Introduction #
The authors introduce the OFFSED: Off-Road Semantic Segmentation Dataset, comprising 203 images with full-image semantic segmentation masks. These masks categorically assign one of 19 classes to each pixel. The selection of images was done in a way that captures the whole range of environments and human poses. In addition to pixel labels, a few selected countable classes also come with instance identifiers. This allows for the use of the dataset in instance and panoptic segmentation tasks.
Note, similar OFFSED: Off-Road Semantic Segmentation Dataset dataset is also available on the DatasetNinja.com:
Motivation
In the past decade, significant strides in neural network technology have led to notable advancements in automated driver assistance systems. The ability of self-driving vehicles to navigate their surroundings reliably and autonomously relies on their capacity to infer semantic information about the environment. While a considerable portion of research focuses on private passenger cars and cargo trucks, which typically operate in common environments like paved roads, highways, and cities, it’s crucial to address the unique challenges faced by industrial vehicles such as tractors and excavators. These industrial vehicles constitute a substantial portion of the global motorized vehicle fleet and operate in distinctly different settings.
Current state-of-the-art approaches in semantic full image segmentation predominantly rely on convolutional neural networks. These networks take a monocular RGB image as input and assign a class label to every pixel in the image. Training these networks requires large image datasets with ground truth semantic labels for each pixel. However, existing datasets, primarily focused on private passenger cars and cargo trucks, predominantly feature urban environments or highway roads. These settings are unsuitable for training assistance systems on industrial vehicles and working machines, which operate in semantically and visually distinct surroundings. For instance, the passable ground for a passenger car differs significantly from that of an excavator, encountering different objects than cars in urban environments.
To address this gap, the authors present a OFFSED: Off-Road Semantic Segmentation Dataset, that introduces an off-road variant to the collection of semantic segmentation datasets. This dataset captures off-road environments, including meadows, woods, construction sites, farmland, and paddocks. Pedestrians in various poses, some highly unconventional in the Advanced Driver Assistance Systems (ADAS) context, such as crouching, lying down, or handstands, are depicted to provide extremes for comprehensive training and testing scenarios. Dataset contains 203 images by incorporating full-image semantic segmentation masks, assigning one of 19 classes to each pixel. The selection of images was thoughtfully curated to encompass a diverse range of environments and human poses featured in the original dataset. In addition to pixel labels, certain countable classes also include instance identifiers for enhanced precision.
Full image segmentation masks (right) and their corresponding images (left). Construction sites are common environments for mobile working machines, but are underrepresented in most deep learning datasets.
Dataset creation
The images in this dataset are a subset of the data from authors previous work, OPEDD. It consists of 203 images that were captured in five different environments, meadows, woods, construction sites, farm-land and paddocks. The images were extracted from stereo video sequences recorded with a ZED camera in lossless compression. Manually annotating images for semantic segmentation is a labour intensive and costly task. The ground truth semantic segmentation annotations were created manually with the help of the annotation tool CVAT. In this annotation tool, annotators delineate polygons for each object and assign labels based on a predefined set of 19 classes. Notably, these polygons are endowed with a z-layer level, establishing a depth ordering that streamlines the annotation process by drawing borders between polygons only once, thus optimizing time. Subsequently, full-image pixel masks are generated from these polygons, taking into account the polygon depth.
The selection of classes was carefully curated to mirror potential environments for mobile working machines. Emphasis was placed on specific classes such as drivable and nondrivable surfaces like dirt or pavement/concrete. These classes, crucial for determining the suitability of terrain for mobile working machines, are inherently linked to the ego-vehicle and are susceptible to semantic and visual ambiguity. For instance, a dirt heap traversable by a larger machine may pose an obstacle for a smaller one. Throughout the annotation process, considerations were guided by the context of ADAS for mobile working machines, influencing decisions regarding distinctions between drivable and non-drivable dirt, with a focus on addressing potential challenges arising from semantic and visual nuances.
Note: the authors of the dataset did not provide a way to compare instance identifier with the corresponding image.
Summary #
OFFSED: Off-Road Semantic Segmentation Dataset is a dataset for instance segmentation, semantic segmentation, object detection, identification, and stereo depth estimation tasks. It is used in the automotive industry.
The dataset consists of 406 images with 8346 labeled objects belonging to 20 different classes including obstacle, person, tree, and other: grass, drivable dirt, sky, bush, nondrivable dirt, building, held object, wall, drivable pavement, crops, excavator, nondrivable pavement, car, guard rail, truck, camper, and background.
Images in the OFFSED dataset have pixel-level instance segmentation annotations. Due to the nature of the instance segmentation task, it can be automatically transformed into a semantic segmentation (only one mask for every class) or object detection (bounding boxes for every object) tasks. There are 203 (50% of the total) unlabeled images (i.e. without annotations). There are 2 splits in the dataset: left (203 images) and right (203 images). The dataset was released in 2021 by the University of Kaiserslautern, Germany.
Here are the visualized examples for the classes:
Explore #
OFFSED dataset has 406 images. Click on one of the examples below or open "Explore" tool anytime you need to view dataset images with annotations. This tool has extended visualization capabilities like zoom, translation, objects table, custom filters and more. Hover the mouse over the images to hide or show annotations.
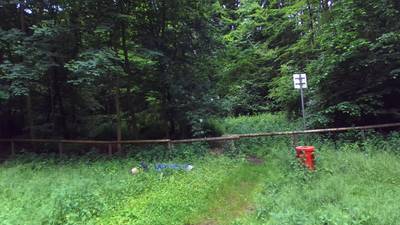

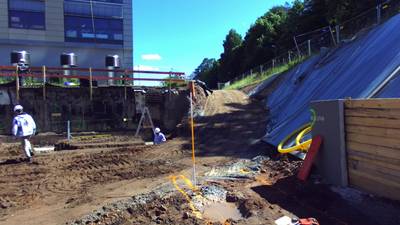
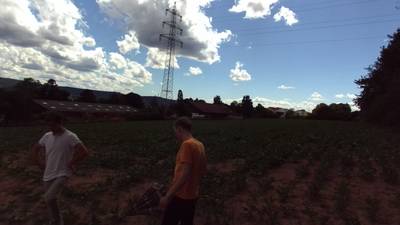

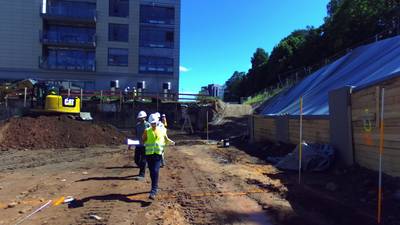

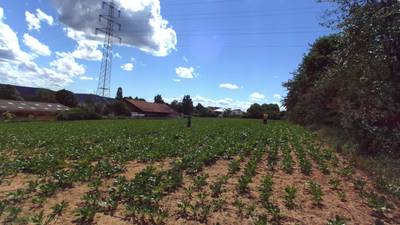
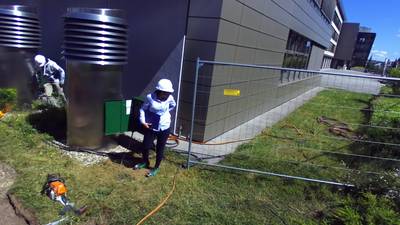
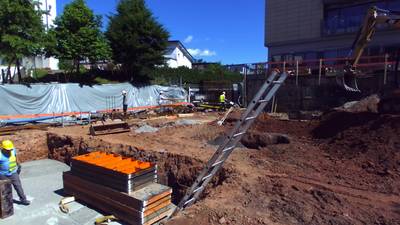
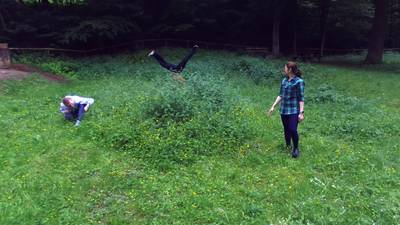
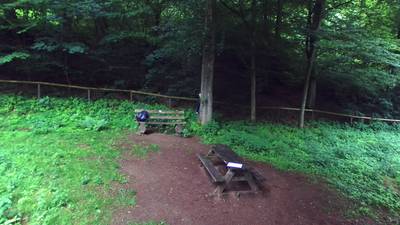
Class balance #
There are 20 annotation classes in the dataset. Find the general statistics and balances for every class in the table below. Click any row to preview images that have labels of the selected class. Sort by column to find the most rare or prevalent classes.
Class ã…¤ | Images ã…¤ | Objects ã…¤ | Count on image average | Area on image average |
---|---|---|---|---|
obstacleâž” mask | 199 | 1045 | 5.25 | 10.46% |
personâž” mask | 198 | 645 | 3.26 | 3.27% |
treeâž” mask | 193 | 1034 | 5.36 | 16.05% |
grassâž” mask | 174 | 906 | 5.21 | 26.98% |
drivable dirtâž” mask | 152 | 627 | 4.12 | 15.98% |
skyâž” mask | 150 | 621 | 4.14 | 17.39% |
bushâž” mask | 122 | 532 | 4.36 | 4.84% |
nondrivable dirtâž” mask | 120 | 840 | 7 | 6.68% |
buildingâž” mask | 109 | 1199 | 11 | 10.67% |
held objectâž” mask | 75 | 123 | 1.64 | 0.45% |
Co-occurrence matrix #
Co-occurrence matrix is an extremely valuable tool that shows you the images for every pair of classes: how many images have objects of both classes at the same time. If you click any cell, you will see those images. We added the tooltip with an explanation for every cell for your convenience, just hover the mouse over a cell to preview the description.
Images #
Explore every single image in the dataset with respect to the number of annotations of each class it has. Click a row to preview selected image. Sort by any column to find anomalies and edge cases. Use horizontal scroll if the table has many columns for a large number of classes in the dataset.
Object distribution #
Interactive heatmap chart for every class with object distribution shows how many images are in the dataset with a certain number of objects of a specific class. Users can click cell and see the list of all corresponding images.
Class sizes #
The table below gives various size properties of objects for every class. Click a row to see the image with annotations of the selected class. Sort columns to find classes with the smallest or largest objects or understand the size differences between classes.
Class | Object count | Avg area | Max area | Min area | Min height | Min height | Max height | Max height | Avg height | Avg height | Min width | Min width | Max width | Max width |
---|---|---|---|---|---|---|---|---|---|---|---|---|---|---|
building mask | 1199 | 0.97% | 53.64% | 0% | 3px | 0.24% | 1242px | 100% | 86px | 6.94% | 1px | 0.05% | 2188px | 99.09% |
obstacle mask | 1045 | 1.99% | 76.49% | 0% | 3px | 0.24% | 1242px | 100% | 199px | 16.03% | 1px | 0.05% | 2208px | 100% |
tree mask | 1034 | 3% | 57.57% | 0% | 2px | 0.16% | 1242px | 100% | 136px | 10.95% | 1px | 0.05% | 2208px | 100% |
grass mask | 906 | 5.18% | 96.05% | 0% | 1px | 0.08% | 1242px | 100% | 170px | 13.68% | 6px | 0.27% | 2208px | 100% |
nondrivable dirt mask | 840 | 0.95% | 67.52% | 0% | 1px | 0.08% | 1242px | 100% | 115px | 9.24% | 5px | 0.23% | 2208px | 100% |
person mask | 645 | 1% | 19.05% | 0% | 6px | 0.48% | 1242px | 100% | 214px | 17.25% | 6px | 0.27% | 851px | 38.54% |
drivable dirt mask | 627 | 3.87% | 58.59% | 0% | 3px | 0.24% | 1242px | 100% | 204px | 16.46% | 3px | 0.14% | 2208px | 100% |
sky mask | 621 | 4.2% | 60.11% | 0% | 1px | 0.08% | 1242px | 100% | 135px | 10.87% | 2px | 0.09% | 2208px | 100% |
bush mask | 532 | 1.11% | 24.37% | 0% | 4px | 0.32% | 893px | 71.9% | 95px | 7.63% | 7px | 0.32% | 2208px | 100% |
wall mask | 218 | 1.74% | 27.06% | 0% | 5px | 0.4% | 597px | 48.07% | 142px | 11.4% | 6px | 0.27% | 1756px | 79.53% |
Spatial Heatmap #
The heatmaps below give the spatial distributions of all objects for every class. These visualizations provide insights into the most probable and rare object locations on the image. It helps analyze objects' placements in a dataset.
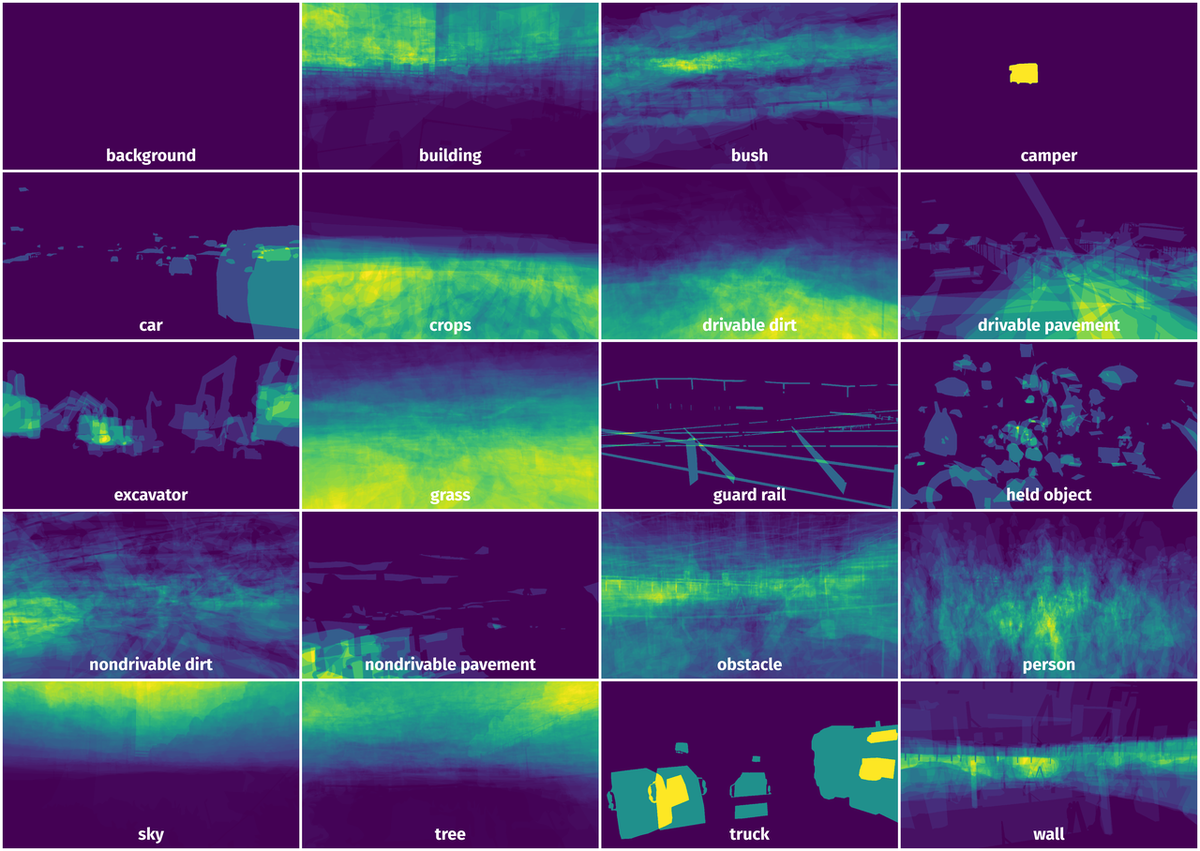
Objects #
Table contains all 8346 objects. Click a row to preview an image with annotations, and use search or pagination to navigate. Sort columns to find outliers in the dataset.
Object ID ã…¤ | Class ã…¤ | Image name click row to open | Image size height x width | Height ã…¤ | Height ã…¤ | Width ã…¤ | Width ã…¤ | Area ã…¤ |
---|---|---|---|---|---|---|---|---|
1âž” | grass mask | 519.png | 1242 x 2208 | 229px | 18.44% | 632px | 28.62% | 1.16% |
2âž” | grass mask | 519.png | 1242 x 2208 | 47px | 3.78% | 165px | 7.47% | 0.16% |
3âž” | grass mask | 519.png | 1242 x 2208 | 42px | 3.38% | 113px | 5.12% | 0.09% |
4âž” | grass mask | 519.png | 1242 x 2208 | 40px | 3.22% | 90px | 4.08% | 0.1% |
5âž” | building mask | 519.png | 1242 x 2208 | 396px | 31.88% | 1008px | 45.65% | 13.46% |
6âž” | building mask | 519.png | 1242 x 2208 | 37px | 2.98% | 76px | 3.44% | 0.07% |
7âž” | building mask | 519.png | 1242 x 2208 | 99px | 7.97% | 171px | 7.74% | 0.45% |
8âž” | building mask | 519.png | 1242 x 2208 | 22px | 1.77% | 76px | 3.44% | 0.05% |
9âž” | building mask | 519.png | 1242 x 2208 | 84px | 6.76% | 212px | 9.6% | 0.21% |
10âž” | building mask | 519.png | 1242 x 2208 | 26px | 2.09% | 76px | 3.44% | 0.07% |
License #
License is unknown for the OFFSED: Off-Road Semantic Segmentation Dataset dataset.
Citation #
If you make use of the OFFSED data, please cite the following reference:
@INPROCEEDINGS{9551643,
author={Viswanath, Kasi and Singh, Kartikeya and Jiang, Peng and Sujit, P.B. and Saripalli, Srikanth},
booktitle={2021 IEEE 17th International Conference on Automation Science and Engineering (CASE)},
title={OFFSEG: A Semantic Segmentation Framework For Off-Road Driving},
year={2021},
volume={},
number={},
pages={354-359},
doi={10.1109/CASE49439.2021.9551643}}
If you are happy with Dataset Ninja and use provided visualizations and tools in your work, please cite us:
@misc{ visualization-tools-for-offsed-dataset,
title = { Visualization Tools for OFFSED Dataset },
type = { Computer Vision Tools },
author = { Dataset Ninja },
howpublished = { \url{ https://datasetninja.com/offsed } },
url = { https://datasetninja.com/offsed },
journal = { Dataset Ninja },
publisher = { Dataset Ninja },
year = { 2025 },
month = { mar },
note = { visited on 2025-03-26 },
}
Download #
Please visit dataset homepage to download the data.
Disclaimer #
Our gal from the legal dep told us we need to post this:
Dataset Ninja provides visualizations and statistics for some datasets that can be found online and can be downloaded by general audience. Dataset Ninja is not a dataset hosting platform and can only be used for informational purposes. The platform does not claim any rights for the original content, including images, videos, annotations and descriptions. Joint publishing is prohibited.
You take full responsibility when you use datasets presented at Dataset Ninja, as well as other information, including visualizations and statistics we provide. You are in charge of compliance with any dataset license and all other permissions. You are required to navigate datasets homepage and make sure that you can use it. In case of any questions, get in touch with us at hello@datasetninja.com.