Introduction #
The S2TLD: Small Traffic Light Dataset is the traffic light dataset. The scenes cover a variety of lighting, weather and traffic conditions, including busy street scenes inner-city, dense stop-and-go traffic, strong changes in illumination/exposure, flickering/fluctuating traffic lights, multiple visible traffic lights, images parts that can be confused with traffic lights.
Note, similar S2TLD: Small Traffic Light Dataset datasets are also available on the DatasetNinja.com:
Motivation
Reliable traffic light detection and classification is crucial for automated driving in urban environments. Currently, there are no systems that can reliably perceive traffic lights in real-time, without map-based information, and in sufficient distances needed for smooth urban driving.
Dataset description
The S2TLD contains 5,786 images of approximately 1, 080 × 1, 920 pixels (1,222 images) and 720 × 1, 280 pixels (4,564 images). It also contains 5 categories (namely red, yellow, green, off and wait on) of 14,130 instances. The scenes cover a variety of lighting, weather and traffic conditions, including busy street scenes inner city, dense stop-and-go traffic, strong changes in illumination/exposure, flickering/fluctuating traffic lights, multiple visible traffic lights, image parts that can be confused with traffic lights (e.g. large round tail lights).
Illustrations of the five categories and different lighting and weather conditions in collected S2TLD dataset.
Summary #
S2TLD: SJTU Small Traffic Light Dataset is a dataset for an object detection task. It is used in the automotive industry.
The dataset consists of 5786 images with 14130 labeled objects belonging to 5 different classes including red, green, off, and other: wait on and yellow.
Images in the S2TLD dataset have bounding box annotations. There are 3 (0% of the total) unlabeled images (i.e. without annotations). There are no pre-defined train/val/test splits in the dataset. Alternatively, the dataset could be split into 2 normal: normal 2 (3785 images) and normal 1 (779 images), or into 2 resolution: 720x1280 (4564 images) and 1080x1920 (1222 images). The dataset was released in 2022 by the Shanghai Jiao Tong University, China and Anhui University, China.
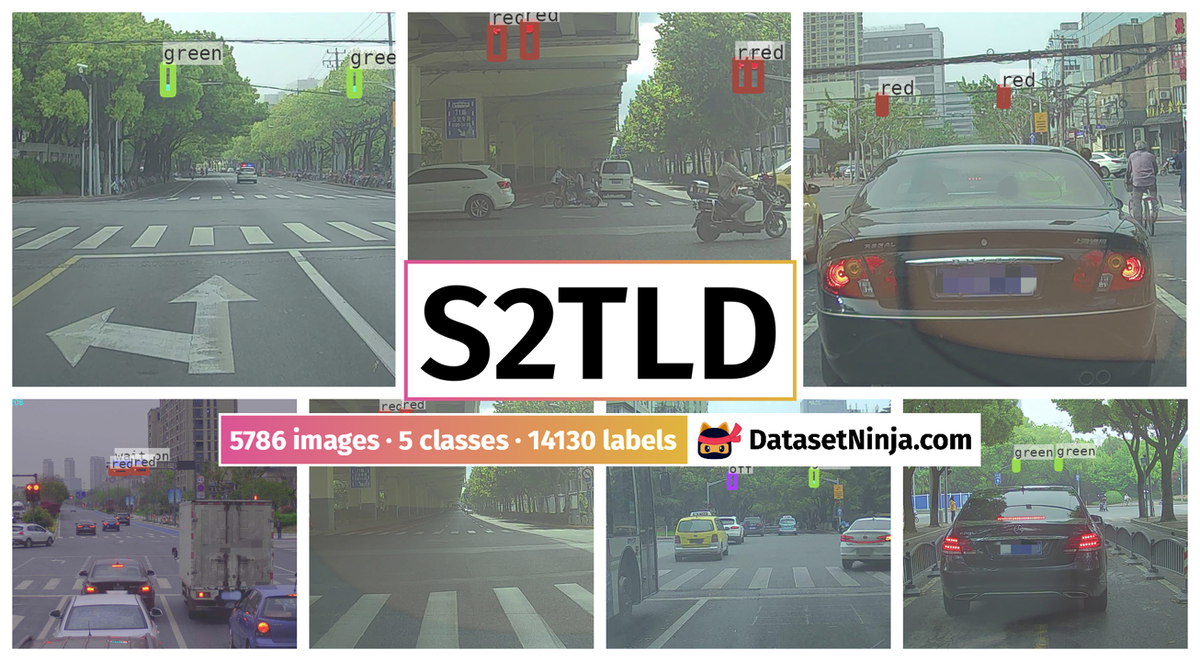
Explore #
S2TLD dataset has 5786 images. Click on one of the examples below or open "Explore" tool anytime you need to view dataset images with annotations. This tool has extended visualization capabilities like zoom, translation, objects table, custom filters and more. Hover the mouse over the images to hide or show annotations.
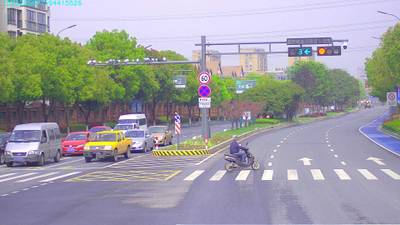
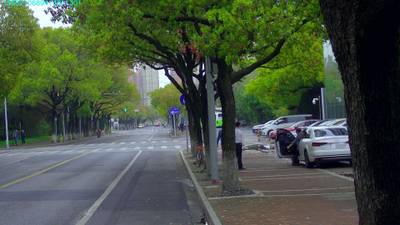
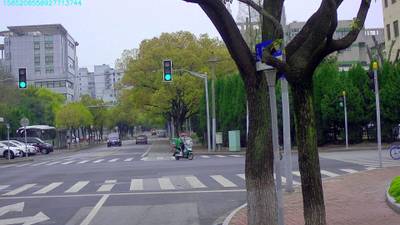


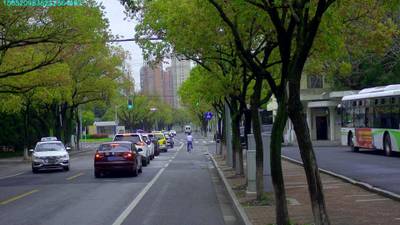
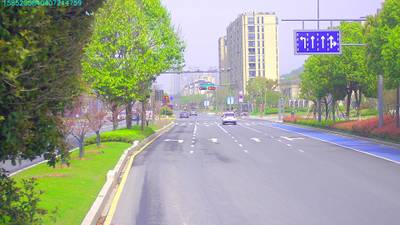
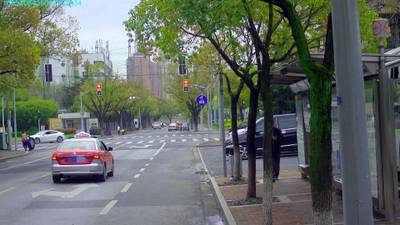
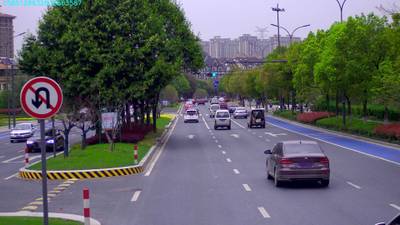
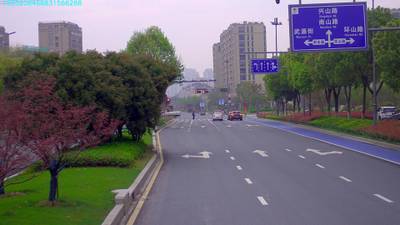
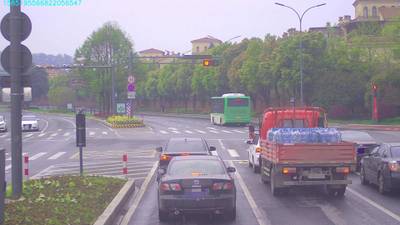
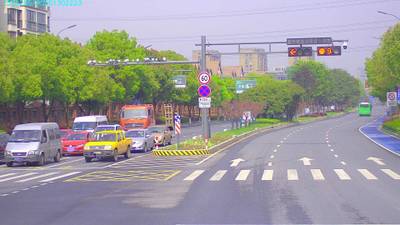
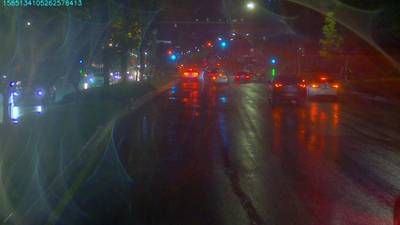
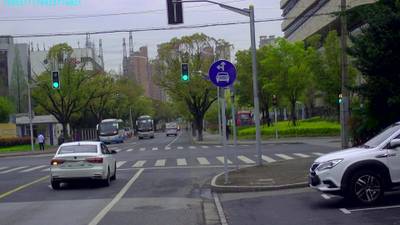
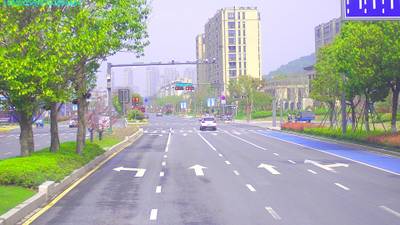
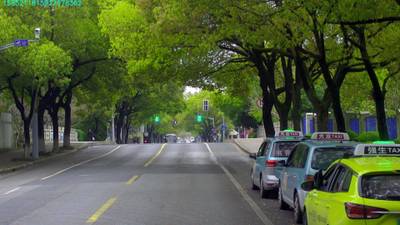
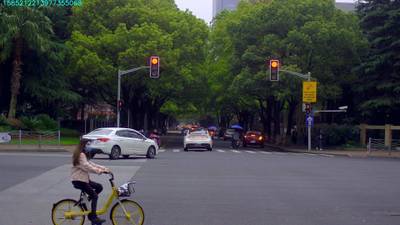

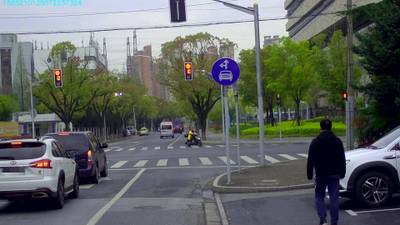
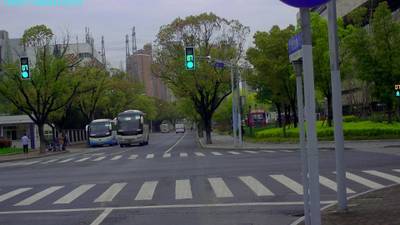
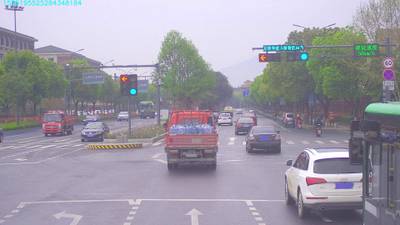
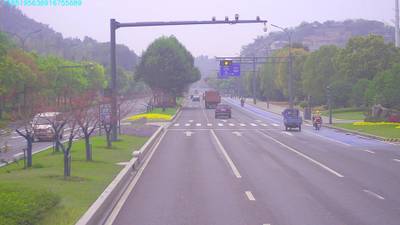
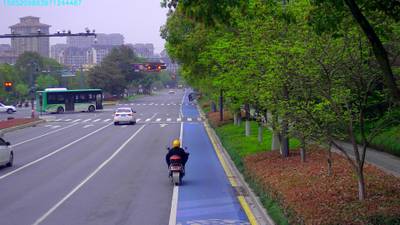
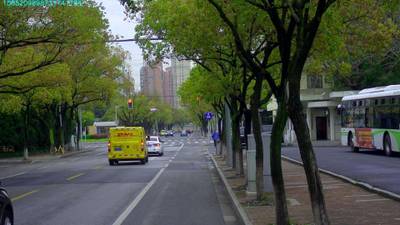
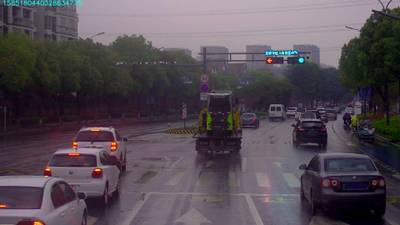
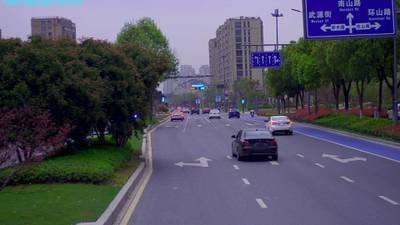
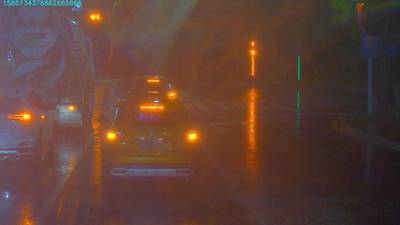
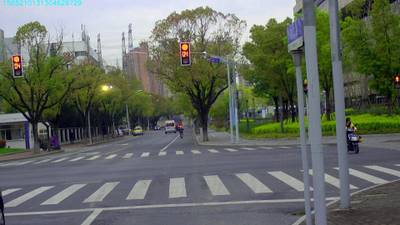
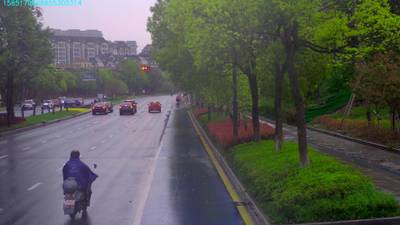
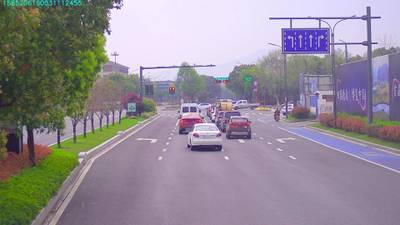
Class balance #
There are 5 annotation classes in the dataset. Find the general statistics and balances for every class in the table below. Click any row to preview images that have labels of the selected class. Sort by column to find the most rare or prevalent classes.
Class ã…¤ | Images ã…¤ | Objects ã…¤ | Count on image average | Area on image average |
---|---|---|---|---|
redâž” rectangle | 3777 | 7708 | 2.04 | 0.26% |
greenâž” rectangle | 2931 | 5344 | 1.82 | 0.28% |
offâž” rectangle | 328 | 507 | 1.55 | 0.18% |
wait onâž” rectangle | 305 | 306 | 1 | 0.15% |
yellowâž” rectangle | 176 | 265 | 1.51 | 0.22% |
Co-occurrence matrix #
Co-occurrence matrix is an extremely valuable tool that shows you the images for every pair of classes: how many images have objects of both classes at the same time. If you click any cell, you will see those images. We added the tooltip with an explanation for every cell for your convenience, just hover the mouse over a cell to preview the description.
Images #
Explore every single image in the dataset with respect to the number of annotations of each class it has. Click a row to preview selected image. Sort by any column to find anomalies and edge cases. Use horizontal scroll if the table has many columns for a large number of classes in the dataset.
Object distribution #
Interactive heatmap chart for every class with object distribution shows how many images are in the dataset with a certain number of objects of a specific class. Users can click cell and see the list of all corresponding images.
Class sizes #
The table below gives various size properties of objects for every class. Click a row to see the image with annotations of the selected class. Sort columns to find classes with the smallest or largest objects or understand the size differences between classes.
Class | Object count | Avg area | Max area | Min area | Min height | Min height | Max height | Max height | Avg height | Avg height | Min width | Min width | Max width | Max width |
---|---|---|---|---|---|---|---|---|---|---|---|---|---|---|
red rectangle | 7708 | 0.13% | 2.16% | 0% | 1px | 0.09% | 220px | 30.56% | 48px | 6.29% | 6px | 0.36% | 209px | 10.89% |
green rectangle | 5344 | 0.15% | 3.5% | 0.01% | 7px | 0.83% | 273px | 37.92% | 51px | 6.78% | 6px | 0.47% | 177px | 9.22% |
off rectangle | 507 | 0.12% | 1.54% | 0.01% | 14px | 1.48% | 185px | 25.69% | 46px | 6.39% | 6px | 0.47% | 115px | 6.25% |
wait on rectangle | 306 | 0.15% | 0.78% | 0.02% | 9px | 0.83% | 56px | 5.19% | 22px | 2.06% | 36px | 1.88% | 321px | 16.72% |
yellow rectangle | 265 | 0.15% | 1.26% | 0.01% | 11px | 1.02% | 171px | 22.64% | 50px | 6.42% | 8px | 0.62% | 142px | 7.4% |
Spatial Heatmap #
The heatmaps below give the spatial distributions of all objects for every class. These visualizations provide insights into the most probable and rare object locations on the image. It helps analyze objects' placements in a dataset.
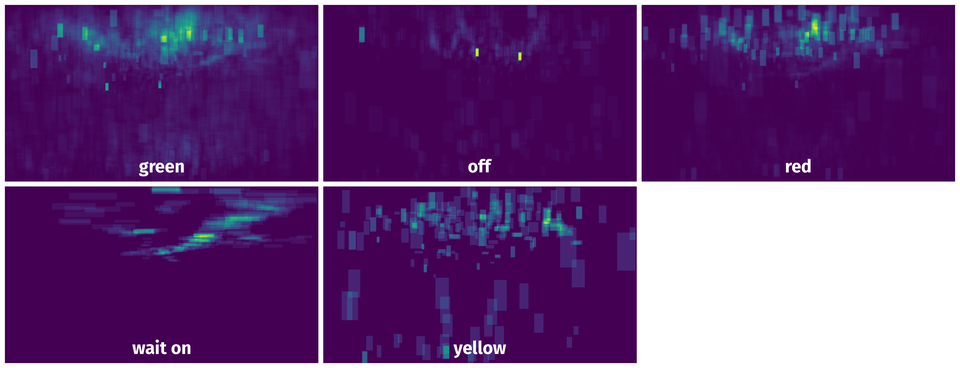
Objects #
Table contains all 14130 objects. Click a row to preview an image with annotations, and use search or pagination to navigate. Sort columns to find outliers in the dataset.
Object ID ã…¤ | Class ã…¤ | Image name click row to open | Image size height x width | Height ã…¤ | Height ã…¤ | Width ã…¤ | Width ã…¤ | Area ã…¤ |
---|---|---|---|---|---|---|---|---|
1âž” | red rectangle | 002471.jpg | 720 x 1280 | 68px | 9.44% | 26px | 2.03% | 0.19% |
2âž” | red rectangle | 002471.jpg | 720 x 1280 | 69px | 9.58% | 26px | 2.03% | 0.19% |
3âž” | red rectangle | 002471.jpg | 720 x 1280 | 59px | 8.19% | 24px | 1.88% | 0.15% |
4âž” | red rectangle | 002471.jpg | 720 x 1280 | 59px | 8.19% | 23px | 1.8% | 0.15% |
5âž” | red rectangle | 003633.jpg | 720 x 1280 | 66px | 9.17% | 26px | 2.03% | 0.19% |
6âž” | green rectangle | 003633.jpg | 720 x 1280 | 68px | 9.44% | 26px | 2.03% | 0.19% |
7âž” | green rectangle | 003633.jpg | 720 x 1280 | 51px | 7.08% | 18px | 1.41% | 0.1% |
8âž” | red rectangle | 003633.jpg | 720 x 1280 | 47px | 6.53% | 19px | 1.48% | 0.1% |
9âž” | wait on rectangle | 2020-04-04 11_13_00.777872252.jpg | 1080 x 1920 | 36px | 3.33% | 321px | 16.72% | 0.56% |
10âž” | green rectangle | 2020-04-04 11_13_00.777872252.jpg | 1080 x 1920 | 66px | 6.11% | 168px | 8.75% | 0.53% |
License #
Citation #
If you make use of the S2TLD data, please cite the following reference:
@article{yang2022scrdet++,
title={Scrdet++: Detecting small, cluttered and rotated objects via instance-level feature denoising and rotation loss smoothing},
author={Yang, Xue and Yan, Junchi and Liao, Wenlong and Yang, Xiaokang and Tang, Jin and He, Tao},
journal={IEEE Transactions on Pattern Analysis and Machine Intelligence},
year={2022},
publisher={IEEE}
}
If you are happy with Dataset Ninja and use provided visualizations and tools in your work, please cite us:
@misc{ visualization-tools-for-s2tld-dataset,
title = { Visualization Tools for S2TLD Dataset },
type = { Computer Vision Tools },
author = { Dataset Ninja },
howpublished = { \url{ https://datasetninja.com/s2tld } },
url = { https://datasetninja.com/s2tld },
journal = { Dataset Ninja },
publisher = { Dataset Ninja },
year = { 2025 },
month = { may },
note = { visited on 2025-05-18 },
}
Download #
Dataset S2TLD can be downloaded in Supervisely format:
As an alternative, it can be downloaded with dataset-tools package:
pip install --upgrade dataset-tools
… using following python code:
import dataset_tools as dtools
dtools.download(dataset='S2TLD', dst_dir='~/dataset-ninja/')
Make sure not to overlook the python code example available on the Supervisely Developer Portal. It will give you a clear idea of how to effortlessly work with the downloaded dataset.
The data in original format can be downloaded here:
Disclaimer #
Our gal from the legal dep told us we need to post this:
Dataset Ninja provides visualizations and statistics for some datasets that can be found online and can be downloaded by general audience. Dataset Ninja is not a dataset hosting platform and can only be used for informational purposes. The platform does not claim any rights for the original content, including images, videos, annotations and descriptions. Joint publishing is prohibited.
You take full responsibility when you use datasets presented at Dataset Ninja, as well as other information, including visualizations and statistics we provide. You are in charge of compliance with any dataset license and all other permissions. You are required to navigate datasets homepage and make sure that you can use it. In case of any questions, get in touch with us at hello@datasetninja.com.