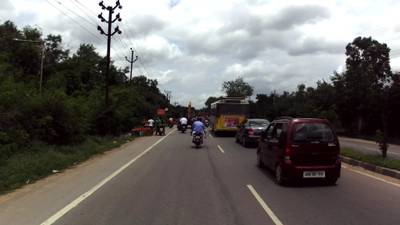
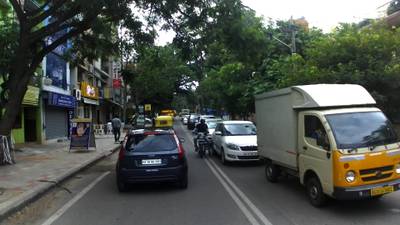

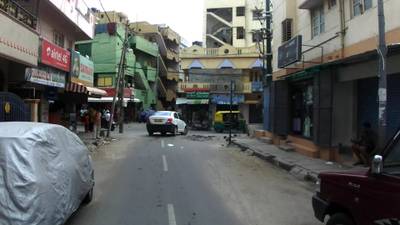

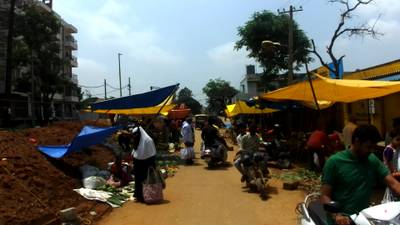
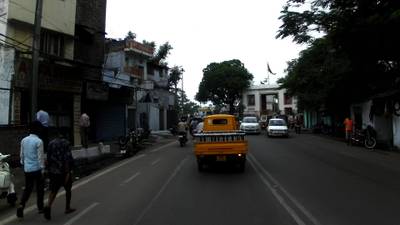
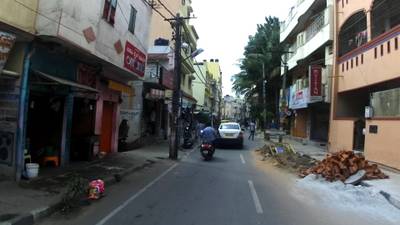
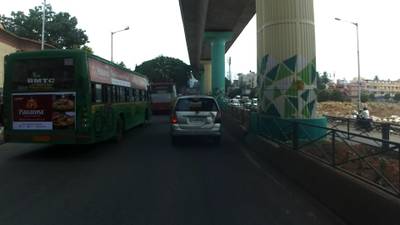
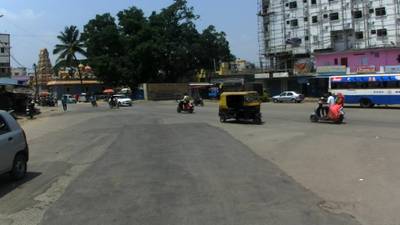
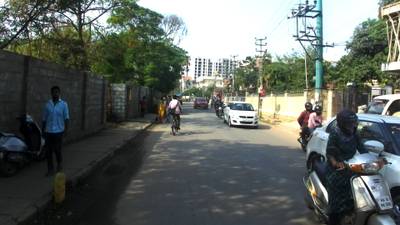
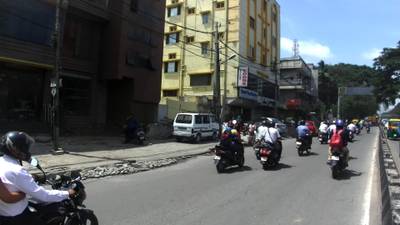
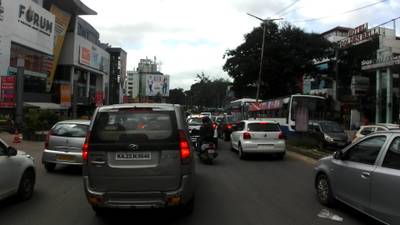
Class balance #
There are 7 annotation classes in the dataset. Find the general statistics and balances for every class in the table below. Click any row to preview images that have labels of the selected class. Sort by column to find the most rare or prevalent classes.
Class ㅤ | Images ㅤ | Objects ㅤ | Count on image average | Area on image average |
---|---|---|---|---|
car➔ rectangle | 3843 | 7951 | 2.07 | 8.84% |
motorcycle➔ rectangle | 3059 | 5293 | 1.73 | 3.28% |
scooter➔ rectangle | 2637 | 4347 | 1.65 | 2.72% |
autorickshaw➔ rectangle | 2314 | 3777 | 1.63 | 7.98% |
truck➔ rectangle | 1311 | 1552 | 1.18 | 10.72% |
bus➔ rectangle | 970 | 1215 | 1.25 | 13.13% |
mini-bus➔ rectangle | 298 | 315 | 1.06 | 6.96% |
Co-occurrence matrix #
Co-occurrence matrix is an extremely valuable tool that shows you the images for every pair of classes: how many images have objects of both classes at the same time. If you click any cell, you will see those images. We added the tooltip with an explanation for every cell for your convenience, just hover the mouse over a cell to preview the description.
Images #
Explore every single image in the dataset with respect to the number of annotations of each class it has. Click a row to preview selected image. Sort by any column to find anomalies and edge cases. Use horizontal scroll if the table has many columns for a large number of classes in the dataset.
Object distribution #
Interactive heatmap chart for every class with object distribution shows how many images are in the dataset with a certain number of objects of a specific class. Users can click cell and see the list of all corresponding images.
Class sizes #
The table below gives various size properties of objects for every class. Click a row to see the image with annotations of the selected class. Sort columns to find classes with the smallest or largest objects or understand the size differences between classes.
Class | Object count | Avg area | Max area | Min area | Min height | Min height | Max height | Max height | Avg height | Avg height | Min width | Min width | Max width | Max width |
---|---|---|---|---|---|---|---|---|---|---|---|---|---|---|
car rectangle | 7951 | 4.43% | 36.92% | 0.01% | 7px | 0.65% | 1040px | 96.3% | 224px | 21.64% | 8px | 0.42% | 1551px | 80.78% |
motorcycle rectangle | 5293 | 1.94% | 17.94% | 0% | 3px | 0.28% | 589px | 54.54% | 198px | 18.56% | 6px | 0.31% | 831px | 43.28% |
scooter rectangle | 4347 | 1.68% | 15.36% | 0.03% | 25px | 2.31% | 506px | 46.85% | 184px | 17.36% | 16px | 0.94% | 811px | 42.24% |
autorickshaw rectangle | 3777 | 5% | 43.73% | 0.01% | 7px | 0.83% | 872px | 80.74% | 292px | 27.73% | 15px | 0.94% | 1369px | 71.3% |
truck rectangle | 1552 | 9.08% | 69.25% | 0.06% | 38px | 3.52% | 1080px | 100% | 391px | 37.06% | 35px | 1.82% | 1343px | 69.91% |
bus rectangle | 1215 | 10.5% | 56.7% | 0.01% | 8px | 0.74% | 1080px | 100% | 399px | 37.63% | 8px | 0.42% | 1178px | 61.35% |
mini-bus rectangle | 315 | 6.6% | 41.39% | 0.04% | 19px | 1.76% | 1026px | 95% | 311px | 29.22% | 31px | 1.61% | 919px | 47.86% |
Spatial Heatmap #
The heatmaps below give the spatial distributions of all objects for every class. These visualizations provide insights into the most probable and rare object locations on the image. It helps analyze objects' placements in a dataset.
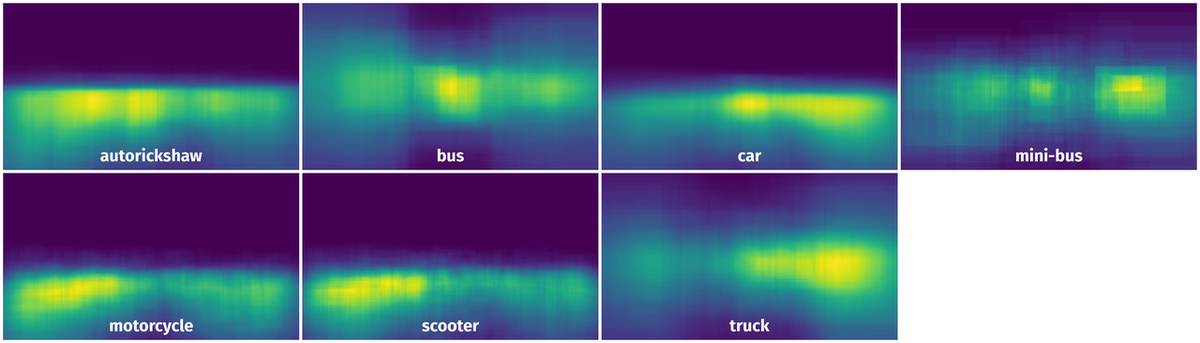
Objects #
Table contains all 24450 objects. Click a row to preview an image with annotations, and use search or pagination to navigate. Sort columns to find outliers in the dataset.
Object ID ㅤ | Class ㅤ | Image name click row to open | Image size height x width | Height ㅤ | Height ㅤ | Width ㅤ | Width ㅤ | Area ㅤ |
---|---|---|---|---|---|---|---|---|
1➔ | motorcycle rectangle | 1307.jpg | 1080 x 1920 | 328px | 30.37% | 287px | 14.95% | 4.54% |
2➔ | car rectangle | 1307.jpg | 1080 x 1920 | 189px | 17.5% | 271px | 14.11% | 2.47% |
3➔ | car rectangle | 1307.jpg | 1080 x 1920 | 232px | 21.48% | 255px | 13.28% | 2.85% |
4➔ | truck rectangle | 1307.jpg | 1080 x 1920 | 686px | 63.52% | 498px | 25.94% | 16.48% |
5➔ | car rectangle | 3503.jpg | 1080 x 1921 | 258px | 23.89% | 246px | 12.81% | 3.06% |
6➔ | mini-bus rectangle | 3503.jpg | 1080 x 1921 | 192px | 17.78% | 303px | 15.77% | 2.8% |
7➔ | car rectangle | 3503.jpg | 1080 x 1921 | 294px | 27.22% | 369px | 19.21% | 5.23% |
8➔ | car rectangle | 3503.jpg | 1080 x 1921 | 159px | 14.72% | 190px | 9.89% | 1.46% |
9➔ | scooter rectangle | 3503.jpg | 1080 x 1921 | 374px | 34.63% | 262px | 13.64% | 4.72% |
10➔ | autorickshaw rectangle | 3503.jpg | 1080 x 1921 | 527px | 48.8% | 312px | 16.24% | 7.93% |
License #
Citation #
If you make use of the FGVD data, please cite the following reference:
@dataset{prafful_kumar_khoba_2022_7488960,
author = {Prafful Kumar Khoba and
Chirag Parikh and
Rohit Saluja and
Ravi Kiran Sarvadevabhatla and
C.V. Jawahar},
title = {{A Fine-Grained Vehicle Detection (FGVD) Dataset
for Unconstrained Roads}},
journal = {Proceedings of the Indian Conference on Computer Vision,
Graphics and Image Processing (ICVGIP)},
month = dec,
year = 2022,
publisher = {Zenodo},
doi = {10.1145/3571600.3571626},
url = {https://doi.org/10.1145/3571600.3571626}
}
If you are happy with Dataset Ninja and use provided visualizations and tools in your work, please cite us:
@misc{ visualization-tools-for-fgvd-dataset,
title = { Visualization Tools for FGVD Dataset },
type = { Computer Vision Tools },
author = { Dataset Ninja },
howpublished = { \url{ https://datasetninja.com/fgvd } },
url = { https://datasetninja.com/fgvd },
journal = { Dataset Ninja },
publisher = { Dataset Ninja },
year = { 2025 },
month = { jun },
note = { visited on 2025-06-12 },
}
Download #
Dataset FGVD can be downloaded in Supervisely format:
As an alternative, it can be downloaded with dataset-tools package:
pip install --upgrade dataset-tools
… using following python code:
import dataset_tools as dtools
dtools.download(dataset='FGVD', dst_dir='~/dataset-ninja/')
Make sure not to overlook the python code example available on the Supervisely Developer Portal. It will give you a clear idea of how to effortlessly work with the downloaded dataset.
The data in original format can be downloaded here.
Disclaimer #
Our gal from the legal dep told us we need to post this:
Dataset Ninja provides visualizations and statistics for some datasets that can be found online and can be downloaded by general audience. Dataset Ninja is not a dataset hosting platform and can only be used for informational purposes. The platform does not claim any rights for the original content, including images, videos, annotations and descriptions. Joint publishing is prohibited.
You take full responsibility when you use datasets presented at Dataset Ninja, as well as other information, including visualizations and statistics we provide. You are in charge of compliance with any dataset license and all other permissions. You are required to navigate datasets homepage and make sure that you can use it. In case of any questions, get in touch with us at hello@datasetninja.com.