Introduction #
The authors of the iSAID: A Large-scale Dataset for Instance Segmentation in Aerial Images dataset have introduced the first benchmark dataset for instance segmentation in aerial imagery, which merges instance-level object detection and pixel-level segmentation tasks. It contains 655,451 object instances spanning 15 different categories across 2,806 high-resolution images. Precise per-pixel annotations have been provided for each instance, ensuring accurate localization for detailed scene analysis. Compared to existing small-scale aerial image-based instance segmentation datasets, iSAID boasts 15 times the number of object categories and 5 times the number of instances.
The authors’ primary goal is to encourage advancements in aerial imagery for Earth observation. While datasets for object detection and semantic labeling have been introduced, they do not offer per-pixel accurate labeling for each object instance in aerial images, making them unsuitable for instance segmentation tasks. Publicly available instance segmentation datasets typically focus on a single object category, limiting their applicability. In contrast, iSAID addresses these limitations by providing a comprehensive and large-scale dataset suitable for real-world applications in complex aerial scenes.
To create this instance segmentation dataset, the authors have utilized the DOTA dataset (also available at dninja) as a basis, which contains 2,806 images collected from multiple sensors and platforms to reduce bias. However, DOTA originally only provided bounding box annotations for object detection, making it unsuitable for accurate instance segmentation. To ensure accuracy and completeness, the iSAID dataset has been independently annotated from scratch, resulting in a substantial increase in the number of instances compared to the original DOTA dataset.
It’s worth noting that instance segmentation in aerial images presents unique challenges compared to standard image datasets. Aerial images typically offer less object detail, smaller sizes, and different viewpoints. Existing aerial image datasets are often annotated with bounding boxes or point labels, which coarsely localize object instances. In contrast, iSAID provides a large number of instances accurately marked with masks, denoting their exact locations in images.
Statistics of classes and instances in iSAID. (a) Histogram of the number of instances per class (sorted by frequency). (b) Histogram of number of instances per image. (c) Histogram of number of classes per image. (d) Number of instances vs. instances per image (comparison of our dataset with other large-scale conventional datasets). The size of the circle denotes the number of categories, e.g., a big circle represents the presence of a large number of object categories.
To ensure the quality and consistency of annotations, the authors have implemented a comprehensive annotation pipeline, including annotation guidelines: 1) All clearly visible objects of the 15 categories must be annotated; 2) Segmentation masks for each instance should match its visual margin in the image; 3) Images should be zoomed in or out, when necessary, to obtain annotations with refined boundaries; 4) Cases of unclear/difficult objects should be reported to the team supervisors and then discussed to get annotations with high confidence; 5) All work should be done at a single facility using the same software. The professional annotators have been trained, and a rigorous assessment protocol has been used to select the best annotators for the task.
Boxplot depicting the range of areas for each object category. The size of objects varies greatly both among and across classes.
Quality control procedures have been applied: 1) The labelers were asked to examine their own annotated images and correct issues like double labels, false labels, missing objects, and inaccurate boundaries. 2) The annotators reviewed the work of other peers on a rotational basis. In this stage, object masks for each class were cropped and placed in one specific directory, so that the annotation errors could be easily identified and corrected. 3) The supervisory team randomly sampled 70% of images (around 2000) and analyzed their quality. 4) A team of experts sampled 20% of images (around 500) and ensured the quality of annotations. In case of problems, the annotations were iteratively sent back to the annotators for refinement until the experts were satisfied with the labels. 5) Finally, several statistics (e.g., instance areas, aspect ratios, etc.) were computed.
Statistics of images and instances in iSAID. (a) The ratio between areas of the largest and smallest object shows a huge variation in scale. (b) shows that instances in iSAID exhibit large variations in aspect ratio.
iSAID statistics reveal its unique properties, such as high spatial resolution (ranges from 800 to 13, 000 in width), a large number of instances per image (655,451 annotated instances of 15 categories), significant variations in object size (objects in the range of 10 to 144 pixels as small, 144 to 1024 pixels as medium, and 1024 and above as large. The percentage of small, medium, and large objects in iSAID is 52.0, 33.7, and 9.7, respectively), with an aspect ratio (reaching up to 90 (2.4 on average)), and the presence of various object categories. These characteristics distinguish iSAID from other existing datasets and emphasize its potential for real-world applications in aerial imagery analysis. The dataset also offers various statistics regarding image resolution, instance count, object areas, and aspect ratios, highlighting its suitability for handling small, medium, and large objects in aerial scenes.
In summary, the authors have created the iSAID dataset, addressing the limitations of existing aerial image datasets and providing a valuable resource for instance segmentation in complex aerial imagery analysis.
Summary #
iSAID: A Large-scale Dataset for Instance Segmentation in Aerial Images is a dataset for instance segmentation, semantic segmentation, and object detection tasks. It is used in the geospatial domain.
The dataset consists of 2806 images with 471760 labeled objects belonging to 15 different classes including small_vehicle, large_vehicle, tennis_court, and other: ground_track_field, ship, harbor, storage_tank, swimming_pool, plane, bridge, roundabout, baseball_diamond, soccer_ball_field, basketball_court, and helicopter.
Images in the iSAID dataset have pixel-level instance segmentation annotations. Due to the nature of the instance segmentation task, it can be automatically transformed into a semantic segmentation (only one mask for every class) or object detection (bounding boxes for every object) tasks. There are 937 (33% of the total) unlabeled images (i.e. without annotations). There are 3 splits in the dataset: train (1411 images), test (937 images), and val (458 images). The dataset was released in 2019 by the Inception.AI, UAE, Wuhan UNiversity, China, and Huazhong University of Science and Technology, China.
Here are the visualized examples for the classes:
Explore #
iSAID dataset has 2806 images. Click on one of the examples below or open "Explore" tool anytime you need to view dataset images with annotations. This tool has extended visualization capabilities like zoom, translation, objects table, custom filters and more. Hover the mouse over the images to hide or show annotations.


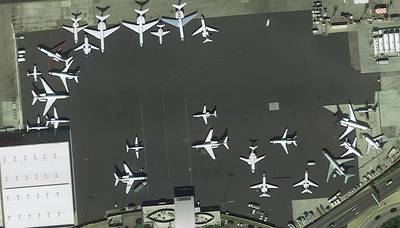
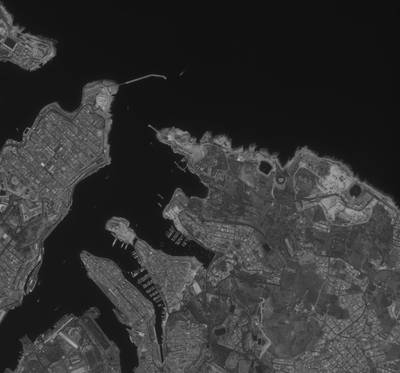

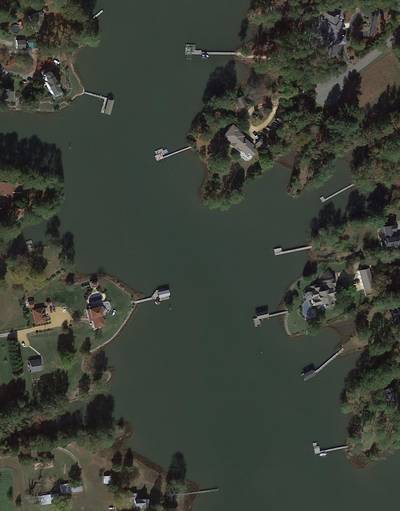
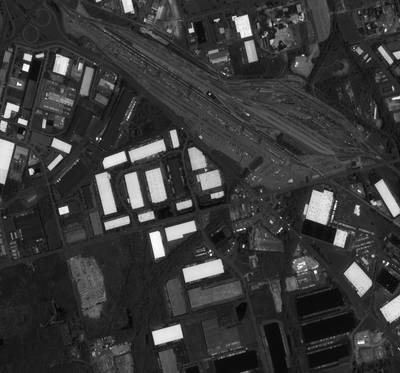


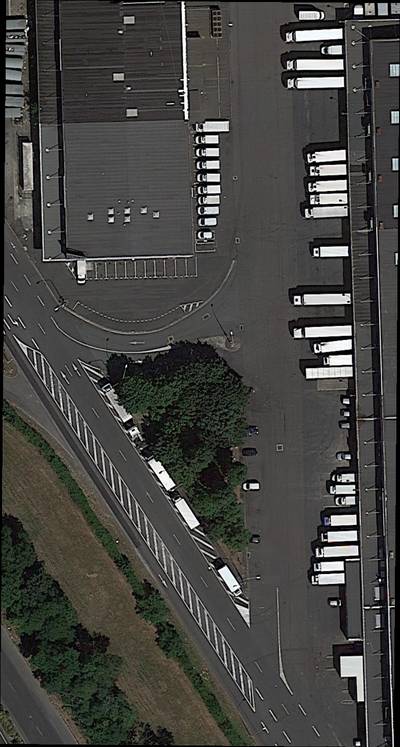

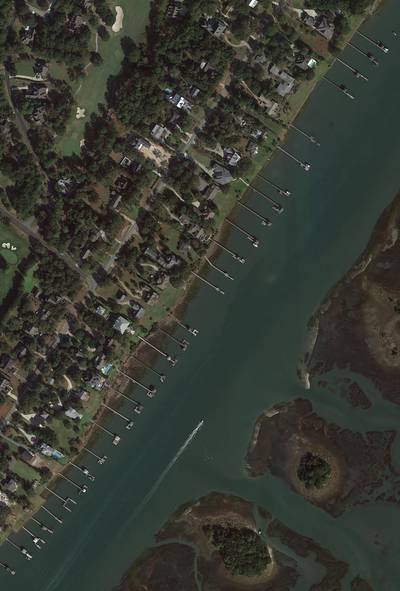



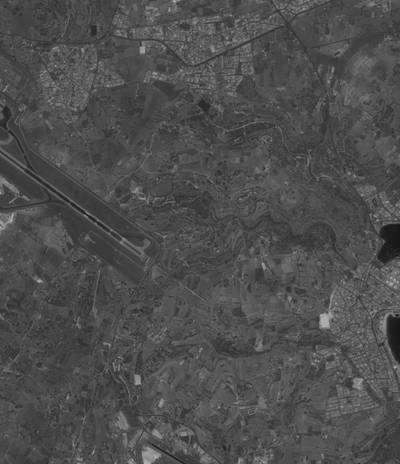



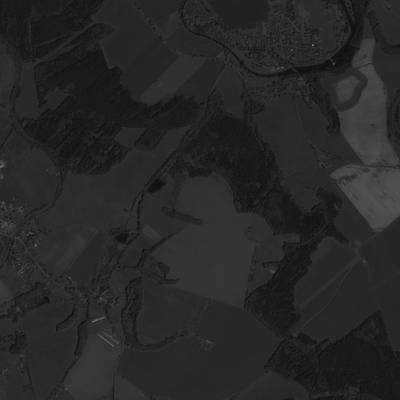
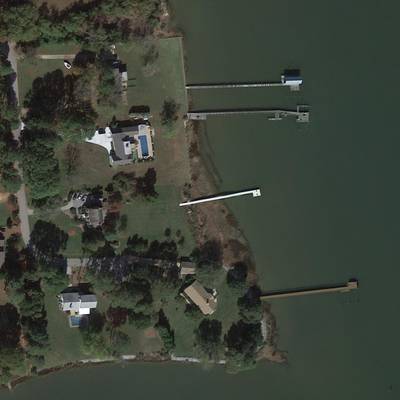









Class balance #
There are 15 annotation classes in the dataset. Find the general statistics and balances for every class in the table below. Click any row to preview images that have labels of the selected class. Sort by column to find the most rare or prevalent classes.
Class ㅤ | Images ㅤ | Objects ㅤ | Count on image average | Area on image average |
---|---|---|---|---|
small_vehicle➔ polygon | 1156 | 253077 | 218.92 | 0.95% |
large_vehicle➔ polygon | 841 | 39874 | 47.41 | 1.7% |
tennis_court➔ polygon | 537 | 11632 | 21.66 | 4.89% |
ground_track_field➔ polygon | 536 | 84456 | 157.57 | 1.98% |
ship➔ polygon | 480 | 35755 | 74.49 | 1.5% |
harbor➔ polygon | 418 | 6492 | 15.53 | 1.67% |
storage_tank➔ polygon | 395 | 18549 | 46.96 | 0.96% |
swimming_pool➔ polygon | 334 | 2605 | 7.8 | 1.11% |
plane➔ polygon | 292 | 8781 | 30.07 | 3.08% |
bridge➔ polygon | 289 | 2272 | 7.86 | 0.27% |
Co-occurrence matrix #
Co-occurrence matrix is an extremely valuable tool that shows you the images for every pair of classes: how many images have objects of both classes at the same time. If you click any cell, you will see those images. We added the tooltip with an explanation for every cell for your convenience, just hover the mouse over a cell to preview the description.
Images #
Explore every single image in the dataset with respect to the number of annotations of each class it has. Click a row to preview selected image. Sort by any column to find anomalies and edge cases. Use horizontal scroll if the table has many columns for a large number of classes in the dataset.
Class sizes #
The table below gives various size properties of objects for every class. Click a row to see the image with annotations of the selected class. Sort columns to find classes with the smallest or largest objects or understand the size differences between classes.
Class | Object count | Avg area | Max area | Min area | Min height | Min height | Max height | Max height | Avg height | Avg height | Min width | Min width | Max width | Max width |
---|---|---|---|---|---|---|---|---|---|---|---|---|---|---|
small_vehicle polygon | 253077 | 0% | 8.19% | 0% | 1px | 0.03% | 405px | 37.05% | 13px | 0.56% | 1px | 0.02% | 455px | 38.02% |
ground_track_field polygon | 84456 | 0.01% | 34.5% | 0% | 1px | 0.03% | 1574px | 77.5% | 14px | 0.59% | 1px | 0.03% | 1585px | 94.18% |
large_vehicle polygon | 39874 | 0.03% | 1.44% | 0% | 1px | 0.04% | 1205px | 46.67% | 36px | 2.02% | 1px | 0.03% | 749px | 31.41% |
ship polygon | 35755 | 0.02% | 15.23% | 0% | 2px | 0.06% | 1651px | 71.38% | 35px | 1.6% | 2px | 0.07% | 1061px | 74.16% |
storage_tank polygon | 18549 | 0.02% | 20.22% | 0% | 1px | 0.04% | 871px | 79.33% | 36px | 1.51% | 1px | 0.04% | 1109px | 76.17% |
tennis_court polygon | 11632 | 0.22% | 6.2% | 0% | 1px | 0.05% | 236px | 39.57% | 61px | 4.65% | 1px | 0.06% | 254px | 42.65% |
plane polygon | 8781 | 0.1% | 3.39% | 0% | 2px | 0.05% | 659px | 37.05% | 84px | 3.43% | 3px | 0.05% | 707px | 32.91% |
harbor polygon | 6492 | 0.1% | 12.95% | 0% | 1px | 0.03% | 2847px | 100% | 127px | 7.88% | 1px | 0.03% | 1939px | 85.95% |
roundabout polygon | 3053 | 0.07% | 13.21% | 0% | 2px | 0.04% | 777px | 41.6% | 88px | 2.99% | 3px | 0.07% | 775px | 43.69% |
helicopter polygon | 2716 | 0.1% | 6.87% | 0% | 1px | 0.04% | 992px | 65.77% | 118px | 7.21% | 1px | 0.03% | 2380px | 82.16% |
Spatial Heatmap #
The heatmaps below give the spatial distributions of all objects for every class. These visualizations provide insights into the most probable and rare object locations on the image. It helps analyze objects' placements in a dataset.
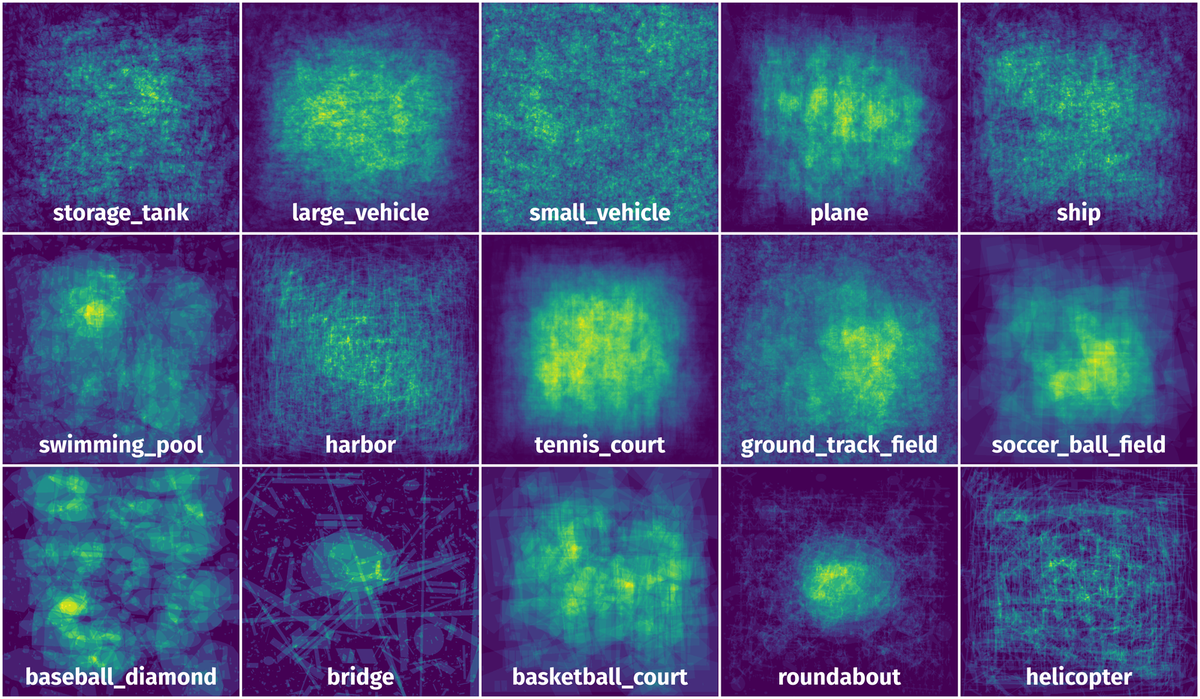
Objects #
Table contains all 86423 objects. Click a row to preview an image with annotations, and use search or pagination to navigate. Sort columns to find outliers in the dataset.
Object ID ㅤ | Class ㅤ | Image name click row to open | Image size height x width | Height ㅤ | Height ㅤ | Width ㅤ | Width ㅤ | Area ㅤ |
---|---|---|---|---|---|---|---|---|
1➔ | large_vehicle polygon | P2029.png | 605 x 387 | 35px | 5.79% | 42px | 10.85% | 0.2% |
2➔ | large_vehicle polygon | P2029.png | 605 x 387 | 42px | 6.94% | 37px | 9.56% | 0.21% |
3➔ | large_vehicle polygon | P2029.png | 605 x 387 | 34px | 5.62% | 43px | 11.11% | 0.18% |
4➔ | large_vehicle polygon | P2029.png | 605 x 387 | 40px | 6.61% | 37px | 9.56% | 0.2% |
5➔ | large_vehicle polygon | P2029.png | 605 x 387 | 41px | 6.78% | 38px | 9.82% | 0.2% |
6➔ | large_vehicle polygon | P2029.png | 605 x 387 | 40px | 6.61% | 38px | 9.82% | 0.19% |
7➔ | large_vehicle polygon | P2029.png | 605 x 387 | 41px | 6.78% | 38px | 9.82% | 0.21% |
8➔ | large_vehicle polygon | P2029.png | 605 x 387 | 33px | 5.45% | 28px | 7.24% | 0.14% |
9➔ | large_vehicle polygon | P2029.png | 605 x 387 | 45px | 7.44% | 41px | 10.59% | 0.23% |
10➔ | large_vehicle polygon | P2029.png | 605 x 387 | 41px | 6.78% | 39px | 10.08% | 0.22% |
License #
The images of iSAID is the same as the DOTA-v1.0 dataset, which are manily collected from the Google Earth, some are taken by satellite JL-1, the others are taken by satellite GF-2 of the China Centre for Resources Satellite Data and Application.
Use of the images from Google Earth must respect the corresponding terms of use: “Google Earth” terms of use.
All images and their associated annotations in iSAID can be used for academic purposes only, but any commercial use is prohibited.
Citation #
If you make use of the iSAID data, please cite the following reference:
@inproceedings{waqas2019isaid,
title={iSAID: A Large-scale Dataset for Instance Segmentation in Aerial Images},
author={Waqas Zamir, Syed and Arora, Aditya and Gupta, Akshita and Khan, Salman and Sun, Guolei and Shahbaz Khan, Fahad and Zhu, Fan and Shao, Ling and Xia, Gui-Song and Bai, Xiang},
booktitle={Proceedings of the IEEE Conference on Computer Vision and Pattern Recognition Workshops},
pages={28--37},
year={2019}
}
@InProceedings{Xia_2018_CVPR,
author = {Xia, Gui-Song and Bai, Xiang and Ding, Jian and Zhu, Zhen and Belongie, Serge and Luo, Jiebo and Datcu, Mihai and Pelillo, Marcello and Zhang, Liangpei},
title = {DOTA: A Large-Scale Dataset for Object Detection in Aerial Images},
booktitle = {The IEEE Conference on Computer Vision and Pattern Recognition (CVPR)},
month = {June},
year = {2018}
}
If you are happy with Dataset Ninja and use provided visualizations and tools in your work, please cite us:
@misc{ visualization-tools-for-isaid-dataset,
title = { Visualization Tools for iSAID Dataset },
type = { Computer Vision Tools },
author = { Dataset Ninja },
howpublished = { \url{ https://datasetninja.com/isaid } },
url = { https://datasetninja.com/isaid },
journal = { Dataset Ninja },
publisher = { Dataset Ninja },
year = { 2025 },
month = { feb },
note = { visited on 2025-02-18 },
}
Download #
Dataset iSAID can be downloaded in Supervisely format:
As an alternative, it can be downloaded with dataset-tools package:
pip install --upgrade dataset-tools
… using following python code:
import dataset_tools as dtools
dtools.download(dataset='iSAID', dst_dir='~/dataset-ninja/')
Make sure not to overlook the python code example available on the Supervisely Developer Portal. It will give you a clear idea of how to effortlessly work with the downloaded dataset.
The data in original format can be downloaded here.
Disclaimer #
Our gal from the legal dep told us we need to post this:
Dataset Ninja provides visualizations and statistics for some datasets that can be found online and can be downloaded by general audience. Dataset Ninja is not a dataset hosting platform and can only be used for informational purposes. The platform does not claim any rights for the original content, including images, videos, annotations and descriptions. Joint publishing is prohibited.
You take full responsibility when you use datasets presented at Dataset Ninja, as well as other information, including visualizations and statistics we provide. You are in charge of compliance with any dataset license and all other permissions. You are required to navigate datasets homepage and make sure that you can use it. In case of any questions, get in touch with us at hello@datasetninja.com.